Expressional and functional characteristics of checkpoint kinase 1 as a prognostic biomarker in hepatocellular carcinoma
Introduction
Hepatocellular carcinoma (HCC) is the most common pathological subtype of liver cancer and the third leading cause of cancer death worldwide (1,2). Current treatments of liver cancer mostly include surgical resection, chemotherapy, radiotherapy, radiofrequency ablation, interventional therapy, and liver transplantation. In addition, targeted drugs and immunotherapy programs are widely used in clinical practice (3). However, the 5-year survival is not satisfactory due to the high recurrence and metastasis in patients with advanced HCC (4). The development of HCC is extraordinarily complicated, containing multiple steps, such as dysregulation of oncogenic genes and metabolic alternations (5). Thus, it is urgent to identify the underlying mechanisms of HCC occurrence and development and explore specific biomarkers and therapeutic targets to improve patient survival.
Checkpoint kinase 1 (CHEK1) is an essential serine/threonine kinase which prevents impaired DNA from being copied and passed on to the offspring cells (6). Under genotoxic stress, CHEK1 blocks the cells in the S phase and G2/M phases of the cell cycle (7). There is increasing evidence that CHEK1 is an oncogene for tumorigenesis and its activity is essential for tumor cells to survive after radiotherapy and chemotherapy (8-10). According to a previous study, the up-regulation of CHEK1 in breast cancer appeared to be associated with adverse prognostic characteristics and clinicopathological category (11). Furthermore, the overexpression of CHEK1 was found in multiple human tumors, including nasopharyngeal, breast, and colon cancers (12-14).
At present, there are few studies of CHEK1 in HCC, and the molecular mechanisms of CHEK1 in HCC are still not fully understood. Bao et al. found that CHEK1 was negatively regulated by miR-126 in HCC (15). Guo et al. reported that the inhibition of ataxia telangiectasia and Rad3-related protein (ATR)-CHEK1 signaling led to the induction of DNA replication stress in liver cancer (16). However, these studies undertook only basic research of CHEK1 in HCC. There has been no comprehensive study undertaken to identify the prognostic significance, expression characteristics, and regulatory mechanisms of CHEK1 in hepatocellular cancer. In addition, there is a lack of analysis of gene-enriched cells (EC) with CHEK1 coexpression in HCC. Therefore, we analyzed CHEK1 expression in data downloaded from multiple public databases, and we performed a bioinformatics analysis to systematically evaluate the biological function and potential mechanism of CHEK1 in HCC. Our study found that CHEK1 might regulate DNA replication and cell cycle arrest through pathways related to E2F1 and several cancer-associated kinases. The experiments of cell function indicated that knockdown of CHEK1 suppressed the aggressive behavior and proliferation of HCC cells in vitro. Furthermore, we found that overexpression of E2F1 increased the proliferation and invasion of HCC cells in vitro. The rescue experiment showed that CHEK1 silence dampened cell invasion induced by E2F1 overexpression. For the first time, we present findings to suggest that E2F1 promotes the malignant phenotype of HCC cells by regulating CHEK1. We also identified the gene network coexpressed with CHEK1 in HCC through single-cell RNA sequencing and Multiscale Embedded Gene Co-expression Network Analysis (MEGENA). We present the following article in accordance with the REMARK reporting checklist (available at https://tcr.amegroups.com/article/view/10.21037/tcr-22-1701/rc).
Methods
Data sources
The Cancer Genome Atlas (TCGA) data for liver hepatocellular carcinoma (LIHC) patients were downloaded from the cBioportal (www.cbioportal.org). There were 369 HCC tissues and 160 normal tissues in TCGA. Four datasets, GSE112790, GSE55092, GSE9843, and GSE151530, were downloaded from the Gene Expression Omnibus (GEO) database, and CHEK1 messenger RNA (mRNA) expression in these datasets was calculated by R software (The R Foundation for Statistical Computing, Vienna, Austria). There were 183 HCC tissues and 15 normal tissues in GSE112790. There were 49 HCC tissues and 91 normal tissues in GSE55092.
Differential expression analysis
The web-based tool, Gene Expression Profiling Interactive Analysis (GEPIA; http://gepia.cancer-pku.cn/), was used to evaluate the CHEK1 mRNA level in multiple cancers and normal paired tissues from TCGA database. Differentially expressed genes (DEGs) in the CHEK1-low group and the CHEK1-high group were identified by the “Limma” (v. 3.50.0) R package. A |fold change| >2 and P<0.01 were defined as the thresholds.
Survival analysis and significant enrichment analysis
The “survminer” (v. 0.4.9) R package and “survival” (v. 3.2-13) R package were used to compare the survival outcome between the CHEK1-low group and CHEK1-high group. To enrich the Kyoto Encyclopedia of Genes and Genomes (KEGG) pathways and analyze the Gene Ontology (GO) functions of CHEK1 DEGs, the “clusterProfiler” (v. 4.2.2) R package was employed. A false discovery rate (FDR) below 0.05 was defined as significant enrichment. The Kaplan-Meier plotter (www.kmplot.com) was used to generate the survival curve of CHEK1 and hsa-miR-195 levels in LIHC patients.
CHEK1 promoter methylation and prediction of upstream regulatory microRNAs (miRNAs)
The cBioportal was used to identify the correlation between CHEK1 expression level and CHEK1 promoter methylation in TCGA-LIHC (17). The miRwalk (www.mirwalk.umm.ini-heidelberg.de), miRDB (www.mirdb.org), and TargetScan (www.targetscan.org) databases were used to explore the potential regulatory miRNAs of CHEK1 (18-20). The University of Alabama at Birmingham Cancer (UALCAN) data analysis portal (http://ualcan.path.uab.edu/index.html) was used to analyze the hsa-miR-195 and CHEK1 methylation levels in LIHC patients and assess their effect on prognosis (21).
Coexpression network analysis
Networks of CHEK1 coexpressed genes were analyzed using the established MEGENA (22,23). Briefly, all gene pairs were analyzed by Spearman correlation coefficients (SCCs). An FDR below 0.05 was considered statistically significant. The planar maximally filtered graph (PMFG) algorithm (24) was then used to iteratively test ranked significant SCCs and construct a planar filtered network (PFN). Multiscale cluster analysis (MCA) was used to analyze the PFN and identify coexpressed modules at different scales.
Processing of single-cell RNA sequencing data
Seurat (v. 3.2.2) (25) was utilized to process the unique molecular identifier (UMI) count matrix (GSE151530) downloaded from the GEO database, and the annotation of major cell types was referred to in the original text (26) without modification. Malignant cells were extracted for further re-clustering and analysis. The batch effect was removed using Harmony (v. 18 0.1.0) R package (27) according to sample identification. Seurat clusters of malignant cells were identified using the “FindClusters” function within the default parameter.
Definition of cell scores
We selected the “AddModuleScore” function in Seurat to define the average expression level of CHEK1 coexpressed genes as the module score for malignant cells. To grade the expression level of functional gene sets defined in the HALLMARK database, the standard setting in the Gene Set Variation Analysis (GSVA; V1.36.0) software package for R (28) was applied.
All the R package and analytic methods cited above were operated by Statistical Computing 2020 (v. 4.0.3) for R foundation.
Cell lines
Six hepatoma cell lines (Hep3B, MHCC97L, MHCC97H, PLC/PRF/5, Huh7, and HCCLM3) and a normal liver cell line (L02) were acquired from the Liver Cancer Institute of Zhongshan Hospital (Shanghai, China). All cells were cultivated in Dulbecco’s Modified Eagle Medium (DMEM; Gibco, Thermo Fisher Scientific, Waltham, MA, USA) mixed with 10% heat-inactivated fetal bovine serum (FBS; Gibco) at 5% CO2 and 37 ℃.
Western blotting
Western blot was conducted as previously described (29), and the primary antibodies were as follows: glyceraldehyde 3-phosphate dehydrogenase (GAPDH; 1:10,000, #AC002, Abclonal, Wuhan, China), E2F1 (1:1,000, #A19579, Abclonal). CHEK1 (1:1,000, #A7653, Abclonal). Blots were visualized using enhanced chemiluminescence (ECL) reagent (Bio-Rad, Hercules, CA, USA). The density of the blots was analyzed by Image J software v. 1.8.0 (US National Institutes of Health, Bethesda, MD, USA).
Cell transfection
Three short interfering RNAs (siRNAs) targeting CHEK1 were synthesized by Genepharma (Shanghai, China). Their sequence information were as follows: siCHEK1-1, 5'-GCAGUGAAGAUUGUAGAUATT-3'; siCHEK1-2, 5'-GGUUUAUCUGCAUGGUAUUTT-3'; and siCHEK1-3, 5'-GGCAACAGUAUUUCGGUAUTT-3'. E2F1 overexpression plasmid was purchased from Genechem (Shanghai, China). Transfection was conducted using Lip2000 transfection reagent (Invitrogen, Thermo Fisher Scientific) as recommended. The knockdown and overexpression efficiency in the protein level was validated through Western blotting.
Cell function assays
The cell viability was tested using Cell Counting Kit 8 (CCK-8; Beyotime, Shanghai, China) as described (29). The absorbance of each well was detected by a microplate reader at 450 nm. The flow cytometry was performed to analyze the cell cycle as described (29). The Transwell invasion and migration assay was undertaken in Transwell insert chambers (8.0 µm; BD Biosciences, San Jose, CA, USA) precoated with or without 100 µL of Matrigel. Cells were planted in a chamber at the density of 1×105 cells per insert, and 4% paraformaldehyde was used to fix the cells, after which crystal violet was used to stain them. The images were captured by a light microscope (Nikon, Tokyo, Japan) in 6 random fields.
Statistical analysis
All the cell experimental data were replicated 3 times independently (n=3). Results are displayed as mean ± standard deviation (SD). One-way analysis of variance (ANOVA) and Student t-test were used to compare the differences among groups. The Wilcoxon test was used to compare the differential expression of CHEK1 between the tumor tissues and normal paired tissues in TCGA. The log-rank chi-square test was used to analyze the difference in survival outcomes between CHEK1-high and CHEK1-low patients. The statistical tests were performed using R software (The R Foundation for Statistical Computing) or GraphPad Prism v. 6.0 (GraphPad Software). A P value below 0.05 was defined as statistically significant.
Ethical statement
The study was conducted in accordance with the Declaration of Helsinki (as revised in 2013). Institutional ethical approval and informed consent were waived.
Results
CHEK1 was upregulated in HCC and was associated with poor prognosis
We first used GEPIA to screen DEGs that have a significant influence on relapse-free survival (RFS) and overall survival (OS) time (Figure 1A) in HCC samples from TCGA database. Four genes were overlapped in 3 algorithms, and CHEK1 was selected as a potential prognostic biomarker (Table 1). Subsequently, we analyzed CHEK1 mRNA levels in different cancers using the GEPIA database. In the GEPIA, CHEK1 mRNA levels were dramatically overexpressed in 20 of 33 cancer tissues compared with their normal counterparts, including LIHC (Figure 1B,1C). Next, the CHEK1 expression was validated using 2 HCC cohorts from the GEO database. Analysis from 2 different cohorts (GSE112790 and GSE55092) also showed upregulation of CHEK1 in HCC tissues (Figure 1D,1E). To identify the relationship between clinico-pathological characteristics and CHEK1 expression in HCC, we performed a subgroup analysis of multiple clinicopathological features of the TCGA-LIHC samples using UALCAN. The results consistently showed significantly elevated CHEK1 mRNA levels in patients with HCC compared to normal controls in subgroup analyses according to pathological type, lymph node metastasis status, disease stage, and tumor grade (Figure 2A-2D). We then plotted the Kaplan-Meier survival curve to investigate the correlation between CHEK1 level and survival outcome in HCC patients. Based on the median value of CHEK1 mRNA levels, 371 patients from the TCGA-LIHC cohort were separated into 2 groups. The results showed that the progression-free survival (PFS) and OS in CHEK1-high patients were remarkably shorter than that in CHEK1-low patients (Figure 2E,2F). In summary, our results indicated that CHEK1 expression was upregulated in HCC and was associated with survival outcomes.
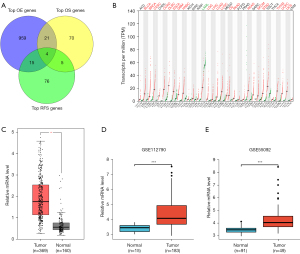
Table 1
Names | Number | Elements |
---|---|---|
Most OE genes | 4 | CHEK1; KIF2C; PTTG1; UBE2S |
Top OS genes | ||
Top RFS genes | ||
Most OE genes | 21 | NUP37; KIFC1; SAC3D1; FAM189B; CCT4; TMEM106C; CDC20; |
Top OS genes | DTYMK; HM13; SRD5A3; CCT3; SNRPEP2; SLC41A3; DKN2C; | |
PPM1G; PIGU; ATP1B3; TMEM147; UQCRH; GARS; UCK2 | ||
Most OE genes | 15 | CCNB1; NRM; KPNA2; CENPH; CDC25C; MCM3; PHF19; DDAH2; |
Top RFS genes | RAD51C; ZWINT; STMN1; LMNB1; RPL39P3; MKI67; MCM6 | |
Top OS genes | 5 | GPSM2; DNASE1L3; CLEC3B; CELSR3; XPO5 |
Top RFS genes |
DEGs, differentially expressed genes; HCC, hepatocellular carcinoma; GEPIA, Gene Expression Profiling Interactive Analysis; OE, overexpressed; OS, overall survival; RFS, relapse-free survival.
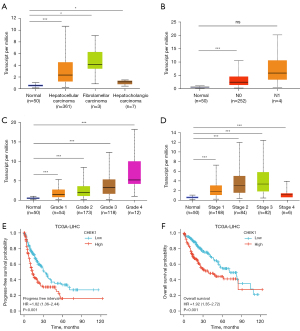
Epigenetic regulation of CHEK1 in HCC
Subsequently, we wanted to explore the potential regulatory mechanism of CHEK1 overexpression in LIHC at the epigenetic level. A recent study has shown that abnormal DNA methylation in cancers leads to silence of tumor-suppressor gene and induces tumor progression (30). The correlation analysis by the online website cBioportal showed a weak negative correlation between CHEK1 expression and CHEK1 methylation (Figure 3A; Spearman’s r=−0.14, P=9.9229e-3; Pearson’s r=−0.13, P=0.0131). We further evaluated the methylation level of the CHEK1 promoter in TCGA-LIHC using UALCAN and found that it was lower in HCC tissues than in normal liver tissues (Figure 3B). These results suggested that promoter methylation may not be the main factor regulating CHEK1 overexpression.
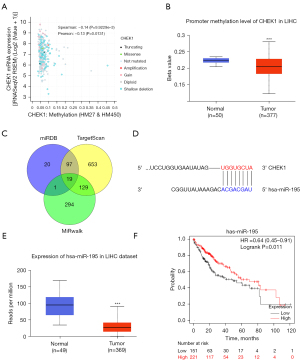
We then screened regulatory miRNAs binding to CHEK1 3 prime untranslated region (3' UTR) in LIHC using the miRWalk, TargetScan, and miRDB databases. Nineteen miRNAs were selected as candidates for potential regulatory miRNAs (Figure 3C; Table S1). After combination with the expression analysis of the UALCAN database, hsa-miR-195 was identified as a CHEK1 promoter binding miRNA (Figure 3D). The result showed that hsa-miR-195 was dramatically downregulated in HCC tissues compared to normal paired tissues (Figure 3E). The Kaplan-Meier (KM) analysis revealed that a lower expression of hsa-miR-195 was associated with poorer OS in LIHC (Figure 3F; P=0.011). These results indicated that hsa-miR-195 may contribute to the upregulation of CHEK1 in LIHC.
Identification of CHEK1 biological function in HCC
To clarify the biological meaning of CHEK1 in the progression of HCC, we applied “Limma” to identify DEGs of CHEK1-low and CHEK1-high groups in the TCGA-LIHC cohort. A total of 132 down-regulation genes and 342 up-regulation genes were screened in the CHEK1-high group, as shown in the volcano plot (Figure 4A). The heatmap plotted by “Pheatmap” (v. 1.0.12) in R package indicated the 20 most significant DEGs that were downregulated and upregulated, respectively (Figure 4B). Annotation of the GO term revealed that CHEK1 DEGs involving chromosome segregation and organelle fission were significantly upregulated, and the KEGG pathway analysis displayed significant enrichment in the cell cycle pathway (Figure 4C,4D). We then used NetworkAnalyst to construct a protein-protein interaction (PPI) network of DEGs, and the result showed that molecules associated with the DNA replication process were significantly enriched (Figure 5A). These findings reflect that CHEK1 may play a key role in HCC cell proliferation.
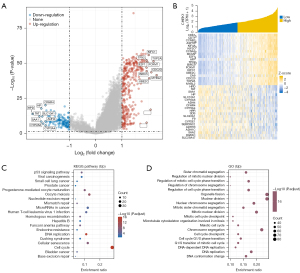
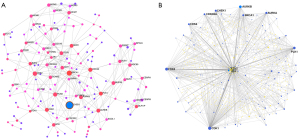
Regulators of CHEK1 networks in HCC
Next, we constructed the transcriptional factor (TF) and kinase networks for these DEGs using NetworkAnalyst to identify the regulators of CHEK1 in HCC. The 5 most prominent kinase networks were mainly associated with Aurora kinase A (AURKA), Aurora kinase B (AURKB), pololike kinase 1 (PLK1), cyclin-dependent kinase 1 (CDK1), and cyclin-dependent kinase 2 (CDK2) (Figure 5B). The TF enrichment was primarily associated with the E2F TF family (Table S2). These results suggested that CHEK1 may regulate cell cycle and DNA replication pathways through the E2F TF family and interacted kinases.
Identification of CHEK1 coexpressed genes in HCC
To identify the CHEK1 coexpressed genes in HCC, we constructed a PFN network via MEGENA of HCC tissues using the transcriptome data in the GSE9843 cohort and then performed MCA to identify coexpressed modules (Figure 6A). The smallest coexpressed subnetwork containing CHEK1 was identified, and these genes were set as a CHEK1-related gene module (Figure 6B). We further performed clustering analysis to define the single-cell profile of CHEK1-related genes in HCC. All cells were integrated according to the sample ID using Harmony v. 18 0.1.0 (Figure 6C), and 21 Seurat clusters were identified (Figure 6D). We then used the “AddModuleScore” function in Seurat to calculate the average expression level of CHEK1-related genes in malignant cells, and the visualization result revealed that these genes were enriched in clusters 4 and 10 (Figure 6E). We defined clusters 4 and 10 as EC and defined other clusters as nonenriched cells (NEC). Cellular (Cyto) Trajectory Reconstruction Analysis using gene Counts and Expression (CytoTRACE) is a gene-counting algorithm that significantly improves the detection of cell differentiation at the single-cell level (31). The CytoTRACE analysis found that EC were placed further forward in ordering than in NEC (Figure 6F), suggesting that EC had the potential to differentiate and were more dangerous tumor cells.

TF activity and expression level of EC
Based on the above result that CHEK1 may be regulated by the transcription factor E2F family, we wanted to determine whether the transcription factor was activated in gene-EC with CHEK1 coexpression. DoRothEA is a web tool containing a collection of gene sets with TF targets that can be used to infer TF activity (32). We combined the Visualization Pipeline for RNA-seq analysis (VIPER) (33) with DoRothEA to analyze the TF activity on enriched and NEC from GSE151530. It was found that the E2F family, TFDP1 and FOXM1 were highly activated in EC. (Figure S1). Further examination of these TF levels showed that E2F1 and FOXM1 expression levels were upregulated in the EC, while the expression of other TFs exhibited no significant change (Figure 6G). We applied GSVA to identify several CHEK1-related hallmarks. The results revealed that the expression of hallmarks related to DNA repair, G2/M checkpoint and E2F target were increased in EC (Figure 6H). These results suggested that overexpression of CHEK1 may be partly attributable to the increased expression level and transcriptional activity of E2F1.
Knockdown of CHEK1 inhibited proliferation, invasion, and migration of HCC cells in vitro
We performed Western blotting to detect the CHEK1 protein level in a normal liver (L02) cell line and 6 hepatoma cell lines. The result showed significant upregulation of CHEK1 in the hepatoma cell lines compared with the L02 cell line. The PLC/PRF/5 and HCCLM3 cell lines had the highest CHEK1 expression levels (Figure S2). To determine the biological role of CHEK1 in HCC cells in vitro, we knocked down CHEK1 by transfecting the PLC/PRF/5 and HCCLM3 cells with short interfering RNA (siRNA). The Western blot assay was conducted to validate the knockdown efficiency (Figure 7A). We discovered that siRNA-3 resulted in knockdown of CHEK1 with the best efficiency, and we used this sequence in the following functional experiments. Subsequently, the effect of CHEK1 knockdown on cell viability was detected using CCK-8. CHEK1 knockdown led to a significant decline of HCC cell proliferation in the HCCLM3 and PLC/PRF/5 cell lines (Figure 7B). Furthermore, the flow cytometry assay showed that knockdown of CHEK1 increased the proportion of G2 phase cells in both the PLC/PRF/5 and HCCLM3 cell lines (Figure S3A,S3B). Finally, the impacts of CHEK1 knockdown on cell migration and invasion were examined. The mobility of HCCLM3 and PLC/PRF/5 cells in the Transwell migration assay was significantly decreased after the knockdown of CHEK1 (Figure 7C). Similarly, in Matrigel invasion assays, CHEK1 knockdown significantly inhibited the invasion of HCCLM3 and PLC/PRF/5 cells (Figure 7D). Our results indicated that CHEK1 knockdown could suppress the aggressive behavior of HCC cells in vitro.
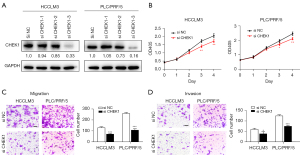
E2F1 promoted HCC invasion by regulating CHEK1 expression
As documented above, we found that E2F1 may regulate the expression of CHEK1 (Figure 5B; Table S2). To verify this, we performed further experiments. First, we overexpressed E2F1 by transfecting PLC/PRF/5 and HCCLM3 cells with plasmid. The results showed that overexpression of E2F1 upregulated the CHEK1 expression level (Figure 8A). Subsequently, the effect of E2F1 on cell viability was detected using CCK-8. E2F1 overexpression led to a significant increase of HCC cell proliferation in HCCLM3 and PLC/PRF/5 cells (Figure 8B). We then examined the effects of E2F1 on cell invasion. The result showed that E2F1 overexpression significantly enhanced the invasion of HCCLM3 and PLC/PRF/5 cells (Figure 8C). To investigate whether E2F1 promotes HCC invasion via CHEK1 induction, we performed knockdown of CHEK1 in E2F1 overexpression cells. The knockdown of CHEK1 was verified at the protein level (Figure 8D). Interestingly, CHEK1 silence dampened cell invasion due to E2F1 overexpression in HCCLM3 and PLC/PRF/5 cells, as measured by invasion assays (Figure 8E,8F). These results suggested that CHEK1 is essential for the E2F1 promotion of HCC cell invasion.
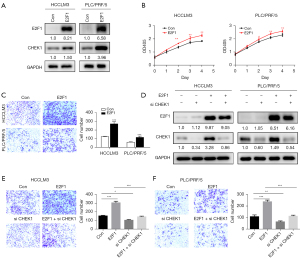
Discussion
Early diagnosis of liver cancer is crucial for clinicians to carry out timely treatment and improve patient survival. Alpha-fetoprotein (AFP) has been applied as a marker in the early detection of HCC for a long time. However, only about 70% of HCC patients have positive AFP results (34). Thus, there is an urgent need to find new indicators and improve the early diagnosis of HCC. In this study, we performed a systematic bioinformatic analysis of CHEK1 in HCC data from multiple public databases. The effects of CHEK1 on the biological function of HCC cells were verified through in vitro cell experiments. We assessed CHEK1 mRNA levels in the GEPIA database and found that CHEK1 was dramatically upregulated in HCC tissues compared with normal paired tissues, which was validated via 2 GEO cohorts (GSE112790 and GSE55092). We observed that upregulation of CHEK1 was significantly associated with lower PFS and OS. These results suggested that the detection of CHEK1 as a biomarker in the tissues of patients with HCC can be used to predict patient prognosis. More clinical specimens would need to be collected to confirm our conclusion.
We further explored the underlying regulatory mechanism of CHEK1 overexpression in HCC at the epigenetic level. miRNAs have been reported to play an essential role in the development of liver cancer by regulating the expression levels of downstream target genes in cells through multiple processes (35). miRNA-122 is the most abundant miRNA in the liver and plays a critical role in multiple biological processes, including metabolism, homeostasis, and liver development (36). Low expression of miRNA-122 in HCC has been reported to be associated with carcinogenesis and adverse clinical outcomes (37). Liu et al. found that overexpression of miRNA-122 can inhibit HCC cell proliferation and increase the sensitivity of HCC to antitumor drugs (38). miRNA-122-mediated tumor suppression involves several signaling pathways, including Wnt family member 1 (WNT1), pyruvate kinase isoform M2 (PKM2), and cyclin G1 (38-40).
In our study, we selected hsa-miR-195 as a potential regulatory miRNA for CHEK1, and the downregulation of hsa-miR-195 was associated with adverse clinical outcomes in HCC. The putative binding between hsa-miR-195 and the CHEK1 3' UTR site further indicated that hsa-miR-195 might be the potential regulator of CHEK1 in HCC. Wang et al. reported that downregulation of hsa-miR-195 was associated with portal vein thrombosis, tumor size, patient survival, and TNM stage. In addition, hsa-miR-195 promotes lung metastasis of liver cancer by inhibiting vascular endothelial growth factor A (VEGFA) and fibroblast growth factor 2 (FGF2) (41). Gao et al. discovered that hsa-miR-195 was the upstream regulator of cyclin E1 (CCNE1), and its downregulation was associated with cisplatin resistance in HCC cells (42). In a future study using molecular experiments, we will verify whether hsa-miR-195 regulates CHEK expression.
To evaluate the biological role of CHEK1 and explore the signaling changes during aberrant CHEK1 expression, the networks of DEGs were analyzed. GSEA indicated that CHEK1 DEGs participated mainly in cell division and cell cycle processes. Further analysis of the liver-specific PPI network showed that CHEK1 critically regulated DNA replication and the cell cycle pathway in HCC. Concerning screening potential regulators which account for CHEK1 upregulation, the E2F family is regarded as the major TF in CHEK1 dysregulation. E2F1, which has traditionally been considered an essential regulator of the cell cycle, works by regulating cell cycle components, such as CCNE1, CDC25a, and CDC2 (43). A recent study showed that E2F1 could promote tumor cell metastasis and chemotherapeutic drug resistance (44). However, E2F1 also shows tumor inhibition by inducing cell apoptosis and senescence. Thus, a fragile equilibrium prevails, and E2F1 may play opposite roles in different tumors (45). In liver cancer progression, E2F oncogenic signaling was observed to be consistently activated (46). It was reported that CHEK1 is vital for E2F1 stabilization and activity under genotoxic stress (47). Our findings indicate that E2F1 may be a vital regulator of CHEK1, by which CHEK1 regulates HCC cell proliferation. In luciferase experiments, Bargiela-Iparraguirre et al. found that CHEK1 was regulated by RB/E2F1 and p53 at the transcriptional level in gastric cancer (48). These findings indicate that there is a complex regulatory loop between CHEK1 and E2F1. More research is required to explore the deep regulatory mechanisms of CHEK1 and E2F1. Bao et al. reported that miR-126 negatively regulated PLK4 to impact the development of HCC via the ATR/CHEK1 pathway (15). Our regulatory network analysis was performed by NetworkAnalyst, and the result showed that PLK1 correlated with CHEK1. Since both PLK1 and PLK4 belong to the pololike kinase family members, we hypothesized that PLK1 is also involved in the molecular mechanism of CHEK1 in HCC. We performed several functional experiments on HCCLM3 and PLC/PRF/5 cells and demonstrated that CHEK1 knockdown inhibited cell viability, invasion, and migration of HCC cells in vitro. Using a colony formation assay, Guo et al. demonstrated that the CHEK1 inhibitor, MK-8776, significantly suppresses the proliferation of HCC cells (16), which is consistent with our findings using the CCK-8 assay.
It has been reported that the knockdown or inhibition of CHEK1 increases the sensitivity of tumor cells to multiple types of chemotherapy agents containing cisplatin, 5-fluorouracil, gemcitabine, and doxorubicin (49). MK-8776 is an efficient and selective CHEK1 inhibitor that has been found to abolish S-phase arrest and apoptosis induced by cytarabine in acute myelogenous leukemia cells (50). A comparative study of 2 CHEK1 inhibitors with similar structure showed that GNE-783 could effectively increase the activity of antimetabolites, whereas GNE-900 preferentially sensitized cells to gemcitabine (51). Although preclinical studies of the impact of CHEK1 inhibitors on chemosensitization are impressive, the results of clinical trials are less promising (52,53), and various adverse reactions limit the clinical application of CHEK1 inhibitors (54,55). A new generation of CHEK1 inhibitors with high efficiency, low toxicity, and high tolerance needs to be developed in the future. There is reason to believe that CHEK1 inhibitors are promising as a single agent or in combination with chemotherapy to improve the therapeutic efficacy of patients with liver cancer.
However, our study had some limitations. First, the generalization of the conclusion is limited by the sample size, and larger samples will be collected and analyzed in the future. Second, because of the difficulty in obtaining patient samples, the conclusions of this study have not been validated in clinical samples. In a future study, we will submit ethical applications to support the collection of clinical samples for analysis and validation. In addition, further detailed experiments are required to clarify the underlying regulatory relationships between E2F1, hsa-miR-195, and CHEK1.
Conclusions
In this study, we have provided evidence at multiple levels to support the essential role of CHEK1 in carcinogenesis and the potential of CHEK1 as a prognostic biomarker in HCC. Our findings indicate that the overexpression of CHEK1 in HCC might have profound effects on mitosis and genome stability, as well as on multiple processes of the cell cycle. These findings need to be corroborated by further studies, including large-scale genome research of HCC.
Acknowledgments
Funding: This work was supported by
Footnote
Reporting Checklist: The authors have completed the REMARK reporting checklist Available at https://tcr.amegroups.com/article/view/10.21037/tcr-22-1701/rc
Conflicts of Interest: All authors have completed the ICMJE uniform disclosure form (available at https://tcr.amegroups.com/article/view/10.21037/tcr-22-1701/coif). The authors have no conflicts of interest to declare except the funding.
Ethical Statement: The authors are accountable for all aspects of the work in ensuring that questions related to the accuracy or integrity of any part of the work are appropriately investigated and resolved. The study was conducted in accordance with the Declaration of Helsinki (as revised in 2013). Institutional ethical approval and informed consent were waived.
Open Access Statement: This is an Open Access article distributed in accordance with the Creative Commons Attribution-NonCommercial-NoDerivs 4.0 International License (CC BY-NC-ND 4.0), which permits the non-commercial replication and distribution of the article with the strict proviso that no changes or edits are made and the original work is properly cited (including links to both the formal publication through the relevant DOI and the license). See: https://creativecommons.org/licenses/by-nc-nd/4.0/.
References
- Siegel RL, Miller KD, Fuchs HE, et al. Cancer Statistics, 2021. CA Cancer J Clin 2021;71:7-33. [Crossref] [PubMed]
- Perz JF, Armstrong GL, Farrington LA, et al. The contributions of hepatitis B virus and hepatitis C virus infections to cirrhosis and primary liver cancer worldwide. J Hepatol 2006;45:529-38. [Crossref] [PubMed]
- Zucman-Rossi J, Villanueva A, Nault JC, et al. Genetic Landscape and Biomarkers of Hepatocellular Carcinoma. Gastroenterology 2015;149:1226-39.e4. [Crossref] [PubMed]
- Louafi S, Boige V, Ducreux M, et al. Gemcitabine plus oxaliplatin (GEMOX) in patients with advanced hepatocellular carcinoma (HCC): results of a phase II study. Cancer 2007;109:1384-90. [Crossref] [PubMed]
- Levrero M, Zucman-Rossi J. Mechanisms of HBV-induced hepatocellular carcinoma. J Hepatol 2016;64:S84-101. [Crossref] [PubMed]
- Zhang Y, Hunter T. Roles of Chk1 in cell biology and cancer therapy. Int J Cancer 2014;134:1013-23. [Crossref] [PubMed]
- Smith J, Tho LM, Xu N, et al. The ATM-Chk2 and ATR-Chk1 pathways in DNA damage signaling and cancer. Adv Cancer Res 2010;108:73-112. [Crossref] [PubMed]
- Tho LM, Libertini S, Rampling R, et al. Chk1 is essential for chemical carcinogen-induced mouse skin tumorigenesis. Oncogene 2012;31:1366-75. [Crossref] [PubMed]
- Lee JH, Choy ML, Ngo L, et al. Role of checkpoint kinase 1 (Chk1) in the mechanisms of resistance to histone deacetylase inhibitors. Proc Natl Acad Sci U S A 2011;108:19629-34. [Crossref] [PubMed]
- Bao S, Wu Q, McLendon RE, et al. Glioma stem cells promote radioresistance by preferential activation of the DNA damage response. Nature 2006;444:756-60. [Crossref] [PubMed]
- Al-Kaabi MM, Alshareeda AT, Jerjees DA, et al. Checkpoint kinase1 (CHK1) is an important biomarker in breast cancer having a role in chemotherapy response. Br J Cancer 2015;112:901-11. [Crossref] [PubMed]
- Madoz-Gúrpide J, Cañamero M, Sanchez L, et al. A proteomics analysis of cell signaling alterations in colorectal cancer. Mol Cell Proteomics 2007;6:2150-64. [Crossref] [PubMed]
- Verlinden L, Vanden Bempt I, Eelen G, et al. The E2F-regulated gene Chk1 is highly expressed in triple-negative estrogen receptor /progesterone receptor /HER-2 breast carcinomas. Cancer Res 2007;67:6574-81. [Crossref] [PubMed]
- Sriuranpong V, Mutirangura A, Gillespie JW, et al. Global gene expression profile of nasopharyngeal carcinoma by laser capture microdissection and complementary DNA microarrays. Clin Cancer Res 2004;10:4944-58. [Crossref] [PubMed]
- Bao J, Yu Y, Chen J, et al. MiR-126 negatively regulates PLK-4 to impact the development of hepatocellular carcinoma via ATR/CHEK1 pathway. Cell Death Dis 2018;9:1045. [Crossref] [PubMed]
- Guo Y, Wang J, Benedict B, et al. Targeting CDC7 potentiates ATR-CHK1 signaling inhibition through induction of DNA replication stress in liver cancer. Genome Med 2021;13:166. [Crossref] [PubMed]
- Cerami E, Gao J, Dogrusoz U, et al. The cBio cancer genomics portal: an open platform for exploring multidimensional cancer genomics data. Cancer Discov 2012;2:401-4. [Crossref] [PubMed]
- Agarwal V, Bell GW, Nam JW, et al. Predicting effective microRNA target sites in mammalian mRNAs. Elife 2015;4:e05005. [Crossref] [PubMed]
- Chen Y, Wang X. miRDB: an online database for prediction of functional microRNA targets. Nucleic Acids Res 2020;48:D127-31. [Crossref] [PubMed]
- Sticht C, De La Torre C, Parveen A, et al. miRWalk: An online resource for prediction of microRNA binding sites. PLoS One 2018;13:e0206239. [Crossref] [PubMed]
- Chandrashekar DS, Bashel B, Balasubramanya SAH, et al. UALCAN: A Portal for Facilitating Tumor Subgroup Gene Expression and Survival Analyses. Neoplasia 2017;19:649-58. [Crossref] [PubMed]
- Song WM, Agrawal P, Von Itter R, et al. Network models of primary melanoma microenvironments identify key melanoma regulators underlying prognosis. Nat Commun 2021;12:1214. [Crossref] [PubMed]
- Wang Q, Zhang Y, Wang M, et al. The landscape of multiscale transcriptomic networks and ’ey regulators in Parkinson's disease. Nat Commun 2019;10:5234. [Crossref] [PubMed]
- Tumminello M, Aste T, Di Matteo T, et al. A tool for filtering information in complex systems. Proc Natl Acad Sci U S A 2005;102:10421-6. [Crossref] [PubMed]
- Stuart T, Butler A, Hoffman P, et al. Comprehensive Integration of Single-Cell Data. Cell 2019;177:1888-902.e21. [Crossref] [PubMed]
- Sun Y, Wu L, Zhong Y, et al. Single-cell landscape of the ecosystem in early-relapse hepatocellular carcinoma. Cell 2021;184:404-21.e16. [Crossref] [PubMed]
- Korsunsky I, Millard N, Fan J, et al. Fast, sensitive and accurate integration of single-cell data with Harmony. Nat Methods 2019;16:1289-96. [Crossref] [PubMed]
- Hänzelmann S, Castelo R, Guinney J. GSVA: gene set variation analysis for microarray and RNA-seq data. BMC Bioinformatics 2013;14:7. [Crossref] [PubMed]
- Tang W, Zhou Y, Sun D, et al. Oncogenic role of phospholipase C-γ1 in progression of hepatocellular carcinoma. Hepatol Res 2019;49:559-69. Erratum in: Hepatol Res 2019;49:1088. [Crossref] [PubMed]
- Ehrlich M. DNA hypermethylation in disease: mechanisms and clinical relevance. Epigenetics 2019;14:1141-63. [Crossref] [PubMed]
- Gulati GS, Sikandar SS, Wesche DJ, et al. Single-cell transcriptional diversity is a hallmark of developmental potential. Science 2020;367:405-11. [Crossref] [PubMed]
- Garcia-Alonso L, Holland CH, Ibrahim MM, et al. Benchmark and integration of resources for the estimation of human transcription factor activities. Genome Res 2019;29:1363-75. [Crossref] [PubMed]
- Alvarez MJ, Shen Y, Giorgi FM, et al. Functional characterization of somatic mutations in cancer using network-based inference of protein activity. Nat Genet 2016;48:838-47. [Crossref] [PubMed]
- Luo P, Yin P, Hua R, et al. A Large-scale, multicenter serum metabolite biomarker identification study for the early detection of hepatocellular carcinoma. Hepatology 2018;67:662-75. [Crossref] [PubMed]
- Mohr R, Özdirik B, Lambrecht J, et al. From Liver Cirrhosis to Cancer: The Role of Micro-RNAs in Hepatocarcinogenesis. Int J Mol Sci 2021;22:1492. [Crossref] [PubMed]
- Xu H, He JH, Xiao ZD, et al. Liver-enriched transcription factors regulate microRNA-122 that targets CUTL1 during liver development. Hepatology 2010;52:1431-42. [Crossref] [PubMed]
- Szabo G, Bala S. MicroRNAs in liver disease. Nat Rev Gastroenterol Hepatol 2013;10:542-52. [Crossref] [PubMed]
- Liu AM, Xu Z, Shek FH, et al. miR-122 targets pyruvate kinase M2 and affects metabolism of hepatocellular carcinoma. PLoS One 2014;9:e86872. [Crossref] [PubMed]
- Ahsani Z, Mohammadi-Yeganeh S, Kia V, et al. WNT1 Gene from WNT Signaling Pathway Is a Direct Target of miR-122 in Hepatocellular Carcinoma. Appl Biochem Biotechnol 2017;181:884-97. [Crossref] [PubMed]
- Gramantieri L, Ferracin M, Fornari F, et al. Cyclin G1 is a target of miR-122a, a microRNA frequently down-regulated in human hepatocellular carcinoma. Cancer Res 2007;67:6092-9. [Crossref] [PubMed]
- Wang M, Zhang J, Tong L, et al. MiR-195 is a key negative regulator of hepatocellular carcinoma metastasis by targeting FGF2 and VEGFA. Int J Clin Exp Pathol 2015;8:14110-20. [PubMed]
- Gao L, Zhang X. Propofol enhances the lethality of cisplatin on liver cancer cells by up-regulating miR-195-5p. Tissue Cell 2022;74:101680. [Crossref] [PubMed]
- Chen HZ, Tsai SY, Leone G. Emerging roles of E2Fs in cancer: an exit from cell cycle control. Nat ’ev Cancer 2009;9:785-97. [PubMed]
- O'Connor DJ, Lu X. Stress signals induce transcriptionally inactive E2F-1 independently of p53 and Rb. Oncogene 2000;19:2369-76. [Crossref] [PubMed]
- Pützer BM, Engelmann D. E2F1 apoptosis counterattacked: evil strikes back. Trends Mol Med 2013;19:89-98. [Crossref] [PubMed]
- Zhan L, Huang C, Meng XM, et al. Promising roles of mammalian E2Fs in hepatocellular carcinoma. Cell Signal 2014;26:1075-81. [Crossref] [PubMed]
- Urist M, Tanaka T, Poyurovsky MV, et al. p73 induction after DNA damage is regulated by checkpoint kinases Chk1 and Chk2. Genes Dev 2004;18:3041-54. [Crossref] [PubMed]
- Bargiela-Iparraguirre J, Prado-Marchal L, Fernandez-Fuente M, et al. CHK1 expression in Gastric Cancer is modulated by p53 and RB1/E2F1: implications in chemo/radiotherapy response. Sci Rep 2016;6:21519. [Crossref] [PubMed]
- Rundle S, Bradbury A, Drew Y, et al. Targeting the ATR-CHK1 Axis in Cancer Therapy. Cancers (Basel) 2017;9:41. [Crossref] [PubMed]
- Schenk EL, Koh BD, Flatten KS, et al. Effects of selective checkpoint kinase 1 inhibition on cytarabine cytotoxicity in acute myelogenous leukemia cells in vitro. Clin Cancer Res 2012;18:5364-73. [Crossref] [PubMed]
- Xiao Y, Ramiscal J, Kowanetz K, et al. Identification of preferred chemotherapeutics for combining with a CHK1 inhibitor. Mol Cancer Ther 2013;12:2285-95. [Crossref] [PubMed]
- Krug LM, Wozniak AJ, Kindler HL, et al. Randomized phase II trial of pemetrexed/cisplatin with or without CBP501 in patients with advanced malignant pleural mesothelioma. Lung Cancer 2014;85:429-34. [Crossref] [PubMed]
- Scagliotti G, Kang JH, Smith D, et al. Phase II evaluation of LY2603618, a first-generation CHK1 inhibitor, in combination with pemetrexed in patients with advanced or metastatic non-small cell lung cancer. Invest New Drugs 2016;34:625-35. [Crossref] [PubMed]
- Sausville E, Lorusso P, Carducci M, et al. Phase I dose-escalation study of AZD7762, a checkpoint kinase inhibitor, in combination with gemcitabine in US patients with advanced solid tumors. Cancer Chemother Pharmacol 2014;73:539-49. [Crossref] [PubMed]
- Seto T, Esaki T, Hirai F, et al. Phase I, dose-escalation study of AZD7762 alone and in combination with gemcitabine in Japanese patients with advanced solid tumours. Cancer Chemother Pharmacol 2013;72:619-27. [Crossref] [PubMed]