High expression of RTN4IP1 predicts adverse prognosis for patients with breast cancer
Highlight box
Key findings
• RTN4IP1 is over-expressed in breast cancer tissue, and predicts adverse prognosis for patients with breast cancer, especially in infiltrating ductal carcinoma, infiltrating lobular carcinoma, Stage II, Stages III&IV and luminal A subtype.
What is known and what is new?
• RTN4IP1 mutation was reported to cause deficits in mitochondrial respiratory complex I and IV activities, which drives cellular oxygen consumption.
• RTN4IP1 might involve in glutamine metabolism and mitoribosome-associated quality control. High expression of RTN4IP1 predicts adverse prognosis for patients with breast cancer.
What is the implication, and what should change now?
• Mitochondria's malfunction might play an important role in the genesis and development of breast cancer. Co-localized with mitochondrial ATPase protein, RTN4IP1 might bring new shed into breast cancer.
Introduction
As one of the most prevalent cancers, breast cancer (BC) alone accounts for almost 30% of all female cancers globally (1). There were estimated 2,261,419 new cases and 684,996 deaths worldwide in 2020 (2). Molecularly, BC is a heterogeneous disease, including different human epidermal growth factor receptor 2 (HER2) status, estrogen receptor (ER) status, progesterone receptor (PR) status and BRCA mutations (3). One of the most prominent advances in BC research is the molecular categorization based on gene expression profiles, which classifies BC into four major intrinsic molecular subtypes (4,5). However, it remains the leading cause of cancer-related deaths in women (1). Even with optimal management, about 10% of BC patients would loco-regionally relapse with concomitant or hetero-chronic metastasis (6). So we need to understand BC’s molecular players better and further dissect the regulatory mechanisms, so as to develop more delicate prognostic models.
The RTN4IP1 gene is also known as NOGO-Interacting Mitochondrial Protein (NIMP) (7) and optic atrophy-10 (OPA10) (8). It’s located on chromosome 6q21, a chromosomal region frequently deleted without or with loss of heterozygosity in a variety of human malignancies. RTN4IP1 is evolutionarily conserved among vertebrates and ubiquitously expressed in mitochondria-enriched tissues, such as skin, placenta and 24 other tissues. As its name indicated, RTN4IP1 interacts with reticulon-4 (RTN4), a membranous protein of endoplasmic reticulum. RTN is a potent inhibitor of regeneration following spinal cord injury. This interaction may be important for RTN-induced inhibition of neurite growth. Mutations in this gene can cause optic atrophy, cognitive disability and seizures (9).
But the role of RTN4IP1 in tumor is mainly masked. All we can find in PubMed is that RTN4IP1 is up-regulated in BC patients with visceral organ metastasis (10). Our initial analysis demonstrated that the expression of RTN4IP1 increased in various types of cancer. So in this study, the expressions of RTN4IP1 of BC with relevant clinic data were downloaded to investigate the significance of RTN4IP1 in the prognosis of BC. We present the following article in accordance with the TRIPOD reporting checklist (available at https://tcr.amegroups.com/article/view/10.21037/tcr-22-2350/rc).
Methods
Cohort from The Cancer Genome Atlas (TCGA) and Genotype-Tissue Expression (GTEx) datasets
RNAseq data with corresponding clinical information of The Cancer Genome Atlas Breast Invasive Carcinoma (TCGA-BRCA) project were extracted from the Genomic Data Commons (https://portal.gdc.cancer.gov/). Then the initial level 3 HTSeq-FPKM format were pre-processed into transcripts per million reads (TPM) by Toil (a portable open-source workflow software) (11), with several unavailable data estimated as missing values. Given that there are far less normal samples than cancerous ones in TCGA, RNAseq data (TPM) pre-processed by Toil from GTEx databank via UCSC XENA (https://xenabrowser.net/datapages/) were retrieved too. All qualified cases were dichotomized into RTN4IP1high and RTN4IP1low groups by the median expression level. And the expression levels between cancerous and adjacent tissues (or normal tissues) were compared by Wilcoxon rank sum test or Wilcoxon signed rank test. Considering this study was in total accordance with the publication guidelines of TCGA, no additional ethics approval was required. The study was conducted in accordance with the Declaration of Helsinki (as revised in 2013).
Differentially expressed genes (DEGs) and Functional Enrichment between RTN4IP1high and RTN4IP1low groups
Expression profiles (HTSeq-Counts) were investigated between RTN4IP1high and RTN4IP1low groups to screen DEGs via the DESeq2 R package [|log fold change (FC)| >1.5, adjusted P value <0.05] (12). DEGs were visualize with volcano plots and heatmaps via the ggplot 2 R package (version 3.1.0, http://github.com/tidyverse/ggplot2).
Enrichment analysis of Gene Ontology (GO) and Kyoto Encyclopedia of Genes and Genomes (KEGG) of DEGs between RTN4IP1high and RTN4IP1low groups were performed via the ClusterProfiler R package (v 3.12.0). Terms with P<0.01, minimum count >3, enrichment fact >1.5 were taken as statistically significant (13). Bubble charts were utilized to visualize top enriched terms of molecular function (MF), biological process (BP), cellular component (CC) and KEGG pathways.
Based on the gene coexpression network and matrices of expressed genes in TCGA-BRCA project, gene set enrichment analysis (GSEA) between RTN4IP1high and RTN4IP1low groups were processed via the cluster Profiler R package (13), with c2.cp.v7.0.symbols of MSigDB Collections as reference gene sets, where adjusted P value <0.05 and false discovery rate (FDR) <0.25 were taken as significant enrichment) (14).
Immune infiltration analysis by single-sample GSEA (ssGSEA)
ssGSEA were applied by GSVA R package. Relative tumor infiltration levels of 24 immune cell types were quantified by interrogating expression levels of genes in published signature gene lists, which comprised a diverse set of adaptive and innate immune cell types and contained 509 genes in total (15). Infiltration levels were compared between RTN4IP1high and RTN4IP1low groups, and followed by Spearman correlation analysis for the correlation between RTN4IP1 and 24 types of immune cells.
Protein-protein interaction (PPI) networks
The Search Tool for the Retrieval of Interacting Genes (STRING) public database (http://string-db.org, version 11.0) were employed to get insight of potential PPI networks (16), where the PPI threshold of DEGs correlation coefficient was 0.4, and visualized by the open software Cytoscape (Version 3.8.0).
Statistical analysis
R (v3.6.3) was used for all statistical analysis and plots. In all tests, P<0.05 was considered statistically significant. Wilcoxon rank sum test and Wilcoxon signed rank test were used in comparisons of RTN4IP1 expression levels between cancerous tissues and normal (or adjacent) tissues (non-paired samples and paired samples, respectively).
The correlations between clinicopathologic characters and RTN4IP1 were tested using Wilcoxon rank sum test (Kruskal-Wallis test, if there were more than two groups). Using pROC package (17), receiver operating characteristic (ROC) analysis was drawn to evaluate the accuracy of the expression of RTN4IP1 to discriminate BC tissues from non-cancerous ones. The logistic regression was conducted for the correlations between RTN4IP1 expression level (TPM) and clinicopathologic features.
Prognostic model
Based on the survival data of Liu et al.’s study (18), Kaplan-Meier curves by Survminer R package were drawn to depict disease-specific survival (DSS) and overall survival (OS), differences between curves were tested by log-rank test. DSS was counted from diagnosis of BC to death or final follow-up, excluding those who died from causes other than BC.
Univariate COX proportional hazards regression calculated the hazard ratio (HR) for DSS and OS, then the significant variables (P<0.1) were engaged in multivariate analysis. Further study of subgroups’ survival was also conducted.
To visualize the survival probability of 1, 3 and 5 years, nomograms by rms R package were generated from the COX analysis. Calibration curves mapped the prediction plots against the observed dots, with the 45° line representing ideal prediction. C-index (concordance) was calculated by a bootstrap approach with 1,000 samples.
Results
RTN4IP1 expression correlated with some clinicopathologic parameters
Altogether, 1,065 cases of RNAseq data with corresponding clinical information were extracted from TCGA-BRCA project. Given that there are far less normal samples than cancerous ones in TCGA, we downloaded RNAseq data (TPM) from both TCGA and GTEx databank from UCSC XENA (https://xenabrowser.net/datapages/), which were pre-processed by Toil, a portable open-source workflow software (11).
Pathologic stage (P=0.012), ER status (P<0.001), PR status (P<0.001), HER2 status (P<0.001), prediction analysis of microarray 50 (PAM50) (P<0.001), histological type (P<0.001), race (P=0.023) and TP53 status (P<0.001) were statistically significantly associated with RTN4IP1 expression level, while neither T, N, M stages, PIK3CA status, nor age were differently distributed between low and high RTN4IP1 expression groups (shown in Table 1, Figure 1A-1H). According to the ROC curve of RTN4IP1, area under the curve (AUC) of 0.784 showed moderate potential to discriminate tumor from normal tissue (shown in Figure 1I).
Table 1
Characteristics | Level | RTN4IP1low (n=533) | RTN4IP1high (n=532) | P | Test |
---|---|---|---|---|---|
Histology, n (%) | Infiltrating ductal | 334 (72.1) | 423 (85.3) | <0.001 | |
Infiltrating lobular | 129 (27.9) | 73 (14.7) | |||
Race, n(%) | Asian | 32 (6.5) | 28 (5.8) | 0.023 | Exact |
Black | 74 (15.0) | 105 (21.8) | |||
White | 388 (78.5) | 349 (72.4) | |||
Age, years, n (%) | ≤60 | 304 (57.0) | 284 (53.4) | 0.256 | |
>60 | 229 (43.0) | 248 (46.6) | |||
T stage, n (%) | T1 | 150 (28.2) | 125 (23.5) | 0.143 | Exact |
T2 | 289 (54.4) | 326 (61.4) | |||
T3 | 74 (13.9) | 63 (11.9) | |||
T4 | 18 (3.4) | 17 (3.2) | |||
N stage, n (%) | N0 | 265 (50.5) | 242 (46.4) | 0.587 | Exact |
N1 | 171 (32.6) | 178 (34.2) | |||
N2 | 54 (10.3) | 62 (11.9) | |||
N3 | 35 (6.7) | 39 (7.5) | |||
M stage, n (%) | M0 | 452 (98.3) | 437 (97.3) | 0.373 | Exact |
M1 | 8 (1.7) | 12 (2.7) | |||
Pathologic stage, n (%) | Stage I | 108 (20.6) | 72 (13.9) | 0.012 | Exact |
Stage II | 288 (55.0) | 318 (61.4) | |||
Stage III | 122 (23.3) | 116 (22.4) | |||
Stage IV | 6 (1.1) | 12 (2.3) | |||
ER, n (%) | Negative | 73 (14.5) | 164 (32.1) | <0.001 | |
Positive | 431 (85.5) | 347 (67.9) | |||
PR, n (%) | Negative | 131 (26.0) | 207 (40.7) | <0.001 | |
Positive | 372 (74.0) | 302 (59.3) | |||
HER2, n (%) | Negative | 296 (83.9) | 252 (71.6) | <0.001 | |
Positive | 57 (16.1) | 100 (28.4) | |||
PAM50, n (%) | Basal | 56 (10.5) | 134 (25.2) | NA | Exact |
Her2 | 24 (4.5) | 58 (10.9) | |||
LumA | 329 (61.7) | 222 (41.7) | |||
LumB | 95 (17.8) | 107 (20.1) | |||
Normal | 29 (5.4) | 11 (2.1) | |||
TP53, n (%) | Mut | 109 (22.9) | 226 (47.0) | <0.001 | |
WT | 366 (77.1) | 255 (53.0) | |||
PIK3CA, n (%) | Mut | 163 (34.3) | 151 (31.4) | 0.372 | |
WT | 312 (65.7) | 330 (68.6) |
T, tumor; N, lymph node; M, metastasis; ER, estrogen receptor; PR, progesterone receptor; HER2, human epidermal growth factor receptor 2; PAM50, prediction analysis of microarray 50; TP53, tumor protein p53; PIK3CA, phosphatidylinositol-4,5-bisphosphate 3-kinase; NA, not applicable.
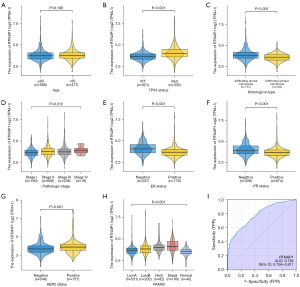
RTN4IP1 expression is upregulated in BC
Compared to para-tumor tissues, the expression of RTN4IP1 was significantly up-regulated in cancerous ones (P<0.001, shown in Figure 2A,2B). Also, the expression level of RTN4IP1 of BC samples was significantly higher than that of normal samples (P<0.001, shown in Figure 2C). By the same way, we downloaded TCGA pan-cancer data to show RTN4IP1 expression in various types of tumors, which showed that RTN4IP1 expression were significantly higher in most type of tumorous samples than in normal ones (P<0.05, shown in Figure 2D,2E).
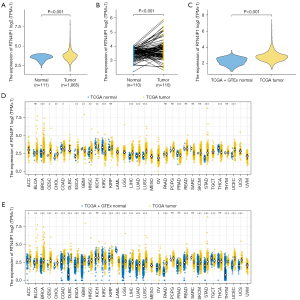
Identification of DEGs and functional enrichment of RTN4IP1
DESeq2 R package was used to identify DEGs between high and low RTN4IP1 expression groups (|log FC| >1.5, adjusted P value <0.01). A total of 771 DEGs were screened (201 upregulated and 570 down-regulated) and illustrated by volcano plot (shown in Figure 3A). Relative expression profiles of the topmost 20 DEGs between RTN4IP1high and RTN4IP1low groups, demonstrated in Figure 3B, were QRSL1, C6orf203, ATG5, CD24, HDAC2, COQ3, PREP, RPF2, CCNC, CD24P4, RP11-182I10.3, CHAD, RGS5, ATP1A2, C1orf132, EBF2, TPRG1, RP11-519C12.1, SCN7A, ANKRD29. GO enrichment items of DEGs, were to indicate latent functions of RTN4IP1. Topmost 9 ones of BP, CC, MF and KEGG pathways were displayed in Figure 3C-3F, respectively. Organelle fission, chromosome segregation, regulation of mitotic cell cycle phase transition, mitotic nuclear division and regulation of DNA metabolic process toped in BP; mitochondrial matrix, chromosome, centromeric region, spindle, mitochondrial inner membrane, microtubule, kinetochore toped in CC; ATPase activity, catalytic activity acting on RNA and DNA, ubiquitin-like protein ligase binding, ligase and helicase activity toped in MF; cell cycle, amyotrophic lateral sclerosis, Huntington disease, Alzheimer disease, Parkinson disease, Prion disease, cellular senescence, RNA transport and Oocyte meiosis toped KEGG pathways.
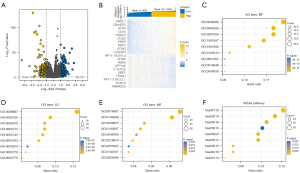
To identify related signaling pathways, GSEA between RTN4IP1high and RTN4IP1low groups unmasked 1,155 data sets of significant differences in enrichment of MSigDB collections. For instance, RTN4IP1 was associated with G1_S DNA damage checkpoints, ESR1 upregulation, ERBB2 upregulation, difference between invasive ductal carcinoma and lobular carcinoma, luminal B subtype, grade escalating, drug resistance and metastasis (shown in Figure 4).
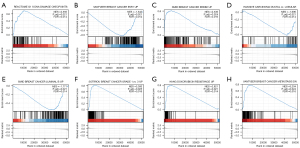
The correlation between RTN4IP1 expression and Immune infiltration
Infiltration of eosinophils, natural killer (NK) cells and plasma dendritic cells (pDCs) are lower in RTN4IP1high group, while Infiltration of Th2 cells represents the otherwise (shown in Figure S1). Spearman correlation was applied for the association between the expression levels (TPM) of RTN4IP1 and immune infiltration levels quantified by ssGSEA in the tumor microenvironment (shown in Figure 5). Eosinophil cells, NK cells and Th2 cells were found to be slightly correlated with RTN4IP1 expression (R=−0.290, −0.277, and 0.266, respectively, P<0.001).
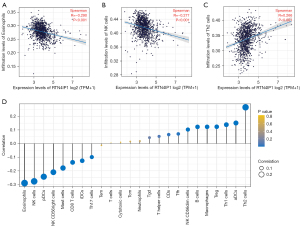
PPI networks
To further discover underlying interaction networks between associated proteins, PPI network was depicted in Figure S2, showing interactions between RTN4IP1 and QRSL1, SEC63, BEND3 and C6orf203.
Logistic regression between RTN4IP1 expression and clinicopathologic parameters
The logistic regression showed that the correlations between RTN4IP1 expression level (TPM) and PR status (P=0.003), ER status (P=0.003), HER2 status (P=0.003), histological type (P<0.001), TP53 status (P<0.001) are all statistically significant (shown in Table 2).
Table 2
Characteristics | OR in RTN4IP1 expression | OR (95% CI) | P value |
---|---|---|---|
T stage (T3&4 vs. T1&2) | 1,062 | 1.00 (1.00–1.01) | 0.318 |
N stage (N1&2&3 vs. N0) | 1,046 | 1.01 (1.00–1.02) | 0.105 |
M stage (M1 vs. M0) | 909 | 0.99 (0.94–1.01) | 0.771 |
Pathologic stage (Stages III&IV vs. Stage I&II) | 1,042 | 1.00 (1.00–1.01) | 0.179 |
PR status (positive vs. negative) | 1,012 | 0.98 (0.97–0.99) | 0.003 |
ER status (positive vs. negative) | 1,015 | 0.99 (0.98–0.99) | 0.003 |
HER2 status (positive vs. negative) | 705 | 1.02 (1.01–1.03) | 0.003 |
Histology (lobular vs. ductal) | 959 | 0.91 (0.88–0.94) | <0.001 |
TP53 status (Mut vs. WT) | 956 | 1.06 (1.04–1.08) | <0.001 |
PIK3CA status (Mut vs. WT) | 956 | 1.00 (0.99–1.01) | 0.584 |
OR, odds ratio; T, tumor; N, lymph node; M, metastasis; PR, progesterone receptor; ER, estrogen receptor; HER2, human epidermal growth factor receptor 2; TP53, tumor protein p53; PIK3CA, phosphatidylinositol-4,5-bisphosphate 3-kinase.
RTN4IP1 has independent prognostic value for BC patients
Kaplan-Meier plots revealed the prognostic value of RTN4IP1 in infiltrative BC patients, which indicated that RTN4IP1high BC had a worse DSS than RTN4IP1low ones [HR =2.37; 95% confidential interval (CI): (1.48–3.78), P<0.001, shown in Figure 6A]. Univariate analyses of DSS indicated that PR status (P=0.006), ER status (P=0.007), histological type (P=0.065), M stage (P<0.001), T stage (P=0.004), N stage (P<0.001), RTN4IP1 (P<0.001) were qualified (P<0.1) for COX multivariate analyses of survival. Then M stage (P=0.008), N stage (P<0.001), and RTN4IP1 (P=0.003) were found to be independent prognostic values (P<0.05, shown in Table 3), and integrated in the nomogram (shown in Figure 6B). The C-index was 0.703 (0.666–0.740). The bias-corrected line in calibration was close to the ideal line, indicating sufficient accuracy of prediction (shown in Figure 6C).
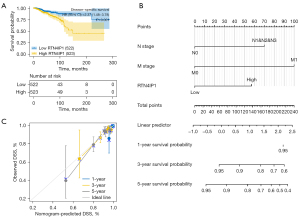
Table 3
Characteristics | Total (N) | Univariate | Multivariate | |||
---|---|---|---|---|---|---|
HR (95% CI) | P value | HR (95% CI) | P value | |||
Age (>60 vs. ≤60) | 1,045 | 1.418 (0.913–2.201) | 0.120 | |||
Race (White vs. Asian & Black) | 957 | 0.795 (0.478–1.322) | 0.377 | |||
Histology (ductal vs. lobular) | 941 | 2.002 (0.957–4.188) | 0.065 | 1.437 (0.535–3.860) | 0.472 | |
T stage (T1&2 vs. T3&4) | 1,042 | 0.491 (0.302–0.799) | 0.004 | 1.087 (0.500–2.366) | 0.833 | |
N stage (N0&1 vs. N2&3) | 1,027 | 0.382 (0.232–0.629) | <0.001 | 0.269 (0.143–0.507) | <0.001 | |
M stage (M0 vs. M1) | 891 | 0.130 (0.069–0.243) | <0.001 | 0.303 (0.126–0.728) | 0.008 | |
PR (positive vs. negative) | 993 | 0.529 (0.336–0.833) | 0.006 | 0.786 (0.347–1.781) | 0.564 | |
ER (positive vs. negative) | 996 | 0.523 (0.326–0.838) | 0.007 | 0.646 (0.273–1.529) | 0.320 | |
HER2 (positive vs. negative) | 695 | 1.481 (0.740–2.965) | 0.267 | |||
TP53 (Mut vs. WT) | 936 | 1.481 (0.925–2.371) | 0.102 | |||
PIK3CA (Mut vs. WT) | 936 | 0.885 (0.526–1.489) | 0.646 | |||
RTN4IP1 (high vs. low) | 1,045 | 2.369 (1.484–3.784) | <0.001 | 2.608 (1.374–4.950) | 0.003 |
HR, hazard ratio; CI, confidential interval; T, tumor; N, lymph node; M, metastasis; PR, progesterone receptor; ER, estrogen receptor; HER2, human epidermal growth factor receptor 2; TP53, tumor protein p53; PIK3CA, phosphatidylinositol-4,5-bisphosphate 3-kinase.
In subgroup analysis, the prognostic value of RTN4IP1 in TCGA-BRCA DSS was visualized in forest plots (shown in Figure 7). It’s statistically significant in infiltrating ductal carcinoma [HR =2.704 (1.506–4.855), P<0.001], infiltrating lobular carcinoma [HR =7.978 (1.607–39.609), P=0.011], Stage II [HR =3.444 (1.481–8.012), P=0.004], Stages III&IV [HR =2.212 (1.136–4.308), P=0.020], luminal A subtype [HR =3.362 (1.616–6.995), P=0.001] and PIK3CA wild type (WT) [HR =2.600 (1.393–4.854), P=0.003].
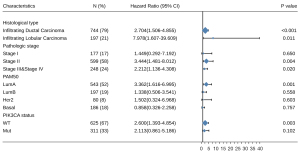
Discussion
The idea of precision oncology is based on the presumption that the knowledge of patient’s genomic basis would guide the targeted therapies. The survival of BC patients varies according to their molecular heterogeneity. Current molecular categorizations mainly base on hormonal receptors and growth factor receptors, such as ER, PR and HER2. Now five major subtypes can be distinguished based on the measurement of transcript levels of just 50 genes (PAM50) (19). Novel parameters from different aspects of cancer cells might bring new shed into BC. For instance, energy metabolism in mitochondria is inclined to glycolysis other than the citric acid cycle, regardless of oxygen supply (the Warburg effect) (20). So the mitochondria’s malfunction might play an important role in the genesis and development of BC.
RTN4 is largely localized in the endoplasmic reticulum. When cells are damaged, RTN4 interacts with the ubiquinol-cytochrome c reductase core protein (UQCRC) of mitochondrial respiratory chain (7), and then may be inappropriately guided to mitochondria and bound to specific proteins (RTN4IP1, UQCRC1 and UQCRC2), leading to mitochondrial malfunction. The RTN4IP1 was found to be co-localized with mitochondrial ATPase protein (9). Thus the cross-talk between RTN4IP1 at the surface of mitochondria and RTN4 from the endoplasmic reticulum may mediate the Warburg effect after cellular stress. Also, RTN4 has been implicated in cellular cycle regulation, apoptosis and migration. The mis-expression of RTN4IP1 in cancer cells is frequently detected in malignancies and specifically in comparisons of primary and metastatic/aggressive tumors (21). As mentioned above, RTN4IP1 was up-regulated in the metastatic BC cells (10).
Given that little is known about the expression of RTN4IP1 and its prognostic value in BRCA, bioinformatics analyses of TCGA RNA sequencing data were performed. Elevated RTN4IP1 expression in BRCA was found to be correlated to advanced clinical features and poor survival. Even though the AUC of RTN4IP1 in the ROC curve reached 0.784, RTN4IP1 is far from being qualified as a diagnostic marker yet.
Histologically, the outer layer is made of basal cells which are in direct contact with the basement membrane. The inner luminal layer is made of gland cells which are able to produce milk upon hormone induction. Compared to their counterparts, patients with negative ER status, negative PR status or positive HER2 status have higher RTN4IP1 expression, reinforced by that the basal subtype of PAM50 has the highest RTN4IP1 expression level. The triple negative BC are characterized by a basal-like transcriptional profile and frequent TP53 mutation (3). Compared to white or Asian people, African American have the highest expression level of RTN4IP1, whose functions is found out to be linked to mitochondrial physiology and response to ultraviolet (UV) light (8).
As plotted in the heatmap of Figure 3B, QRSL1’s expression is significantly correlated with RTN4IP1. Given that QRSL1 is a subunit of a glutamine amidotransferase GatCAB complex (22,23), this indicated that RTN4IP1 might involve in glutamine metabolism. Another import DEG in the heatmap is C6orf203, a putative human mitochondrial protein, is proposed to be a novel RNA-binding protein involved in mitochondrial translation, expanding the repertoire of factors engaged in this process (24). C6orf203 is also known as Mitochondrial Transcription Rescue Factor 1 (MTRES1), an example of a protein that protects the cell from mitochondrial RNA loss during stress (25), and activates mitoribosome-associated quality control (26). Connectivity among QRSL1, C6orf203 and RTN4IP1 is confirmed by PPI analysis.
Annotation of GO and KEGG showed us that RTN4IP1 high-expressed phenotype is enriched in regulation of DNA metabolic process, mitochondrial matrix and inner membrane, ATPase activity, cell cycle and cellular senescence; whereas GSEA told us that RTN4IP1 is involved in broad regulation of cellular cycle, G1_S DNA damage checkpoints, drug resistance and metastasis. All these bio-informatics shed light on the involvement of RTN4IP1 in breast carcinogenesis and development.
Flow cytometry has revealed that, compared to normal breast, immune infiltrates are higher in cancerous breast tissues (27). The interplay between immune cells known as tumor microenvironment, including the cytokines and chemokines they secrete, serve important roles in BC progression and anticancer treatment (28). For instance, cytotoxic CD8+ T cells, CD4+ Th cells, NK cells and DCs all assist in anti-cancer immune response, while regulatory T (Treg) cells are involved in suppressive immunity (29). Hence, another enrichment analysis was performed and found out that RTN4IP1 expression was associated with diverse immune infiltration levels in BRCA. There is moderate positive correlation between RTN4IP1 expression level and infiltration levels of eosinophils and NK cells, and moderate negative correlation between RTN4IP1 expression level and infiltration level of Th2. Eosinophils are primitive cells of innate immunity and play key roles in allergic diseases. Patients with low eosinophil counts in blood have increased recurrent risk compared to those with normal or high counts (30). These results revealed the potential regulating role of RTN4IP1 in BC microenvironment, and gave us clues into therapeutic manipulation to overcome drug resistance by enhancing metabolic potential of BC.
Back to clinical relevance, correlation between RTN4IP1 with clinicopathlogic parameters were confirmed by logistic regression, and its prognostic value was evaluated by univariate and multivariate COX regression. The Kaplan-Meier survival curve certified that high expression of RTN4IP1 predicted adverse prognosis for patients with BC. Then a simply-equipped nomogram provided an easy calculation of survival probability, which awaits to be tested in clinical usage.
Subgroup analysis revealed RTN4IP1’s prognostic value in infiltrating ductal carcinoma, infiltrating lobular carcinoma, Stage II, Stages III&IV and luminal A subtype, which agreed with our analysis of functional enrichment of RTN4IP1-related DEGs.
Last but not the least, due to the limitation of online bioinformatics, further validation of RTN4IP1’s prognostic value is warranted in order to test the reproducibility and the robustness of the correlations between the expression level and cellular behavior of breast carcinoma.
Conclusions
Overexpressed in BC tissue, RTN4IP1 might involve in glutamine metabolism and mitoribosome-associated quality control. High expression of RTN4IP1 predicts adverse prognosis for patients with BC, especially in infiltrating ductal carcinoma, infiltrating lobular carcinoma, Stage II, Stages III&IV and luminal A subtype.
Acknowledgments
Funding: None.
Footnote
Reporting Checklist: The authors have completed the TRIPOD reporting checklist. Available at https://tcr.amegroups.com/article/view/10.21037/tcr-22-2350/rc
Conflicts of Interest: All authors have completed the ICMJE uniform disclosure form (available at https://tcr.amegroups.com/article/view/10.21037/tcr-22-2350/coif). The authors have no conflicts of interest to declare.
Ethical Statement: The authors are accountable for all aspects of the work in ensuring that questions related to the accuracy or integrity of any part of the work are appropriately investigated and resolved. The study was conducted in accordance with the Declaration of Helsinki (as revised in 2013).
Open Access Statement: This is an Open Access article distributed in accordance with the Creative Commons Attribution-NonCommercial-NoDerivs 4.0 International License (CC BY-NC-ND 4.0), which permits the non-commercial replication and distribution of the article with the strict proviso that no changes or edits are made and the original work is properly cited (including links to both the formal publication through the relevant DOI and the license). See: https://creativecommons.org/licenses/by-nc-nd/4.0/.
References
- Siegel RL, Miller KD, Jemal A. Cancer statistics, 2020. CA Cancer J Clin 2020;70:7-30. [Crossref] [PubMed]
- Sung H, Ferlay J, Siegel RL, et al. Global Cancer Statistics 2020: GLOBOCAN Estimates of Incidence and Mortality Worldwide for 36 Cancers in 185 Countries. CA Cancer J Clin 2021;71:209-49. [Crossref] [PubMed]
- Harbeck N, Penault-Llorca F, Cortes J, et al. Breast cancer. Nat Rev Dis Primers 2019;5:66. [Crossref] [PubMed]
- Perou CM, Sørlie T, Eisen MB, et al. Molecular portraits of human breast tumours. Nature 2000;406:747-52. [Crossref] [PubMed]
- Stricker TP, Brown CD, Bandlamudi C, et al. Robust stratification of breast cancer subtypes using differential patterns of transcript isoform expression. PLoS Genet 2017;13:e1006589. [Crossref] [PubMed]
- Yates LR, Knappskog S, Wedge D, et al. Genomic Evolution of Breast Cancer Metastasis and Relapse. Cancer Cell 2017;32:169-184.e7. [Crossref] [PubMed]
- Hu WH, Hausmann ON, Yan MS, et al. Identification and characterization of a novel Nogo-interacting mitochondrial protein (NIMP). J Neurochem 2002;81:36-45. [Crossref] [PubMed]
- Charif M, Nasca A, Thompson K, et al. Neurologic Phenotypes Associated With Mutations in RTN4IP1 (OPA10) in Children and Young Adults. JAMA Neurol 2018;75:105-13. [Crossref] [PubMed]
- Angebault C, Guichet PO, Talmat-Amar Y, et al. Recessive Mutations in RTN4IP1 Cause Isolated and Syndromic Optic Neuropathies. Am J Hum Genet 2015;97:754-60. [Crossref] [PubMed]
- Savci-Heijink CD, Halfwerk H, Koster J, et al. A specific gene expression signature for visceral organ metastasis in breast cancer. BMC Cancer 2019;19:333. [Crossref] [PubMed]
- Vivian J, Rao AA, Nothaft FA, et al. Toil enables reproducible, open source, big biomedical data analyses. Nat Biotechnol 2017;35:314-6. [Crossref] [PubMed]
- Love MI, Huber W, Anders S. Moderated estimation of fold change and dispersion for RNA-seq data with DESeq2. Genome Biol 2014;15:550. [Crossref] [PubMed]
- Yu G, Wang LG, Han Y, et al. clusterProfiler: an R package for comparing biological themes among gene clusters. OMICS 2012;16:284-7. [Crossref] [PubMed]
- Subramanian A, Tamayo P, Mootha VK, et al. Gene set enrichment analysis: a knowledge-based approach for interpreting genome-wide expression profiles. Proc Natl Acad Sci U S A 2005;102:15545-50. [Crossref] [PubMed]
- Bindea G, Mlecnik B, Tosolini M, et al. Spatiotemporal dynamics of intratumoral immune cells reveal the immune landscape in human cancer. Immunity 2013;39:782-95. [Crossref] [PubMed]
- Szklarczyk D, Gable AL, Lyon D, et al. STRING v11: protein-protein association networks with increased coverage, supporting functional discovery in genome-wide experimental datasets. Nucleic Acids Res 2019;47:D607-13. [Crossref] [PubMed]
- Robin X, Turck N, Hainard A, et al. pROC: an open-source package for R and S+ to analyze and compare ROC curves. BMC Bioinformatics 2011;12:77. [Crossref] [PubMed]
- Liu J, Lichtenberg T, Hoadley KA, et al. An Integrated TCGA Pan-Cancer Clinical Data Resource to Drive High-Quality Survival Outcome Analytics. Cell 2018;173:400-416.e11. [Crossref] [PubMed]
- Chia SK, Bramwell VH, Tu D, et al. A 50-gene intrinsic subtype classifier for prognosis and prediction of benefit from adjuvant tamoxifen. Clin Cancer Res 2012;18:4465-72. [Crossref] [PubMed]
- Hanahan D, Weinberg RA. Hallmarks of cancer: the next generation. Cell 2011;144:646-74. [Crossref] [PubMed]
- Rahbari R, Kitano M, Zhang L, et al. RTN4IP1 is down-regulated in thyroid cancer and has tumor-suppressive function. J Clin Endocrinol Metab 2013;98:E446-54. [Crossref] [PubMed]
- Friederich MW, Timal S, Powell CA, et al. Pathogenic variants in glutamyl-tRNAGln amidotransferase subunits cause a lethal mitochondrial cardiomyopathy disorder. Nat Commun 2018;9:4065. [Crossref] [PubMed]
- Cai RJ, Su HW, Li YY, et al. Forward Genetics Reveals a gatC-gatA Fusion Polypeptide Causes Mistranslation and Rifampicin Tolerance in Mycobacterium smegmatis. Front Microbiol 2020;11:577756. [Crossref] [PubMed]
- Gopalakrishna S, Pearce SF, Dinan AM, et al. C6orf203 is an RNA-binding protein involved in mitochondrial protein synthesis. Nucleic Acids Res 2019;47:9386-99. [Crossref] [PubMed]
- Kotrys AV, Cysewski D, Czarnomska SD, et al. Quantitative proteomics revealed C6orf203/MTRES1 as a factor preventing stress-induced transcription deficiency in human mitochondria. Nucleic Acids Res 2019;47:7502-17. [Crossref] [PubMed]
- Desai N, Yang H, Chandrasekaran V, et al. Elongational stalling activates mitoribosome-associated quality control. Science 2020;370:1105-10. [Crossref] [PubMed]
- Gil Del Alcazar CR, Huh SJ, Ekram MB, et al. Immune Escape in Breast Cancer During In Situ to Invasive Carcinoma Transition. Cancer Discov 2017;7:1098-115. [Crossref] [PubMed]
- Thompson ED, Zahurak M, Murphy A, et al. Patterns of PD-L1 expression and CD8 T cell infiltration in gastric adenocarcinomas and associated immune stroma. Gut 2017;66:794-801. [Crossref] [PubMed]
- Luen S, Virassamy B, Savas P, et al. The genomic landscape of breast cancer and its interaction with host immunity. Breast 2016;29:241-50. [Crossref] [PubMed]
- Grisaru-Tal S, Itan M, Klion AD, et al. A new dawn for eosinophils in the tumour microenvironment. Nat Rev Cancer 2020;20:594-607. [Crossref] [PubMed]