Identification and experimental verification of an anoikis and immune related signature in prognosis for lung adenocarcinoma
Highlight box
Key findings
• An anoikis and immune related signature was constructed to predict the prognosis in lung adenocarcinoma.
What is known and what is new?
• The risk signature constructed by single gene set have some limitations.
• This risk signature facilitates further understanding of the tumor immune microenvironment, as anoikis and immune related genes are extremely associated with the tumor microenvironment.
What is the implication, and what should change now?
• It means that it is necessary to concern the variability of different individuals in the treatment of lung adenocarcinoma.
Introduction
Lung cancer, a common heterogeneous malignant tumor like other malignancies, is usually classified as small cell lung cancer and non-small cell lung cancer (adenocarcinoma and squamous carcinoma) (1-3). Among them, lung adenocarcinoma (LUAD) is the most common type of lung cancer pathology. In recent years, targeted therapy and immunotherapy play an important role in the treatment of LUAD as a result of the development of precision therapy.
Anoikis, as a form of programmed cell death, is characterized by the loss of cell adhesion to the extracellular matrix (ECM) or the improper adhesion resulting in rapid apoptosis of normal epithelial cells (4-6). In contrast to normal cells, tumor cells have to develop strong resistance to anoikis in order to keep cancer cells alive in metastasis and proliferation (7-10). Tumor cells can survive in an inappropriate ECM only after the tumor cells initiate the anti-anoikis feature. Several publications have reported that anoikis-related genes (ARGs) play major roles in tumor resistance and development (11,12).
The development of tumor cells is not only dependent on the accumulation of mutations in the tumor cells themselves, but also tightly linked to the ECM, immune cells and mesenchymal cells in the tumor microenvironment (13,14). Different types of immune infiltration may suggest different effects of immunotherapy (15). Therefore, understanding the various molecular changes in tumor immunity is crucial for targeted therapy and immunotherapy. Traditional treatment modalities, although being well-developed, still have significant limitations due to the individual variability of the oncology patients (16). Therefore, there is a need to develop more accurate risk assessment signatures to provide more precise clinical interventions for different patients. In recent years, the explosion of sequencing data and bioinformatics have opened up the possibility of precision therapy. More and more predictive signatures are being developed to provide ideas for clinical immunotherapy and targeted therapies (17-19).
Although experimental studies of ARGs and immune-related genes (IRGs) are gradually gaining more attention in recent years, studies using these genes as prognostic factors to construct risk signature have not yet emerged (20,21). Therefore, we integrated anoikis and immune related genes (AIRGs) to construct a reliable and clinically meaningful risk-prognostic signature through effective bioinformatics tools. We present the following article in accordance with the TRIPOD reporting checklist (available at https://tcr.amegroups.com/article/view/10.21037/tcr-22-2550/rc).
Methods
Data acquisition and analysis
Data and clinical information of LUAD patients were taken from The Cancer Genome Atlas (TCGA) Program and the GSE68465 from the Gene Expression Omnibus (GEO) (22,23). The sample selection criteria are as follows: (I) patients need to have complete survival information, including survival time and survival state. (II) Patients have complete clinical information. (III) Total sample size in each dataset >500. ARGs and IRGs were obtained from GeneCards and immPorts, respectively (24). Anoikis and immune-related regulators (AIRRs) were obtained from the online site Venny2.1. Functional protein associated networks were mapped by STRING online database. Differential expression of AIRRs were analyzed by the R “limma” package.
Clustering analysis
Based on the differential expression of AIRRs, the clustering analysis was performed by “consensusClusterPlus” to identify the different patterns of AIRRs (25). The differential genes were then analyzed by “limma” package.
Risk signature construction
Using the TCGA database as the training set and GSE68465 as the validation set, overall survival (OS) related genes were ascertained by univariate Cox regression, and overfitting was avoided by least absolute shrinkage and selection operator (LASSO) regression. Subsequently, patients were divided into two risk groups according to median risk score. K-M survival curves were plotted for both data sets using the “survival” package. Time-dependent receiver operating characteristic (ROC) curves were plotted by the “timeROC” package. Principal component analysis (PCA) and t-distributed stochastic neighbor embedding (t-SNE) were plotted by the “Rtsne” package in R.
Model verification
Independent prognostic analysis was performed by the “survival” package. The expression of AIRRs in the two groups were illustrated by the “limma” and the “pheatmap” package. The “rms” package was utilized to construct a prognostic nomogram and to evaluate the accuracy between the predicted and actual OS of the nomogram.
Function enrichment analysis
Gene Ontology (GO) enrichment of differential genes were analyzed by the “GOplot” package to illustrate the biological function of differential genes. The Kyoto Encyclopedia of Genes and Genomes (KEGG) pathway enrichment was demonstrated by gene set enrichment analysis (GSEA) to describe distinct signal pathway between the two risk groups.
Estimation of tumor mutation burden (TMB) and immune cell infiltration
The data of TMB in LUAD was download by TCGA. TMB was assessed for both risk groups. Meanwhile, the differential expression of different immune cells in the two risk groups was assessed by single-sample gene set enrichment analysis (ssGSEA) and CIBRSORT. In addition, the relationship between 10 AIRGs and immune infiltrating cells was evaluated by TIMER online database (26).
Drug sensitivity
Half maximal inhibitory concentration (IC50) of chemotherapeutic drugs and targeted drugs by “pRRophetic” R package was evaluated (27).
Survival analysis of AIRGs and differential genes expression authentication
The survival curves of AIRGs were illustrated by Kaplan-Meier (K-M) plotter (28). By quantitative real-time PCR (qRT-PCR), the expression of 9 AIRGs on BEAS-2b (lung normal cell line) and A549 and PC-9 (two lung cancer cell lines) was validated. Total mRNA was extracted by TRIzol and reverse transcription PCR by Prime Script RT Master. Subsequently, the cDNA was tested by qRT-PCR. The relative mRNA expression was calculated by 2−ΔΔCT. All primers are shown in Table 1. Primers for the gene ANXA2P2 were not found.
Table 1
Gene name | Primer sequences |
---|---|
GAPDH | Forward: CAGGAGGCATTGCTGATGAT |
Reverse: GAAGGCTGGGGCTCATTT | |
ABCA3 | Forward: GGACCTCCTTAGCCCTGTC |
Reverse: TCTTCGAGCACCCCTTCAAC | |
ADH1B | Forward: GGGCCATTGTGATTGAAG |
Reverse: TGTGGGGCATTTTATTTGA | |
TCN1 | Forward: GGAAGCACACAATGGCACTC |
Reverse: GGCGGTTGAGTAGTTCCCAT | |
TNS4 | Forward: CCCAGTGTCTGATGTCAGCTAT |
Reverse: CTGGAGGAAGAGTTGGCTGG | |
DSG2 | Forward: TGCTGCTTCTCCTGATCTGC |
Reverse: ATCCTCTCCCTCCCGAAGAG | |
FRS3 | Forward: ATGTGAGCCCCAGGTGT |
Reverse: CCCCATGGTGTCAGAGCAG | |
UNC13B | Forward: TCCTACCTCCCAGCGAT |
Reverse: TCACCGTCTGAGCGAAC | |
STC1 | Forward: AGCCTCTTGGAAATCAGGT |
Reverse: TGGCTTAGTTGGGTTTGC | |
HLA-DQB2 | Forward: TGTGCTACTTCACCAACGG |
Reverse: TGTTCCAGTCCTCGATGC |
Statistical analysis
Most of the plots and statistical analyses were performed using R x64 4.1.2. T-test was used to evaluate the differential expression of individual genes. A P value less than 0.05 was defined as a statistically significant difference.
Ethical statement
The study was conducted in accordance with the Declaration of Helsinki (as revised in 2013).
Results
Acquisition of differential AIRRs
The GeneCards and immPorts databases were used to acquire 434 and 1,793 ARGs and IRGs, respectively (available online: https://cdn.amegroups.cn/static/public/tcr-22-2550-1.xlsx, https://cdn.amegroups.cn/static/public/tcr-22-2550-2.xlsx). One hundred and nine genes were defined as AIRRs by Venn plot (Figure 1A) (available online: https://cdn.amegroups.cn/static/public/tcr-22-2550-3.xlsx). Protein interaction networks showed a strong correlation between AIRRs (Figure 1B). Eighty-five differential genes were identified between normal lung tissue and LUAD (Figure 1C). Finally, the expression of 10 AIRRs was shown by box line plots (Figure 1D).
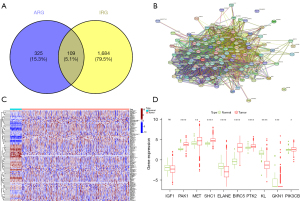
Clustering construction
The LUAD patients were clustered by the expression of AIRRs and the best clustering results were obtained at K=2 (Figure 2A-2C). The prognosis of cluster 2 was significantly worse than the corresponding cluster (Figure 2D). In addition, the heat map revealed that multiple AIRRs were upregulated in cluster 1. And the clustering results showed significant correlation between T-stage, gender, and age (Figure 2E). In conclude, a close correlation between the expression of AIRRs and LUAD was demonstrated.
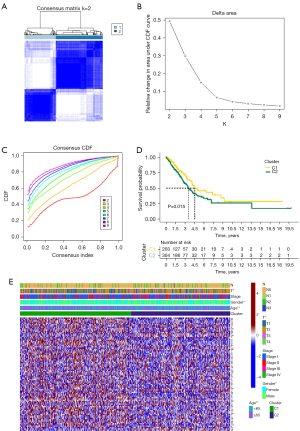
Developing a risk-prognosis signature
One hundred and thirty-three AIRGs with significant differences were found in C1 and C2. Fifteen genes were significantly associated with OS by univariate analysis (Figure 3A). By LASSO regression analysis, 10 AIRGs associated with OS were identified (Figure 3B,3C). K-M curves demonstrated a disappointing prognosis in the high-risk group in the GEO and TCGA datasets, respectively (Figure 3D,3E). ROC curves showed well predictive function of predictive models for OS (Figure 3F,3G). Meanwhile, the higher the risk score, the worse the prognosis (Figure 3H-3K). Finally, the PCA and t-SNE showed that patients were appropriately divided into two categories (Figure 3I-3O).
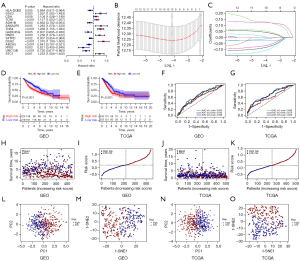
Validation of prognostic signature
Risk score could be treated as an independent prognostic factor for survival by univariate and multifactor independent prognosis, which further illustrated the reliability of the constructed model (Figure 4A-4D). Meanwhile, the heat map illustrated the expression of 10 AIRGs in the two risk groups. Among them, TCN1, ANXA2P2, TNS4, DSG2, STC1 were highly expressed in the high-risk group (Figure 4E,4F). In addition, the nomogram plots were constructed to predict OS at different time points (Figure 4G-4J). These results indicated that the model constructed was meaningful.
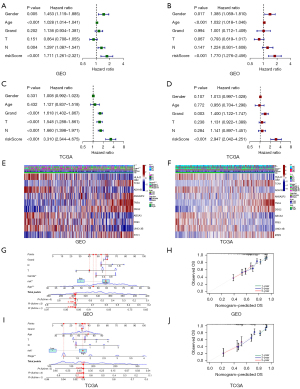
Functional enrichment analysis
GO enrichment mainly indicated that the differential genes were mainly related to human immune response, antimicrobial humoral response, and cell-cell junctions (Figure 5A,5B). KEGG enrichment showed that genes in the high risk group were mainly enriched in cell cycle, homologous recombination and P53 signaling pathway, while genes in the low risk group were primarily enriched in α-linolenic acid, arachidonic acid and asthma (Figure 5C,5D).
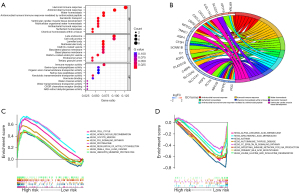
Immune microenvironment analysis
In the same cancer species, different immune cell infiltration can lead to different treatment outcomes. Therefore, the immune cell infiltration and immune function were analyzed in two risk groups. The results showed that there were significant differences in activated dendritic cells (aDCs), B cells, macrophages and mast cells among the two different risk groups. Higher abundance of aDCs, B cells, immature dendritic cells (iDCs), etc. in the low-risk group (Figure 6A-6F). These results provided some theoretical basis for clinical immunotherapy. The TMB was analyzed in different risk groups as TMB was associated with immune escape in tumor patients. The results indicated that the higher the risk score, the higher the TMB (Figure 7A-7C). In contrast, Tumor Immune Dysfunction and Exclusion (TIDE) scores were negatively correlated with risk scores (Figure 7D). In addition, the relationship between 10 AIRGs and B cells, CD8+ T cells, macrophages, CD4+ T cells also were described by TIMER database (Figure 8A-8J).
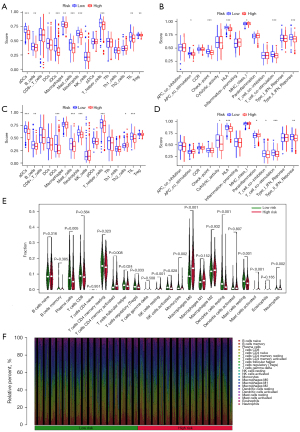
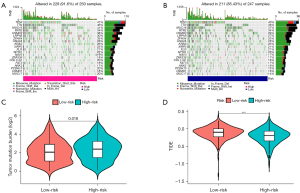
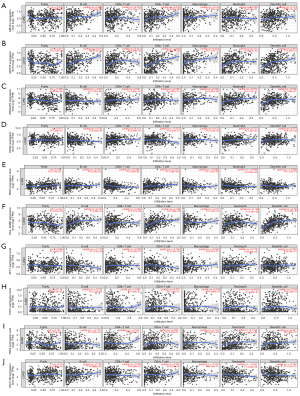
Drug sensitivity analysis of prognostic signature
Targeted drugs are one of the main treatment options for LUAD. Drug sensitivity testing is essential for patients who require targeted therapy. The IC50 of the various drugs differed significantly across the two risk groups, include Akt inhibitor, PARP inhibitor, Lck inhibitor, VEGFR inhibitor, AMPK inhibitor and JNK inhibitor (Figure 9A-9H). These results can provide guidance to clinical targeted drug administration.
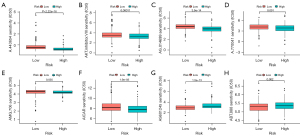
The survival analysis and experimental validation of differential genes
By K-M plotter, high expression of ANXA2P2, DSG2, FRS3, STC1, TCN1, TNS4 predicted a worse prognosis, while in contrast, ABCA3, ADH1B, HLA-DQB2 and UNC13B demonstrated a better prognosis (Figure 10A-10J). Finally, the mRNA levels of different genes in the three cell lines were analyzed by qRT-PCR. The results showed that all genes except TCN1 and TNS4 were down-regulated in expression in both tumor cell lines (Figure 11A-11I).
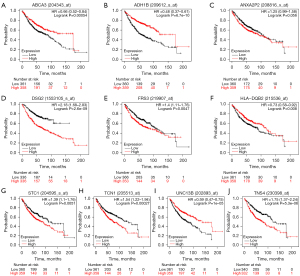
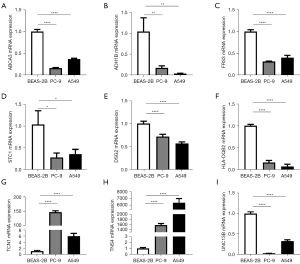
Discussion
Lung cancer, as one of the deadliest malignant tumors worldwide, has a number of subtypes (29). LUAD is the most general reason of cancer-related death among all lung cancer subtypes. Due to the unknown pathogenesis, the treatment of LUAD is unsatisfactory (30). Thus, finding new molecular markers should be seen as an urgent need.
Tumor metastasis is the leading cause of tumor-related death (31). Even though drug and surgical treatments are well developed, the five-year survival rate for patients with metastatic disease is still low (30). Currently, studies on tumor metastasis mainly focused on epithelial mesenchymal transformation of cancer cells, and neovascularization (32). However, the ECM also plays a particularly important role in the metastasis of tumors (33). Both fibroblasts and vascular endothelial cells in the tumor microenvironment produce a variety of cytokines that promote tumor migration and invasion (34-36). Meanwhile, various matrix proteins, collagen and other soluble molecules in the tumor microenvironment can also promote tumor metastasis.
The anti-anoikis behavior of tumors can promote tumor survival during metastasis (37). There is increasing evidences that anoikis plays an important role in the biological behavior of tumors (38,39). For example, bone morphogenetic proteins (BMPs) can promote anoikis resistance through upregulation of Notch signaling, and promote proliferation and metastasis of breast cancer (40). In non-small cell lung cancer, aberrant expression of the zinc finger protein (ZIC) family was associated with anoikis, and silencing ZIC2 reduced anoikis resistance in NSCLC cells. In addition, the number of circulating tumor cells and ZIC2 expression were positively correlated (41). Also, in gastric cancer, anoikis resistance interacts biologically with angiogenesis, thus promoting peritoneal metastasis of gastric cancer (42). Therefore, anoikis-related gene is a very important target for tumor-targeted therapy.
Five-year survival rates for LUAD patients have been improved significantly due to the rapid development of immunotherapy (43). However, the degree of response to immunotherapy varies from patient to patient due to individual heterogeneity (44,45). Different levels of immune cell infiltration and TMB often lead to contrary clinical outcomes compared to expectations (46,47). Therefore, there is an urgent need for integration of IRGs for precision therapy.
In our study, we present for the first time anoikis-related and IRGs in LUAD. Stratified clustering by AIRRs was performed to classify LUAD patients in TCGA into C1 and C2 categories. Then, we performed prognostic modeling by differential genes significantly associated with OS in both clusters. Finally, ten differential genes were identified (HLA-DQB2, TCA1, ADH1B, ANXA2P2, TNS4, DSG2, ABCA3, FRS3, UNC13B, STC1). Among these genes, ADH1B, a metabolism-related gene, has been associated with immune regulation and therapeutic response in a variety of cancers (48-50). TNS4 can promote tumorigenicity in colorectal cancer cells, and inhibition of TNS4 can make colorectal cancer more sensitive to cetuximab (51). Meanwhile, high expression of TNS4 suggested a worse prognosis for gastric cancer (52). DSG2 is an independent prognostic factor in multiple myeloma (53). Meanwhile, DSG2 promoted the proliferation and migration of LUAD cell lines and increased the resistance to Osimertinib (54). In glioblastoma, the cyclic RNA circPOSTN can promote neovascularization by regulating the miR-219a-2-3p/STC1 axis and promoting the expression of VEGFA (55). Meanwhile, ABCA3, FRS3 and other genes have also been reported in a variety of cancers (56,57). Therefore, more in-depth studies are need for these genes in LUAD. Subsequently, we verified the risk signature by K-M curves, ROC curves, PCA, t-SNE, nomogram, and independent prognostic analysis. All these results indicated that the prognostic signature had good predictive function. Then, we enriched GO and KEGG for differential genes in different risk groups and found that these genes were mainly enriched in human immune response, immune receptor activation, cell cycle, and metabolic pathways, so we also analyzed immune cells, immune infiltration function, TMB, and immune escape for patients in two risk groups (58,59). The results showed that immune cells, such as aDCs, B cells, iDCs, mast cells, TIL, were different in the both risk groups. And the results demonstrated that TMB was significantly higher in high-risk patients than comparable patients, while the TIDE score was significantly lower than in low-risk patients, suggesting that high-risk patients may gain more benefit from immune blockade therapy (60-62).
A novel risk-prognosis signature was built to guide the clinical diagnosis and treatment of LUAD by using the TCGA database as internal validation and the GEO dataset for external validation. Meanwhile, the mRNA level of AIRGs was verified by qRT-PCR. However, our study still has significant limitations. Firstly, the data were all from public databases. Secondly, we only validated the mRNA level for AIRGs by three cell lines. Therefore, we will subsequently collect clinical data for further testing of the prognostic model. In addition, we will perform further in vitro and in vivo validation for AIRGs.
Conclusions
Based on the ARGs and IRGs, we established a 10-gene risk model and performed a simple experimental validation. The establishment of new biological markers is important for predicting prognosis and targeted therapy in LUAD.
Acknowledgments
All our authors are grateful to the TCGA database and GEO database for providing clinical patient information. Also, we thank the staff who built the GeneCards and immPorts databases.
Funding: This research was supported by
Footnote
Reporting Checklist: The authors have completed the TRIPOD reporting checklist. Available at https://tcr.amegroups.com/article/view/10.21037/tcr-22-2550/rc
Peer Review File: Available at https://tcr.amegroups.com/article/view/10.21037/tcr-22-2550/prf
Conflicts of Interest: All authors have completed the ICMJE uniform disclosure form (available at https://tcr.amegroups.com/article/view/10.21037/tcr-22-2550/coif). The authors have no conflicts of interest to declare.
Ethical Statement: The authors are accountable for all aspects of the work and in ensuring that questions related to the accuracy or integrity of any part of the work are appropriately investigated and resolved. The study was conducted in accordance with the Declaration of Helsinki (as revised in 2013).
Open Access Statement: This is an Open Access article distributed in accordance with the Creative Commons Attribution-NonCommercial-NoDerivs 4.0 International License (CC BY-NC-ND 4.0), which permits the non-commercial replication and distribution of the article with the strict proviso that no changes or edits are made and the original work is properly cited (including links to both the formal publication through the relevant DOI and the license). See: https://creativecommons.org/licenses/by-nc-nd/4.0/.
References
- Zito Marino F, Bianco R, Accardo M, et al. Molecular heterogeneity in lung cancer: from mechanisms of origin to clinical implications. Int J Med Sci 2019;16:981-9. [Crossref] [PubMed]
- Wang DC, Wang W, Zhu B, et al. Lung Cancer Heterogeneity and New Strategies for Drug Therapy. Annu Rev Pharmacol Toxicol 2018;58:531-46. [Crossref] [PubMed]
- Hua X, Zhao W, Pesatori AC, et al. Genetic and epigenetic intratumor heterogeneity impacts prognosis of lung adenocarcinoma. Nat Commun 2020;11:2459. [Crossref] [PubMed]
- Taddei ML, Giannoni E, Fiaschi T, et al. Anoikis: an emerging hallmark in health and diseases. J Pathol 2012;226:380-93. [Crossref] [PubMed]
- Simpson CD, Anyiwe K, Schimmer AD. Anoikis resistance and tumor metastasis. Cancer Lett 2008;272:177-85. [Crossref] [PubMed]
- Chiarugi P, Giannoni E. Anoikis: a necessary death program for anchorage-dependent cells. Biochem Pharmacol 2008;76:1352-64. [Crossref] [PubMed]
- Yu B, Gu D, Zhang X, et al. Regulation of pancreatic cancer metastasis through the Gli2-YAP1 axis via regulation of anoikis. Genes Dis 2022;9:1427-30. [Crossref] [PubMed]
- Han P, Lei Y, Liu J, et al. Cell adhesion molecule BVES functions as a suppressor of tumor cells extrusion in hepatocellular carcinoma metastasis. Cell Commun Signal 2022;20:149. [Crossref] [PubMed]
- Ye G, Yang Q, Lei X, et al. Nuclear MYH9-induced CTNNB1 transcription, targeted by staurosporin, promotes gastric cancer cell anoikis resistance and metastasis. Theranostics 2020;10:7545-60. [Crossref] [PubMed]
- Wang YN, Zeng ZL, Lu J, et al. CPT1A-mediated fatty acid oxidation promotes colorectal cancer cell metastasis by inhibiting anoikis. Oncogene 2018;37:6025-40. [Crossref] [PubMed]
- Meng Q, Lu YX, Wei C, et al. Arginine methylation of MTHFD1 by PRMT5 enhances anoikis resistance and cancer metastasis. Oncogene 2022;41:3912-24. [Crossref] [PubMed]
- Du S, Miao J, Zhu Z, et al. NADPH oxidase 4 regulates anoikis resistance of gastric cancer cells through the generation of reactive oxygen species and the induction of EGFR. Cell Death Dis 2018;9:948. [Crossref] [PubMed]
- Wu T, Dai Y. Tumor microenvironment and therapeutic response. Cancer Lett 2017;387:61-8. [Crossref] [PubMed]
- Hernández-Camarero P, López-Ruiz E, Marchal JA, et al. Cancer: a mirrored room between tumor bulk and tumor microenvironment. J Exp Clin Cancer Res 2021;40:217. [Crossref] [PubMed]
- Li L, Yu R, Cai T, et al. Effects of immune cells and cytokines on inflammation and immunosuppression in the tumor microenvironment. Int Immunopharmacol 2020;88:106939. [Crossref] [PubMed]
- Dagogo-Jack I, Shaw AT. Tumour heterogeneity and resistance to cancer therapies. Nat Rev Clin Oncol 2018;15:81-94. [Crossref] [PubMed]
- Li B, Cui Y, Diehn M, et al. Development and Validation of an Individualized Immune Prognostic Signature in Early-Stage Nonsquamous Non-Small Cell Lung Cancer. JAMA Oncol 2017;3:1529-37. [Crossref] [PubMed]
- Tang Y, Tian W, Xie J, et al. Prognosis and Dissection of Immunosuppressive Microenvironment in Breast Cancer Based on Fatty Acid Metabolism-Related Signature. Front Immunol 2022;13:843515. [Crossref] [PubMed]
- Zhou Y, Gao S, Yang R, et al. Identification of a three-gene expression signature and construction of a prognostic nomogram predicting overall survival in lung adenocarcinoma based on TCGA and GEO databases. Transl Lung Cancer Res 2022;11:1479-96. [Crossref] [PubMed]
- Garaud S, Buisseret L, Solinas C, et al. Tumor infiltrating B-cells signal functional humoral immune responses in breast cancer. JCI Insight 2019;5:e129641. [Crossref] [PubMed]
- Sakamoto S, Kyprianou N. Targeting anoikis resistance in prostate cancer metastasis. Mol Aspects Med 2010;31:205-14. [Crossref] [PubMed]
- Deng M, Brägelmann J, Schultze JL, et al. Web-TCGA: an online platform for integrated analysis of molecular cancer data sets. BMC Bioinformatics 2016;17:72. [Crossref] [PubMed]
- Director's Challenge Consortium for the Molecular Classification of Lung Adenocarcinoma. Gene expression-based survival prediction in lung adenocarcinoma: a multi-site, blinded validation study. Nat Med 2008;14:822-7. [Crossref] [PubMed]
- Rebhan M, Chalifa-Caspi V, Prilusky J, et al. GeneCards: a novel functional genomics compendium with automated data mining and query reformulation support. Bioinformatics 1998;14:656-64. [Crossref] [PubMed]
- Wilkerson MD, Hayes DN. ConsensusClusterPlus: a class discovery tool with confidence assessments and item tracking. Bioinformatics 2010;26:1572-3. [Crossref] [PubMed]
- Li T, Fan J, Wang B, et al. TIMER: A Web Server for Comprehensive Analysis of Tumor-Infiltrating Immune Cells. Cancer Res 2017;77:e108-10. [Crossref] [PubMed]
- Geeleher P, Cox N, Huang RS. pRRophetic: an R package for prediction of clinical chemotherapeutic response from tumor gene expression levels. PLoS One 2014;9:e107468. [Crossref] [PubMed]
- Tang Z, Li C, Kang B, et al. GEPIA: a web server for cancer and normal gene expression profiling and interactive analyses. Nucleic Acids Res 2017;45:W98-W102. [Crossref] [PubMed]
- Sung H, Ferlay J, Siegel RL, et al. Global Cancer Statistics 2020: GLOBOCAN Estimates of Incidence and Mortality Worldwide for 36 Cancers in 185 Countries. CA Cancer J Clin 2021;71:209-49. [Crossref] [PubMed]
- Hu FF, Chen H, Duan Y, et al. CBX2 and EZH2 cooperatively promote the growth and metastasis of lung adenocarcinoma. Mol Ther Nucleic Acids 2021;27:670-84. [Crossref] [PubMed]
- Weiss F, Lauffenburger D, Friedl P. Towards targeting of shared mechanisms of cancer metastasis and therapy resistance. Nat Rev Cancer 2022;22:157-73. [Crossref] [PubMed]
- Lin PP. Aneuploid Circulating Tumor-Derived Endothelial Cell (CTEC): A Novel Versatile Player in Tumor Neovascularization and Cancer Metastasis. Cells 2020;9:1539. [Crossref] [PubMed]
- Girigoswami K, Saini D, Girigoswami A. Extracellular Matrix Remodeling and Development of Cancer. Stem Cell Rev Rep 2021;17:739-47. [Crossref] [PubMed]
- Schwager SC, Young KM, Hapach LA, et al. Weakly migratory metastatic breast cancer cells activate fibroblasts via microvesicle-Tg2 to facilitate dissemination and metastasis. Elife 2022;11:e74433. [Crossref] [PubMed]
- Desbois M, Wang Y. Cancer-associated fibroblasts: Key players in shaping the tumor immune microenvironment. Immunol Rev 2021;302:241-58. [Crossref] [PubMed]
- Maishi N, Hida K. Tumor endothelial cells accelerate tumor metastasis. Cancer Sci 2017;108:1921-6. [Crossref] [PubMed]
- Song J, Liu Y, Liu F, et al. The 14-3-3σ protein promotes HCC anoikis resistance by inhibiting EGFR degradation and thereby activating the EGFR-dependent ERK1/2 signaling pathway. Theranostics 2021;11:996-1015. [Crossref] [PubMed]
- Takeshita Y, Motohara T, Kadomatsu T, et al. Angiopoietin-like protein 2 decreases peritoneal metastasis of ovarian cancer cells by suppressing anoikis resistance. Biochem Biophys Res Commun 2021;561:26-32. [Crossref] [PubMed]
- Surette A, Yoo BH, Younis T, et al. Tumor levels of the mediators of ErbB2-driven anoikis resistance correlate with breast cancer relapse in patients receiving trastuzumab-based therapies. Breast Cancer Res Treat 2021;187:743-58. [Crossref] [PubMed]
- Sharma R, Gogoi G, Saikia S, et al. BMP4 enhances anoikis resistance and chemoresistance of breast cancer cells through canonical BMP signaling. J Cell Commun Signal 2022;16:191-205. [Crossref] [PubMed]
- Liu A, Xie H, Li R, et al. Silencing ZIC2 abrogates tumorigenesis and anoikis resistance of non-small cell lung cancer cells by inhibiting Src/FAK signaling. Mol Ther Oncolytics 2021;22:195-208. [Crossref] [PubMed]
- Du S, Yang Z, Lu X, et al. Anoikis resistant gastric cancer cells promote angiogenesis and peritoneal metastasis through C/EBPβ-mediated PDGFB autocrine and paracrine signaling. Oncogene 2021;40:5764-79. [Crossref] [PubMed]
- Li B, Severson E, Pignon JC, et al. Comprehensive analyses of tumor immunity: implications for cancer immunotherapy. Genome Biol 2016;17:174. [Crossref] [PubMed]
- Galluzzi L, Humeau J, Buqué A, et al. Immunostimulation with chemotherapy in the era of immune checkpoint inhibitors. Nat Rev Clin Oncol 2020;17:725-41. [Crossref] [PubMed]
- Bie F, Tian H, Sun N, et al. Comprehensive analysis of PD-L1 expression, tumor-infiltrating lymphocytes, and tumor microenvironment in LUAD: differences between Asians and Caucasians. Clin Epigenetics 2021;13:229. [Crossref] [PubMed]
- Dai X, Lu L, Deng S, et al. USP7 targeting modulates anti-tumor immune response by reprogramming Tumor-associated Macrophages in Lung Cancer. Theranostics 2020;10:9332-47. [Crossref] [PubMed]
- Chen J, Yang H, Teo ASM, et al. Genomic landscape of lung adenocarcinoma in East Asians. Nat Genet 2020;52:177-86. [Crossref] [PubMed]
- Xu Z, Peng B, Kang F, et al. The Roles of Drug Metabolism-Related ADH1B in Immune Regulation and Therapeutic Response of Ovarian Cancer. Front Cell Dev Biol 2022;10:877254. [Crossref] [PubMed]
- Feng D, Shi X, Zhang F, et al. Energy Metabolism-Related Gene Prognostic Index Predicts Biochemical Recurrence for Patients With Prostate Cancer Undergoing Radical Prostatectomy. Front Immunol 2022;13:839362. [Crossref] [PubMed]
- Choi CK, Shin MH, Cho SH, et al. Association between ALDH2 and ADH1B Polymorphisms and the Risk for Colorectal Cancer in Koreans. Cancer Res Treat 2021;53:754-62. [Crossref] [PubMed]
- Kim S, Kim N, Kang K, et al. Whole Transcriptome Analysis Identifies TNS4 as a Key Effector of Cetuximab and a Regulator of the Oncogenic Activity of KRAS Mutant Colorectal Cancer Cell Lines. Cells 2019;8:878. [Crossref] [PubMed]
- Sawazaki S, Oshima T, Sakamaki K, et al. Clinical Significance of Tensin 4 Gene Expression in Patients with Gastric Cancer. In Vivo 2017;31:1065-71. [PubMed]
- Ebert LM, Vandyke K, Johan MZ, et al. Desmoglein-2 expression is an independent predictor of poor prognosis patients with multiple myeloma. Mol Oncol 2022;16:1221-40. [Crossref] [PubMed]
- Jin R, Wang X, Zang R, et al. Desmoglein-2 modulates tumor progression and osimertinib drug resistance through the EGFR/Src/PAK1 pathway in lung adenocarcinoma. Cancer Lett 2020;483:46-58. [Crossref] [PubMed]
- Long N, Xu X, Lin H, et al. Circular RNA circPOSTN promotes neovascularization by regulating miR-219a-2-3p/STC1 axis and stimulating the secretion of VEGFA in glioblastoma. Cell Death Discov 2022;8:349. [Crossref] [PubMed]
- Valencia T, Joseph A, Kachroo N, et al. Role and expression of FRS2 and FRS3 in prostate cancer. BMC Cancer 2011;11:484. [Crossref] [PubMed]
- Ceraulo A, Lapillonne H, Cheok MH, et al. Prognostic impact of ABCA3 expression in adult and pediatric acute myeloid leukemia: an ALFA-ELAM02 joint study. Blood Adv 2022;6:2773-7. [Crossref] [PubMed]
- Raverot G, Ilie MD. Immunotherapy in pituitary carcinomas and aggressive pituitary tumors. Best Pract Res Clin Endocrinol Metab 2022;36:101712. [Crossref] [PubMed]
- Dumauthioz N, Labiano S, Romero P. Tumor Resident Memory T Cells: New Players in Immune Surveillance and Therapy. Front Immunol 2018;9:2076. [Crossref] [PubMed]
- Liu L, Bai X, Wang J, et al. Combination of TMB and CNA Stratifies Prognostic and Predictive Responses to Immunotherapy Across Metastatic Cancer. Clin Cancer Res 2019;25:7413-23. [Crossref] [PubMed]
- Picard E, Verschoor CP, Ma GW, et al. Relationships Between Immune Landscapes, Genetic Subtypes and Responses to Immunotherapy in Colorectal Cancer. Front Immunol 2020;11:369. [Crossref] [PubMed]
- Jiang P, Gu S, Pan D, et al. Signatures of T cell dysfunction and exclusion predict cancer immunotherapy response. Nat Med 2018;24:1550-8. [Crossref] [PubMed]