A narrative review on machine learning in diagnosis and prognosis prediction for tongue squamous cell carcinoma
Introduction
Oral cavity squamous cell carcinoma (OCSCC) is one of the most common cancers worldwide, with a 5-year survival rate of less than 60% (1,2). The most common subtype of OCSCC is tongue squamous cell carcinoma (TSCC), which has the worst prognosis. Recently, an increasing incidence of TSCC among young people has been observed (2,3). Challenges in diagnosis and prognosis prediction for TSCC, such as developing effective strategies for early diagnosis and evaluating the risk of postoperative recurrence, still exist, but reliable approaches to solve these problems are elusive (4-6).
Machine learning (ML) is a technique that involves learning from input data, analyzing data, and outputting the result, which can be used for feature extraction, feature selection, model construction, etc. The ML protocol is shown in Figure 1. ML has been widely used for medical research and has shown excellent performance in multiple aspects, such as diagnosis, efficacy evaluation, and prognosis prediction (7-10).
Radiomics and deep learning (DL), which fall under ML, have developed rapidly. Radiomics extracts numerous features, such as first-order and texture features, from the region of interest (ROI) on medical images while DL can extract deep features (11,12). The features extracted by radiomics and DL, which are invisible to the naked eye, can be analyzed to determine their relationship with diseases (13,14). The utilization of radiomics and DL enables a more accurate and more objective method for medical research and clinical decision (15,16).
In this paper, studies on ML in TSCC were reviewed to highlight that ML may be a potential approach to solve the challenges regarding diagnosis and prognosis prediction for TSCC. We present the following article in accordance with the Narrative Review reporting checklist (available at https://tcr.amegroups.com/article/view/10.21037/tcr-22-1669/rc).
Methods
Literature searches were performed on May 3, 2022 and July 18, 2022 in PubMed, Scopus, Web of Science, and China National Knowledge Infrastructure databases. Papers in English or Chinese, published between the dates of inception of these databases and April 30, 2022, were included. The keyword “tongue cancer” or “tongue squamous cell carcinoma” was used together with either “machine learning”, “radiomics” or “deep learning”. The search results were screened by two reviewers independently through titles and abstracts to select the eligible studies. A summary of the search strategy and the search flow are shown in Table 1 and Figure 2 respectively. After the exclusion of duplicates, irrelevant papers, and papers involving other oral lesions, 24 papers were finally included in the review. Among the papers included, 21 focused on diagnosis and 3 focused on prognosis prediction. There was only 1 article regarding ML in TSCC in 2018, whereas the number of articles concerning ML in TSCC published per year has reached or exceeded 5 since 2019.
Table 1
Items | Specification |
---|---|
Date of search | May 3, 2022 and July 18, 2022 |
Databases and other sources searched | PubMed, Scopus, Web of Science and China National Knowledge Infrastructure |
Search terms used | “tongue cancer” or “tongue squamous cell carcinoma” with either “machine learning”, “radiomics” or “deep learning” |
Timeframe | From inception to April 30, 2022 |
Inclusion and exclusion criteria | Papers in English or Chinese were included |
Selection process | Two reviewers screened the search results independently through titles and abstracts to select the eligible ones |
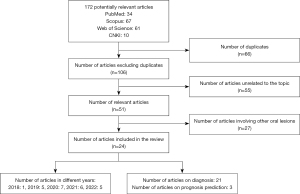
Applications of ML in TSCC
Diagnosis
The conventional screening methods for OCSCC are inspection and palpation. A biopsy is needed to confirm the diagnosis of suspicious lesions (17). However, the result relies largely on the experience of the clinician. Even for experienced clinicians, the result of the biopsy may not represent the whole lesion due to the heterogeneity within the tumor. Additionally, this approach is invasive and expensive (18,19). Thus, it is necessary to develop a noninvasive and reliable diagnostic method.
Lu et al. (19) used hyperspectral imaging (HSI), autofluorescence imaging, and vital-dye fluorescence imaging combined with a variety of ML algorithms for tongue neoplasia detection in mouse models. The area under the curve (AUC), sensitivity, and specificity of the HSI in tongues in vivo were 0.84, 78%, and 78% respectively, and those in tongues ex vivo were 0.86, 79%, and 79% respectively. Due to its non-invasiveness, less dependence on the experience of the clinician and contrast agent, and good performance in vivo and ex vivo, HSI was considered a potential method to detect tongue neoplasia. Manni et al. (20) successfully built a support vector machine (SVM) model based on patient HSI data to detect TSCC, with an AUC of 0.92. Several researchers have constructed DL models based on HSI data, which showed good performance in distinguishing TSCC from normal tissue (21-23). The results supported the point that HSI augmented with ML may have notable potential as a supportive tool for clinicians.
Yu et al. (24) used convolutional neural network (CNN), linear discriminate analysis, and SVM to analyze the Raman spectrum data of tongue specimens to discriminate between TSCC and normal tissue. The sensitivity, specificity, precision, and accuracy of the CNN model were 99.31%, 94.40%, 94.70%, and 96.90%, respectively, which were superior to those of other models. Yan et al. (25,26) found that the CNN model combined with Raman spectroscopy had great potential as a useful tool for the intraoperative evaluation of the resection margins of TSCC. Xia et al. (27) combined CNN and SVM to develop a model for TSCC detection, whose AUC reached 0.99. Ding et al. (28) used the residual network, a type of CNN, to construct a diverse spectral band-based model, which could also distinguish TSCC from normal tissue. CNN was also used to analyze clinical images of tongues for the early detection of TSCC, which showed promising performance (29). These studies indicated that the CNN model can be applied for the evaluation of resection margins during surgery to reduce the possibility of reoperation due to insufficient resection.
Yu et al. (30) constructed magnetic resonance (MR) imaging-based radiomics models with good performance in predicting the degree of pathological differentiation in TSCC. Committeri et al. (31) successfully built an ML model combining radiomics features and clinical parameters to predict tumor grading, with an accuracy of 0.82. These results confirmed the superiority of radiomics in pathologic diagnosis.
Regional lymph node metastasis is considered one of the most important prognostic factors (32). Accurate detection of lymph node metastasis in TSCC can help to select the appropriate treatment strategy. To predict late cervical metastasis in early TSCC, Ariji et al. (33) developed a DL model based on intraoral Doppler ultrasound images, with an AUC of 0.883. Several studies were also conducted to predict lymph node metastasis in early-stage TSCC. Ren et al. (34) found that T2 weighted imaging radiomics signature was an independent predictor of occult lymph node metastasis. Shan et al. (35) used clinicopathologic features to construct four ML models, which showed better performance than the depth of invasion (DOI), neutrophil-to-lymphocyte ratio, and tumor budding. Yuan et al. (36) extracted texture features from MR images and constructed six ML models, among which the Naïve Bayes model achieved the best performance. Kubo et al. (37) used radiomics features of lymph nodes to construct ML models for predicting occult cervical lymph node metastasis. Zhong et al. (38) built artificial neural network (ANN) models incorporating computed tomography radiomics of the primary tumor with traditional lymph node evaluation to detect cervical lymph node metastasis. Both studies showed promising results. Kudoh et al. (39) also found that the model based on positron emission tomography radiomics features performed well in predicting cervical lymph node metastasis in TSCC. Wang et al. (40) showed that models based on MR radiomics signature from the primary tumor with 10 mm peritumoral extensions and clinicopathological characteristics had the highest AUC of 0.995 in the training cohort and 0.872 in the testing cohort. These satisfactory results have revealed the promising prospect of ML.
Prognosis prediction
Surgical resection is the primary treatment for TSCC, but the postoperative recurrence rate cannot be ignored. In a multicenter international study, 27.8% of the patients with early-stage TSCC experienced recurrence, which indicated that patients with a high risk of recurrence may require early intervention to improve prognosis (4). Therefore, the assessment of the risk of recurrence has an important impact on the treatment strategy for TSCC.
Almangush et al. (4) showed that patients with TSCC whose DOI exceeded 4 mm had a higher risk of local recurrence. Alabi et al. (41) compared four ML models with a DOI-based model and found that all the ML models performed significantly better than the DOI-based one. In another research, they constructed two ANN models to estimate the risk of locoregional recurrence in early-stage TSCC based on several parameters of 311 early-stage TSCC patients. The parameters included T stage, WHO histologic grade, DOI, tumor budding, and perineural invasion. The accuracy of the ANN model was 92.7%, which was higher than that of the logistic regression model. The study also indicated that the number of tumor buds and DOI were the most important prognostic factors (42). Another study suggested that ML models could provide a more accurate prediction of overall survival in patients with TSCC compared to a nomogram (43). The excellent performance of the ML models has shown their potential to assist clinical decisions.
Discussion and summary
As the most common type of OCSCC, TSCC is malignant and has a high recurrence rate. The difficulties in diagnosis and prognosis prediction for TSCC still need to be addressed. In recent years, ML has been applied to the analysis of TSCC medical data and performed well in various aspects, such as early detection and recurrence risk evaluation. Despite promising results, ML has its limitations, such as the bias caused by differences in image quality due to various scanners with different parameters, time consumption caused by manual ROI delineation, and the difficulty in explaining the biological significance of radiomics features (44-46). Although some measures have been employed to solve these problems, such as image normalization to standardize image quality, semi-automatic or automatic ROI delineation, and radiogenomics which focuses on the relationship between imaging phenotypes and genomics (47-49), the challenges are still notable. Therefore, more efforts are required to improve ML to make it more helpful for diagnosis and prognosis prediction in TSCC.
Acknowledgments
Funding: This work was supported by
Footnote
Reporting Checklist: The authors have completed the Narrative Review reporting checklist. Available at https://tcr.amegroups.com/article/view/10.21037/tcr-22-1669/rc
Peer Review File: Available at https://tcr.amegroups.com/article/view/10.21037/tcr-22-1669/prf
Conflicts of Interest: All authors have completed the ICMJE uniform disclosure form (available at https://tcr.amegroups.com/article/view/10.21037/tcr-22-1669/coif). The authors have no conflicts of interest to declare.
Ethical Statement: The authors are accountable for all aspects of the work in ensuring that questions related to the accuracy or integrity of any part of the work are appropriately investigated and resolved.
Open Access Statement: This is an Open Access article distributed in accordance with the Creative Commons Attribution-NonCommercial-NoDerivs 4.0 International License (CC BY-NC-ND 4.0), which permits the non-commercial replication and distribution of the article with the strict proviso that no changes or edits are made and the original work is properly cited (including links to both the formal publication through the relevant DOI and the license). See: https://creativecommons.org/licenses/by-nc-nd/4.0/.
References
- Amit M, Yen TC, Liao CT, et al. Improvement in survival of patients with oral cavity squamous cell carcinoma: An international collaborative study. Cancer 2013;119:4242-8. [Crossref] [PubMed]
- Ng JH, Iyer NG, Tan MH, et al. Changing epidemiology of oral squamous cell carcinoma of the tongue: A global study. Head Neck 2017;39:297-304. [Crossref] [PubMed]
- Oliver JR, Wu SP, Chang CM, et al. Survival of oral tongue squamous cell carcinoma in young adults. Head Neck 2019;41:2960-8. [Crossref] [PubMed]
- Almangush A, Bello IO, Coletta RD, et al. For early-stage oral tongue cancer, depth of invasion and worst pattern of invasion are the strongest pathological predictors for locoregional recurrence and mortality. Virchows Arch 2015;467:39-46. [Crossref] [PubMed]
- Han S, Yang X, Qi Q, et al. Potential screening and early diagnosis method for cancer: Tongue diagnosis. Int J Oncol 2016;48:2257-64. [Crossref] [PubMed]
- Jéhannin-Ligier K, Dejardin O, Lapôtre-Ledoux B, et al. Oral cancer characteristics in France: Descriptive epidemiology for early detection. J Stomatol Oral Maxillofac Surg 2017;118:84-9. [Crossref] [PubMed]
- Mao B, Ma J, Duan S, et al. Preoperative classification of primary and metastatic liver cancer via machine learning-based ultrasound radiomics. Eur Radiol 2021;31:4576-86. [Crossref] [PubMed]
- Greener JG, Kandathil SM, Moffat L, et al. A guide to machine learning for biologists. Nat Rev Mol Cell Biol 2022;23:40-55. [Crossref] [PubMed]
- Wang Y, Ji C, Wang Y, et al. Predicting postoperative liver cancer death outcomes with machine learning. Curr Med Res Opin 2021;37:629-34. [Crossref] [PubMed]
- Dong Z, Lin Y, Lin F, et al. Prediction of Early Treatment Response to Initial Conventional Transarterial Chemoembolization Therapy for Hepatocellular Carcinoma by Machine-Learning Model Based on Computed Tomography. J Hepatocell Carcinoma 2021;8:1473-84. [Crossref] [PubMed]
- Park HJ, Park B, Lee SS. Radiomics and Deep Learning: Hepatic Applications. Korean J Radiol 2020;21:387-401. [Crossref] [PubMed]
- Park JE, Kickingereder P, Kim HS. Radiomics and Deep Learning from Research to Clinical Workflow: Neuro-Oncologic Imaging. Korean J Radiol 2020;21:1126-37. [Crossref] [PubMed]
- Esteva A, Robicquet A, Ramsundar B, et al. A guide to deep learning in healthcare. Nat Med 2019;25:24-9. [Crossref] [PubMed]
- Shur JD, Doran SJ, Kumar S, et al. Radiomics in Oncology: A Practical Guide. Radiographics 2021;41:1717-32. [Crossref] [PubMed]
- Ni M, Wen X, Chen W, et al. A Deep Learning Approach for MRI in the Diagnosis of Labral Injuries of the Hip Joint. J Magn Reson Imaging 2022;56:625-34. [Crossref] [PubMed]
- Li G, Li L, Li Y, et al. An MRI radiomics approach to predict survival and tumour-infiltrating macrophages in gliomas. Brain 2022;145:1151-61. [Crossref] [PubMed]
- Rashid A, Warnakulasuriya S. The use of light-based (optical) detection systems as adjuncts in the detection of oral cancer and oral potentially malignant disorders: a systematic review. J Oral Pathol Med 2015;44:307-28. [Crossref] [PubMed]
- van den Brekel MW, Lodder WL, Stel HV, et al. Observer variation in the histopathologic assessment of extranodal tumor spread in lymph node metastases in the neck. Head Neck 2012;34:840-5. [Crossref] [PubMed]
- Lu G, Wang D, Qin X, et al. Detection and delineation of squamous neoplasia with hyperspectral imaging in a mouse model of tongue carcinogenesis. J Biophotonics 2018; [Crossref] [PubMed]
- Manni F, van der Sommen F, Zinger S, et al. Automated tumor assessment of squamous cell carcinoma on tongue cancer patients with hyperspectral imaging. Conference on Medical Imaging - Image-Guided Procedures, Robotic Interventions, and Modeling; 2019 Feb 17-19; San Diego, CA 2019.
- Weijtmans PJC, Shan C, Tan T, et al. A dual stream network for tumor detection in hyperspectral images. 16th IEEE International Symposium on Biomedical Imaging (ISBI); 2019 Apr 08-11; Venice, Italy 2019.
- Brouwer de Koning SG, Weijtmans P, Karakullukcu MB, et al. Toward assessment of resection margins using hyperspectral diffuse reflection imaging (400-1,700 nm) during tongue cancer surgery. Lasers Surg Med 2020;52:496-502. [Crossref] [PubMed]
- Trajanovski S, Shan C, Weijtmans PJC, et al. Tongue Tumor Detection in Hyperspectral Images Using Deep Learning Semantic Segmentation. IEEE Trans Biomed Eng 2021;68:1330-40. [Crossref] [PubMed]
- Yu M, Yan H, Xia J, et al. Deep convolutional neural networks for tongue squamous cell carcinoma classification using Raman spectroscopy. Photodiagnosis Photodyn Ther 2019;26:430-5. [Crossref] [PubMed]
- Yan H, Yu MX, Xia JB, et al. Tongue squamous cell carcinoma discrimination with Raman spectroscopy and convolutional neural networks. Vibrational Spectroscopy 2019;103: [Crossref]
- Yan H, Yu MX, Xia JB, et al. Diverse Region-Based CNN for Tongue Squamous Cell Carcinoma Classification With Raman Spectroscopy. IEEE Access 2020;8:127313-28.
- Xia JB, Zhu LQ, Yu MX, et al. Analysis and classification of oral tongue squamous cell carcinoma based on Raman spectroscopy and convolutional neural networks. J Mod Opt 2020;67:481-9. [Crossref]
- Ding J, Yu M, Zhu L, et al. Diverse spectral band-based deep residual network for tongue squamous cell carcinoma classification using fiber optic Raman spectroscopy. Photodiagnosis Photodyn Ther 2020;32:102048. [Crossref] [PubMed]
- Jubair F, Al-Karadsheh O, Malamos D, et al. A novel lightweight deep convolutional neural network for early detection of oral cancer. Oral Dis 2022;28:1123-30. [Crossref] [PubMed]
- Yu B, Huang C, Xu J, et al. Prediction of the degree of pathological differentiation in tongue squamous cell carcinoma based on radiomics analysis of magnetic resonance images. BMC Oral Health 2021;21:585. [Crossref] [PubMed]
- Committeri U, Fusco R, Di Bernardo E, et al. Radiomics Metrics Combined with Clinical Data in the Surgical Management of Early-Stage (cT1-T2 N0) Tongue Squamous Cell Carcinomas: A Preliminary Study. Biology (Basel) 2022.
- Pantel K, Brakenhoff RH. Dissecting the metastatic cascade. Nat Rev Cancer 2004;4:448-56. [Crossref] [PubMed]
- Ariji Y, Fukuda M, Kise Y, et al. A preliminary application of intraoral Doppler ultrasound images to deep learning techniques for predicting late cervical lymph node metastasis in early tongue cancers. Oral Science International 2020;17:59-66. [Crossref]
- Ren J, Song Q, Yuan Y, et al. Value of MRI radiomics for predicting occult cervical lymph nodes metastases in early-stage oral tongue squamous cell carcinoma. Chinese Journal of Radiology 2022;56:30-5.
- Shan J, Jiang R, Chen X, et al. Machine Learning Predicts Lymph Node Metastasis in Early-Stage Oral Tongue Squamous Cell Carcinoma. J Oral Maxillofac Surg 2020;78:2208-18. [Crossref] [PubMed]
- Yuan Y, Ren J, Tao X. Machine learning-based MRI texture analysis to predict occult lymph node metastasis in early-stage oral tongue squamous cell carcinoma. Eur Radiol 2021;31:6429-37. [Crossref] [PubMed]
- Kubo K, Kawahara D, Murakami Y, et al. Development of a radiomics and machine learning model for predicting occult cervical lymph node metastasis in patients with tongue cancer. Oral Surg Oral Med Oral Pathol Oral Radiol 2022;134:93-101. [Crossref] [PubMed]
- Zhong YW, Jiang Y, Dong S, et al. Tumor radiomics signature for artificial neural network-assisted detection of neck metastasis in patient with tongue cancer. J Neuroradiol 2022;49:213-8. [Crossref] [PubMed]
- Kudoh T, Haga A, Kudoh K, et al. Radiomics analysis of [(18)F]-fluoro-2-deoxyglucose positron emission tomography for the prediction of cervical lymph node metastasis in tongue squamous cell carcinoma. Oral Radiol 2022; Epub ahead of print. [Crossref]
- Wang F, Tan R, Feng K, et al. Magnetic Resonance Imaging-Based Radiomics Features Associated with Depth of Invasion Predicted Lymph Node Metastasis and Prognosis in Tongue Cancer. J Magn Reson Imaging 2022;56:196-209. [Crossref] [PubMed]
- Alabi RO, Elmusrati M, Sawazaki-Calone I, et al. Comparison of supervised machine learning classification techniques in prediction of locoregional recurrences in early oral tongue cancer. Int J Med Inform 2020;136:104068. [Crossref] [PubMed]
- Alabi RO, Elmusrati M, Sawazaki-Calone I, et al. Machine learning application for prediction of locoregional recurrences in early oral tongue cancer: a Web-based prognostic tool. Virchows Arch 2019;475:489-97. [Crossref] [PubMed]
- Alabi RO, Mäkitie AA, Pirinen M, et al. Comparison of nomogram with machine learning techniques for prediction of overall survival in patients with tongue cancer. Int J Med Inform 2021;145:104313. [Crossref] [PubMed]
- Huang YQ, Liang CH, He L, et al. Development and Validation of a Radiomics Nomogram for Preoperative Prediction of Lymph Node Metastasis in Colorectal Cancer. J Clin Oncol 2016;34:2157-64. [Crossref] [PubMed]
- Li X, Liang D, Meng J, et al. Development and Validation of a Novel Computed-Tomography Enterography Radiomic Approach for Characterization of Intestinal Fibrosis in Crohn's Disease. Gastroenterology 2021;160:2303-2316.e11. [Crossref] [PubMed]
- Shin J, Seo N, Baek SE, et al. MRI Radiomics Model Predicts Pathologic Complete Response of Rectal Cancer Following Chemoradiotherapy. Radiology 2022;303:351-8. [Crossref] [PubMed]
- Liu Z, Wang S, Dong D, et al. The Applications of Radiomics in Precision Diagnosis and Treatment of Oncology: Opportunities and Challenges. Theranostics 2019;9:1303-22. [Crossref] [PubMed]
- Huang B, Lin X, Shen J, et al. Accurate and Feasible Deep Learning Based Semi-Automatic Segmentation in CT for Radiomics Analysis in Pancreatic Neuroendocrine Neoplasms. IEEE J Biomed Health Inform 2021;25:3498-506. [Crossref] [PubMed]
- Zhang M, Wong SW, Wright JN, et al. MRI Radiogenomics of Pediatric Medulloblastoma: A Multicenter Study. Radiology 2022;304:406-16. [Crossref] [PubMed]