Low correlation of lncRNA and target gene expression in microarray data
Introduction
With the advancement in high-throughput genomic technologies such as microarrays and next-generation sequencing (NGS), many non-coding RNAs (ncRNAs) have been reported (1,2). The most important feature of these ncRNAs is that no functional protein products are generated from their RNA transcripts. Although the biological functions and signaling pathways of ncRNAs remain unclear, several studies have indicated that ncRNAs may be an important player in a variety of biochemical processes and diseases (3,4).
The long non-coding RNAs (lncRNAs) are a subset of ncRNAs characterized by lengths longer than 200 nucleotides. Similar to ncRNAs in general, the biological functions of lncRNAs are not fully elucidated; however, several studies have suggested that lncRNAs may participate in regulating the expression of genes and proteins at both the transcriptional and translational levels (5). One study demonstrated that lncRNAs are able to recruit some chromatin-modifying enzymes to activate or inhibit their target genes at the transcriptional level (6). For example, the lncRNA HOTAIR can interact with Polycomb repressive complex 2 (PRC2) to modify the chromatin status of its target genes in mammalian cells (7). In addition, lncRNAs are known to regulate mRNA splicing and degradation, as well as the translation and activity of proteins. However, further investigation is required to understand these regulatory mechanisms and the biological importance of lncRNAs in disease.
Several experimental technologies can be used to measure the expression level of lncRNAs, including real-time polymerase chain reaction (PCR), microarrays, and NGS. Real-time PCR is a standard approach to analyze the expression levels of mRNAs and lncRNAs. Based on the precise primer design in real-time PCR, the specificity is superior to the other two approaches. However, a critical limitation of real-time PCR is its low throughput, which makes it difficult to analyze a whole genome. In contrast, microarray and NGS technologies are high-throughput methods, which allow researchers to examine the expression levels of genome-wide RNA transcripts in only one experiment.
Recently, several studies have shown that the expression level of lncRNAs is detectable in both microarray and NGS platforms (8,9). Since microarray methods have been used for more than two decades, many microarray data from different biological conditions and disease types are available in the public domain. However, lncRNA is a relatively new concept and thus few microarray experiments have been designed specifically for lncRNAs. To address this issue, a previous report has shown that the expression levels of some lncRNAs can be obtained using the Affymetrix gene expression microarray platforms, based on the re-annotation of their probe sequences (10). A NGS study indicated that the expression level of lncRNAs varies from tissue to tissue according to the RNA-seq data in multiple human tissues (11,12). Compared with microarrays, NGS technology is a better approach to investigate lncRNAs, because it does not require a priori knowledge of the nucleotide sequences of lncRNAs. Currently, the most important limitations for NGS technology in analyzing lncRNAs are the high cost and the small sample sizes of data in the public domain. Therefore, we focused on the gene expression microarray data to do the bioinformatics analyses of lncRNAs in this study.
Identification of the target genes of lncRNAs poses a major challenge. Our knowledge of lncRNAs and their target genes is still limited, even though some online databases are available for depositing information on lncRNAs (13,14). To date, the most popular approach to identify possible target genes of lncRNAs is based on the correlation coefficients between the expression levels of lncRNAs and genes (15,16). Only a few lncRNA-gene pairs have been validated by in vitro experiments (17). However, whether correlation coefficients are a good way to identify possible target genes of lncRNAs remains unclear. To address this issue, known lncRNAs and their target genes were downloaded from a publicly available database and manually curated based on the literature. Pearson correlation coefficients were calculated to examine the associations between the expression levels of the lncRNAs and their target genes in several biological scenarios. The findings indicate that using expression levels is not a good approach for identifying possible target genes of lncRNAs.
Methods
Microarray datasets
Ten published and publicly available gene expression microarrays from the Affymetrix u133plus 2.0 platform were retrieved from the Gene Expression Omnibus (GEO) (18). The sample characteristics are summarized in Table 1. Five different tissues, including breast, blood, brain, lung, and skin were analyzed. For each probe, the gene symbol was obtained from the official annotation file provided by Affymetrix. All analyses were conducted using the affy package with the default parameters in the R program (29). The robust multiarray average (RMA) algorithm was performed to reduce the systematic bias in one dataset. The correspondence between probe names and lncRNAs is provided in a previous study (10).
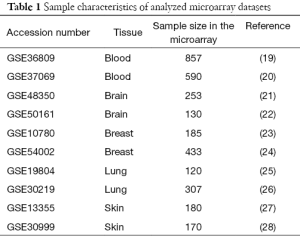
Full table
Identification of long non-coding RNA (lncRNA)-target gene pairs
Known pairings of lncRNAs with their target genes were retrieved from an online database, LncRNA2Target (13). Because the microarray data investigated the expression levels of messenger RNA (mRNA), we checked whether the association of a lncRNA with its target gene was reflected in the mRNA levels as described in the original references (Table S1). Lastly, after mapping to the Affymetrix u133plus 2.0 microarray platform, 956 pairs of lncRNAs and their target genes were identified (Table S2).
Associations of long non-coding RNAs (lncRNAs) and their target genes in different biological scenarios
For each microarray dataset, the Pearson correlation coefficients were calculated to quantitatively estimate the strength of the association between the expression of a lncRNA and the expression of its target gene(s). The correlation coefficients were further classified into three groups as the follows: low correlation (r<0.3), medium correlation (0.3<r<0.7), and high correlation (r>0.7). A total of six scenarios were investigated. The first scenario was to investigate the 956 pairs of lncRNAs and their target genes. Subsequently, to establish a null baseline for comparison, a re-sampling test was performed (scenario 2). That is, we randomly selected a pair of probes targeting one lncRNA and one gene to calculate their correlation, and this re-sampling procedure was repeated 100,000 times. Furthermore, samples were classified into two phenotype groups based on their characteristics defined by their original references (Table 2). Based on the groupings, an unpaired t-test was performed to identify differentially expressed lncRNAs (P<0.05). The significant lncRNAs were further classified into two groups based on which phenotype group had higher expression levels (scenarios 3 and 4).
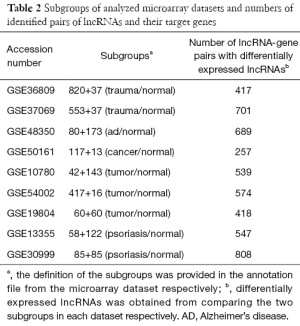
Full table
Associations of long non-coding RNAs (lncRNAs) and their target genes in lung tissue
Since several studies have indicated that the expression levels of lncRNAs are tissue-specific (11,12), we further annotated the mapping file to include the tissue type, based on the original references. Although we intended to analyze all five tissues shown in Table 1, the published information about the tissue in which pairs of lncRNAs and their target genes are expressed is relatively limited. The highest number (N=27) of annotated lncRNA was reported in lung tissue, and thus we focused on the two lung datasets, GSE19804 and GSE30219, to do the comparisons. On the microarray platform, a total of 506 probe combinations of lncRNAs and their target genes were identified according to the 27 lncRNAs specific to lung tissue (Table S2), and only these 506 pairs showing significantly differential expression were selected for further analyses (scenarios 5 and 6).
Results
Identification of pairs of long non-coding RNAs (lncRNAs) and their target genes in the Affymetrix u133plus 2.0 microarray platform
Since all microarray data were examined in the Affymetrix u133plus 2.0 microarray, we obtained the gene symbol for each probe based on the official annotation file provided by Affymetrix. To identify the probes targeting lncRNAs, a previous study (10) was utilized, and 3,029 probes were found to correspond to lncRNA sequences. Subsequently, pairs of lncRNAs and their target genes were downloaded from the LncRNA2Target database (13). Since the microarray data contained the expression levels of mRNA, we focused on the lncRNA-gene pairs reported to participate in the regulation of transcription. A total of 74 pairs were identified and summarized in Table S1. Since microarray data contain multiple probes targeting the same gene, the 74 pairs could be mapped to 956 combinations of probes (Table S2). Therefore, further analyses were performed on these 956 pairs.
Low correlations between the expression levels of long non-coding RNAs (lncRNAs) and their target genes (scenarios 1–2)
To examine whether the expression level of a lncRNA is associated with that of its target gene, Pearson correlation coefficients were calculated among the 956 combinations of known lncRNAs and their target genes. The results are summarized in Table 3. We plotted the distribution of correlations in Figure 1. The correlation values are shown in the x-axis and the cumulated frequency of lncRNA-gene pairs is shown in the y-axis. Low correlation values were reported in the scenario 1 across all ten datasets, and the absolute value of the maximum deviation to zero is only around 0.05. Therefore, the expression level of probes targeting lncRNA is not correlated with the expression level of their target genes. To further confirm the low correlations, a resampling test was performed with 100,000 iterations. The details about the resampling test are described in the Methods. As shown in Figure 1, the distributions of correlation values obtained from the resampling test in all ten datasets are all similar to those calculated for the 956 curated pairs. The maximum difference of the correlation values between the curated pairs and the resampling test is less than 0.1 and most of them are less than 0.03. These results demonstrated that no strong correlations exist between the expression levels of lncRNAs and their target genes, suggesting that it is not advisable to identify target genes of lncRNAs based on the similarity of their expression patterns in microarray data.
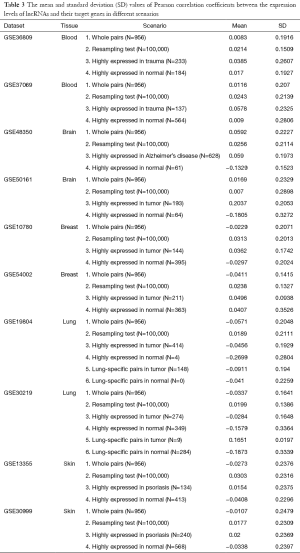
Full table
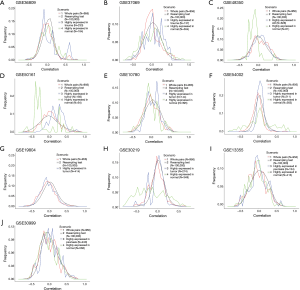
Higher correlations were observed when the long non-coding RNAs (lncRNAs) were differentially expressed (scenarios 3–4)
It is possible that the low correlations between lncRNAs and their target genes were observed because most of the examined lncRNAs were not expressed under the conditions of the microarray experiment. To exclude this possibility, the unpaired t-test was performed to identify lncRNAs with significantly different expression (P<0.05) in two different phenotypes. The phenotypes groups in each dataset were shown in Table 2 and the definitions were based on their original references. Subsequently, the differentially expressed lncRNAs were classified into two groups based on the tissue type in which they had higher expression levels. Similar to the previous approach, Pearson correlation coefficients were calculated to evaluate the associations between expression of lncRNAs and their target genes. Intriguingly, the absolute value of the correlations became higher (Table 3), suggesting stronger associations of the lncRNAs and their target genes were reported. However, the directions of the correlations were not consistent in different datasets. For examples, a peak of the positive correlations exists in the GSE36809 dataset, whereas a peak of the negative correlations was observed in the GSE48350 and GSE50161 datasets (Figure 1). This makes it difficult to determine the possible target genes, since both positive and negative correlations should be taken into consideration.
Low correlations between the expression of long non-coding RNAs (lncRNAs) and their target genes in lung tissue (scenarios 5–6)
Previous studies have shown that the expression levels of lncRNAs vary in different tissues. To further confirm whether the low correlations described above result from the lncRNAs being expressed in different tissues, we annotated the lncRNAs and their target genes based on their original references. Since lncRNA is a relatively new topic in the research field, information on their tissue types is limited. Among the 5 tissues examined in this study, lung tissue had the highest number of lncRNAs (N=27) with documentation. In the Affymetrix u133plus 2.0 microarray platform, these 27 lncRNAs and their target genes specific to lung tissue can be mapped as 506 pairs. Among the 506 pairs, we focused on the pairs with lncRNAs showing differential expression, since higher correlations were reported. The two microarray datasets targeting lung cancer patients, GSE19804 and GSE3021, were analyzed accordingly (Figure 2).
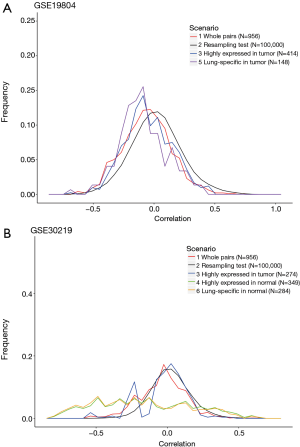
The Pearson correlation coefficients of lung-specific lncRNA-gene pairs in different scenarios are summarized in Table 3 (scenarios 5 and 6). Although their absolute values were higher than those in scenarios 3 and 4 (the classification by phenotype), the absolute values were still less than 0.2, which is regarded as low. However, more lncRNAs and their target genes specific to lung tissue should be analyzed in the future to confirm the conclusion, since the sample sizes were quite limited (N=0 for scenario 6 in GSE19804 and N=9 for scenario 5 in GSE30219). These results suggest that the correlation between the expression levels of lncRNAs and their target genes was low even if the lncRNAs exhibited differential expression and were derived from a particular tissue.
Discussion
LncRNAs have been revealed as an important player in modulating the expression levels of their target genes and proteins (30,31). It has become essential to identify possible target genes of lncRNAs. The most popular approach for identifying the target gene of a lncRNA is to use the similarity in their expression levels. However, no systematic investigations have been performed to examine the validity of such associations. Our results demonstrated that this approach is ineffective, as indicated by the fact that the correlation coefficients between lncRNA and target gene expression levels in biological samples were not significantly different than those in the random resampling test (Table 3). Three pairs of scenarios were considered in this study, and all of them showed similar correlation values, suggesting that no differences exist. Therefore, other approaches for predicting possible target genes of lncRNAs should be developed.
We selected the Affymetrix u133plus 2.0 microarray to do the comparisons for two reasons. First, the Affymetrix u133plus 2.0 microarray has the largest numbers of datasets and samples in the public domain and thus more tissues types and biological statuses can be analyzed concurrently. Second, several previous studies have demonstrated that the probes in the Affymetrix u133plus 2.0 platform can be used to examine the expression levels of lncRNAs (8,10). Therefore, we can directly use data from the gene expression microarray to simultaneously investigate lncRNAs and genes without introducing systematic bias. In addition to the Affymetrix platform, many studies have been conducted using microarrays from Illumina. Unfortunately, the probe sequences in the Illumina microarrays are not available to the public and thus re-annotation is not possible for detecting lncRNAs.
The Pearson correlation coefficients of the expression levels of lncRNAs and their target genes were low in all scenarios (Table 3), suggesting the association of lncRNA with mRNA expression levels is low. One possible reason for this finding is the incompleteness of the probes targeting lncRNAs. Since the original purpose of the Affymetrix u133plus 2.0 microarray was targeting coding genes, not all lncRNAs are detected using the microarray data. In addition, our knowledge about lncRNAs is still very limited. Only a few pairs of lncRNAs and their target genes have been reported, which may cause us to unknowingly discard or overlook some relevant information about the whole picture of associations. However, this issue can only be addressed after more data are accumulated.
Although low correlations of the lncRNAs and their target genes were reported in all scenarios, certain limitations exist in this study. We examined ten microarray datasets with at least 100 samples in five different tissues. However, the sample sizes and the tissue types are still limited. Larger sample sizes and more independent microarray datasets are required to confirm the results. In addition, many of the 10 datasets have unbalanced sample distributions for the two phenotype groups within them, which may introduce some bias into the identification of those significant lncRNAs. Additional analyses should be conducted with similar sample sizes in the two phenotype groups. We attempted to explore whether the associations were specific to different tissue types; however, only the lung tissue had enough annotated lncRNAs and target gene pairs to do this. Consequently, only lung tissue was examined in this study, and low correlations were observed. More tissue types should be analyzed using the same approach in the future to further demonstrate the associations.
In conclusion, pairs of lncRNAs and their corresponding target genes showed low correlation between lncRNA and gene expression in all scenarios. Neither differential expression of the lncRNAs nor limiting the analysis to a specific tissue type improved the correlations. Therefore, researchers should be more conservative when identifying possible target genes of lncRNAs using gene expression microarray data and should seek to validate the proposed targets by other methods.
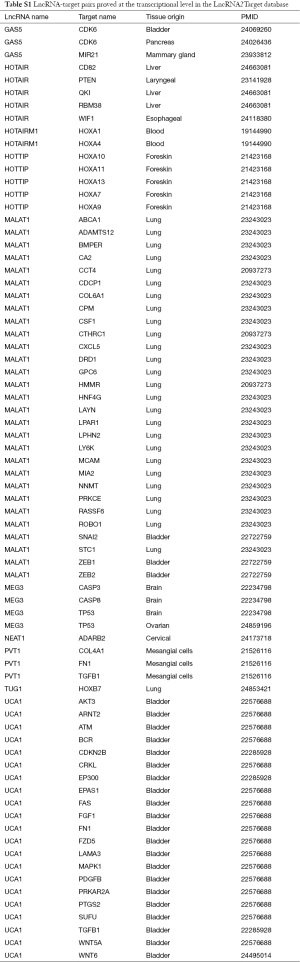
Full table

Full table
Acknowledgments
We thank Melissa Stauffer, PhD, of Scientific Editing Solutions, for editing the manuscript.
Funding: This work was supported in part by the Center of Genomic Medicine, National Taiwan University, Taiwan with the grant number: 104R8400, the YongLin biomedical engineering center, National Taiwan University, Taiwan with the grant number: FB0027, and the Center of Biotechnology, National Taiwan University, Taiwan with the grant number: GTZ300. The funders had no role in the study design; in the collection, analysis, or interpretation of data; in the writing of the manuscript; or in the decision to submit the manuscript for publication.
Footnote
Conflicts of Interest: All authors have completed the ICMJE uniform disclosure form (available at http://dx.doi.org/10.21037/tcr.2016.04.07). Eric Y. Chuang serves as the Editor-in-Chief of Translational Cancer Research. The other author has no conflicts of interest to declare.
Ethical Statement: The authors are accountable for all aspects of the work in ensuring that questions related to the accuracy or integrity of any part of the work are appropriately investigated and resolved. The study was conducted in accordance with the Declaration of Helsinki (as revised in 2013).
Open Access Statement: This is an Open Access article distributed in accordance with the Creative Commons Attribution-NonCommercial-NoDerivs 4.0 International License (CC BY-NC-ND 4.0), which permits the non-commercial replication and distribution of the article with the strict proviso that no changes or edits are made and the original work is properly cited (including links to both the formal publication through the relevant DOI and the license). See: https://creativecommons.org/licenses/by-nc-nd/4.0/.
References
- Schena M, Shalon D, Davis RW, et al. Quantitative monitoring of gene expression patterns with a complementary DNA microarray. Science 1995;270:467-70. [Crossref] [PubMed]
- Marguerat S, Bähler J. RNA-seq: from technology to biology. Cell Mol Life Sci 2010;67:569-79. [Crossref] [PubMed]
- Costa FF. Non-coding RNAs: new players in eukaryotic biology. Gene 2005;357:83-94. [Crossref] [PubMed]
- Mercer TR, Dinger ME, Mattick JS. Long non-coding RNAs: insights into functions. Nat Rev Genet 2009;10:155-9. [Crossref] [PubMed]
- Fitzgerald KA, Caffrey DR. Long noncoding RNAs in innate and adaptive immunity. Curr Opin Immunol 2014;26:140-6. [Crossref] [PubMed]
- Plath K, Fang J, Mlynarczyk-Evans SK, et al. Role of histone H3 lysine 27 methylation in X inactivation. Science 2003;300:131-5. [Crossref] [PubMed]
- Rinn JL, Kertesz M, Wang JK, et al. Functional demarcation of active and silent chromatin domains in human HOX loci by noncoding RNAs. Cell 2007;129:1311-23. [Crossref] [PubMed]
- Michelhaugh SK, Lipovich L, Blythe J, et al. Mining Affymetrix microarray data for long non-coding RNAs: altered expression in the nucleus accumbens of heroin abusers. J Neurochem 2011;116:459-66. [Crossref] [PubMed]
- Atkinson SR, Marguerat S, Bähler J. Exploring long non-coding RNAs through sequencing. Semin Cell Dev Biol 2012;23:200-5. [Crossref] [PubMed]
- Du Z, Fei T, Verhaak RG, et al. Integrative genomic analyses reveal clinically relevant long noncoding RNAs in human cancer. Nat Struct Mol Biol 2013;20:908-13. [Crossref] [PubMed]
- Tsoi LC, Iyer MK, Stuart PE, et al. Analysis of long non-coding RNAs highlights tissue-specific expression patterns and epigenetic profiles in normal and psoriatic skin. Genome Biol 2015;16:24. [Crossref] [PubMed]
- Iyer MK, Niknafs YS, Malik R, et al. The landscape of long noncoding RNAs in the human transcriptome. Nat Genet 2015;47:199-208. [Crossref] [PubMed]
- Jiang Q, Wang J, Wu X, et al. LncRNA2Target: a database for differentially expressed genes after lncRNA knockdown or overexpression. Nucleic Acids Res 2015;43:D193-6. [Crossref] [PubMed]
- Quek XC, Thomson DW, Maag JL, et al. lncRNAdb v2.0: expanding the reference database for functional long noncoding RNAs. Nucleic Acids Res 2015;43:D168-73. [Crossref] [PubMed]
- Liao Q, Liu C, Yuan X, et al. Large-scale prediction of long non-coding RNA functions in a coding-non-coding gene co-expression network. Nucleic Acids Res 2011;39:3864-78. [Crossref] [PubMed]
- Dong R, Jia D, Xue P, et al. Genome-wide analysis of long noncoding RNA (lncRNA) expression in hepatoblastoma tissues. PLoS One 2014;9:e85599 [Crossref] [PubMed]
- Wan ZY, Song F, Sun Z, et al. Aberrantly expressed long noncoding RNAs in human intervertebral disc degeneration: a microarray related study. Arthritis Res Ther 2014;16:465. [Crossref] [PubMed]
- Edgar R, Domrachev M, Lash AE. Gene Expression Omnibus: NCBI gene expression and hybridization array data repository. Nucleic Acids Res 2002;30:207-10. [Crossref] [PubMed]
- Xiao W, Mindrinos MN, Seok J, et al. A genomic storm in critically injured humans. J Exp Med 2011;208:2581-90. [Crossref] [PubMed]
- Seok J, Warren HS, Cuenca AG, et al. Genomic responses in mouse models poorly mimic human inflammatory diseases. Proc Natl Acad Sci U S A 2013;110:3507-12. [Crossref] [PubMed]
- Berchtold NC, Cribbs DH, Coleman PD, et al. Gene expression changes in the course of normal brain aging are sexually dimorphic. Proc Natl Acad Sci U S A 2008;105:15605-10. [Crossref] [PubMed]
- Griesinger AM, Birks DK, Donson AM, et al. Characterization of distinct immunophenotypes across pediatric brain tumor types. J Immunol 2013;191:4880-8. [Crossref] [PubMed]
- Chen DT, Nasir A, Culhane A, et al. Proliferative genes dominate malignancy-risk gene signature in histologically-normal breast tissue. Breast Cancer Res Treat 2010;119:335-46. [Crossref] [PubMed]
- Tan TZ, Miow QH, Miki Y, et al. Epithelial-mesenchymal transition spectrum quantification and its efficacy in deciphering survival and drug responses of cancer patients. EMBO Mol Med 2014;6:1279-93. [Crossref] [PubMed]
- Lu TP, Tsai MH, Lee JM, et al. Identification of a novel biomarker, SEMA5A, for non-small cell lung carcinoma in nonsmoking women. Cancer Epidemiol Biomarkers Prev 2010;19:2590-7. [Crossref] [PubMed]
- Rousseaux S, Debernardi A, Jacquiau B, et al. Ectopic activation of germline and placental genes identifies aggressive metastasis-prone lung cancers. Sci Transl Med 2013;5:186ra66.
- Nair RP, Duffin KC, Helms C, et al. Genome-wide scan reveals association of psoriasis with IL-23 and NF-kappaB pathways. Nat Genet 2009;41:199-204. [Crossref] [PubMed]
- Suárez-Fariñas M, Li K, Fuentes-Duculan J, et al. Expanding the psoriasis disease profile: interrogation of the skin and serum of patients with moderate-to-severe psoriasis. J Invest Dermatol 2012;132:2552-64. [Crossref] [PubMed]
- Gautier L, Cope L, Bolstad BM, et al. affy--analysis of Affymetrix GeneChip data at the probe level. Bioinformatics 2004;20:307-15. [Crossref] [PubMed]
- Nagano T, Fraser P. No-nonsense functions for long noncoding RNAs. Cell 2011;145:178-81. [Crossref] [PubMed]
- Monnier P, Martinet C, Pontis J, et al. H19 lncRNA controls gene expression of the Imprinted Gene Network by recruiting MBD1. Proc Natl Acad Sci U S A 2013;110:20693-8. [Crossref] [PubMed]