Comprehensive analysis of necroptosis-related long noncoding RNA to predict prognosis, immune status, and immunotherapeutic response in clear cell renal cell carcinoma
Introduction
Renal carcinoma is currently one of the most common urinary tract malignancies, the morbidity and mortality of which have been increasing in recent years. It has been reported that more than 400,000 new cases of renal carcinoma are diagnosed each year worldwide (1). Clear cell renal cell carcinoma (ccRCC) is the major histological subtype, which accounts for about 75–80% of all cases (2). Although partial and radical nephrectomy shows good outcomes in treating patients with early stage ccRCC, about 30% of localized ccRCC recur after surgical resection (3). Current strategies for stratifying patients with ccRCC in clinical practice are confined to a set of clinical and pathologic parameters, such as the tumor-node-metastasis (TNM) staging system, which relies primarily on anatomical information rather than biological features (4). The recent improvements of genetic testing technology and the emergence of new targeted drugs have brought new hope for treating end-stage or recurrent ccRCC (5). However, the prognosis of patients can differ significantly, even if they have identical clinical parameters and are receiving the same regimens. Thus, identification of reliable predictive biomarkers to indicate the risk, prognosis, and treatment response of patients is required.
It was previously thought that necrosis was the opposite type of cell death to apoptosis. However, recent research has shown that apoptosis and necrosis frequently share common triggers (6). This form of programmed cell death, called necroptosis, is primarily mediated by receptor-interacting protein kinase 1 (RIPK1), RIPK3, and mixed lineage kinase domain like pseudokinase (MLKL) and typically manifests with morphological features of necrosis (6,7). Previous reports showed that necroptosis can be activated by the tumor necrosis factor receptor (TNFR) superfamily, pattern recognition receptors (PRRs), T cell receptors (TCRs), interferons, and anticancer drugs, and these reports suggested that necroptosis plays a critical role in cancer progression (6,8). Interestingly, necroptosis has been reported to have dual effects of promoting and reducing tumor progression in different cancers (9).
For instance, the expression of RIPK1 has been reported to be downregulated in head and neck squamous cell carcinoma and has been confirmed to be correlated with tumor progression (10). Similarly, tumor-suppressing effects of RIPK3 have been demonstrated, and patients with colorectal cancer and lower RIPK3 expression have been found to have worse prognosis (11). By contrast, the upregulation of RIPK1 expression is correlated with a poorer prognosis in patients with lung cancer and glioblastoma, which suggests an oncogenic effect of RIPK1 (12). Therefore, the function of necroptosis in cancer development is complex. However, there are limited studies reporting an association between necroptosis and tumorigenesis, progression, and metastasis of ccRCC. Therefore, further study of the relationship between necroptosis and the biological characteristics of ccRCC is needed to provide a basis for more precise management and therapy.
Long noncoding RNA (lncRNA) is a type of RNA that does not encode proteins, with a length exceeding 200 nucleotides. LncRNA is recognized as playing crucial roles in numerous cellular processes, some of which are associated with cancer initiation, progression, and metastasis, and whose dysfunction may contribute to tumorigenesis (13,14).
Based on the gene expression profile of ccRCC obtained from The Cancer Genome Atlas (TCGA) database, comprehensive bioinformatics analyses were performed to explore lncRNAs related to necroptosis genes and to construct the relevant prognostic signature. Patients with ccRCC were classified into two different clusters based on their prognostic lncRNAs expression. We present the following article in accordance with the TRIPOD reporting checklist (available at https://tcr.amegroups.com/article/view/10.21037/tcr-22-1764/rc).
Methods
Data acquisition and processing
The study workflow is shown in Figure 1. The high-throughput sequencing count data (HTSeq-counts) of transcriptome profiling containing messenger RNA (mRNA), lncRNA, microRNA (miRNA), somatic mutation profiles, and clinical manifestation data of kidney renal clear cell carcinoma (KIRC) were retrieved from TCGA KIRC program (https://tcga-data.nci.nih.gov/tcga/) and Genomic Data Commons (GDC) data portal (https://portal.gdc.cancer.gov/). Genes expressed in less than 75% of samples were excluded. The eligibility criteria were as follows: (I) patients with complete follow-up information and with an overall survival (OS) of more than 30 days, and (II) samples with complete lncRNA expression levels. Clinical and RNA-seq data from 3 prospective clinical trials which applied the anti-programmed cell death-1 (PD-1) antibody nivolumab in advanced ccRCC were used to examine the effects of immunotherapy with the established signature (15).
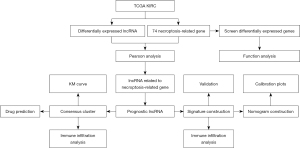
A total of 74 necroptosis-related genes were retrieved, including 12 genes were obtained from the Molecular Signatures Database (https://www.gsea-msigdb.org/gsea/msigdb/), and the other 62 genes were retrieved from previous research and used for further analysis (16).
The study was conducted in accordance with the Declaration of Helsinki (as revised in 2013).
Identification of differentially expressed necroptosis-related genes and enrichment analysis
The R package ‘bioMart’ was used for gene annotation (17). A total of 19,712 mRNA and 14,805 lncRNA were identified. Subsequently, the expression matrix of 74 necroptosis-related genes was extracted from the filtered mRNA expression matrix. The R package ‘limma’ was used to screen differentially expressed genes (DEGs) in KIRC and normal tissues with cut-off criteria of an adjusted P value (adj.P value) <0.05, and |logFC| ≥1 (18). The heatmap was plotted using the R package ‘pheatmap’. Gene Ontology (GO) was applied for enrichment analysis of the DEGs (19).
Identification of lncRNAs related to necroptosis-related genes
To identify differentially expressed lncRNAs, the R package ‘limma’ was used (18). A total of 528 differentially expressed lncRNAs were screened using an adj.P value of 0.05, and |logFC| ≥2 as criteria. Next, Pearson correlation analysis between the expression profile of differentially expressed lncRNAs and necroptosis-related genes was performed. The thresholds of P<0.01 and |R| >0.6 were used to select lncRNAs (20).
Identification necroptosis-associated lncRNAs prognostic signatures for ccRCC
Using the sample screening criteria mentioned above, the R package ‘caret’ was used to randomly divide the 487 eligible ccRCC samples into the training and testing cohorts at a ratio of 7:3, which was also used to randomly divide the 487 ccRCC samples into 10 groups based on the number of days survived.
To identify necroptosis-associated lncRNAs correlated with survival, univariate Cox regression analysis was conducted in the training cohort using the cut-off value of P<0.01. Subsequently, the least absolute shrinkage and selection operator (LASSO) regression analysis was performed to select candidate prognostic lncRNAs. Next, candidate lncRNAs were subjected to multivariate Cox regression analysis using the R package ‘glmnet’ to establish the best prognostic risk profile. Furthermore, the risk score for each patient was calculated according to the following formula: Risk score=coef (lncRNA1) × expression (lncRNA1) + coef (lncRNA2) × expression (lncRNA2) + … + coef (lncRNAn) × expression (lncRNAn). In the formula above, coef (lncRNAn) was defined as the regression coefficient of lncRNAs calculated using multivariate Cox regression analysis, and expression (lncRNAn) was defined as the expression level of lncRNAs (21).
Evaluation of the prognostic signature
The median risk score value was used to classify samples in the training cohort into low- or high-risk groups. Moreover, the test cohort and entire cohort were used to validate the model’s reliability. Kaplan-Meier (KM) survival curves were plotted to evaluate the survival difference between patients in the two groups. Meanwhile, a time-dependent receiver operating characteristic (ROC) curve was created to evaluate the predictive ability of the signature. The area under the ROC curve (AUC) was calculated from the ROC curves. The R packages ‘survival’ and ‘survminer’ were used to perform these analyses. Furthermore, univariate and multivariate Cox regression analyses were used to assess whether the risk score was independent of other clinical variables based on age, clinicopathological characters, and signature in the training, test, and the entire cohort. The stratified analysis was performed to assess the relationship between the prognostic risk score and clinical features with a threshold as P<0.05.
Establishment and validation of nomogram
In addition to assessing the survival probability of patients, traditional clinical characteristics, such as age, clinicopathologic characteristics, and risk score, were considered to construct a nomogram using the R package ‘rms’ (22). The 1-, 5-, and 7-year OS probabilities of patients can be predicted by calculating the nomogram points. Subsequently, time-dependent ROC and calibration plots were created to evaluate the performance of the nomogram, and the AUC of risk score, grade, stage, and age 5 years from diagnosis were used to assess the accuracy for predicting OS.
Immune assessment, drug prediction, and immunotherapeutic efficacy of the risk model
Next, single sample gene set enrichment analysis (ssGSEA) was applied to obtain the extent of immune cells infiltration of ccRCC samples (23). The association between the risk scores and lncRNAs was used to construct the signature and the extent of infiltration of immune cells in ccRCC. The fractions of stromal and immune of each ccRCC sample were obtained using the Estimation of STromal and Immune cells in MAlignant Tumor tissues using Expression data (ESTIMATE) algorithm (24). In addition, the correlation between the risk score of the signature and checkpoints, such as programmed cell death (CD274), sialic acid binding Ig-like lectin 15 (SIGLEC15), cytotoxic T-lymphocyte-associated protein 4 (CTLA4), programmed cell death 1 ligand 2 (PDCD1LG2), tumor necrosis factor receptor superfamily, member 7 (CD27), programmed cell death 1 (PDCD1), hepatitis A virus cellular receptor 2 (HAVCR2), and lymphocyte-activation gene 3 (LAG-3) were investigated. The chemotherapeutic response represented by the half-maximal inhibitory concentration (IC50) of patients with KIRC from the Cancer Genome Project (CGP) were analyzed using the R package ‘pRRophetic’ (25). Gene set enrichment analysis (GSEA) was employed to assess the biological processes that were significantly different between the 2 risk groups using the R package ‘clusterProfiler’.
Mutation landscape analysis based on 6 prognostic lncRNAs
The R package ‘maftools’ was used to evaluate differences in the somatic mutations between the two risk groups. Subsequently, differences of tumor mutational burden (TMB) of patients in the two risk groups were explored.
Clusters based on 6 prognostic lncRNAs
Patients with ccRCC were divided into two potential molecular subgroups using the R package ‘ConsensusClusterPlus’ based on the expression of 6 necroptosis-associated lncRNAs related to prognosis (26). A KM survival curve was plotted to evaluate the survival difference between subgroups of patients. The correlation between clusters and immune cells was further evaluated. The ESTIMATE algorithm was also used to acquire the fraction of stromal and immune of each ccRCC sample. Furthermore, the correlation between clusters and checkpoints and immunotherapeutic response prediction was assessed using the R package ‘pRRophetic’.
Statistical analysis
R software v.4.0.1 was used for all statistical analyses in this study. The Pearson χ2 test or Fisher’s exact test was used to analyze categorical variables. The t-test or non-parametric Wilcoxon rank-sum test was used to analyze quantitative variables. Stratified k-fold cross-validation was used to evaluate model performance. Unless otherwise specified, P<0.05 was considered statistically significant.
Results
Identification of differentially expressed necroptosis-related genes and enrichment analysis
After differentially analyzing the extracted necroptosis-related gene expression profile of the tissue samples from patients with ccRCC from TCGA database, 18 differentially expressed mRNAs were identified, which were then subjected to enrichment analysis (Figure 2A,2B). As shown in Figure 2C, these genes were enriched in necrotic cell death, programmed necrotic cell death, necroptotic process, regulation of endothelial cell apoptotic process, cellular response to tumor necrosis factor, and regulation of inflammatory response, which suggests the potential role of necroptosis-related genes in the tumor immune response.
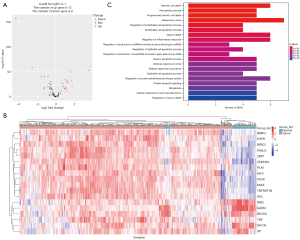
Identification a necroptosis-associated lncRNAs prognostic signatures for ccRCC
In all, 528 differentially expressed lncRNAs were obtained from the lncRNA expression matrix. Then, necroptosis-associated lncRNAs were identified by conducting Pearson correlation analysis between the differentially expressed lncRNAs and the necroptosis-related genes.
Based on the inclusion criteria, 487 patients with ccRCC were retained and classified into a training and a testing cohort of 341 and 146 patients, respectively. LASSO regression analysis and univariate Cox regression analysis were used for the necroptosis-associated lncRNAs and identified 6 candidate lncRNAs that were significantly associated with the prognosis of ccRCC (Figure 3A,3B). Then, multivariate Cox regression analysis of these lncRNAs was performed to establish a prognostic signature. The results showed that the high expression of FLJ22763, RP11-968O1.5, and CTD-3162L10.3 were associated with a good prognosis, whereas the high expression of RP11-932O9.1, EMX2OS, and LINC00460 were associated with a poorer prognosis (Figure 3C). However, the confidence intervals of EMX2OS and CTD-3162L10.3 included zero, and the P value was greater than 0.05, which indicated that the odds ratio (OR) was not significant. The risk score of each sample can be calculated using the following formula:
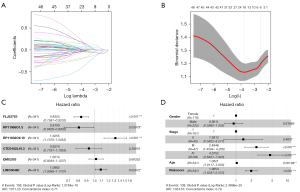
The KM survival curve of each lncRNA was plotted to evaluate the survival difference between patients in the different expression subgroups (Figure S1). It is worth noting that the KM survival curve shows that high expression of EMX2OS is associated with a favorable prognosis (Figure S1D).
Using the median risk score as a threshold, the sample was divided into the high- and low-risk groups. The expression of 6 lncRNAs in the high- and low-risk groups is shown in Figure S2. The Cox regression results indicate that lncRNAs signature is an independent prognostic factor for patients with ccRCC (Figure 3D, Table 1). Figure 4A-4C shows the distribution of risk scores, the survival status, and the expression of the 6 lncRNAs in the 2 risk groups, which demonstrate that patients in the low-risk group generally had better survival. As shown in Figure 4D, the survival time of patients in the high-risk group was shorter than that in the low-risk group. The AUC for 1-, 5-, and 7-year survival prediction were 0.73, 0.72, and 0.77, respectively (Figure 4E), which demonstrates the accuracy of the signature’s prediction ability.
Table 1
Variables | Univariate Cox regression analysis | Multivariate Cox regression analysis | |||||||
---|---|---|---|---|---|---|---|---|---|
HR | Lower 95% CI | Upper 95% CI | P value | HR | Lower 95% CI | Upper 95% CI | P value | ||
Age (years) | 1.03 | 1.02 | 1.04 | <0.001 | 1.03 | 1.01 | 1.05 | 0.002 | |
Gender | 0.967 | 0.7 | 1.34 | 0.843 | 1 | 0.67 | 1.51 | 0.989 | |
Stage I | 1 | – | – | – | 1 | – | – | – | |
Stage II | 1.213 | 0.6 | 2.44 | 0.587 | 1.5 | 0.63 | 3.53 | 0.358 | |
Stage III | 2.878 | 1.87 | 4.44 | <0.001 | 2.37 | 1.38 | 4.07 | 0.002 | |
Stage IV | 6.899 | 4.6 | 10.35 | <0.001 | 4.41 | 2.55 | 7.64 | <0.001 | |
Risk score | 3.65 | 2.78 | 4.79 | <0.001 | 1.86 | 1.47 | 2.35 | <0.001 |
HR, hazard ratio; CI, confidence interval.
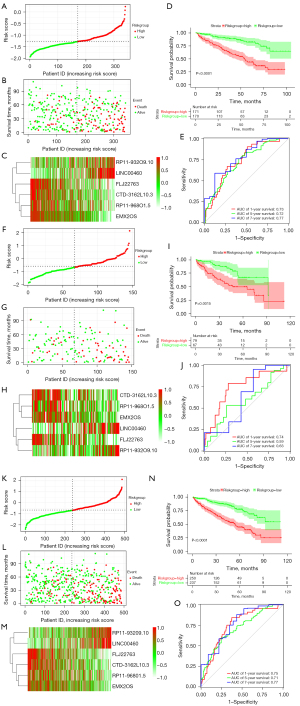
Validation of the 6 lncRNAs prognostic signature
To further validate the robustness of the prognostic model, further examinations in the testing and the entire sets were performed with the same cut-off values as those used in the training cohort.
Figure 4F-4H show the distribution of risk scores, survival status, and expression of the 6 lncRNAs in the 2 risk groups of the testing cohort. In addition, KM curves indicated that survival time of patients in the high-risk group was shorter than that in the low-risk group (Figure 4I). The AUC for 1-, 5-, and 7-year survival prediction of the time-dependent ROC curves were 0.74, 0.59, and 0.63, respectively (Figure 4J).
Figure 4K-4M shows the distribution of risk scores, survival status, and expression of the 6 lncRNAs in the 2 risk groups of the whole samples. In addition, KM curves indicated that the high-risk patients with ccRCC had a worse prognosis than the low-risk patients (Figure 4N). As shown in Figure 4O, the AUCs for 1-, 5-, and 7-year survival prediction of the time-dependent ROC curves were 0.75, 0.71, and 0.77, respectively.
Taking into account that the model may be overfitted, stratified k-fold cross-validation was conducted to assess the stability of model performance. The samples were divided equally into 10 groups for the validation, which was performed 10 times, whereby 9 groups were selected for each run. The 1-, 5-, and 7-year mean AUCs of the 10 runs were 0.71, 0.69, and 0.71, respectively (Table S1).
Independent analysis of clinicopathological characteristics and the signature
To further validate whether the signature was an independent factor of clinicopathological characteristics, univariate and multivariate Cox regression analyses were performed on the signature and clinicopathological characteristics including age, gender, grade, and stage. In addition, stage was also demonstrated to be an independent clinicopathological prognostic factor for patients with ccRCC.
Correlations between the necroptosis-associated lncRNAs signature and clinicopathological characteristics
The results showed that patients with high- (III–IV) and advanced stage (III–IV) ccRCC had higher risk scores (Figure S3A,S3B). The KM curves and AUC of the time-dependent ROC curves demonstrated that necroptosis-associated lncRNAs signatures were still significantly correlated with worse survival in patients with advanced- (Stage III–IV) or early-stage (stage Ⅰ–Ⅱ) ccRCC and those with higher- (grade Ⅰ–Ⅱ) or lower-grade (grade III–IV) ccRCC (all P<0.05; Figure S3C-S3F), which suggests that the signature-based risk stratification still had significant effectiveness for patients with ccRCC of the same stage and grade.
Immune assessment, drug prediction, and immunotherapeutic efficacy of the risk model
The extent of infiltration of 28 immune cells of cancer tissue samples were obtained using ssGSEA, which was then applied to explore the relationship between the extent of infiltration of immune cells and the risk scores in ccRCC (Figure 5A). The correlations between the immune cells and lncRNAs are also shown in Figure 5B. These findings demonstrate correlations of the high-risk group with higher levels of immune suppressive cells, such as regulatory T cells (Tregs), T follicular helper cells, and macrophages. Using the ESTIMATE algorithm, the estimate and immune scores of each ccRCC sample were obtained. As shown in Figure 5C-5E, immune and stromal scores were significantly and positively correlated with the risk score. Furthermore, we found that the expression level of immune checkpoints such as CD27, CTLA-4, LAG-3, and PDCD1 were increased in the high-risk group, while CD274 and HAVCR2 were decreased (Figure 6A), which further suggests that patients with a higher risk score were more likely to be in an immunosuppressive microenvironment. The results of prediction of chemotherapeutic response of patients with ccRCC are shown in Figure 6B-6H. Patients with higher risk scores were more sensitive to chemotherapeutic drugs, indicating that patients in the high risk-group may benefit from therapy that may prolong life.
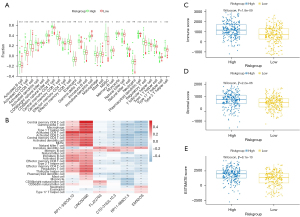
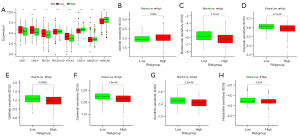
Mutation data of 294 ccRCC samples were then analyzed and curated to investigate the potential mechanisms associated with the risk model. The top 10 genes with the highest frequency of alteration in the high- and low-risk groups are shown in Figure 7A,7B, respectively. It was further found that tumor mutation burden was higher in the high-risk group than in the low-risk group (Figure 7C). Since immune checkpoint blockade targeting checkpoints has emerged as a promising strategy for treating cancers in clinical practice, the public immunotherapy cohorts were used to investigate the association between signature and immunotherapeutic response. Using progression-free survival (PFS) as a criterion, the R package ‘survminer’ was used to identify the best cut-off point to divide patients into two groups. The KM curve indicated that high-risk patients with ccRCC had a significantly shorter survival than low-risk patients (Figure 7D). We further examined whether clinical benefit rates were significantly different between these two groups. The results suggested that patients with a lower risk score may be more likely to benefit from immunotherapy (Figure 7E).
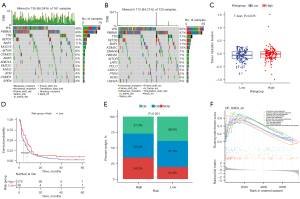
The results of GSEA suggested that genes related to high-risk scores were mainly enriched in natural killer (NK) cell-mediated cytotoxicity, cytokine-cytokine receptor interaction, Th17 cell differentiation, and the TNF signaling pathway (Figure 7F).
Construction and validation of the nomogram
To promote the clinical utility of this novel model, a nomogram for predicting the 1-, 5-, and 7-year OS among patients with ccRCC was constructed based on the independent factors assessed above by using the R package ‘rms’ (Figure 8A). Time-dependent ROC curves were plotted and showed good discrimination of the nomogram, in that AUCs of 1-, 5-, and 7-year follow-up ROCs were 0.82, 0.78, and 0.79, respectively (Figure 8B). Additionally, calibration plots were plotted to demonstrate the good predictive performance of the nomogram (Figure 8C-8E). Furthermore, we plotted multiparameter ROC curves to evaluate the discriminative ability of the 5-year OS of the nomogram (Figure 8F). All of the above results suggest that the 6 lncRNA prognostic signatures for ccRCC were robust.
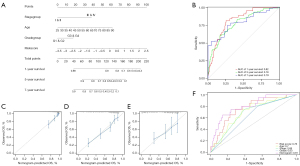
Clusters of necroptosis-associated patterns in patients with ccRCC
We performed K-means consensus clustering based on the expression of the 6 necroptosis-associated lncRNAs described above to regroup patients with ccRCC using the R package ‘ConsensusClusterPlus’. The K-means consensus clustering placed patients into two groups, referred to as Clusters A and B with different immune infiltration levels (Figure 9A-9C). A KM curve with a log-rank test indicated that Cluster A had a worse prognosis than Cluster B (Figure 9D). Interestingly, the fraction of low-risk patients was significantly higher in Cluster B, which was consistent with the result of the KM curve. The patients in Cluster A had a stronger correlation with immune infiltration level, and nearly all of the 28 immune cells were highly infiltrated. The ESTIMATE results further suggested that the immune and stromal scores were significantly higher in Cluster A (Figure 9E-9G). The results above indicated that the tumor microenvironments of the 2 clusters were significantly different. According to previous research, tumors that have high immune infiltration were considered hot tumors (27,28). We further explored whether Cluster A which was considered as hot tumors, would contribute to patients’ immunotherapy in high-risk groups. Almost all immune checkpoints, such as CD27, LAG-3, CTLA-4, and PDCD1, were more strongly expressed in Cluster A (Figure 9H). The results of the prediction of drug response suggest that hot tumors were more susceptible to chemotherapy (Figure 9I).
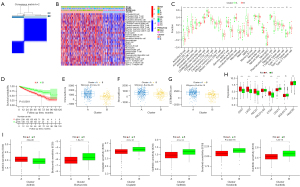
Discussion
CcRCC is the most common type of renal cell carcinoma (RCC), accounting for about 75% of RCC cases, and is associated with substantial morbidity, mortality, and cost (2,29). With the advance in understanding of the molecular biology and genetics of clear ccRCC, although partial and radical nephrectomy remain the gold-standard treatment for early stage ccRCC (30), the therapeutic options for advanced ccRCC have expanded. Immunotherapy with checkpoint inhibition and targeted molecular therapeutics has been applied to provide ‘precision treatment’ and prolong the OS and PFS of patients (31). However, drug resistance and insufficient understanding of molecular markers of ccRCC progression remain important obstacles to improving patient outcomes in clinical practice. Thus, a better understanding of the potential molecular mechanisms underlying ccRCC progression is vital for the management of ccRCC. The identification of effective molecular biomarkers to predict ccRCC progression and prognosis and to overcome drug resistance is critical.
Although numerous ccRCC prognostic models based on molecular biomarkers of especial gene sets have been developed in recent years, the signatures associated with necroptosis-associated lncRNAs have not been fully explored, especially in ccRCC. Necroptosis is a novel mode of programmed cell death, which is different from traditional apoptosis in that it has a mechanistic resemblance to apoptosis and a morphological resemblance to necrosis (6,32). Recent evidence suggests that high-grade RCC cells are correlated with higher expression of RIPK1 and RIPK3, which in turn are more prone to necroptosis (33). In the present study, we developed a signature of necroptosis-related lncRNA based on the lncRNAs related to necroptosis-related genes, which was validated to be associated with ccRCC progression and found to be an independent prognostic factor of the survival of patients with ccRCC. KM survival curves demonstrated that patients in the high-risk group had shorter survival than patients in the low-risk group, indicating that the signature was effective for predicting prognosis. The ROC analysis and AUCs for 1-, 5-, and 7- year follow-up results confirmed the signature was a highly effective tool for predicting prognosis. Moreover, the signature showed a significant correlation with clinical clinicopathological characters in that patients with higher-grade (III–IV) and -stage (III–IV) ccRCC had higher risk scores, thereby further demonstrating the robustness of prognostic prediction. The univariate and multivariate Cox regression results suggested that the signature can be utilized as an independent factor for the survival of patients with ccRCC. In summary, these findings demonstrated excellent prognostic and discriminable value of our lncRNAs signature.
The nomogram was further constructed based on age, stage, grade, and our signature to predict 1-, 5-, and 7-year survival rates of patients with ccRCC. Time-dependent ROC verified the effectiveness of our prognostic signature. Calibration analysis showed great convergency to the standard curve, which demonstrated the superior clinical utility of the nomogram. These results may help facilitate the individualized treatment of patients with ccRCC.
In addition, the tumor microenvironment plays a major role in the tumorigenesis and progression of cancers, and immunotherapy has promised to be an effective treatment for cancers (34). Necroptosis directly activates and modulates the inflammatory response, and necroptosis regulators, such as RIPK1 and RIPK3, have been shown to act independently in inflammation (6). Based on the statistical analysis between the association between infiltration level and risk score, we found that the microenvironment of patients with higher risk scores was more prone to immunosuppressive phenotypes, which is consist with previous reports and may influence immunotherapeutic resistance and efficacy (35,36). Consistent with these findings, we further found that patients in high-risk group had higher immune and stromal scores. Almost all of the expression of immune checkpoints was increased in the high-risk group. This underscores that necroptosis remodels the ccRCC tissue microenvironment, and that the underlying relationship between necroptosis and immune cell infiltration needs to be assessed further. Additional exploration in the intermediate clinical benefit cohort showed that low-risk patients had an improved response to immunotherapy, which provides a new therapeutic strategy for clinicians.
Finally, we divided patients into two groups using K-means consensus clustering. Cluster B included a higher fraction of low-risk patients and showed better prognosis and was associated with the presence of cold tumors. The results suggested that patients can be classified effectively according to lncRNA, and that the biological functions of the two groups are significantly different. The immune infiltration status of hot tumors was more active than that of the cold tumors, and hot tumors were also more sensitive to immunotherapeutic drugs. These results suggest that the lncRNAs selected in our study could not only predict prognosis but also guide tumor treatment.
LncRNA is involved in the regulation of protein translation and mRNA transcription. Aberrant expression of lncRNA is a common biological phenomenon and is closely related to the prognosis of cancers (37). LINC00460 is widely and highly expressed in human cancers and acts as a potential oncogene, which is a potential biomarker for cancer diagnosis, prognosis, and therapy (38). The correlation between lncRNAs and immune cells suggests that LINC00460 was highly and positively associated with immune status, indicating it might be the essential biomarker for the signature. The underlying mechanism of LINC00460 needs to be assessed further. Moreover, EMX2OS has been reported previously as being downregulated in ccRCC tissues and has been found to be significantly associated with higher grade, advanced stage, and poorer outcome (39). EMX2OS has been found and validated as a prognosis-associated enhancer in gastric cancer and papillary thyroid cancer (40,41). Additionally, FLJ22763 is downregulated in gastric cancer tissues and has been revealed to play a protective role in the prognosis of gastric cancer (42). The results suggest that the lncRNAs selected for the signature play an important role in cancer progression and might be potential biomarkers for treatment.
Our study has some limitations. First, the constructed signature was only verified internally. Second, because our study was a retrospective study and the clinical information of patients is prone to bias, prospective studies are needed to further validate the findings. Third, the ability of the risk score to predict drug response needs to be confirmed in sufficiently large samples using experimental validation of clinical studies. Finally, the specific molecular mechanisms for necroptosis-associated lncRNAs in ccRCC need to be investigated further in in vivo and in vitro experiments.
Conclusions
A 6 necroptosis-associated lncRNAs prognostic risk score model was developed to act as a novel independent prognostic factor for ccRCC. The signature was positively and significantly correlated with the infiltration level of immune cells, such as T follicular helper cells, Tregs, and macrophages, which suggests that the risk level was correlated with the tumor immunosuppressive microenvironment. We also found that patients with a lower risk score might benefit from immunotherapy. The predictive nomograms of the 6 lncRNA signature may help to predict the individual odds of death, which may assist clinicians with the selection of precision therapy for patients with ccRCC. Samples were identified as either hot or cold tumors based on the 6 prognostic lncRNAs to help clinicians predict tumor immune status and select individual chemotherapy regimens. Necroptosis remodels the ccRCC microenvironment, which was important for understanding response and resistance to immunotherapy.
Acknowledgments
We would like to thank TCGA and MSigDB databases for the availability of the data. We also thank the R programming package developers.
Funding: This work was supported by
Footnote
Reporting Checklist: The authors completed the TRIPOD reporting checklist. Available at https://tcr.amegroups.com/article/view/10.21037/tcr-22-1764/rc
Conflicts of Interest: All authors have completed the ICMJE uniform disclosure form (available at https://tcr.amegroups.com/article/view/10.21037/tcr-22-1764/coif). The authors have no conflicts of interest to declare.
Ethical Statement: The authors are accountable for all aspects of the work in ensuring that questions related to the accuracy or integrity of any part of the work are appropriately investigated and resolved. The study was conducted in accordance with the Declaration of Helsinki (as revised in 2013).
Open Access Statement: This is an Open Access article distributed in accordance with the Creative Commons Attribution-NonCommercial-NoDerivs 4.0 International License (CC BY-NC-ND 4.0), which permits the non-commercial replication and distribution of the article with the strict proviso that no changes or edits are made and the original work is properly cited (including links to both the formal publication through the relevant DOI and the license). See: https://creativecommons.org/licenses/by-nc-nd/4.0/.
References
- Sung H, Ferlay J, Siegel RL, et al. Global Cancer Statistics 2020: GLOBOCAN Estimates of Incidence and Mortality Worldwide for 36 Cancers in 185 Countries. CA Cancer J Clin 2021;71:209-49. [Crossref] [PubMed]
- Makhov P, Joshi S, Ghatalia P, et al. Resistance to Systemic Therapies in Clear Cell Renal Cell Carcinoma: Mechanisms and Management Strategies. Mol Cancer Ther 2018;17:1355-64. [Crossref] [PubMed]
- Kotecha RR, Motzer RJ, Voss MH. Towards individualized therapy for metastatic renal cell carcinoma. Nat Rev Clin Oncol 2019;16:621-33. [Crossref] [PubMed]
- Ljungberg B, Albiges L, Abu-Ghanem Y, et al. European Association of Urology Guidelines on Renal Cell Carcinoma: The 2022 Update. Eur Urol 2022;82:399-410. [Crossref] [PubMed]
- Argentiero A, Solimando AG, Krebs M, et al. Anti-angiogenesis and Immunotherapy: Novel Paradigms to Envision Tailored Approaches in Renal Cell-Carcinoma. J Clin Med 2020;9:1594. [Crossref] [PubMed]
- From the American Association of Neurological Surgeons (AANS). Multisociety Consensus Quality Improvement Revised Consensus Statement for Endovascular Therapy of Acute Ischemic Stroke. Int J Stroke 2018;13:612-32. [PubMed]
- Gong Y, Fan Z, Luo G, et al. The role of necroptosis in cancer biology and therapy. Mol Cancer 2019;18:100. [Crossref] [PubMed]
- Gao W, Wang X, Zhou Y, et al. Autophagy, ferroptosis, pyroptosis, and necroptosis in tumor immunotherapy. Signal Transduct Target Ther 2022;7:196. [Crossref] [PubMed]
- Mohammad NS, Nazli R, Zafar H, et al. Effects of lipid based Multiple Micronutrients Supplement on the birth outcome of underweight pre-eclamptic women: A randomized clinical trial. Pak J Med Sci 2022;38:219-26. [PubMed]
- McCormick KD, Ghosh A, Trivedi S, et al. Innate immune signaling through differential RIPK1 expression promote tumor progression in head and neck squamous cell carcinoma. Carcinogenesis 2016;37:522-9. [Crossref] [PubMed]
- O’Donnell MA, Perez-Jimenez E, Oberst A, et al. Caspase 8 inhibits programmed necrosis by processing CYLD. Nat Cell Biol 2011;13:1437-42. [Crossref] [PubMed]
- Park S, Hatanpaa KJ, Xie Y, et al. The receptor interacting protein 1 inhibits p53 induction through NF-kappaB activation and confers a worse prognosis in glioblastoma. Cancer Res 2009;69:2809-16. [Crossref] [PubMed]
- Bridges MC, Daulagala AC, Kourtidis A. LNCcation: lncRNA localization and function. J Cell Biol 2021; [Crossref] [PubMed]
- Peng WX, Koirala P, Mo YY. LncRNA-mediated regulation of cell signaling in cancer. Oncogene 2017;36:5661-7. [Crossref] [PubMed]
- Braun DA, Hou Y, Bakouny Z, et al. Interplay of somatic alterations and immune infiltration modulates response to PD-1 blockade in advanced clear cell renal cell carcinoma. Nat Med 2020;26:909-18. [Crossref] [PubMed]
- Xin S, Mao J, Duan C, et al. Identification and Quantification of Necroptosis Landscape on Therapy and Prognosis in Kidney Renal Clear Cell Carcinoma. Front Genet 2022;13:832046. [Crossref] [PubMed]
- Zhang J, Haider S, Baran J, et al. BioMart: a data federation framework for large collaborative projects. Database (Oxford) 2011;2011:bar038. [Crossref] [PubMed]
- Ritchie ME, Phipson B, Wu D, et al. limma powers differential expression analyses for RNA-sequencing and microarray studies. Nucleic Acids Res 2015;43:e47. [Crossref] [PubMed]
- Ashburner M, Ball CA, Blake JA, et al. Gene ontology: tool for the unification of biology. The Gene Ontology Consortium. Nat Genet 2000;25:25-9. [Crossref] [PubMed]
- Zhang G, Li Y, Li N, et al. Functional implications of aging-related lncRNAs for predicting prognosis and immune status in glioma patients. Aging (Albany NY) 2022;14:2348-66. [Crossref] [PubMed]
- Xia P, Li Q, Wu G, et al. An Immune-Related lncRNA Signature to Predict Survival In Glioma Patients. Cell Mol Neurobiol 2021;41:365-75. [Crossref] [PubMed]
- Iasonos A, Schrag D, Raj GV, et al. How to build and interpret a nomogram for cancer prognosis. J Clin Oncol 2008;26:1364-70. [Crossref] [PubMed]
- Hänzelmann S, Castelo R, Guinney J. GSVA: gene set variation analysis for microarray and RNA-seq data. BMC Bioinformatics 2013;14:7. [Crossref] [PubMed]
- Yoshihara K, Shahmoradgoli M, Martínez E, et al. Inferring tumour purity and stromal and immune cell admixture from expression data. Nat Commun 2013;4:2612. [Crossref] [PubMed]
- Geeleher P, Cox NJ, Huang RS. Clinical drug response can be predicted using baseline gene expression levels and in vitro drug sensitivity in cell lines. Genome Biol 2014;15:R47. [Crossref] [PubMed]
- Wilkerson MD, Hayes DN. ConsensusClusterPlus: a class discovery tool with confidence assessments and item tracking. Bioinformatics 2010;26:1572-3. [Crossref] [PubMed]
- DeBerardinis RJ. Tumor Microenvironment, Metabolism, and Immunotherapy. N Engl J Med 2020;382:869-71. [Crossref] [PubMed]
- Zheng Y, Tian H, Zhou Z, et al. A Novel Immune-Related Prognostic Model for Response to Immunotherapy and Survival in Patients With Lung Adenocarcinoma. Front Cell Dev Biol 2021;9:651406. [Crossref] [PubMed]
- Jonasch E, Walker CL, Rathmell WK. Clear cell renal cell carcinoma ontogeny and mechanisms of lethality. Nat Rev Nephrol 2021;17:245-61. [Crossref] [PubMed]
- Motzer RJ, Jonasch E, Agarwal N, et al. Kidney Cancer, Version 2.2017, NCCN Clinical Practice Guidelines in Oncology. J Natl Compr Canc Netw 2017;15:804-34. [Crossref] [PubMed]
- Hah YS, Koo KC. Immunology and Immunotherapeutic Approaches for Advanced Renal Cell Carcinoma: A Comprehensive Review. Int J Mol Sci 2021; [Crossref] [PubMed]
- Christofferson DE, Yuan J. Necroptosis as an alternative form of programmed cell death. Curr Opin Cell Biol 2010;22:263-8. [Crossref] [PubMed]
- Al-Lamki RS, Lu W, Manalo P, et al. Tubular epithelial cells in renal clear cell carcinoma express high RIPK1/3 and show increased susceptibility to TNF receptor 1-induced necroptosis. Cell Death Dis 2016;7:e2287. [Crossref] [PubMed]
- Hinshaw DC, Shevde LA. The Tumor Microenvironment Innately Modulates Cancer Progression. Cancer Res 2019;79:4557-66. [Crossref] [PubMed]
- Hu D, Zhou M, Zhu X. Deciphering Immune-Associated Genes to Predict Survival in Clear Cell Renal Cell Cancer. Biomed Res Int 2019;2019:2506843. [Crossref] [PubMed]
- Petitprez F, Meylan M, de Reyniès A, et al. The Tumor Microenvironment in the Response to Immune Checkpoint Blockade Therapies. Front Immunol 2020;11:784. [Crossref] [PubMed]
- Qi-Dong X, Yang X, Lu JL, et al. Development and Validation of a Nine-Redox-Related Long Noncoding RNA Signature in Renal Clear Cell Carcinoma. Oxid Med Cell Longev 2020;2020:6634247. [Crossref] [PubMed]
- Chen X, Song J, Wang X, et al. LncRNA LINC00460: Function and mechanism in human cancer. Thorac Cancer 2022;13:3-14. [Crossref] [PubMed]
- Jiang H, Chen H, Wan P, et al. Downregulation of enhancer RNA EMX2OS is associated with poor prognosis in kidney renal clear cell carcinoma. Aging (Albany NY) 2020;12:25865-77. [Crossref] [PubMed]
- Gu Y, Feng C, Liu T, et al. The downregulation of lncRNA EMX2OS might independently predict shorter recurrence-free survival of classical papillary thyroid cancer. PLoS One 2018;13:e0209338. [Crossref] [PubMed]
- Liu GX, Tan YZ, He GC, et al. EMX2OS plays a prognosis-associated enhancer RNA role in gastric cancer. Medicine (Baltimore) 2021;100:e27535. [Crossref] [PubMed]
- Zhang G, Wang Q, Lu J, et al. Long non-coding RNA FLJ22763 is involved in the progression and prognosis of gastric cancer. Gene 2019;693:84-91. [Crossref] [PubMed]