A novel nomogram predicting cancer-specific survival in small cell lung cancer patients with brain metastasis
IntroductionOther Section
Lung cancer is one of the most common malignancies as well as the leading cause of cancer-related death among both men and women in the United States (US), which can be categorized into non-small cell lung cancer (NSCLC) and small cell lung cancer (SCLC) (1). SCLC accounts for approximately 15% of all the lung cancer (2). Each year, SCLC comprises an estimated 250,000 new cases and more than 200,000 deaths all over the world (3). The prognosis for SCLC patients remains very poor, with a 5-year survival rate of less than 7% and an average survival time of only about 10 months (4).
Brain metastasis (BM) is common in patients with SCLC. It is estimated that 10% to 20% of patients with SCLC will have at least one BM at the time of diagnosis (5). BM has become a serious threatening factor for patient’s survival. The standard therapy for SCLC patients who initially present with BM have been limited to systemic chemotherapy and whole brain radiotherapy (6). In recent studies, lung cancer patients with BM can also benefit from molecularly targeted therapy and immunotherapy (7-9). However, long-term survival is still rare in patients with extensive-stage disease. It is crucial to identify the prognostic factors for SCLC patients with BM. Accurate estimation of each patient’s prognosis can benefit both the patient and the physician in all aspects of decision-making (10).
However, there are very few studies on the prognostic factors of survival in SCLC patients with BM, especially population-based study. Reddy et al. reported that lack of insurance, male sex, older age, larger tumor size, liver metastasis and lung metastasis were associated with an increased risk of mortality among SCLC patients with BM, while Asian patients had a lower risk of mortality (6). Shan et al. found that age, sex, race, marital status, T stage and N stage were independent risk factors for prognosis in SCLC patients with BM (11), but specific metastasis and treatment information were not included in this research. Therefore, it is very important to develop an accurate as well as effective predictive model for prognosis of SCLC patients with BM.
Nomograms are statistical prediction tools that incorporate the contribution of each factor and have shown favorable efficiency in predicting survival outcomes for the individual in various cancers (12-14). In this study, we analyzed the prognostic factors for SCLC patients with BM from the Surveillance, Epidemiology, and End Results (SEER) database, which covers approximately 30% of the US population (15). Furthermore, a novel nomogram to predict the cancer-specific survival (CSS) of SCLC patients with BM was developed and validated. We present the following article in accordance with the TRIPOD reporting checklist (available at https://tcr.amegroups.com/article/view/10.21037/tcr-22-1561/rc).
MethodsOther Section
Patients
Data of SCLC patients with BM diagnosed between 2010 and 2015 were obtained from the SEER database using the SEER*Stat software version 8.3.9. The inclusion criteria were as follows: (I) International Classification of Diseases for Oncology Third Edition (ICD-O-3) histology codes (8002/3; 8041/3, 8042/3, 8043/3, 8044/3 and 8045/3) and site codes (C34.0, C34.1, C34.2, C34.3, C34.8, and C34.9); (II) SCLC was the only primary tumor; (III) patients were initially diagnosed with SCLC with BM; (IV) diagnostic confirmation was based on pathological examination. The exclusion criteria were as follows: (I) patients with incomplete survival data or survival time <1 month; (II) age at diagnosis <18 years; (III) lack of important clinicopathological information, such as race, marital status, tumor, node, metastasis (TNM) stage and surgery of primary site. The study was conducted in accordance with the Declaration of Helsinki (as revised in 2013). Institutional review board approval and informed consent were not required for this study because SEER database is available and free for public.
Study variables
The demographic and clinicopathological variables included age, sex (female or male), race (white, black, or other), marital status (married or unmarried), primary site (upper lobe, middle lobe, lower lobe, main bronchus or other), laterality (left, right or other), grade (I/II, III/IV or unknown), T stage (T1, T2, T3, or T4), N stage (N0, N1, N2, or N3), surgery of primary site (yes or no), radiation (yes or no/unknown), chemotherapy (yes or no/unknown), bone metastasis (yes or no), liver metastasis (yes or no), lung metastasis (yes or no), survival months and cancer-specific death. Age was converted into categorical variables according to the cutoff values determined by the X-tile software. The optimal cutoff values for age were 65 and 76 years, and age was divided into <66, 66–76 or >76 years groups. CSS was defined as the time from diagnosis to death due to cancer. The 7th edition of the TNM stage was used as the stage system.
Statistical analysis
The patients that we finally included were randomly divided into training cohort (70%) and validation cohort (30%). A univariate Cox regression analysis was firstly performed in the training cohort. The variables that were considered clinically relevant or that showed a univariate relationship with outcome were then subjected to a multivariate Cox regression analysis to identify independent prognostic factors (16). These identified factors were finally applied to construct the prognostic nomogram to predict 6-, 9- and 12-month CSS (17). The concordance index (C-index) was used to evaluate the discriminative ability of the nomogram. The receiver operating characteristic (ROC) curve was used to assess the predictive accuracy by calculating the area under ROC curve (AUC). Calibration plot was established to reveal the relationship between predicted probability and actual probability. The closer the calibration curve is to the diagonal, the more accurate the prediction of model is to the actuality. The clinical usefulness of nomogram was evaluated through decision curve analysis (DCA). In addition, the prognosis of patients between groups were compared with Kaplan-Meier survival analysis with the log-rank test.
The chi-square test was used to compare categorical variables between different groups. Statistical analysis was performed using the SPSS software version 22. The nomogram, ROC curves, calibration plots and DCA plots were constructed using the R software version 4.0.3. A two-tailed P value <0.05 was considered as statistically significant.
ResultsOther Section
Characteristics of patients
Finally, a total of 2,462 SCLC patients with BM diagnosed between 2010 and 2015 were included in our research. The flowchart of patient selection could be viewed in Figure 1. Eligible patients were randomly divided into training cohort (n=1,723) and validation cohort (n=739). The baseline demographic and clinicopathological characteristics of the study population were shown in Table 1. There was no statistical difference in each variable between the two groups.
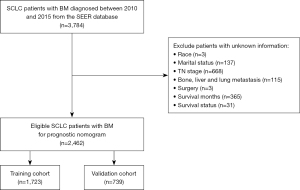
Table 1
Variables | Whole cohort (n=2,462) | Training cohort (n=1,723) | Validation cohort (n=739) | P value |
---|---|---|---|---|
Age (years) | 0.799 | |||
<66 | 1,298 (52.7) | 916 (53.2) | 382 (51.7) | |
66–76 | 906 (36.8) | 628 (36.4) | 278 (37.6) | |
>76 | 258 (10.5) | 179 (10.4) | 79 (10.7) | |
Sex | 0.476 | |||
Female | 1,163 (47.2) | 822 (47.7) | 341 (46.1) | |
Male | 1,299 (52.8) | 901 (52.3) | 398 (53.9) | |
Race | 0.859 | |||
White | 2,075 (84.3) | 1,453 (84.3) | 622 (84.2) | |
Black | 272 (11.0) | 192 (11.1) | 80 (10.8) | |
Other | 115 (4.7) | 78 (4.5) | 37 (5.0) | |
Marital status | 0.508 | |||
Married | 1,311 (53.2) | 925 (53.7) | 386 (52.2) | |
Unmarried | 1,151 (46.8) | 798 (46.3) | 353 (47.8) | |
Primary site | 0.106 | |||
Upper lobe | 1,287 (52.3) | 916 (53.2) | 371 (50.2) | |
Middle lobe | 93 (3.8) | 60 (3.5) | 33 (4.5) | |
Lower lobe | 528 (21.4) | 378 (21.9) | 150 (20.3) | |
Main bronchus | 260 (10.6) | 166 (9.6) | 94 (12.7) | |
Other | 294 (11.9) | 203 (11.8) | 91 (12.3) | |
Laterality | 0.619 | |||
Left | 1,062 (43.1) | 740 (42.9) | 322 (43.6) | |
Right | 1,324 (53.8) | 926 (53.7) | 398 (53.9) | |
Other | 76 (3.1) | 57 (3.3) | 19 (2.6) | |
Grade | 0.952 | |||
I/II | 11 (0.4) | 8 (0.5) | 3 (0.4) | |
III/IV | 594 (24.1) | 418 (24.3) | 176 (23.8) | |
Unknown | 1,857 (75.4) | 1,297 (75.3) | 560 (75.8) | |
T stage | 0.587 | |||
T1 | 265 (10.8) | 187 (10.9) | 78 (10.6) | |
T2 | 646 (26.2) | 465 (27.0) | 181 (24.5) | |
T3 | 555 (22.5) | 382 (22.2) | 173 (23.4) | |
T4 | 996 (40.5) | 689 (40.0) | 307 (41.5) | |
N stage | 0.811 | |||
N0 | 370 (15.0) | 257 (14.9) | 113 (15.3) | |
N1 | 193 (7.8) | 141 (8.2) | 52 (7.0) | |
N2 | 1342 (54.5) | 937 (54.4) | 405 (54.8) | |
N3 | 557 (22.6) | 388 (22.5) | 169 (22.9) | |
Surgery | 0.676 | |||
No | 2,429 (98.7) | 1,701 (98.7) | 728 (98.5) | |
Yes | 33 (1.3) | 22 (1.3) | 11 (1.5) | |
Radiation | 0.331 | |||
No/unknown | 564 (22.9) | 404 (23.4) | 160 (21.7) | |
Yes | 1,898 (77.1) | 1,319 (76.6) | 579 (78.3) | |
Chemotherapy | 0.204 | |||
No/unknown | 519 (21.1) | 375 (21.8) | 144 (19.5) | |
Yes | 1,943 (78.9) | 1,348 (78.2) | 595 (80.5) | |
Bone metastasis | 0.415 | |||
No | 1,780 (72.3) | 1,254 (72.8) | 526 (71.2) | |
Yes | 682 (27.7) | 469 (27.2) | 213 (28.8) | |
Liver metastasis | 0.180 | |||
No | 1,706 (69.3) | 1,208 (70.1) | 498 (67.4) | |
Yes | 756 (30.7) | 515 (29.9) | 241 (32.6) | |
Lung metastasis | 0.823 | |||
No | 2,009 (81.6) | 1,404 (81.5) | 605 (81.9) | |
Yes | 453 (18.4) | 319 (18.5) | 134 (18.1) |
Data are expressed as n (%). SCLC, small cell lung cancer; BM, brain metastasis.
Among all patients, the median follow-up time was 6 months (range, 1–83 months), and 2,292 (93.1%) cancer-specific deaths were identified. For sex, 1,163 (47.2%) were female and 1,299 (52.8%) were male. The most common primary site was upper lobe of lung (52.3%). There were 682 (27.7%), 756 (30.7%) and 453 (18.4%) of patients combined with bone, liver and lung metastasis, respectively.
Independent prognostic factors in the training cohort
Univariate Cox regression analyses showed that age, primary site, N stage, surgery, radiation, chemotherapy, bone metastasis, liver metastasis and lung metastasis were significantly related to CSS in SCLC patients with BM (Table 2). These identified variables combined with other clinically relevant factors (marital status, grade and T stage) were then subjected to the multivariate Cox regression analysis. We found that age, N stage, surgery, radiation, chemotherapy, bone metastasis, liver metastasis and lung metastasis were independent prognostic factors of CSS (Table 2).
Table 2
Variables | Univariate Cox analysis | Multivariate Cox analysis | |||||
---|---|---|---|---|---|---|---|
HR | 95% CI | P value | HR | 95% CI | P value | ||
Age (years) | |||||||
<66 | Reference | – | – | Reference | – | – | |
66–76 | 1.294 | 1.165–1.437 | <0.001 | 1.254 | 1.128–1.394 | <0.001 | |
>76 | 1.884 | 1.599–2.221 | <0.001 | 1.548 | 1.305–1.837 | <0.001 | |
Sex | |||||||
Female | Reference | – | – | – | – | – | |
Male | 1.055 | 0.957–1.164 | 0.281 | – | – | – | |
Race | |||||||
White | Reference | – | – | – | – | – | |
Black | 0.944 | 0.807–1.104 | 0.468 | – | – | – | |
Other | 0.798 | 0.634–1.006 | 0.056 | – | – | – | |
Marital status | |||||||
Married | Reference | – | – | Reference | – | – | |
Unmarried | 1.099 | 0.997–1.213 | 0.057 | 1.042 | 0.944–1.152 | 0.413 | |
Primary site | |||||||
Upper lobe | Reference | – | – | Reference | – | – | |
Middle lobe | 0.921 | 0.704–1.204 | 0.548 | 0.983 | 0.749–1.290 | 0.904 | |
Lower lobe | 1.142 | 1.008–1.293 | 0.036 | 1.022 | 0.901–1.162 | 0.728 | |
Main bronchus | 1.068 | 0.901–1.268 | 0.447 | 0.995 | 0.837–1.182 | 0.954 | |
Other | 1.185 | 1.013–1.386 | 0.033 | 1.016 | 0.865–1.193 | 0.845 | |
Laterality | |||||||
Left | Reference | – | – | – | – | – | |
Right | 1.014 | 0.918–1.121 | 0.778 | – | – | – | |
Other | 1.226 | 0.926–1.621 | 0.154 | – | – | – | |
Grade | |||||||
I/II | Reference | – | – | Reference | – | – | |
III/IV | 1.086 | 0.513–2.296 | 0.830 | 1.734 | 0.810–3.712 | 0.156 | |
Unknown | 1.181 | 0.561–2.487 | 0.662 | 1.801 | 0.845–3.837 | 0.127 | |
T stage | |||||||
T1 | Reference | – | – | Reference | – | – | |
T2 | 0.945 | 0.794–1.125 | 0.525 | 0.954 | 0.800–1.137 | 0.601 | |
T3 | 1.166 | 0.974–1.396 | 0.092 | 1.089 | 0.908–1.306 | 0.357 | |
T4 | 1.088 | 0.922–1.285 | 0.314 | 1.064 | 0.897–1.263 | 0.474 | |
N stage | |||||||
N0 | Reference | – | – | Reference | – | – | |
N1 | 0.921 | 0.742–1.144 | 0.460 | 0.909 | 0.731–1.131 | 0.392 | |
N2 | 1.214 | 1.051–1.403 | 0.008 | 1.256 | 1.083–1.459 | 0.002 | |
N3 | 1.300 | 1.102–1.533 | 0.001 | 1.342 | 1.130–1.595 | <0.001 | |
Surgery | |||||||
No | Reference | – | – | Reference | – | – | |
Yes | 0.341 | 0.204–0.567 | <0.001 | 0.501 | 0.299–0.838 | 0.008 | |
Radiation | |||||||
No/unknown | Reference | – | – | Reference | – | – | |
Yes | 0.669 | 0.597–0.751 | <0.001 | 0.873 | 0.775–0.984 | 0.025 | |
Chemotherapy | |||||||
No/unknown | Reference | – | – | Reference | – | – | |
Yes | 0.299 | 0.265–0.338 | <0.001 | 0.294 | 0.259–0.335 | <0.001 | |
Bone metastasis | |||||||
No | Reference | – | – | Reference | – | – | |
Yes | 1.373 | 1.231–1.532 | <0.001 | 1.247 | 1.104–1.410 | <0.001 | |
Liver metastasis | |||||||
No | Reference | – | – | Reference | – | – | |
Yes | 1.468 | 1.318–1.634 | <0.001 | 1.341 | 1.189–1.512 | <0.001 | |
Lung metastasis | |||||||
No | Reference | – | – | Reference | – | – | |
Yes | 1.313 | 1.159–1.487 | <0.001 | 1.161 | 1.018–1.325 | 0.025 |
SCLC, small cell lung cancer; BM, brain metastasis; CSS, cancer-specific survival; HR, hazard ratio; CI, confidence interval.
Nomogram construction and validation
These independent prognostic factors identified in training cohort were used for the construction of nomogram for predicting CSS (Figure 2). By adding up the scores of each selected variable, the probability of individual survival could be easily calculated. The detailed points of each prognostic factor in the nomogram were presented in Table 3.
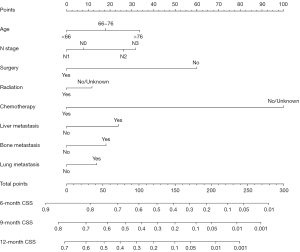
Table 3
Variables | CSS nomogram |
---|---|
Age (years) | |
<66 | 0 |
66–76 | 18.0 |
>76 | 33.7 |
N stage | |
N0 | 7.8 |
N1 | 0 |
N2 | 26.2 |
N3 | 31.8 |
Surgery | |
No | 59.8 |
Yes | 0 |
Radiation | |
No/unknown | 11.7 |
Yes | 0 |
Chemotherapy | |
No/unknown | 100.0 |
Yes | 0 |
Liver metastasis | |
No | 0 |
Yes | 23.7 |
Bone metastasis | |
No | 0 |
Yes | 18.2 |
Lung metastasis | |
No | 0 |
Yes | 13.8 |
CSS, cancer-specific survival.
The C-indexes of nomogram were 0.683 [95% confidence interval (CI): 0.667–0.699] in the training cohort and 0.659 (95% CI: 0.634–0.684) in the validation cohort. Time-dependent ROC curves were generated to assess the predictive accuracy of the nomogram (Figure 3). The AUC values of 6-, 9- and 12-month CSS were 0.723, 0.742 and 0.737 respectively in the training cohort, while 0.715, 0.737 and 0.739 in the validation cohort. The results showed satisfying discriminative ability and predictive accuracy in both training and validation cohorts. The calibration plots presented good agreement between nomogram-predicted CSS and actual CSS, suggesting that the nomogram was reliable for predicting survival in newly diagnosed SCLC patients with BM (Figure 4). Furthermore, DCA plot was used to evaluate the clinical usefulness of nomogram, and results revealed a wide range of threshold probability in both cohorts, indicating the excellent clinical applicability of nomogram for predicting CSS (Figure 5).
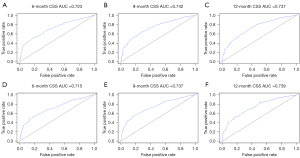
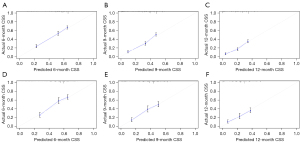
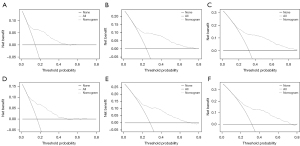
Survival analyses
The cutoff values of risk score were determined by X-tile software (Figure 6A), and the optimal cutoff values were 0.8 and 1.8. These SCLC patients with BM were then divided into low-risk group (risk score <0.8), medium-risk group (risk score 0.8–1.8) and high-risk group (risk score >1.8). Kaplan-Meier survival analyses with the log-rank test were then performed to compare the prognosis of patients. As shown in Figure 6B-6D, patients in high-risk group had a worse survival outcome than patients in medium-risk group and low-risk group in whole cohort (P<0.001), training cohort (P<0.001) and validation cohort (P<0.001).
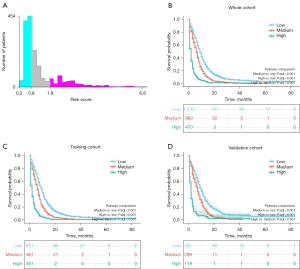
DiscussionOther Section
In the current study, we developed a novel nomogram for predicting the prognosis of newly diagnosed SCLC patients with BM from the SEER database. A total of 2,462 cases were enrolled, and eight significant prognostic factors (age, N stage, surgery, radiation, chemotherapy, bone metastasis, liver metastasis and lung metastasis) that represented demographic, pathological and therapeutic information were identified by using univariate and multivariate Cox regression analyses in the training cohort. These factors were used to construct the nomogram for predicting CSS of SCLC patients with BM. This nomogram was proved to have high accuracy and reliability by the validation of discrimination and calibration, as well as possess favorable clinical applicability.
The identification of risk factors among SCLC patients with BM is crucial, which can provide additional evidence for deciding on optimal treatments. Previous studies with large population revealed that age was an important predictor for survival outcome, which was consistent with the finding of this research (6,11,18). Patients with older age had a worse prognosis, which might be related to the poor physical function. Among NSCLC patients with distant organ metastasis, lymph node metastasis was demonstrated to be associated with a worse prognosis in terms of longer survival except patients with liver metastasis (19). As shown in our nomogram, higher N stage (N2 or N3) corresponded to higher score for CSS, which was consistent with the fact that survival was negatively associated with the number of lymph node metastasis (20).
Surgery was also a prognostic factor for SCLC patients with BM. It has been reported that surgery on primary site was negatively associated with overall mortality for patients who developed BM at initial lung cancer diagnosis (21). In another study, patients with brain metastases from NSCLC underwent primary lung surgery that were obviously associated with better long-term survival rates (22). SCLC is very sensitive to chemotherapy. For advanced or extensive stage SCLC, combined chemotherapy and immunotherapy have become the novel standard first-line treatment, with modest improvement in overall survival (23). Radiotherapy plays an important role in cancer control, and prophylactic cranial irradiation has become an accepted part of the management of both limited and extensive SCLC, contributing to decrease the risk of BM and improve survival (24). Furthermore, some European experts suggested that chemotherapy alone was the most commonly recommended first-line treatment in asymptomatic SCLC patients with BM, and whole brain radiotherapy followed by chemotherapy was recommended most commonly for symptomatic patients (25). In the current study, chemotherapy and radiotherapy were identified as positive prognostic factors for SCLC patients with BM.
The number of extracranial organs was a prognostic factor for NSCLC patients with BM, and patients with 0–1 extracranial metastasis had better survival than those with larger number of extracranial metastases (26). Huang et al. reported that bone metastasis and liver metastasis were prognostic factors for lung adenocarcinoma patients with BM, and patients with bone metastasis and liver metastasis had a poor prognosis (18). These findings were consistent with our results.
As we know, the heterogeneity of SCLC made it difficult to predict the prognosis through traditional stage method. Nowadays, many studies have demonstrated that nomograms could be applied to predict the survival outcome of SCLC patients. Wang et al. constructed and validated a prognostic nomogram for SCLC patients, which exhibited higher prognostic accuracy than the 8th edition of TNM stage system (27). Pan et al. established a novel nomogram for predicting overall survival of patients with SCLC, and this nomogram performed better than the existing staging systems (28). Moreover, among SCLC patients with bone metastasis, a prognostic nomogram was developed and proved to be an accurate and personalized prediction tool (29). However, there are very few studies on the prognosis in SCLC patients with BM. Based on identified independent prognostic factors in our study, the novel nomogram for predicting CSS of SCLC patients with BM was successfully constructed. The survival prediction model showed good performance in estimating the prognosis individually. For instance, compared with similar predictive models (30,31), the net benefit of DCA plots in our nomogram was larger, which indicated that the nomogram was a reliable clinical tool for predicting survival.
Several limitations should be acknowledged in the current study. Firstly, this is a retrospective study from the SEER database, which may introduce the risk of selection bias. When age is transformed into a categorical variable, some information may disappear. Secondly, some important variables that may influence survival could not be obtained from the SEER database, such as smoking status, serum biomarkers, immunotherapy, chemotherapy drugs and radiotherapy area. Thirdly, the predictive model was developed with the SEER database and was not verified by external data.
ConclusionsOther Section
In brief, we developed and validated a novel nomogram that can accurately predict the CSS of SCLC patients with BM according to prognostic factors identified from SEER database. In this way, doctors can identify patients with high-risk factors and provide them with the best individualized treatment.
AcknowledgmentsOther Section
The authors would like to thank SEER for open access to the database.
Funding: None.
FootnoteOther Section
Reporting Checklist: The authors have completed the TRIPOD reporting checklist. Available at https://tcr.amegroups.com/article/view/10.21037/tcr-22-1561/rc
Peer Review File: Available at https://tcr.amegroups.com/article/view/10.21037/tcr-22-1561/prf
Conflicts of Interest: All authors have completed the ICMJE uniform disclosure form (available at https://tcr.amegroups.com/article/view/10.21037/tcr-22-1561/coif). The authors have no conflicts of interest to declare.
Ethical Statement: The authors are accountable for all aspects of the work in ensuring that questions related to the accuracy or integrity of any part of the work are appropriately investigated and resolved. The study was conducted in accordance with the Declaration of Helsinki (as revised in 2013).
Open Access Statement: This is an Open Access article distributed in accordance with the Creative Commons Attribution-NonCommercial-NoDerivs 4.0 International License (CC BY-NC-ND 4.0), which permits the non-commercial replication and distribution of the article with the strict proviso that no changes or edits are made and the original work is properly cited (including links to both the formal publication through the relevant DOI and the license). See: https://creativecommons.org/licenses/by-nc-nd/4.0/.
ReferencesOther Section
- Siegel RL, Miller KD, Jemal A. Cancer statistics, 2020. CA Cancer J Clin 2020;70:7-30. [Crossref] [PubMed]
- Ju L, Yang J, Zhai C, et al. Survival, Chemotherapy and Chemosensitivity Predicted by CTC Cultured In Vitro of SCLC Patients. Front Oncol 2021;11:683318. [Crossref] [PubMed]
- Rudin CM, Brambilla E, Faivre-Finn C, et al. Small-cell lung cancer. Nat Rev Dis Primers 2021;7:3. [Crossref] [PubMed]
- Chan JM, Quintanal-Villalonga Á, Gao VR, et al. Signatures of plasticity, metastasis, and immunosuppression in an atlas of human small cell lung cancer. Cancer Cell 2021;39:1479-1496.e18. [Crossref] [PubMed]
- Faramand A, Niranjan A, Kano H, et al. Primary or salvage stereotactic radiosurgery for brain metastatic small cell lung cancer. J Neurooncol 2019;144:217-25. [Crossref] [PubMed]
- Reddy SP, Dowell JE, Pan E. Predictors of prognosis of synchronous brain metastases in small-cell lung cancer patients. Clin Exp Metastasis 2020;37:531-9. [Crossref] [PubMed]
- Rybarczyk-Kasiuchnicz A, Ramlau R, Stencel K. Treatment of Brain Metastases of Non-Small Cell Lung Carcinoma. Int J Mol Sci 2021;22:593. [Crossref] [PubMed]
- Ruiz-Cordero R, Devine WP. Targeted Therapy and Checkpoint Immunotherapy in Lung Cancer. Surg Pathol Clin 2020;13:17-33. [Crossref] [PubMed]
- Yu L, Lai Q, Gou L, et al. Opportunities and obstacles of targeted therapy and immunotherapy in small cell lung cancer. J Drug Target 2021;29:1-11. [Crossref] [PubMed]
- Balachandran VP, Gonen M, Smith JJ, et al. Nomograms in oncology: more than meets the eye. Lancet Oncol 2015;16:e173-80. [Crossref] [PubMed]
- Shan Q, Shi J, Wang X, et al. A new nomogram and risk classification system for predicting survival in small cell lung cancer patients diagnosed with brain metastasis: a large population-based study. BMC Cancer 2021;21:640. [Crossref] [PubMed]
- Xiong Y, Cao H, Zhang Y, et al. Nomogram-Predicted Survival of Breast Cancer Brain Metastasis: a SEER-Based Population Study. World Neurosurg 2019;128:e823-34. [Crossref] [PubMed]
- Pan X, Yang W, Chen Y, et al. Nomogram for predicting the overall survival of patients with inflammatory breast cancer: A SEER-based study. Breast 2019;47:56-61. [Crossref] [PubMed]
- Zuo Z, Zhang G, Song P, et al. Survival Nomogram for Stage IB Non-Small-Cell Lung Cancer Patients, Based on the SEER Database and an External Validation Cohort. Ann Surg Oncol 2021;28:3941-50. [Crossref] [PubMed]
- Doll KM, Rademaker A, Sosa JA. Practical Guide to Surgical Data Sets: Surveillance, Epidemiology, and End Results (SEER) Database. JAMA Surg 2018;153:588-9. [Crossref] [PubMed]
- Stone GW, Maehara A, Lansky AJ, et al. A prospective natural-history study of coronary atherosclerosis. N Engl J Med 2011;364:226-35. [Crossref] [PubMed]
- Dai L, Wang W, Liu Q, et al. Development and validation of prognostic nomogram for lung cancer patients below the age of 45 years. Bosn J Basic Med Sci 2021;21:352-63. [PubMed]
- Huang Z, Tong Y, Tian H, et al. Establishment of a Prognostic Nomogram for Lung Adenocarcinoma with Brain Metastases. World Neurosurg 2020;141:e700-9. [Crossref] [PubMed]
- Yang J, Peng A, Wang B, et al. The prognostic impact of lymph node metastasis in patients with non-small cell lung cancer and distant organ metastasis. Clin Exp Metastasis 2019;36:457-66. [Crossref] [PubMed]
- Wang L, Dou X, Liu T, et al. Tumor size and lymph node metastasis are prognostic markers of small cell lung cancer in a Chinese population. Medicine (Baltimore) 2018;97:e11712. [Crossref] [PubMed]
- Zuo C, Liu G, Bai Y, et al. The construction and validation of the model for predicting the incidence and prognosis of brain metastasis in lung cancer patients. Transl Cancer Res 2021;10:22-37. [Crossref] [PubMed]
- Antuña AR, Vega MA, Sanchez CR, et al. Brain Metastases of Non-Small Cell Lung Cancer: Prognostic Factors in Patients with Surgical Resection. J Neurol Surg A Cent Eur Neurosurg 2018;79:101-7. [Crossref] [PubMed]
- Tariq S, Kim SY, Monteiro de Oliveira Novaes J, et al. Update 2021: Management of Small Cell Lung Cancer. Lung 2021;199:579-87. [Crossref] [PubMed]
- Edelman MJ. Prophylactic Cranial Irradiation for Small-Cell Lung Cancer: Time for a Reassessment. Am Soc Clin Oncol Educ Book 2020;40:24-8. [Crossref] [PubMed]
- Putora PM, Fischer GF, Früh M, et al. Treatment of brain metastases in small cell lung cancer: Decision-making amongst a multidisciplinary panel of European experts. Radiother Oncol 2020;149:84-8. [Crossref] [PubMed]
- Wang M, Wu Q, Zhang J, et al. Prognostic impacts of extracranial metastasis on non-small cell lung cancer with brain metastasis: A retrospective study based on surveillance, epidemiology, and end results database. Cancer Med 2021;10:471-82. [Crossref] [PubMed]
- Wang S, Yang L, Ci B, et al. Development and Validation of a Nomogram Prognostic Model for SCLC Patients. J Thorac Oncol 2018;13:1338-48. [Crossref] [PubMed]
- Pan H, Shi X, Xiao D, et al. Nomogram prediction for the survival of the patients with small cell lung cancer. J Thorac Dis 2017;9:507-18. [Crossref] [PubMed]
- Fan Z, Huang Z, Tong Y, et al. Sites of Synchronous Distant Metastases, Prognosis, and Nomogram for Small Cell Lung Cancer Patients with Bone Metastasis: A Large Cohort Retrospective Study. J Oncol 2021;2021:9949714. [Crossref] [PubMed]
- Tian Y, He Y, Li X, et al. Novel nomograms to predict lymph node metastasis and distant metastasis in resected patients with early-stage non-small cell lung cancer. Ann Palliat Med 2021;10:2548-66. [Crossref] [PubMed]
- Zhang M, Wang B, Liu N, et al. Nomogram for predicting preoperative regional lymph nodes metastasis in patients with metaplastic breast cancer: a SEER population-based study. BMC Cancer 2021;21:565. [Crossref] [PubMed]