Applying gene set analysis to characterize the activities of immune cells in estrogen receptor positive breast cancer
Introduction
Breast cancer is one of the prevalent cancer types in female in the United States and world-wide (1). Three major molecular subtypes of breast cancer have been identified by the genetic profiles in tumors: estrogen receptor positive (ER+), human epidermal growth factor receptor 2 positive (HER2+), and triple negative breast cancer (2,3). About 70% of human breast cancers are ER+, and most ER+ breast cancer patients benefited from hormone therapies, such as tamoxifen (4-6). However, still 30% of ER+ tumors do not respond well to hormone therapy (7).
Previous studies showed the tumor infiltrating lymphocytes (TILs) were associated with prognosis and treatment response of breast cancer (8-12). For example, large amount of TILs were prone to decreased recurrence in triple negative breast cancer (8). High levels of TILs also increased trastuzumab benefit in HER2+ disease (8). However, most of these studies focused on the HER2+ and triple negative subtypes. The role of TILs in ER+ breast cancer was rarely discussed.
Recently, FDA approved ipilimumab (anti-CTLA4 antibody), nivolumab, and pembrolizumab (anti PD-1 antibody) for cancer immunotherapies to inhibit the immune checkpoint blockade, PD-1 and CTLA-4 (13-15). One of the determining factors of responses to immunotherapy is the presentation of neoantigens and the activation of immune system. Although the roles of different immune cells in cancer progression have been individually studied and reviewed (16,17), a systematic study which comprehensively investigates all various types of immune cells in specific subtype of breast cancer remains an unchartered territory.
In this study, we utilized a gene set enrichment approach to analyze subtypes of TILs. Using genome-wide expression profiles derived from breast tumors, the landscape of immunology in breast cancer and its association with tumor prognosis were explored. The findings could provide a biological basis of tumor infiltrating immune cells in ER+ tumors and benefit the development of immunotherapy.
Methods
Immune gene sets and microarray data sets
A total of thirty-one immune cell associated gene sets, collected from a previous research (18), were used in the study. We analyzed three data sets of breast cancer, GSE4922 (19), GSE2034 (20) and GSE2990 (21). The gene expression profiles were downloaded from Gene Expression Omnibus (GEO) (22). A total of 714 samples were used in the study, and the summary of the three data set was list in Table S1. Patients without survival information or ER status were excluded, and all samples were divided into subgroups based on ER status (ER+ and ER
Gene set enrichment score
In gene set analysis, we used gene set enrichment scores to represent gene activities of immunological gene sets. The gene set enrichment score was defined as the averaged normalized expression value of the member genes in a given data set. Suppose a gene set s consist N genes and the log2-transformed expression level of gene j in sample i be xj,i. The enrichment score is defined as below:
Where is the mean value of gene j in a gene set among samples, and is the standard deviation.
Statistical analysis
Patient survival information in the data sets was retrieved from the GEO database. Both Kaplan-Meier estimator method (23) and Cox hazard proportional model (24) were applied. In Kaplan-Meier analysis, patients were divided into two groups based on the median of gene set enrichment score. For multivariate analysis, the analyzed risk factors in the Cox hazard proportional model were the status of lymph node (LN) involvement, enrichment score of cell cycle indexing genes (25), consensus ER+ prognostic genes (26), and the immune cell associated gene sets. Association between each pair of immune gene sets was calculated by Pearson correlation.
Annotation of biological functions by gene set enrichment analysis (GSEA)
GSEA (27) was applied to identify the gene sets enriched in the sub-clusters of 211 breast cancer samples. The gene sets are collected from the Molecular Signatures Database (MSigDB) v5.0 (28). A total of 3,951 gene sets including the gene set of C2-curated chemical or genetic perturbations (CGP), C3-transcription factor targets (TFT), C5-gene ontology biological process (GO-BP), and C6-oncogenic signatures were analyzed in this study.
Results
Study overview
To explore the association of TILs and patient’s survival in ER+ breast cancer, 31 previously defined gene sets were used to present the subpopulation of TILs. The immune cell gene sets were applied for survival analysis. The overall analysis flowchart is showed in Figure 1. The samples were first divided into two groups according to the ER status, and the enrichment score of each gene set was computed for each sample. Then, for each group, the enrichment scores were applied for Cox regression analysis. Statistically significant gene sets were identified based on the threshold of Cox P value <0.05. Moreover, to validate the results from survival analysis, we included two other datasets, GSE2990 and GSE2034. The results were consistent to what we found in the discovery dataset. The survival analysis results for the three datasets were visualized by Kaplan-Meier plots.
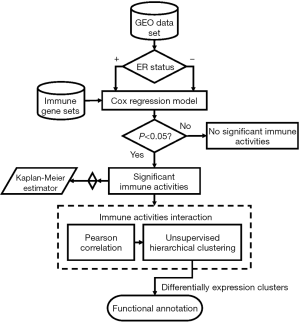
Further investigation of the immunological interactions between each survival associated immune cell gene sets was performed by Pearson correlation on the enrichment scores. Gene sets that showed strong interactions were grouped. Based on the enrichment scores of these gene sets we performed the unsupervised hierarchical clustering with respect to samples. Two clusters were chosen for subsequent analysis. One of them was patients with better survival and the other was the opposite. Finally, GSEA software was executed to provide functional interpretations of these two clusters.
Predictive immune signatures for breast cancer prognosis
To identify the activities of immune cell subtypes that may affect the clinical outcome of breast cancer patients, enrichment score of each gene set was calculated for each sample and was then applied to univariate Cox regression model. The yielded hazard ratios and P values are listed in Table 1. Nine of the 31 immune cell gene sets were found with statistical significance (P<0.05) in the ER+ group while only one of them in the ER− group. For the ER+ group, the significant gene sets were assigned to two groups, the protective and the risk group, according to the hazard ratio. The risk group included four gene sets: activated CD8, activated CD4, effector memory CD4, and NK56 dim, and the protective group contained five gene sets: mast cells, Th2, Treg, eosinophil, and DC. Among the nine gene sets, the activated CD8, activated CD4, effector memory CD4, Th2 and Treg gene set were related to adaptive immune system, and the NK56 dim, mast cells, eosinophil, and DC gene sets are associated with innate immune system. This implied that certain immunological signaling pathways and the cooperation of the innate and adaptive immune systems may affect the prognosis of the breast cancer patient. Previous studies also showed some relation between immune system and cancer microenvironment (29,30). Although the mechanisms underlying the result were still unclear, this may be an interesting topic for future research.
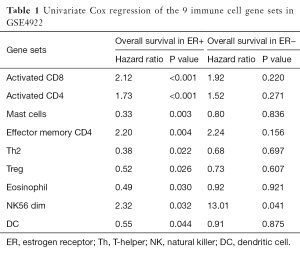
Full table
Validation for the identified predictive TIL subtypes
We validated the findings in the discovery dataset in two validation data sets (Table S2). Eight and five out of the nine gene sets showed concordant significance in the validation data sets, GSE2034 and GSE2990. This demonstrates the potential importance of the immune activities to ER+ breast cancer patients’ survival. Kaplan-Meier plot of the three most significant gene sets are activated CD4 (Cox P value <0.001), activated CD8 (Cox P value <0.001), and mast cell (Cox P value =0.003) (Figure 2). All of them had associations with patient survival in the ER+ group across the three datasets. The Cox P value of the CD4, CD8 and Mast cell gene set were P<0.001, P=0.002, and P=0.019 for GSE2034; P<0.001, P=0.003, and P=0.025 for GSE2990, respectively. Breast cancer patients with higher expression in both activated CD4 and activated CD8 gene sets had poor survival while upregulated mast cell gene set was associated with better prognosis. The result was in consistence with the earlier study which suggested the correlation between mast cell activities and lower grade tumors in stromal cells (31,32).
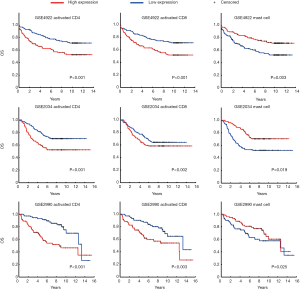
Multi-variate Cox analysis with other prognostic features
In addition to univariate Cox regression model for immune-related gene sets, we explored the potential to cooperate the immune cell gene sets with other well-known prognostic features. One prognostic features used in clinic (LN status) and two factors identified in the previous studies (cell cycle gene signature and ER+ consensus prognostic genes) were considered. The results showed that two tumor infiltration leukocytes can be an independent predictor comparing with the prognostic features (Table S3). Activated CD8 and mast cell signatures showed the statistical significance (P=0.03 and P=0.04), comparing with the variable of “lymph node status” which was one of the factors of current staging system used in clinic.
Construction the TIL interaction network
We assessed connectivity of the 7 out of 9 immune cell gene sets identified from ER+ group by Pearson correlation. The results are shown in Figure 3. In the ER+ group, two groups of gene sets showed strong correlations. One group contains effector memory CD4, activated CD4 and activated CD8 gene set while the other group includes mast cells, Treg, eosinophil and DC gene set. In the ER
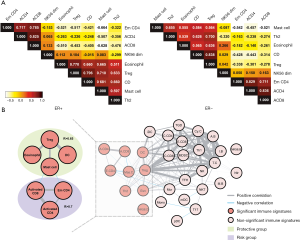
Seven identified immune cell gene sets were analyzed by two-way unsupervised hierarchical clustering for the purpose of testing the predictive power of these gene sets. The clustering result is shown in Figure 4. There were apparently two clusters in the dendrogram. On the left is the cluster of patients with poor survival, which tends to have higher expression in the three gene sets of risk group and lower expression in the four gene sets of protective group. On the other hand, on the right is the cluster of patients with better survival, and their expression in the seven gene sets is the opposite of the one on the left (higher expression in the protective group and lower expression in the risk group). Such result revealed the potential of these seven gene sets as predictive signature.
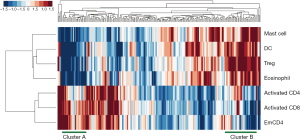
Functional relevance in the integrative subgroups
We conducted GSEA software to investigate the underlying mechanisms that affect the survival of the two clusters of patients. After computing the enrichment scores, we set the filtering threshold as FDR <0.001 and absolute enrichment score >0.6. A total of 27 gene sets were identified in cluster A (Table S4) and 51 gene sets were identified in cluster B (Table S5). Among these gene sets, several gene sets have been reported to be associated with breast cancer. We further interpreted two gene sets, “SHEN SMARCA2 TARGETS UP” and “SMID BREAST CANCER NORMAL LIKE UP” (Figure 5). In cluster A, “SHEN SMARCA2 TARGETS UP” is a set of genes whose expressions are positively correlated with the SMARCA2 gene. It has been reported that the SMARCA2 gene is associated with the transcription of certain genes related to poor survival of breast cancer patients (34,35). As for cluster B, an identified gene set, “SMID BREAST CANCER NORMAL LIKE UP”, has been reported to have differential expression in normal-like molecular subtypes of breast cancer (36).
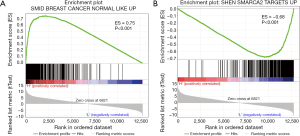
Discussion
In this study, we utilized the gene set approach to identify the activities of TILs subtypes in ER+ breast cancer. Nine of them were associated with patients’ survival. Nevertheless, only one of the nine immune cell gene sets showed correlation to prognosis in ER
Immune cells cooperate in immune response with a complicated mechanism; how to parse these immune subtypes networks in a specific cancer type cohort still remains a challenging task. We used Cox regression model and Kaplan-Meier estimator to investigate the prognosis trend with several TIL subtypes. Some of them are associated with adverse prognosis, such as regulatory T cells (37,38). However, several literatures suggested that Treg appear to play a dual role depending on tumor microenvironment (39), which can regulate the inflammation and trigger different signal pathways to oncogenesis, acting as antitumor suppressor or tumor suppressor. In this study, Treg accumulation led to favorable prognosis. With variety of immunosuppressive antigens, immune system signal transduction and cytokines secretion were interfered, causing immune dysfunction (40), that may be a reason of Treg diversity. The activated CD4 and CD8 immune signatures, analogous to regulatory T cell, were not expected in survival analysis. We inferred that this was caused by the divergence in CD4 and CD8 expression on the T cells in different clinical variation. Data source of gene collection was multi-faceted, which contained various clinical conditions. Breast cancer cohorts may have specificity of these two gene sets but with different prognosis expectancy.
In the survival analysis, we demonstrated some of the TILs subtypes were associated with overall survival, inclusive of activated CD8 and mast cells. TILs resulting in favorable and poor prognosis were different from the literature of immune subtype gene sets source. In Angelova’s research (18), few survival associated immune subtypes showed inverse trend to our study. For instance, regulatory T cell was a poor prognostic factor among TILs subtypes and also an independent survival predictor, but that was not found in our study, reflecting the molecular subtype diversities in cancer development and patient heterogeneity. Here, we focus subsequently analysis on ER+ cohort. Based on the concordant results achieved in two validation data sets, we confirmed the TILs and patient’s outcome modulated by ER status.
We pooled nine survival correlated immune cell gene sets to evaluate the independence of prognostic predictors. Cell cycle and LN status were known to predict patient survival. In our multivariate analysis LN status remained a dominant factor among all predictors in other survival associated TIL subtypes, overtaking the cell cycle genes. Thus, we can take activated CD8, mast cell and LN status as a group of powerful prognostic predictors in ER+ patients.
Functional annotations suggested that differentially activated immune signatures play important roles on other molecular signatures upon breast cancer oncogenesis. In large retrospective studies, mast cell was differentially correlated to prognostic in various cancer types (18,41,42). It was reported with the capability of defense against allergic pathogens. We analyzed biological functions associated with two clusters of protective immune signatures expression. Because in protective immune signatures, mast cell was a significant prognostic factor in multivariate Cox regression, we further investigated the mast cell functioning as principle on breast tumor. In two clusters of functional annotation, there appeared inverse regulation of a gene set modulate SMARCA2 in prostate cancer (43). Upregulation of SMARCA2 genes was seen in low expression of protective immune signatures, and downregulation of SMARCA2 genes in high expression of protective immune activities. SMARCA2 encodes the SWI/SNF chromatin remodeling complex which is an effector of DNA repair transcription. It has been demonstrated a functional association between BRCA1 and the SWI/SNF-related complex; with their combination and participation in transcriptional regulatory mechanism, mutation occurring in any or both of them can be a potential factor causing breast cancers (44). Among lower enrichment score annotated functions (ES =−0.58), low expression of protective group showed connectivity with the genes down regulated in the brain relapse of breast cancer (36). Based on the findings of this study, future works may build up more accurate predicting criteria and clear modulation network, contributing a genetic framework to promote immunotherapy.
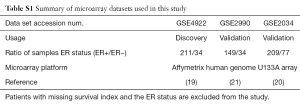
Full table
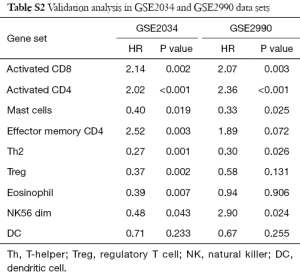
Full table
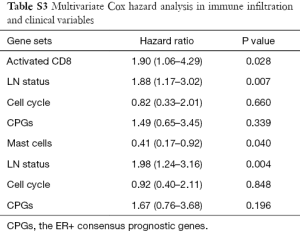
Full table
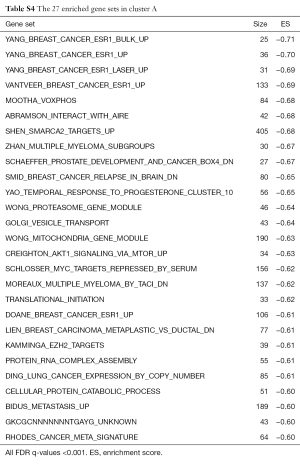
Full table
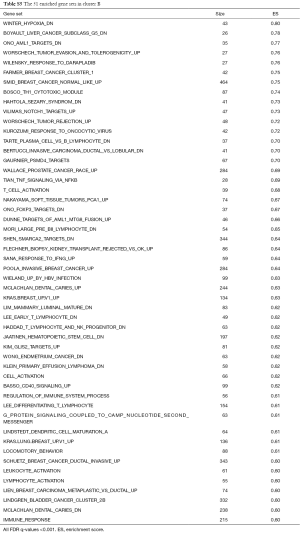
Full table
Acknowledgments
Funding: This work was supported by the Taichung Veterans General Hospital (TCVGH-YM1040205, TCVGH-YM1050204, and TCVGH-VTA105A1-2).
Footnote
Conflicts of Interest: All authors have completed the ICMJE uniform disclosure form (available at http://dx.doi.org/10.21037/tcr.2016.04.09). Eric Y. Chuang serves as the Editor-in-Chief of Translational Cancer Research. The other author has no conflicts of interest to declare.
Ethical Statement: The authors are accountable for all aspects of the work in ensuring that questions related to the accuracy or integrity of any part of the work are appropriately investigated and resolved. The study was conducted in accordance with the Declaration of Helsinki (as revised in 2013).
Open Access Statement: This is an Open Access article distributed in accordance with the Creative Commons Attribution-NonCommercial-NoDerivs 4.0 International License (CC BY-NC-ND 4.0), which permits the non-commercial replication and distribution of the article with the strict proviso that no changes or edits are made and the original work is properly cited (including links to both the formal publication through the relevant DOI and the license). See: https://creativecommons.org/licenses/by-nc-nd/4.0/.
References
- Siegel RL, Miller KD, Jemal A. Cancer statistics, 2015. CA 2015;65:5-29. [PubMed]
- Perou CM, Sorlie T, Eisen MB, et al. Molecular portraits of human breast tumours. Nature 2000;406:747-52. [Crossref] [PubMed]
- Malhotra GK, Zhao X, Band H, et al. Histological, molecular and functional subtypes of breast cancers. Cancer Biol Ther 2010;10:955-60. [Crossref] [PubMed]
- Masood S. Estrogen and progesterone receptors in cytology: a comprehensive review. Diagn Cytopathol 1992;8:475-91. [Crossref] [PubMed]
- Mohibi S, Mirza S, Band H, et al. Mouse models of estrogen receptor-positive breast cancer. J Carcinog 2011;10:35. [Crossref] [PubMed]
- Trichopoulos D, MacMahon B, Cole P. Menopause and breast cancer risk. J Natl Cancer Inst 1972;48:605-13. [PubMed]
- Fisher B, Costantino JP, Wickerham DL, et al. Tamoxifen for prevention of breast cancer: report of the National Surgical Adjuvant Breast and Bowel Project P1 Study. J Natl Cancer Inst 1998;90:1371-88. [Crossref] [PubMed]
- Loi S, Michiels S, Salgado R, et al. Tumor infiltrating lymphocytes are prognostic in triple negative breast cancer and predictive for trastuzumab benefit in early breast cancer: results from the FinHER trial. Ann Oncol 2014;25:1544-50. [Crossref] [PubMed]
- Carbognin L, Pilotto S, Nortilli R, et al. Predictive and Prognostic Role of Tumor-Infiltrating Lymphocytes for Early Breast Cancer According to Disease Subtypes: Sensitivity Analysis of Randomized Trials in Adjuvant and Neoadjuvant Setting. Oncologist 2016;21:283-91. [Crossref] [PubMed]
- Adams S, Gray RJ, Demaria S, et al. Prognostic value of tumor-infiltrating lymphocytes in triple-negative breast cancers from two phase III randomized adjuvant breast cancer trials: ECOG 2197 and ECOG 1199. J Clin Oncol 2014;32:2959-66. [Crossref] [PubMed]
- Ibrahim EM, Al-Foheidi ME, Al-Mansour MM, et al. The prognostic value of tumor-infiltrating lymphocytes in triple-negative breast cancer: a meta-analysis. Breast Cancer Res Treat 2014;148:467-76. [Crossref] [PubMed]
- Pruneri G, Vingiani A, Bagnardi V, et al. Clinical validity of tumor-infiltrating lymphocytes analysis in patients with triple-negative breast cancer. Ann Oncol 2016;27:249-56. [Crossref] [PubMed]
- Ansell SM, Lesokhin AM, Borrello I, et al. PD-1 blockade with nivolumab in relapsed or refractory Hodgkin's lymphoma. N Engl J Med 2015;372:311-9. [Crossref] [PubMed]
- Topalian SL, Hodi FS, Brahmer JR, et al. Safety, activity, and immune correlates of anti-PD-1 antibody in cancer. N Engl J Med 2012;366:2443-54. [Crossref] [PubMed]
- Brahmer JR, Tykodi SS, Chow LQ, et al. Safety and activity of anti-PD-L1 antibody in patients with advanced cancer. N Engl J Med 2012;366:2455-65. [Crossref] [PubMed]
- de Visser KE, Eichten A, Coussens LM. Paradoxical roles of the immune system during cancer development. Nat Rev Cancer 2006;6:24-37. [Crossref] [PubMed]
- DeNardo DG, Coussens LM. Inflammation and breast cancer. Balancing immune response: crosstalk between adaptive and innate immune cells during breast cancer progression. Breast Cancer Res 2007;9:212. [Crossref] [PubMed]
- Angelova M, Charoentong P, Hackl H, et al. Characterization of the immunophenotypes and antigenomes of colorectal cancers reveals distinct tumor escape mechanisms and novel targets for immunotherapy. Genome Biol 2015;16:64. [Crossref] [PubMed]
- Ivshina AV, George J, Senko O, et al. Genetic reclassification of histologic grade delineates new clinical subtypes of breast cancer. Cancer Res 2006;66:10292-301. [Crossref] [PubMed]
- Wang Y, Klijn JG, Zhang Y, et al. Gene-expression profiles to predict distant metastasis of lymph-node-negative primary breast cancer. Lancet 2005;365:671-9. [Crossref] [PubMed]
- Sotiriou C, Wirapati P, Loi S, et al. Gene expression profiling in breast cancer: understanding the molecular basis of histologic grade to improve prognosis. J Natl Cancer Inst 2006;98:262-72. [Crossref] [PubMed]
- Edgar R, Domrachev M, Lash AE. Gene Expression Omnibus: NCBI gene expression and hybridization array data repository. Nucleic Acids Res 2002;30:207-10. [Crossref] [PubMed]
- Kaplan EL, Meier P. Nonparametric-Estimation from Incomplete Observations. J Am Stat Assoc 1958;53:457-81. [Crossref]
- Cox DR. Regression Models and Life-Tables. Journal of the Royal Statistical Society. Series B (Methodological) 1972;34:187-220.
- Mizuno H, Nakanishi Y, Ishii N, et al. A signature-based method for indexing cell cycle phase distribution from microarray profiles. BMC Genomics 2009;10:137. [Crossref] [PubMed]
- Teschendorff AE, Naderi A, Barbosa-Morais NL, et al. A consensus prognostic gene expression classifier for ER positive breast cancer. Genome Biol 2006;7:R101. [Crossref] [PubMed]
- Subramanian A, Tamayo P, Mootha VK, et al. Gene set enrichment analysis: A knowledge-based approach for interpreting genome-wide expression profiles. Proc Natl Acad Sci U S A 2005;102:15545-50. [Crossref] [PubMed]
- Liberzon A, Subramanian A, Pinchback R, et al. Molecular signatures database (MSigDB) 3.0. Bioinformatics 2011;27:1739-40. [Crossref] [PubMed]
- Gajewski TF, Schreiber H, Fu YX. Innate and adaptive immune cells in the tumor microenvironment. Nat Immunol 2013;14:1014-22. [Crossref] [PubMed]
- Vesely MD, Kershaw MH, Schreiber RD, et al. Natural innate and adaptive immunity to cancer. Annu Rev Immunol 2011;29:235-71. [Crossref] [PubMed]
- Dabiri S, Huntsman D, Makretsov N, et al. The presence of stromal mast cells identifies a subset of invasive breast cancers with a favorable prognosis. Mod Pathol 2004;17:690-5. [Crossref] [PubMed]
- Rajput AB, Turbin DA, Cheang MC, et al. Stromal mast cells in invasive breast cancer are a marker of favourable prognosis: a study of 4,444 cases. Breast Cancer Res Treat 2008;107:249-57. [Crossref] [PubMed]
- Spörri R, Reis e Sousa C. Inflammatory mediators are insufficient for full dendritic cell activation and promote expansion of CD4+ T cell populations lacking helper function. Nat Immunol 2005;6:163-70. [Crossref] [PubMed]
- Bertucci F, Nasser V, Granjeaud S, et al. Gene expression profiles of poor-prognosis primary breast cancer correlate with survival. Hum Mol Genet 2002;11:863. [Crossref] [PubMed]
- Wilson BG, Roberts CW. SWI/SNF nucleosome remodellers and cancer. Nat Rev Cancer 2011;11:481-92. [Crossref] [PubMed]
- Smid M, Wang Y, Zhang Y, et al. Subtypes of breast cancer show preferential site of relapse. Cancer Res 2008;68:3108-14. [Crossref] [PubMed]
- Liu F, Lang R, Zhao J, et al. CD8+ cytotoxic T cell and FOXP3+ regulatory T cell infiltration in relation to breast cancer survival and molecular subtypes. Breast Cancer Res Treat 2011;130:645-55. [Crossref] [PubMed]
- Bates GJ, Fox SB, Han C, et al. Quantification of regulatory T cells enables the identification of high-risk breast cancer patients and those at risk of late relapse. J Clin Oncol 2006;24:5373-80. [Crossref] [PubMed]
- Whiteside TL. What are regulatory T cells (Treg) regulating in cancer and why? Semin Cancer Biol 2012;22:327-34. [Crossref] [PubMed]
- Ferrone S, Whiteside TL. Tumor microenvironment and immune escape. Surg Oncol Clin N Am 2007;16:755-74. viii. [Crossref] [PubMed]
- Conti P, Castellani ML, Kempuraj D, et al. Role of mast cells in tumor growth. Ann Clin Lab Sci 2007;37:315-22. [PubMed]
- Imada A, Shijubo N, Kojima H, et al. Mast cells correlate with angiogenesis and poor outcome in stage I lung adenocarcinoma. Eur Respir J 2000;15:1087-93. [Crossref] [PubMed]
- Shen H, Powers N, Saini N, et al. The SWI/SNF ATPase Brm is a gatekeeper of proliferative control in prostate cancer. Cancer Res 2008;68:10154-62. [Crossref] [PubMed]
- Bochar DA, Wang L, Beniya H, et al. BRCA1 Is Associated with a Human SWI/SNF-Related Complex: Linking Chromatin Remodeling to Breast Cancer. Cell 2000;102:257-65. [Crossref] [PubMed]