Ferroptosis and cuproptosis prognostic signature for prediction of prognosis, immunotherapy and drug sensitivity in hepatocellular carcinoma: development and validation based on TCGA and ICGC databases
Highlight box
Key findings
• A prognostic model consisting of nine genes (TXNRD1, FTL, GPX4, PRDX1, VDAC2, OTUB1, NRAS, SLC38A1, and SLC1A5) is a strong predictor of hepatocellular carcinoma.
What is known and what is new?
• Ferroptosis and cuproptosis are known to play a key role in the development and metastasis of hepatocellular carcinoma;
• This study combined ferroptosis- with cuproptosis-related genes to construct a prognostic model and found that the new model correlated with prognosis, immunotherapy and drug sensitivity in patients with hepatocellular carcinoma.
What is the implication, and what should change now?
• This study highlights the importance of ferroptosis- with cuproptosis-related genes in clinical practice and implies that ferroptosis and cuproptosis may be a therapeutic priority for patients with hepatocellular carcinoma.
Introduction
Hepatocellular carcinoma (HCC) is one of the most prevalent types of cancerous tumors found all over the world (1), with a significant morbidity and fatality rate (2). Despite surgical resection, interventional therapy, chemoradiotherapy, targeted pharmacological therapy, and liver transplantation currently are the leading treatments for HCC (3), the overall survival (OS) rate for individuals with HCC remains suboptimal (4). The potential for multigene signature to aid in risk stratification and prognosis prediction in HCC is being supported by mounting research (5-7). However, most of these signatures employ only a single gene set, which is slightly less predictive for the complex pathogenesis of tumors. Consequently, the objective of this work is to develop a ferroptosis and cuproptosis signature to forecast HCC patients’ OS.
To put it simply, ferroptosis is a novel kind of programmed cellular death marked by lipid peroxidation that is exceptionally iron-dependent (8). Ferroptosis has garnered substantial interest as a potential cancer treatment pathway since its introduction in 2012 (9). Extensive research has uncovered the essential function of ferroptosis in destroying tumor cells and preventing their progression (10,11). Consequently, inducing ferroptosis has shown promise as a therapeutic technique for hastening the death of cancer cells (12). Numerous genes that play roles in regulating or revealing the presence of ferroptosis have been discovered. For instance, the up-regulation of ACSL4 stimulates tumor expansion and proliferation, but also increases vulnerability to ferroptosis (13). The P53 tumor suppressor, a key repressor of cancer development, is also closely linked to ferroptosis. Ferroptosis and lipid metabolism cooperate to contribute to p53-mediated tumor suppression (14,15).
Copper is a crucial mineral that aids in many different bodily processes. Compared to healthy people, cancer patients have much elevated degree of copper in their bloodstream and tumor tissue, according to studies (16,17). Additionally, copper levels within cells may have a role in cancer’s advancement and growth (18). In a recent study published in Science, the term “cuproptosis” was used to characterize a newly discovered way of copper-induced cell death (19). By forming a covalent bond with the lipoacylated components of the tricarboxylic acid (TCA) cycle, copper induces a toxic protein stress that ultimately leads to cell death that is not mediated by the apoptotic pathway (18). This illustrates the enormous anticancer potential of copper against malignancies that are innately resistant to apoptosis.
Understanding ferroptosis and cuproptosis better is essential for the progression and management of HCC. However, it is mostly undetermined whether these ferroptosis-related genes (FRGs) and cuproptosis-related genes (CRGs) link with HCC patient prognosis. Therefore, we investigated the genome of HCC and thousands of biological targets using high-throughput methodological tools to find FRGs and CRGs that are strongly connected with HCC prognosis and to develop predictive signatures for forecasting HCC patients’ outcomes. Our findings demonstrated that our signature had a beneficial purpose in assessing the responses to immunotherapy, and medication sensitivity for patients with HCC in various subgroups, and that they exhibited outstanding predictive performance for prognosis. We present the following article in accordance with the TRIPOD reporting checklist (available at https://tcr.amegroups.com/article/view/10.21037/tcr-22-2203/rc).
Methods
Data collection
The Cancer Genome Atlas (TCGA) database (https://portal.gdc.cancer.gov) was mined for the mRNA expression and pertinent clinical information of 50 normal hepatocellular tissues and 374 HCC tissues. For external validation, we also retrieved 242 HCC samples from the International Cancer Genome Consortium (ICGC) database (https://dcc.icgc.org/projects/LIRI-JP). Both TCGA and ICGC data are openly accessible to the scientific community. As a result, the current study did not need permission from regional ethics boards. The 259 FRGs were retrieved from the FerrDb website (http://www.zhounan.org/ferrdb/) and prior publications. The 13 CRGs were acquired from the previous top research. For future studies, we compiled this list of genes after searching the literature for relevant articles, eliminating irrelevant ones, and adding in any newly published ones. The study was conducted in accordance with the Declaration of Helsinki (as revised in 2013).
Identification of differentially expressed genes (DEGs)
The mRNA expression data were matched to FRG and CRG, and the cutoff criteria of corrfilter >0.3 and P<0.05 were set to analyze the correlation between CRG and FRG. For the differential expression analysis using the “limma” R package, we looked for DEGs associated with ferroptosis and cuproptosis with the appropriate cut-off criteria: |logFC| >1 and false discovery rate (FDR) <0.05.
Protein-protein interaction (PPI) and functional enrichment analyses
To collect information on PPIs, DEGs were uploaded to the STRING database (http://www.string-db.org/). With the help of Cytoscape (3.7.2), the PPI network was built and mapped out. We utilized the MCODE (1.6.1) plug-in to identify significant nodes (MCODE score ≥8) and built the core target interaction network. The “ClusterProfiler” program was used to analyze Gene Ontology (GO) terms such as molecular function (MF), biological process (BP), and cellular component (CC). Using the same methodology, the Kyoto Encyclopedia of Genes and Genomes (KEGG) pathway analysis was undertaken. Significant enrichment was considered to exist when both the FDR and P<0.05 were met.
Establishment and validation of a predictive signature for prognosis
The TCGA-HCC samples are split into a “7:3” ratio of a training risk set and an internal test risk set using the “caret” R package. In the meantime, the ICGC sample serves as an external validation data set. Univariate Cox regression analysis was used to assess DEGs’ prognostic value, whereas least absolute shrinkage and selection operator (LASSO) regression analysis was used to generate a model for assessing patient prognosis in HCC. This calculator was used to determine the risk levels: risk score = (Coef 1 * expression mRNA 1) + (Coef 2 * expression mRNA 2) + (Coef n * expression mRNA n). The median value of each patient’s risk score was used to classify them as either high-risk or low-risk for the condition being studied. Kaplan-Meier (K-M) analysis was utilized so that a comparison could be made between the two groups’ projected survival times. The prognostic accuracy of the risk model was calculated using a receiver operating characteristic (ROC) analysis that took into account the passage of time using the “survivalROC” R package. Theoretically, a good prediction model should have an area under the curve (AUC) value greater than 0.5. The predictive ability and utility of this HCC signature were also validated by using an internal validation set (n=106) and an external validation set (n=242).
Gene Set Enrichment Analysis (GSEA)
GSEA was performed to determine whether there were any biochemical processes or functioning paths that might be associated with the FRGs and CRGs signature. The cutoffs for significance were set at a P value of less than 0.05 and a FDR of less than 0.25.
Construction of a nomogram using risk score and clinical factors
We investigated the relationship between FRGs and CRGs signature and Clinical variables. We incorporated other clinical variables discovered by multivariate Cox regression and utilized the “rms” R package to develop an outcome-related prediction nomogram for estimating the probability of 1-, 3-, and 5-year OS in HCC patients, as well as the associated calibration plots. The prediction effect was larger when the nomogram had a better prognostic ability and the calibration curve was closer to the 45° line.
Immune cell infiltration analysis
Using CIBERSORT, CIBERSORT-ABS, MCP-counter, QUANTISEQ, TIMER, XCELL, and EPIC algorithms, we compared the level of immune cell infiltration across groups with high and low risk. We looked into the expression of several immunological checkpoints such CD274, SIRPA, CD27, CTLA4, HAVCR2, PDCD1, PDCD1LG2, and TIGIT to better predict the success of immune checkpoint blocking therapy. In addition, the interplay between immune cells and a total of nine FRGs and CRGs was investigated with the assistance of the TIMER database (https://cistrome.shinyapps.io/timer/).
Screening for potential small molecule drugs
The Drug Gene Interaction Database 4.0 (DGIdb, https://www.dgidb.org) was utilized to identify prospective medicines for HCC. We explored the DGIdb to detect prospective medications or chemical compounds that interacted with the FRGs and CRGs, and used the Cytoscape software to display the network of drug-gene interactions that existed between the two.
Drug sensitivity analysis
Half-maximal inhibitory concentration (IC50) values were used to predict drug sensitivity using the Genomics of Drug Sensitivity in Cancer (GDSC) (http://www.cancerrxgene.org) database, while the “pRRophetic” package was used to examine the difference in sensitivity between two groups of pharmaceuticals.
Comparison with published models
Our model’s prediction power was tested against that of 12 other models already in circulation. Multivariate Cox regression analysis was used to produce a risk score for each sample to guarantee that they were representative of the whole. Based on the median risk score, the samples were sorted into high-risk and low-risk categories. Following incorporation of the pertinent genes into each of the 12 models, the ROC curve was generated.
Statistical analysis
The following packages were used in R software (version 4.1.3), for data analysis and visualization purposes: “tidyverse”, “limma”, “pheatmap”, “survival”, “survminer”, “caret”, “glmnet”, “timeROC”, “regplot”, “rms”, “org.Hs.eg.db”, “clusterProfiler”, “enrichplot”, “DOSE”, “ggplot2”, “readr”, “ggpubr”, “pRRophetic”, “genefilter”, “preprocessCore”, and “ridge”. When the probability value was less than 0.05, it was considered significant.
Results
Establishment and validation of FRGs and CRGs signature
Based on the analysis of the correlation, we discovered 158 FRGs and CRGs. The PPI network of these genes indicates that FRGs and CRGs have substantial connections (Figure 1A). Compared to normal liver tissues, the TCGA-HCC dataset identifies 60 genes, 11 of which are down-regulated and 49 of which are up-regulated, as DEGs (Figure 1B). Then, relying on these DEGs, we utilized univariate Cox regression analysis to establish whether or not these DEGs had prognostic value, and found that just 15 of them were indeed useful (Figure 1C).
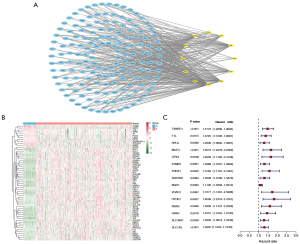
Additionally, we used LASSO regression analysis to create a signature for estimating the outcome of HCC prognosis. Nine genes were successfully incorporated into a risk model (TXNRD1, FTL, GPX4, PRDX1, VDAC2, OTUB1, NRAS, SLC38A1, and SLC1A5). By plugging their coefficients into the following equation, we were able to calculate the risk score associated with these nine genes (Table 1): risk score = (0.175 × TXNRD1 expression) +(0.1245 × FTL expression) + (0.4628 × GPX4 expression) + (0.0366 × PRDX1 expression) + (0.2921 × VDAC2 expression) + (0.0450 × OTUB1 expression) + (0.2476 × NRAS expression) + (0.126 × SLC38A1 expression) + (0.0223 × SLC1A5 expression). The median cutoff value was applied for determining whether or not a patient posed a high or low danger. Mortality rates were dramatically higher in the high-risk group compared to the low-risk group (P<0.001). This was supported by the findings of the K-M analysis, which demonstrated a deteriorating relationship between the risk score and the outcome of the condition. Moreover, the ROC curve revealed that OS prediction using the risk score was possible and accurate. The AUCs for 1-, 3- and 5-year survival were 0.751, 0.727, and 0.743, respectively (Figure 2A-2D). The signature’s predictive power was examined by subjecting it to both internal validation depending on the TCGA and external validation relying on the ICGC. The outcomes corresponded with the signature group. In the internal validation cohort, the high-risk group had considerably more deaths than the low-risk group (P<0.001). Specifically, values of the AUC were 0.826 at 1 year, 0.624 at 3 years, and 0.589 at 5 years (Figure 3A-3D). Within the external validation group, there were more deaths in the high-risk category than the low-risk category (P<0.05). The AUC was 0.699 for a survival rate after 1 year, 0.702 after 3 years, and 0.568 after 5 years (Figure 4A-4D).
Table 1
Gene symbol | Coefficient |
---|---|
TXNRD1 | 0.1753 |
FTL | 0.1245 |
GPX4 | 0.4628 |
PRDX1 | 0.0366 |
VDAC2 | 0.2921 |
OTUB1 | 0.0450 |
NRAS | 0.2476 |
SLC38A1 | 0.1266 |
SLC1A5 | 0.0223 |
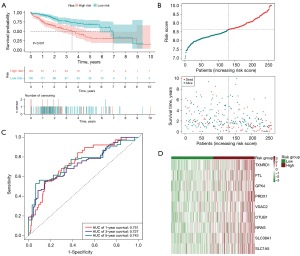
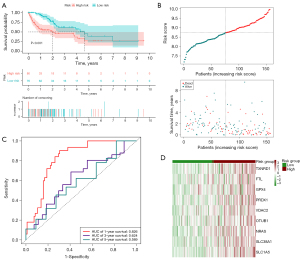
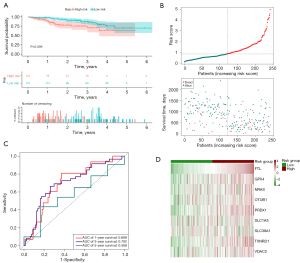
Prognostic significance of the signature in HCC
Using both univariate and multivariate Cox regression models, we set out to determine whether or not this signature possesses the ability to function on its own as a prognostic factor. In a univariate analysis, the risk score and Tumor Node Metastasis (TNM) stage were revealed to be significant predictors of survival among HCC patients (P<0.001) (Figure 5A); even after accounting for other factors, multivariate analysis showed a statistically significant (P<0.001) correlation between the risk score and TNM stage and survival (Figure 5B). The findings indicated that the 9-gene signature might be employed independently in OS prognosis.
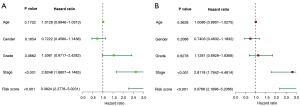
Connection between clinical characteristics and signature
We utilized the Chi-square test to determine if the signature was related to clinical factors (Figure 6A,6B). Statistically, there was a distinction between high- and low-risk groups in terms of grade (P<0.05), but not in terms of TNM stage, T stage, age, or gender (P>0.05). The signature’s predictive significance in distinct classes was then explored by means of stratification analysis. Our signature performed well in predicting outcomes for those who were ≤65 years (P=0.007), >65 years (P=0.005), male (P<0.001), in grade 1–2 (P=0.026), in grade 3–4 (P=0.007), and in T1–T2 (P<0.001), but it was less effective for those in T3–T4 and female (P>0.05, Figure 7).
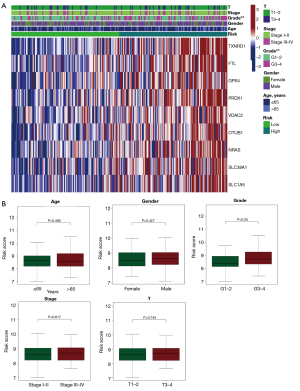
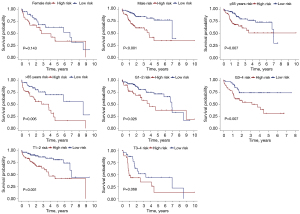
Nomogram-based validation of the signature’s prognostic value in HCC
To validate the prognostic utility of FRGs and CRGs in HCC, we designed nomograms to forecast 1-, 3-, and 5-year OS based on the aforementioned genes (Figure 8A). A strong correlation could be seen between the data and the calibration curves (Figure 8B). The C-index of the nomograms was 0.697. The C-index of the risk score was found to be relatively larger with time, proving high levels of accuracy in their predictions (Figure 8C).
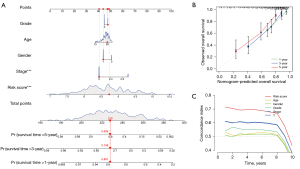
Analyses of functional enrichment and PPIs
To elucidate the probable function of 60 DEGs, we carried the enrichment analysis using both GO and KEGG. The outcomes of BP analyses revealed that 60 DEGs were significantly engaged in cellular reaction to chemical stress, cellular reaction to oxidative stress, reaction to nutritional levels, and peptidyl-serine phosphorylation. CC study pointed to melanosome, pigment granule, late endosome and caveola. Analysis of MF revealed that ferrous iron binding, antioxidant activity, mitogen-activated protein (MAP) kinase activity, and ferric iron binding were primarily enriched (Figure 9A). According to KEGG analysis, autophagy-animal, vascular endothelial growth factor (VEGF) signaling, central carbon metabolism in cancer, and mTOR signaling pathway were significantly enriched (Figure 9B). Using the STRING database to illustrate the PPI network, the result showed that the PPI network of the 60 DEGs comprised of 52 nodes and 120 edges. The top modules, consisting of 8 nodes (MCODE score =8), was discovered using MCODE (Figure 9C).
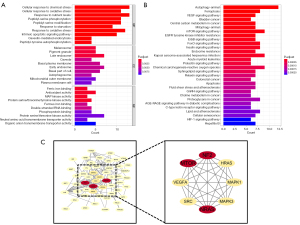
GSEA
To further specify the molecular process behind this signature, a GSEA analysis was conducted. To further specify the molecular process behind this signature, a GSEA analysis was conducted. 2-oxocarboxylic acid metabolism, arginine biosynthesis, cocaine addiction, non-small cell lung cancer, and other glycan degradation were concentrated in the high-risk group, according to the results of the GSEA (Figure S1). Endocytosis, human T-cell leukemia virus 1 infection, neurodegenerative pathway, PI3K-Akt signaling pathway, and protein processing in endoplasmic reticulum were most prevalent in the low-risk group (Figure S1).
Immune infiltration analyses
The heatmap illustrated the association between the signature and immunological infiltration (Figure 10A). According to the findings of CIBERSORT, the fraction of M0 macrophages was much larger in individuals who were considered to be at high risk. In low-risk groups, CD8+ T cells and M1 macrophages were more prevalent than naive B cells (P<0.05) (Figure 10B). In light of the importance of checkpoint blocker immunotherapies, we selected additional immune checkpoints (CD274, SIRPA, CD27, CTLA4, HAVCR2, PDCD1, PDCD1LG2, and TIGIT) to examine the relationship between immune checkpoints and risk scores. Extreme differences were seen in the levels of PDCD1, CTLA4, HAVCR2, TIGIT, SIRPA, and CD27 expression between the two patient groups (Figure 10C).
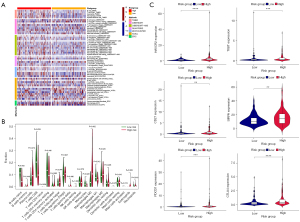
TIMER analysis
We dug deeper into the connection between nine FRGs and CRGs and immune cells by using the TIMER online database. VDAC2, GPX4, NRAS, and OTUB1 were positively linked with tumor purity, according to the conclusions. Multiple immune cells, including CD8+ T cells, CD4+ T cells, macrophages, neutrophils, and dendritic cells, had positive associations with VDAC2, NRAS, OTUB1, SLC1A5, SLC38A1, and TXNRD1. Negative associations were found between FTL and CD8+ T cells, CD4+ T cells, macrophages, neutrophils, and dendritic cells. A positive association was observed between GPX4 and CD4+ T cells, and negative correlations were observed between GPX4 and CD8+ T cells, macrophages, neutrophils, and dendritic cells. CD8+ T cells, macrophages, neutrophils, and dendritic cells were shown to have positive correlations with PRDX1, while CD4+ T cells were found to have negative correlations with PRDX1 (Figure S2).
Screening for potential drugs
When DGIdb was used to investigate drug-gene interactions, a total of 52 medicines were found to have therapeutic promise for HCC patients (Figure 11). In all, 47 of these medications, including Metformin, Panitumumab, Trametinib, Obatoclax, and Osimertinib, were found to have an interaction with NRAS. TXNRD1 was involved in pharmacological interactions with arsenic trioxide, spermidine, and fotemustine. Both VDAC2 and SLC1A5 were found to interact with Olesoxime and Glutamine, respectively.
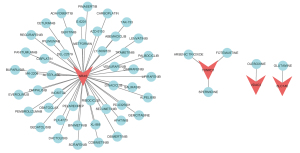
Drug sensitivity analysis
Twelve chemotherapeutic medications were selected for further study to better understand the discrepancies in sensitivity between the two groups of patients and to improve the survival of HCC patients. Patients in the high-risk group exhibited higher IC50 values for medications such as BI.2536, Epothilone.B, Gemcitabine, Mitomycin.C, Sunitinib, Obatoclax.Mesylate, and Vinorelbine than those in the low-risk group, indicating that these patients were considerably more susceptible to these drugs. Patients in the low-risk group were better able to respond to ABT.888, Nilotinib, IPA.3, ATRA, and Doxorubicin, as evidenced by their significantly lower IC50 values (Figure 12).
New model as a new predictor of HCC
We compared our own model to 12 other published HCC prognostic models to better show its predictive power (20-31). Multivariate analysis was used to calculate the risk value and prognostic evaluation for each dataset, leveling the playing field amongst the four models. High-risk individuals had a dismal prognosis, as evidenced by the survival curves for all four models (Figure S3). The ROC curve shows that when compared to our model, the AUC values of the other models are lower (Figure S3). This fact alone demonstrates the improved prediction performance of our model. Then, we created the restricted mean survival (RMS) package to calculate the C-index for each prognostic indicator. Our model’s C-index of 0.697 was greater than that of the other models (Figure S3). RMS can be used to assess the impact of gene features across time on prediction. Our genetic traits were at their peak performance around the sixth year (Figure S3). This indicates that our model is a great predictor when compared to other models.
Discussion
As high-throughput sequencing technology develops, a wide range of diagnostic biomarkers and therapeutic targets are being identified (32). mRNAs, miRNAs, long noncoding RNAs (lncRNAs) and circular RNAs (circRNAs) are some examples of potential biomarkers for the diagnosis and prognosis of cancer (33,34). Prior research has established that ferroptosis and cuproptosis are effective strategies for triggering HCC cell apoptosis; however, their precise molecular alterations and mechanisms of action remain unknown (35,36). In order to better the early identification and treatment of HCC and consequently its clinical prognosis, it is necessary to clarify the clinical importance of ferroptosis and cuproptosis in HCC.
We evaluated the TCGA database for 60 FRGs and CRGs that were differently expressed across HCC samples and normal liver tissues. We investigated the biological pathways of 60 genes systematically and developed PPI networks. Using univariable and lasso-penalized regression analysis, we subsequently identified 9 FRGs and CRGs that were linked with HCC prognosis. On the basis of these nine genes, we developed and validated risk models for the outcomes. Analyses of survival and ROC indicated that the model possessed strong ability to predict. Univariate and multivariate Cox regression analyses corroborated the 9-gene signature’s prognostic power for OS. In addition, we found that the signature was strongly linked to the infiltration of immunocytic, and the efficacy of 52 prospective drugs was assessed for the treatment of HCC individuals.
Go analysis revealed that 60 FRGs and CRGs were predominantly enriched for ferric iron binding, antioxidant activity, MAP kinase activity, and ferrous iron binding; KEGG analysis revealed that autophagy-animal, VEGF signaling pathway, central carbon metabolism in cancer, and mTOR signaling pathway were predominantly enriched. These mechanisms are intimately associated with angiogenesis, one-carbon metabolism, and the malignant phenotype of HCC (37-39). This provided evidence that these genes may play a function in tumor development.
A number of malignancies, including lung cancer (40), esophageal cancer (41), and HCC (42) have been linked to TXNRD1, and this gene has been proven to have a role in their development. TXNRD1 overexpression in HCC cells and tissues is an indicator of a terrible prognosis for HCC patients (43). The mechanism may accelerate the development of HCC cells by downregulating PCK1 via the Nrf2/Keap1 pathway (42). Histological examination of hepatocellular cancer specimens revealed high levels of FTL expression (44), and it figured prominently in HCC apoptosis and iron metabolism (45,46). The selenoenzyme glutathione peroxidase (GPX4) has been identified through genetic investigations in cells and animals as a crucial regulator of ferroptosis (47). Improved sorafenib-induced ferroptosis in HCC cells is mediated by GSTZ1, which acts by blocking the NRF2/GPX4 axis (48). The peroxidase (PRDX) family is widely considered to be the most successful group of enzymes across the evolutionary divide between bacteria, archaea, and eukaryotes (49). The prognosis of HCC patients has been shown to worsen in correlation with high PRDX1 expression (50). VDAC2 is now a non-BCL-2 family protein that is essential for the execution of BAX- and BAK-directed apoptosis in mitochondria (51). As a result, a medication targeting BAK’s interaction with VDAC2 could be useful in treating cancer. The expression of OTUB1 was a novel and independent prognostic indicator for HCC patients. It altered tumor immune cell infiltration and influenced apoptosis and autophagy considerably (52). Sorafenib-resistant HCC cells exhibited considerably higher NRAS expression compared to non-resistant HCC cells (53), suggesting that NRAS expression may contribute to HCC treatment resistance. Overexpression of NRAS was also linked to decreased survival and proliferation in vivo (54). Solute carrier family 38 member 1 (SLC38A1) is one of the primary transporters of glutamine (55), and it has been demonstrated that overexpression of SLC38A1 in malignant tumors promotes tumor cell proliferation, invasion, and metastasis (56-58). The SLC1A5 variation carried glutamine into mitochondria, which plays a crucial role in cancer’s metabolic reprogramming. Knockdown and overexpression of the SLC1A5 variant altered tumor development and enhanced their carcinogenic properties (59).
Based on the findings of the GSEA study, it was hypothesized that the signature linked with ferroptosis and cuproptosis primarily involved metabolic and apoptosis-related pathways. Some examples of these pathways are the 2-oxocarboxylic acid metabolism, the PI3K-Akt signaling system, and the tumor necrosis factor (TNF) signaling route.
Our results are in line with the notion that those at low risk have more CD8+ T cells, as CD8+ T cells are the main “effector” cells in the battle against cancer. Activated CD8+ T lymphocytes killed tumor cells by recognizing antigens on major histocompatibility complex I (MHC I) that were linked with tumors through the use of their expressed T cell receptors (60). Differential expression of PDCD1, CTLA4, HAVCR2, CD276, and CD80 between high-risk and low-risk groups further supports the idea that a suppressed immunological milieu contributes to the poor prognosis of high-risk patients. In addition, it was discovered that ABT.888, Nilotinib, IPA.3, ATRA, and Doxorubicin would be beneficial for patients in the low-risk group, whereas BI.2536, Epothilone.B, Gemcitabine, Mitomycin.C, Obatoclax.Mesylate, Sunitinib, and Vinorelbine might be beneficial for patients in the high-risk group.
In the end, we chose 12 prognostic risk models for HCC from the literature and compared their ability to predict outcomes. The prediction performance of our model was quite high. However, there are still certain restrictions on current research. To begin with, all inferences are made based on the processing and analysis of data obtained from public databases, and there is a lack of clinical data and experimental research to further verify the results. In the future, it will be necessary to collect more HCC cases and conduct many prospective clinical assessments to further evaluate the model’s efficacy in clinical settings.
Conclusions
We conducted a thorough evaluation of CRGs and FRGs, and their utility in the study of clinical features, and HCC prognosis was successfully demonstrated. Results like these highlight the potential clinical significance of CRGs and FRGs and raise the possibility that ferroptosis and cuproptosis could be a therapeutic focus for HCC patients.
Acknowledgments
Funding: The study was supported by fundings from Gansu Province Science and Technology Foundation (No. 18JR2FA001) and Gansu Province Education Science and Technology Innovation Project (No. 2022CXZX-756).
Footnote
Reporting Checklist: The authors have completed the TRIPOD reporting checklist. Available at https://tcr.amegroups.com/article/view/10.21037/tcr-22-2203/rc
Peer Review File: Available at https://tcr.amegroups.com/article/view/10.21037/tcr-22-2203/prf
Conflicts of Interest: All authors have completed the ICMJE uniform disclosure form (available at https://tcr.amegroups.com/article/view/10.21037/tcr-22-2203/coif). The authors have no conflicts of interest to declare.
Ethical Statement: The authors are accountable for all aspects of the work in ensuring that questions related to the accuracy or integrity of any part of the work are appropriately investigated and resolved. The study was conducted in accordance with the Declaration of Helsinki (as revised in 2013).
Open Access Statement: This is an Open Access article distributed in accordance with the Creative Commons Attribution-NonCommercial-NoDerivs 4.0 International License (CC BY-NC-ND 4.0), which permits the non-commercial replication and distribution of the article with the strict proviso that no changes or edits are made and the original work is properly cited (including links to both the formal publication through the relevant DOI and the license). See: https://creativecommons.org/licenses/by-nc-nd/4.0/.
References
- Yang JD, Heimbach JK. New advances in the diagnosis and management of hepatocellular carcinoma. BMJ 2020;371:m3544. [Crossref] [PubMed]
- Villanueva A. Hepatocellular Carcinoma. N Engl J Med 2019;380:1450-62. [Crossref] [PubMed]
- Feng D, Wang M, Hu J, et al. Prognostic value of the albumin-bilirubin grade in patients with hepatocellular carcinoma and other liver diseases. Ann Transl Med 2020;8:553. [Crossref] [PubMed]
- Li W, Chen QF, Huang T, et al. Identification and Validation of a Prognostic lncRNA Signature for Hepatocellular Carcinoma. Front Oncol 2020;10:780. [Crossref] [PubMed]
- Liang JY, Wang DS, Lin HC, et al. A Novel Ferroptosis-related Gene Signature for Overall Survival Prediction in Patients with Hepatocellular Carcinoma. Int J Biol Sci 2020;16:2430-41. [Crossref] [PubMed]
- Wang Y, Song F, Zhang X, et al. Mitochondrial-Related Transcriptome Feature Correlates with Prognosis, Vascular Invasion, Tumor Microenvironment, and Treatment Response in Hepatocellular Carcinoma. Oxid Med Cell Longev 2022;2022:1592905. [Crossref] [PubMed]
- Lin Z, Xu Q, Miao D, et al. An Inflammatory Response-Related Gene Signature Can Impact the Immune Status and Predict the Prognosis of Hepatocellular Carcinoma. Front Oncol 2021;11:644416. [Crossref] [PubMed]
- Qiu Y, Cao Y, Cao W, et al. The Application of Ferroptosis in Diseases. Pharmacol Res 2020;159:104919. [Crossref] [PubMed]
- Dixon SJ. Ferroptosis: bug or feature? Immunol Rev 2017;277:150-7. [Crossref] [PubMed]
- Mou Y, Wang J, Wu J, et al. Ferroptosis, a new form of cell death: opportunities and challenges in cancer. J Hematol Oncol 2019;12:34. [Crossref] [PubMed]
- Hassannia B, Vandenabeele P, Vanden Berghe T. Targeting Ferroptosis to Iron Out Cancer. Cancer Cell 2019;35:830-49. [Crossref] [PubMed]
- Liang C, Zhang X, Yang M, et al. Recent Progress in Ferroptosis Inducers for Cancer Therapy. Adv Mater 2019;31:e1904197. [Crossref] [PubMed]
- Yuan H, Li X, Zhang X, et al. Identification of ACSL4 as a biomarker and contributor of ferroptosis. Biochem Biophys Res Commun 2016;478:1338-43. [Crossref] [PubMed]
- Xie Y, Zhu S, Song X, et al. The Tumor Suppressor p53 Limits Ferroptosis by Blocking DPP4 Activity. Cell Rep 2017;20:1692-704. [Crossref] [PubMed]
- Tarangelo A, Magtanong L, Bieging-Rolett KT, et al. p53 Suppresses Metabolic Stress-Induced Ferroptosis in Cancer Cells. Cell Rep 2018;22:569-75. [Crossref] [PubMed]
- Blockhuys S, Celauro E, Hildesjö C, et al. Defining the human copper proteome and analysis of its expression variation in cancers. Metallomics 2017;9:112-23. [Crossref] [PubMed]
- Ishida S, Andreux P, Poitry-Yamate C, et al. Bioavailable copper modulates oxidative phosphorylation and growth of tumors. Proc Natl Acad Sci U S A 2013;110:19507-12. [Crossref] [PubMed]
- Davis CI, Gu X, Kiefer RM, et al. Altered copper homeostasis underlies sensitivity of hepatocellular carcinoma to copper chelation. Metallomics 2020;12:1995-2008. [Crossref] [PubMed]
- Tsvetkov P, Coy S, Petrova B, et al. Copper induces cell death by targeting lipoylated TCA cycle proteins. Science 2022;375:1254-61. [Crossref] [PubMed]
- Chen X, Hu G, Xiong L, et al. Relationships of Cuproptosis-Related Genes With Clinical Outcomes and the Tumour Immune Microenvironment in Hepatocellular Carcinoma. Pathol Oncol Res 2022;28:1610558. [Crossref] [PubMed]
- Li W, Liu J, Zhang D, et al. The Prognostic Significance and Potential Mechanism of Ferroptosis-Related Genes in Hepatocellular Carcinoma. Front Genet 2022;13:844624. [Crossref] [PubMed]
- Long S, Chen Y, Wang Y, et al. Identification of Ferroptosis-related molecular model and immune subtypes of hepatocellular carcinoma for individual therapy. Cancer Med 2022; Epub ahead of print. [Crossref] [PubMed]
- Luo L, Yao X, Xiang J, et al. Identification of ferroptosis-related genes for overall survival prediction in hepatocellular carcinoma. Sci Rep 2022;12:10007. [Crossref] [PubMed]
- Wang G, Xiao R, Zhao S, et al. Cuproptosis regulator-mediated patterns associated with immune infiltration features and construction of cuproptosis-related signatures to guide immunotherapy. Front Immunol 2022;13:945516. [Crossref] [PubMed]
- Wang H, Yang C, Jiang Y, et al. A novel ferroptosis-related gene signature for clinically predicting recurrence after hepatectomy of hepatocellular carcinoma patients. Am J Cancer Res 2022;12:1995-2011. [PubMed]
- Wang W, Pan F, Lin X, et al. Ferroptosis-Related Hub Genes in Hepatocellular Carcinoma: Prognostic Signature, Immune-Related, and Drug Resistance Analysis. Front Genet 2022;13:907331. [Crossref] [PubMed]
- Wang XX, Wu LH, Ji H, et al. A novel cuproptosis-related prognostic signature and potential value in HCC immunotherapy. Front Mol Biosci 2022;9:1001788. [Crossref] [PubMed]
- Zhang B, Zhao J, Liu B, et al. Development and Validation of a Novel Ferroptosis-Related Gene Signature for Prognosis and Immunotherapy in Hepatocellular Carcinoma. Front Mol Biosci 2022;9:940575. [Crossref] [PubMed]
- Zhang Y, Ren H, Zhang C, et al. Development and validation of four ferroptosis-related gene signatures and their correlations with immune implication in hepatocellular carcinoma. Front Immunol 2022;13:1028054. [Crossref] [PubMed]
- Zhao C, Zhang Z, Jing T. A novel signature of combing cuproptosis- with ferroptosis-related genes for prediction of prognosis, immunologic therapy responses and drug sensitivity in hepatocellular carcinoma. Front Oncol 2022;12:1000993. [Crossref] [PubMed]
- Zhao C, Zhang Z, Tao J. A Novel Ferroptosis-Related Signature for Prediction of Prognosis, Immune Profiles and Drug Sensitivity in Hepatocellular Carcinoma Patients. Curr Oncol 2022;29:6992-7011. [Crossref] [PubMed]
- Xu JH, Guan YJ, Qiu ZD, et al. System Analysis of ROS-Related Genes in the Prognosis, Immune Infiltration, and Drug Sensitivity in Hepatocellular Carcinoma. Oxid Med Cell Longev 2021;2021:6485871. [Crossref] [PubMed]
- Tsuchiya N, Sawada Y, Endo I, et al. Biomarkers for the early diagnosis of hepatocellular carcinoma. World J Gastroenterol 2015;21:10573-83. [Crossref] [PubMed]
- De Stefano F, Chacon E, Turcios L, et al. Novel biomarkers in hepatocellular carcinoma. Dig Liver Dis 2018;50:1115-23. [Crossref] [PubMed]
- Louandre C, Ezzoukhry Z, Godin C, et al. Iron-dependent cell death of hepatocellular carcinoma cells exposed to sorafenib. Int J Cancer 2013;133:1732-42. [Crossref] [PubMed]
- Cobine PA, Brady DC. Cuproptosis: Cellular and molecular mechanisms underlying copper-induced cell death. Mol Cell 2022;82:1786-7. [Crossref] [PubMed]
- Morse MA, Sun W, Kim R, et al. The Role of Angiogenesis in Hepatocellular Carcinoma. Clin Cancer Res 2019;25:912-20. [Crossref] [PubMed]
- Mora MI, Molina M, Odriozola L, et al. Prioritizing Popular Proteins in Liver Cancer: Remodelling One-Carbon Metabolism. J Proteome Res 2017;16:4506-14. [Crossref] [PubMed]
- Fang G, Zhang P, Liu J, et al. Inhibition of GSK-3β activity suppresses HCC malignant phenotype by inhibiting glycolysis via activating AMPK/mTOR signaling. Cancer Lett 2019;463:11-26. [Crossref] [PubMed]
- Guo Q, Yan J, Song T, et al. microRNA-130b-3p Contained in MSC-Derived EVs Promotes Lung Cancer Progression by Regulating the FOXO3/NFE2L2/TXNRD1 Axis. Mol Ther Oncolytics 2020;20:132-46. [Crossref] [PubMed]
- Li X, Song L, Wang B, et al. Circ0120816 acts as an oncogene of esophageal squamous cell carcinoma by inhibiting miR-1305 and releasing TXNRD1. Cancer Cell Int 2020;20:526. [Crossref] [PubMed]
- Tuo L, Xiang J, Pan X, et al. PCK1 Downregulation Promotes TXNRD1 Expression and Hepatoma Cell Growth via the Nrf2/Keap1 Pathway. Front Oncol 2018;8:611. [Crossref] [PubMed]
- Fu B, Meng W, Zeng X, et al. TXNRD1 Is an Unfavorable Prognostic Factor for Patients with Hepatocellular Carcinoma. Biomed Res Int 2017;2017:4698167. [Crossref] [PubMed]
- Ke S, Wang C, Su Z, et al. Integrated Analysis Reveals Critical Ferroptosis Regulators and FTL Contribute to Cancer Progression in Hepatocellular Carcinoma. Front Genet 2022;13:897683. [Crossref] [PubMed]
- Fan Y, Yamada T, Shimizu T, et al. Ferritin expression in rat hepatocytes and Kupffer cells after lead nitrate treatment. Toxicol Pathol 2009;37:209-17. [Crossref] [PubMed]
- Wang L, Ouyang S, Li B, et al. GSK-3β manipulates ferroptosis sensitivity by dominating iron homeostasis. Cell Death Discov 2021;7:334. [Crossref] [PubMed]
- Seibt TM, Proneth B, Conrad M. Role of GPX4 in ferroptosis and its pharmacological implication. Free Radic Biol Med 2019;133:144-52. [Crossref] [PubMed]
- Wang Q, Bin C, Xue Q, et al. GSTZ1 sensitizes hepatocellular carcinoma cells to sorafenib-induced ferroptosis via inhibition of NRF2/GPX4 axis. Cell Death Dis 2021;12:426. [Crossref] [PubMed]
- Knoops B, Argyropoulou V, Becker S, et al. Multiple Roles of Peroxiredoxins in Inflammation. Mol Cells 2016;39:60-4. [Crossref] [PubMed]
- Xu M, Xu J, Zhu D, et al. Expression and prognostic roles of PRDXs gene family in hepatocellular carcinoma. J Transl Med 2021;19:126. [Crossref] [PubMed]
- Yuan Z, Dewson G, Czabotar PE, et al. VDAC2 and the BCL-2 family of proteins. Biochem Soc Trans 2021;49:2787-95. [Crossref] [PubMed]
- Zhang W, Qiu W. OTUB1 Recruits Tumor Infiltrating Lymphocytes and Is a Prognostic Marker in Digestive Cancers. Front Mol Biosci 2020;7:212. [Crossref] [PubMed]
- Song W, Zheng C, Liu M, et al. TRERNA1 upregulation mediated by HBx promotes sorafenib resistance and cell proliferation in HCC via targeting NRAS by sponging miR-22-3p. Mol Ther 2021;29:2601-16. [Crossref] [PubMed]
- Dietrich P, Gaza A, Wormser L, et al. Neuroblastoma RAS Viral Oncogene Homolog (NRAS) Is a Novel Prognostic Marker and Contributes to Sorafenib Resistance in Hepatocellular Carcinoma. Neoplasia 2019;21:257-68. [Crossref] [PubMed]
- Liu Y, Yang Y, Jiang L, et al. High Expression Levels of SLC38A1 Are Correlated with Poor Prognosis and Defective Immune Infiltration in Hepatocellular Carcinoma. J Oncol 2021;2021:5680968. [Crossref] [PubMed]
- Kondoh N, Imazeki N, Arai M, et al. Activation of a system A amino acid transporter, ATA1/SLC38A1, in human hepatocellular carcinoma and preneoplastic liver tissues. Int J Oncol 2007;31:81-7. [Crossref] [PubMed]
- Yu WL. Overexpression of ATA1/SLC38A1 predicts future recurrence and death in Chinese patients with hilar cholangiocarcinoma. J Surg Res 2011;171:663-8. [Crossref] [PubMed]
- Xie J, Li P, Gao HF, et al. Overexpression of SLC38A1 is associated with poorer prognosis in Chinese patients with gastric cancer. BMC Gastroenterol 2014;14:70. [Crossref] [PubMed]
- Yoo HC, Park SJ, Nam M, et al. A Variant of SLC1A5 Is a Mitochondrial Glutamine Transporter for Metabolic Reprogramming in Cancer Cells. Cell Metab 2020;31:267-283.e12. [Crossref] [PubMed]
- Gajewski TF, Schreiber H, Fu YX. Innate and adaptive immune cells in the tumor microenvironment. Nat Immunol 2013;14:1014-22. [Crossref] [PubMed]