Bioinformatics identification of prognostic genes and potential interaction analysis in renal cell carcinoma
Highlight box
Key findings
• ALDOB was identified as the only one gene that impacts on the prognosis of RCC patients.
What is known and what is new?
• RCC is one of the ten most prevalent cancers in the world.
• Five genes were found to be overlappingly expressed in the top 20 greatest FC in two RCC human GEO datasets, however, the under-expressed of ALDOB in human RCC has a novel predictive value for clinical outcome.
What is the implication, and what should change now?
• ALDOB is of great value in the treatment and prognosis of RCC.
Introduction
Renal cell carcinoma (RCC) accounts for 2% to 3% of all types of human cancers (1). More than 338,000 individuals are diagnosed with RCC every year, and is expected to increase by 22% by 2023; with more than 140,000 RCC-related deaths occur each year (2). Over the past decade, the prognosis of RCC patients has been significantly improved with enhanced surgical procedures and the use of specific targeted drugs (3). The clinical outcome of individuals diagnosed at early stage is comparatively good. However, approximately 50% of the patients progress metastatic disease and under this setting, renal cancer remains incurable with a median survival of around of 30 months (4). The development of effective treatment regimens has been hampered by a limited understanding of the basic molecular pathways underpinning RCC carcinogenesis. However, it is still a great challenge to accurately understand the pathological changes of RCC.
Tumor progression depends on the continuation of cancer cells to metabolize biomass simultaneously and the process, at the same time, remains feasible in tumor microenvironment. It is widely believed that the change in cell metabolism would lead to the uncontrolled growth of cancer cells (5). Notably, enhanced glycolysis, first revealed by Warburg in the 1920s, provides a large number of biosynthetic precursors for the production of amino acids, lipids, nucleotides, nicotinamide adenine dinucleotide phosphate (NAPDH), and other biochemical intermediates (6). Otto Warburg observed that cancer cells metabolized glucose faster than normal cells and that the main metabolic product was lactic acid. This indicated that glucose metabolism was suspended at the last step of glycolysis. In most tissue cells, glucose transported into the mitochondria, where glucose is metabolized into carbon dioxide and water through tricarboxylic acid (TCA) cycle, with the accompanying production of 36 molecules of adenosine-triphosphate (ATP) per molecule of glucose. Thus, under hypoxic conditions, glucose metabolism stops at the end of glycolysis while producing lactate acid (7). A single glucose molecule produces 36 ATP molecules, and this produces more ATP than in the absence of oxygen. However, even sufficient oxygen is available, glucose metabolism in cancer cells produces huge amount of lactate acid. This abnormal metabolic pattern is known as “Warburg effect” (8).
Despite the discovery of oncogenes and tumor suppressors which are largely responsible for intrinsic genetic changes in cancer, little progress of cancer treatment has been made over the past 20 years. However, in the past 20 years, the Warburg effect has received increasing attention for playing an essential role in the progression of cancer. This is being revealed in numerous recent reviews and comments. Nevertheless, “reprogramming of energy metabolism” proposed as an emerging hallmark of cancer by Hanahan and Weinberg (9), is perhaps the strongest evidence suggesting that metabolic changes in cancer cells have become the mainstreams of cancer research. A relatively recent publication has shown that the genes involved in aerobic glycolysis are over-expressed in at least 24 types of cancers in human, and that corresponds to approximately 70% of all cancers (10).
Vertebrate aldolases, also known as fructose-(bis) phosphate aldolase (ALDO), is a key enzyme participating in glycolysis and gluconeogenesis that catalyze fructose-1,6-bisphosphate to glyceraldehyde-3-phosphate and dihydroxyacetone phosphate (11). Human aldolase contains three isozymes: ALDOA, -B, and -C. ALDOA is expressed mainly in muscle and fetal liver, but those are not the only sites of expression. A series of literatures have revealed that high levels of ALDOA is closely related to lung carcinoma, advanced oral squamous cell carcinoma, and osteosarcoma (12-14). Some of these studies have reported that ALDOA can play a key role as an independent prognostic factor, as in cases of lung adenocarcinoma, breast cancer, and gastric cancer. ALDOC, the third kind of aldolase, is abundant in the brain (15). Recent studies have revealed abnormal expression of ALDOB is associated with neuronal damage and Alzheimer’s disease (16,17). ALDOC suppresses oral squamous cell migration and negatively correlates to the clinical outcome. ALDOB is predominantly expressed in adult liver, so also known as liver-type aldolase (18), ALDOB has a similar sequence to ALDOA (66% identity) and ALDOC (68% identity) (19). Several genetic mutations and polymorphisms of the ALDOB gene lead to a deficiency in ALDOB and have been identified as the pathogenesis of hereditary fructose intolerance (20). Abnormal expression of ALDOB is associated with a variety of diseases, such as hepatitis, liver cirrhosis, and even cancer (21). Several documents have revealed that under-expression of ALDOB is related to hepatocellular carcinoma, gastric cancer (22). However, the role of ALDOB in RCC is yet to be reported.
Bioinformatics analysis of microarray data has been widely used to identify genetic variations in oncological research, and it is also essential in exploring novel prognostic biomarkers in tumor progression, as in the case of renal carcinoma (23). Recently, massive documental research was conducted to reveal the differentially expressed genes (DEGs) in clear cell RCC (ccRCC) and revealed their roles in biological processes, molecular functions and different pathways.
In the present study, data generated by gene expression microarray were investigated and the differential expression of ALDOB in tumor compared to adjacent non-tumor was found to be ranked with high level in multiple databases. Furthermore, the expression of ALDOB on the prediction of RCC prognosis and its mechanism behind, of which the interaction between ALDOB and molecules and its prognostic significance on RCC are also discussed here. We present the following article in accordance with the REMARK reporting checklist (available at https://tcr.amegroups.com/article/view/10.21037/tcr-22-2242/rc).
Methods
Microarray data
From the Gene Expression Omnibus (GEO) database (https://ncbi.nlm.nih.gov/geo/), two gene expression profiles, GSE15641 and GSE40435 were downloaded. The expression array for GSE15641 consisted of a messenger RNA (mRNA) expression profile of 49 RCC tumors, and 23 normal kidney samples. Transcriptional profiling with oligonucleotide microarrays (22,283 genes, GPL96) was performed on all samples. The expression microarray of GSE40435 included 101 pairs RCC tumors and adjacent non-tumor renal tissues. The data were obtained using lllumina HumanHT-12 v4 expression beadchips (GPL10558). GSE15641 were used to explore the expression of multiple RCC types.
Identification of DEGs
GEO2R (https://www.ncbi.nlm.nih.gov/geo/geo2r/) is an interactive online tool to identify DEGs by comparing samples from GEO series. GEO2R was used to screen for DEGs between RCC and normal tissue samples. Student’s t-test was used to test the statistical significance of differential expression of genes. The Bejamini-Hochberg method was used to calculate the false discovery rate (FDR). The cut-off criteria were an adjusted P value of <0.05, P<0.05 and logFC ≥2 or <−2 for DEGs.
Cell transfection and quantitative real-time polymerase chain reaction (qRT-PCR)
The following lentivirus vectors were purchased from Shanghai GeneChem Co.: expression for short hairpin RNA (shRNA)-MACROD2 and negative control (hU6-MCS-Ubiquitin-EGFP-IRES-puromycin). The target sequence for ALDOB shRNA construction: GTGGGAATCAAGTTAGACCAA. Transduction was performed according to the instructions of the manufacturer. qRT-PCR was detected by using a SYBR PrimeScript RT-PCR Kit (Takara Bio, Shiga, Japan), per the manufacturer’s instructions. Primers sequences are as follows: GAPDH, forward (F): 5'-GGTATGACAACGAATTTGGC-3', reverse (R): 5'-GAGCACAGGGTACTTTATTG-3'; PFKP, F: 5'-TGACGTGGACATCCGCAAAG-3', R: 5'-CTGGAAGGTGGACAGCGAGG-3'; PFKM, F: 5'-GACCCGTGGTTCTCGTCTC-3', R: 5'-AAAGGCTGATGGCGTCCC-3'; PKLR, F: 5'-TGGGAAAACTGGGTGGGATGGATG-3', R: 5'-GAAGGAAGCAGCCGGGGATTTGAC-3'; FBP1, F: 5'-CTACGCCAGGGACTTTGACC-3', R: 5'-GGCCCCATAAGGAGCTGAAT-3'.
Statistical analysis
Protein-protein interaction (PPI) network and module analysis
The Search Tool for the Retrieval of Interacting Genes (STRING) database (https://cn.string-db.org/) is an online software that provides comprehensive interactions of lists of proteins and genes (24).
Survival analysis of genes in RCC
OncoLnc (http://www.oncolnc.org/) is an online software for interactively analyzing survival relationship, and enabling the download of clinical data and gene expression data for mRNAs, microRNAs (miRNAs), or long noncoding RNAs (lncRNAs). OncoLnc includes nearly 9,000 patient survival data from 21 pan-cancer studies accomplished by The Cancer Genome Atlas (TCGA). The total survival analysis of ccRCC was performed by a Kaplan-Meier plot. The ccRCC patients were divided into two groups with a 50% threshold on the basis of high or low expression for kininogen 1 (KNG1), aldolase, fructose-bisphosphate B (ALDOB), complement C1q B chain (C1QB), neuronal pentraxin 2 (NPTX2), triosephosphate isomerase 1 (TPI1), phosphofructokinase, liver type (PFKL), glyceraldehyde-3-phosphate dehydrogenase (GAPDH), phosphofructokinase, platelet (PFKP), phosphofructokinase, muscle (PFKM), GAPDH, spermatogenic (GAPDHS), pyruvate kinase L/R (PKLR), fructose-bisphosphatase 1 (FBP1), fructose-bisphosphatase 2 (FBP2), and pyruvate kinase M1/2 (PKM).
Ethical statement
The study was conducted in accordance with the Declaration of Helsinki (as revised in 2013).
Results
Identification of DEGs in two RCC database
The analysis of the GSE15641 dataset identified 625 DEGs with the threshold of P value of <0.01 and a logFC value of ≥2, including 415 increased and 210 decreased genes. While the genes in the GSE40435 dataset were analyzed and 343 DEGs with 101 upregulated and 242 downregulated genes were identified. The top 20 genes with the greatest fold change (FC) in each dataset were selected and the heat map was made, respectively (Figure 1A,1B). In order to find a more accurate target gene, we took the intersection of two databases and got KNG1, ALDOB, C1QB, NPTX2, and uromodulin (UMOD) for next investigation by Venn diagram (Figure 1C).
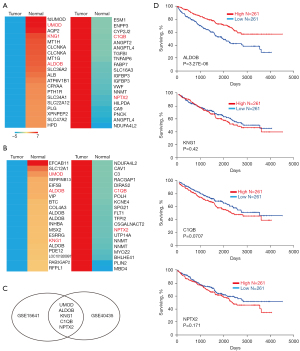
Association between KNG1, ALDOB, C1QB, NPTX2, and UMOD expression with prognosis of ccRCC patients
To better understanding the prognostic impact of the aforesaid differential expressed candidates (KNG1, ALDOB, C1QB, NPTX2, and UMOD) on the overall survival of ccRCC patients, survival information of 522 ccRCC patients from TCGA dataset through OncoLnc data portal was extracted and analyzed. Kaplan-Meier survival analyses were conducted with the high and low expression (threshold: 50%) groups on the basis of these genes and clinical outcome using OncoLnc. As shown in Figure 1D, the survival rate in the ccRCC patients who conferred high expression of ALDOB was significantly increased compared to patients with low expression of ALDOB by log-rank test (P=0.00000327). While the levels of KNG1, C1QB, and NPTX2 showed no statistically significant correlation between clinical prognosis rate of ccRCC patients (P=0.42, P=0.0707, and P=0.171, respectively). UMOD did not meet the expression cutoff for the analysis, so there was no information about UMOD in the OncoLnc. Then the expression of UMOD in the kidney renal papillary cell carcinoma was examined, another subtype of kidney cancer. However, the expression levels of UMOD conferred no significant association with the prognosis of renal papillary cell carcinoma (Figure S1). In addition, to determine the biological effects of ALDOB expression in ccRCC cells, we used shRNA to knock down ALDOB in Caki-1 cell. We detected the proliferation ability of ALDOB in Caki-1 cell by cell counting kit-8 (CCK-8), which showed that the capacity of proliferation was increased in the ALDOB knockdown cells (Figure S2). To sum up, these findings prompted us to study the mechanisms of ALDOB’s progress to ccRCC.
PPI network construction and analysis of modules
In order to investigate the intrinsic interaction of ALDOB in ccRCC progression, we constructed the PPI network by online tool STRING. In total, 55 nodes were mapped in the PPI network of ALDOB. The 10 highest-scoring direct interaction with ALDOB nodes were screened as hub genes (Figure 2A): TPI1, PFKL, GAPDH, PFKP, PFKM, GAPDHS, PKLR, FBP1, FBP2, PKM.
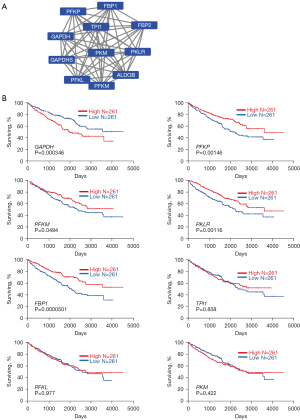
Identification of ALDOB interactors and their association with prognostic of ccRCC patients
To further examine the association of the expression levels of TPI1, PFKL, GAPDH, PFKP, PFKM, GAPDHS, PKLR, FBP1, FBP2, and PKM with the prognosis of ccRCC patients, Kaplan-Meier survival analyses were conducted on the 522 ccRCC patients with the high and low expression (threshold: 50%) groups on the basis of these genes and clinical outcome using OncoLnc. As showed in Figure 2B, the survival rate in the ccRCC patients who conferred high expression of PFKP, PFKM, PKLR, and FBP1 was significantly increased compared to patients with low expression of these genes by log-rank test (P=0.00146, P=0.0494, P=0.0116, P=0.0000501, respectively), while high expression of GAPDH showed poor prognosis (P=0.000346) and the levels of TPI1, PFKL and PKM showed no statistically significant correlation between clinical prognosis rate of ccRCC patients, respectively (P=0.858, P=0.977, P=0.422, respectively). Furthermore, we performed the qRT-PCR experiments to investigate the downstream targets of ALDOB. The results showed that the expression of GAPDH were upregulated and the level of PFKP, PFKM, PKLR, and FBP1 were downregulated in the ALDOB knockdown cell lines (Figure S3).
Discussion
Cancer has traditionally been regard as a disease of uncontrolled cell growth mediated by multiple oncogenes, such as mutations that activate growth factor receptors (25). Although glycolysis provides less energy than the Krebs cycle and less energy is detrimental to cell growth, glycolysis is more common in tumors. This paradox led to a series of discoveries suggesting that many metabolic processes in tumors are not directly linked to proliferation (26). Several classical metabolic pathways are upregulated, depressed or bypassed entirely in cancer cells and many of these metabolic changes, especially in kidney cancers (27). This study has led to changes concept in metabolic concepts, so‑known as metabolic reprogramming, is “successful” for kidney cancer. The reprogramming allows cancer cells to proliferate rapidly to satisfy their basic needs for cell growth components, including nucleic acid and protein constituents, as well as high levels of molecules that adjust the enhanced tumor energy situation.
As one of the most studied cancer, kidney cancer perhaps is a typical representative malignant tumor characterized by metabolic reprogramming (28). Genes that are dysregulated in kidney cancer are involved in many different cellular pathways that modulate various aspects of cell metabolism, such as oxygen sensing, the TCA cycle, glutamine metabolism, and tumor energy metabolism. As a result, kidney cancer has been labelled a metabolic disease (29). The most common type of kidney cancer is ccRCC, an aggressive cancer that derives from the proximal renal tubular epithelium and is highly lethal due to its metastatic nature (30). Metabolic reprogramming, a hallmark of malignancy involving changes in glycolytic enzymes, is becoming widely recognized (31). ALDO, which includes four catalytic tetramers, is a key enzyme in glycolysis. Evidence has shown that ALDO not only takes part in glycometabolism but is also involved in cell proliferation, signal transduction, and transcription. Therefore, the aberration of ALDO could be responsible for malignant transformation (32). In recent years, studies of the underlying roles of ALDO in cancer have become popular, and have revealed that the abnormal expression of ALDO has a close relationship with many kinds of cancers. ALDOB, which contains a large number of ALDOB-specific amino acid residues in its isozyme-specific region 2, is mainly responsible for cleaving fructose-1-phosphate into dihydroxyacetone phosphate and glyceraldehyde. Previous literature revealed that ALDOB were involved in the ccRCC development (33), the findings in this current study.
At the initial screening, we identified five DEGs through two databases. KNG1 is a precursor of kallikrein system associated with cardiovascular and renal function, blood pressure regulation and physiological and pathological processes (34). KNG1 could exhibit anti-angiogenic function and inhibit the proliferation of endothelial cells (35). Recently, KNG1 has been shown to have a role on carcinogenesis. The cancer patients who have a low expression of KNG1 can promote the survival of the cancer cells (36). Through mining data from TCGA, KNG1 was identified as the core factor associated with the prognosis of glioma patients (37). We also obtained the differential expression of KNG1 through database analysis, but it was not related to patient prognosis.
Functionally, C1QB is one of the subunits of complement subcomponent (38), which is related to immune responses in the human body. The expression of C1QB in the serum was associated with the ccRCC tumor stage and grade, but the clinical outcome has not been reported (39). However, we found that the levels of C1QB was much higher than adjacent non-tumor tissues, but had no relationship with prognosis. In this study, the levels of C1QB was differentially expressed, and patients with high expression tended to have shorter survival, but the P value did not reach the statistical difference.
NPTX2 is homologous to NPTX1 (NP1) and neuronal pentraxin receptor (NPTXR, NPR), both of which have been characterized in nervous system tissues (40). NPTX2 has a broader expression pattern, than can observed in many tissues, such as nerve, pancreas, skeletal muscle, heart, and liver (41). NTPX1 and NTPX2 bind to NTPXR to form homopolymers or heteropolymers, binding pentraxins to cell membrane (42). Previous studies have identified the role of pentraxin in neuronal growth and synaptic plasticity of neuronal cells (43,44). Furthermore, NPTX2 is highly expressed in ccRCC primary tumors and metastases, and contributes to the cell viability and promotes cell migration (45). In this study, the levels of NTPX2 were significantly differentially expressed, and patients with high expression tended to have shorter survival, but the P value did not reach the statistically significance.
Phosphofructose kinase (PFK), catalyzes the production of fructose 1,6-bisphosphate and adenosine diphosphate (ADP) from fructose 6-phosphate and ATP, is a key enzyme of the glycolytic pathway. Mammalian PFK includes three L, M, and P isoforms (46). In normal tissues, PFKL is predominately expressed in liver and kidney while platelets are abundant with PFKM and PFKP, respectively. A recent study illustrated that PFKP promotes aerobic glycolysis as well as suppresses p53 to maintain cellular proliferation (47). We also observed that the expression of FPKP or PFKM were overexpressed in RCC compared to normal tissues. Furthermore, we validated the patients with high levels of PFK or PFKM conferred a bad prognosis.
PKLR, as an isozyme of PKL, is the only enzyme for glycolytic pyruvate generation and regulation of biosynthetic pathways, is of great importance for liver cells. Mineralocorticoid receptor inhibits hepatocellular carcinoma progression and the Warburg effect through regulating the miR-338-3p-PKLR axis (48). PKLR facilitates liver colonization by assisting glutathione synthesis in colorectal cancer (49). The levels of PKLR were up-regulated in RCC compared to normal tissues. Notably, we validated the patients with high levels of PFK or PFKM conferred a bad prognosis.
GAPDH is also a glycolytic key enzyme. GAPDH is involved in many cellular functions. For example, GAPDH contributes to nuclear tRNA export, DNA replication and repair, cellular metabolism, carcinogenesis, and cell death (50). Although GAPDH is widely used as an internal control, its expression status is different in different human cell lines (51). GAPDH levels have upregulated significantly and usually associated with reduced survival (52), while GAPDH is considered as a pro-apoptotic agent (53). Therefore, these dysregulations of GAPDH in cancers indicate the dual roles of this enzyme in cell fata. Indeed, as a glycolysis-related factors, GAPDH is essential for cancer cells. Here, we revealed that the patients who had high levels of GAPDH were correlated with reduced survival.
There are many uncertainties about the pathogenesis of RCC, and study of the biomarkers and mechanisms of RCC remains a global prevalence as the disease is on the rise. In this study, total 968 DEGs were filtrated combined with two kind of dataset containing the largest number of clinical specimens, comprising 516 increased regulated genes and 442 decreased regulated genes.
Conclusions
We found five genes that were overlapped in top 20 with the greatest FC in the two large human GEO dataset. ALDOB was identified as the only one gene that impacts on the prognosis of RCC patients. The under-expressed of ALDOB in human RCC has a novel predictive value for clinical outcome. Furthermore, several genes (GAPDH, PFKP, PFKM, PKLR, and FBP1) interacted with ALDOB also exhibited the prognostic value in patients.
Acknowledgments
Funding: The research received funding from
Footnote
Reporting Checklist: The authors have completed the REMARK reporting checklist. Available at https://tcr.amegroups.com/article/view/10.21037/tcr-22-2242/rc
Peer Review File: Available at https://tcr.amegroups.com/article/view/10.21037/tcr-22-2242/prf
Conflicts of Interest: All authors have completed the ICMJE uniform disclosure form (available at https://tcr.amegroups.com/article/view/10.21037/tcr-22-2242/coif). The authors have no conflicts of interest to declare.
Ethical Statement: The authors are accountable for all aspects of the work in ensuring that questions related to the accuracy or integrity of any part of the work are appropriately investigated and resolved. The study was conducted in accordance with the Declaration of Helsinki (as revised in 2013).
Open Access Statement: This is an Open Access article distributed in accordance with the Creative Commons Attribution-NonCommercial-NoDerivs 4.0 International License (CC BY-NC-ND 4.0), which permits the non-commercial replication and distribution of the article with the strict proviso that no changes or edits are made and the original work is properly cited (including links to both the formal publication through the relevant DOI and the license). See: https://creativecommons.org/licenses/by-nc-nd/4.0/.
References
- Li P, Znaor A, Holcatova I, et al. Regional geographic variations in kidney cancer incidence rates in European countries. Eur Urol 2015;67:1134-41. [Crossref] [PubMed]
- Ferlay J, Soerjomataram I, Dikshit R, et al. Cancer incidence and mortality worldwide: sources, methods and major patterns in GLOBOCAN 2012. Int J Cancer 2015;136:E359-86. [Crossref] [PubMed]
- Capitanio U, Montorsi F. Renal cancer. Lancet 2016;387:894-906. [Crossref] [PubMed]
- Li QK, Pavlovich CP, Zhang H, et al. Challenges and opportunities in the proteomic characterization of clear cell renal cell carcinoma (ccRCC): A critical step towards the personalized care of renal cancers. Semin Cancer Biol 2019;55:8-15. [Crossref] [PubMed]
- Pavlova NN, Thompson CB. The Emerging Hallmarks of Cancer Metabolism. Cell Metab 2016;23:27-47. [Crossref] [PubMed]
- DeBerardinis RJ, Chandel NS. Fundamentals of cancer metabolism. Sci Adv 2016;2:e1600200. [Crossref] [PubMed]
- DeBerardinis RJ, Lum JJ, Hatzivassiliou G, et al. The biology of cancer: metabolic reprogramming fuels cell growth and proliferation. Cell Metab 2008;7:11-20. [Crossref] [PubMed]
- Lameirinhas A, Miranda-Gonçalves V, Henrique R, et al. The Complex Interplay between Metabolic Reprogramming and Epigenetic Alterations in Renal Cell Carcinoma. Genes (Basel) 2019;10:264. [Crossref] [PubMed]
- Hanahan D, Weinberg RA. Hallmarks of cancer: the next generation. Cell 2011;144:646-74. [Crossref] [PubMed]
- Altenberg B, Greulich KO. Genes of glycolysis are ubiquitously overexpressed in 24 cancer classes. Genomics 2004;84:1014-20. [Crossref] [PubMed]
- Penhoet E, Rajkumar T, Rutter WJ. Multiple forms of fructose diphosphate aldolase in mammalian tissues. Proc Natl Acad Sci U S A 1966;56:1275-82. [Crossref] [PubMed]
- Dalby AR, Tolan DR, Littlechild JA. The structure of human liver fructose-1,6-bisphosphate aldolase. Acta Crystallogr D Biol Crystallogr 2001;57:1526-33. [Crossref] [PubMed]
- Chang YC, Chan YC, Chang WM, et al. Feedback regulation of ALDOA activates the HIF-1alpha/MMP9 axis to promote lung cancer progression. Cancer Lett 2017;403:28-36. [Crossref] [PubMed]
- Chen X, Yang TT, Zhou Y, et al. Proteomic profiling of osteosarcoma cells identifies ALDOA and SULT1A3 as negative survival markers of human osteosarcoma. Mol Carcinog 2014;53:138-44. [Crossref] [PubMed]
- Mukai T, Joh K, Arai Y, et al. Tissue-specific expression of rat aldolase A mRNAs. Three molecular species differing only in the 5'-terminal sequences. J Biol Chem 1986;261:3347-54. [Crossref] [PubMed]
- Singh A, Kukreti R, Saso L, et al. Oxidative Stress: A Key Modulator in Neurodegenerative Diseases. Molecules 2019;24:1583. [Crossref] [PubMed]
- Levine J, Kwon E, Paez P, et al. Traumatically injured astrocytes release a proteomic signature modulated by STAT3-dependent cell survival. Glia 2016;64:668-94. [Crossref] [PubMed]
- Sakakibara M, Mukai T, Yatsuki H, et al. Human aldolase isozyme gene: the structure of multispecies aldolase B mRNAs. Nucleic Acids Res 1985;13:5055-69. [Crossref] [PubMed]
- Rottmann WH, Deselms KR, Niclas J, et al. The complete amino acid sequence of the human aldolase C isozyme derived from genomic clones. Biochimie 1987;69:137-45. [Crossref] [PubMed]
- Tolan DR. Molecular basis of hereditary fructose intolerance: mutations and polymorphisms in the human aldolase B gene. Hum Mutat 1995;6:210-8. [Crossref] [PubMed]
- Ali M, Cox TM. Diverse mutations in the aldolase B gene that underlie the prevalence of hereditary fructose intolerance. Am J Hum Genet 1995;56:1002-5. [PubMed]
- Asaka M, Miyazaki T, Hollinger FB, et al. Human aldolase B serum levels: a marker of liver injury. Hepatology 1984;4:531-5. [Crossref] [PubMed]
- Asaka M, Kimura T, Meguro T, et al. Alteration of aldolase isozymes in serum and tissues of patients with cancer and other diseases. J Clin Lab Anal 1994;8:144-8. [Crossref] [PubMed]
- Malay AD, Allen KN, Tolan DR. Structure of the thermolabile mutant aldolase B, A149P: molecular basis of hereditary fructose intolerance. J Mol Biol 2005;347:135-44. [Crossref] [PubMed]
- Cline MJ, Slamon DJ, Lipsick JS. Oncogenes: implications for the diagnosis and treatment of cancer. Ann Intern Med 1984;101:223-33. [Crossref] [PubMed]
- Warburg O. On the origin of cancer cells. Science 1956;123:309-14. [Crossref] [PubMed]
- Weiss RH, Lin PY. Kidney cancer: identification of novel targets for therapy. Kidney Int 2006;69:224-32. [Crossref] [PubMed]
- Hu SL, Chang A, Perazella MA, et al. The Nephrologist's Tumor: Basic Biology and Management of Renal Cell Carcinoma. J Am Soc Nephrol 2016;27:2227-37. [Crossref] [PubMed]
- Hakimi AA, Reznik E, Lee CH, et al. An Integrated Metabolic Atlas of Clear Cell Renal Cell Carcinoma. Cancer Cell 2016;29:104-16. [Crossref] [PubMed]
- Motzer RJ, Bacik J, Mazumdar M. Prognostic factors for survival of patients with stage IV renal cell carcinoma: memorial sloan-kettering cancer center experience. Clin Cancer Res 2004;10:6302S-3S. [Crossref] [PubMed]
- Lincet H, Icard P. How do glycolytic enzymes favour cancer cell proliferation by nonmetabolic functions? Oncogene 2015;34:3751-9. [Crossref] [PubMed]
- Mamczur P, Gamian A, Kolodziej J, et al. Nuclear localization of aldolase A correlates with cell proliferation. Biochim Biophys Acta 2013;1833:2812-22. [Crossref] [PubMed]
- Caspi M, Perry G, Skalka N, et al. Aldolase positively regulates of the canonical Wnt signaling pathway. Mol Cancer 2014;13:164. [Crossref] [PubMed]
- Rhaleb NE, Yang XP, Carretero OA. The kallikrein-kinin system as a regulator of cardiovascular and renal function. Compr Physiol 2011;1:971-93. [Crossref] [PubMed]
- Huang H, Zhu L, Huang C, et al. Identification of Hub Genes Associated With Clear Cell Renal Cell Carcinoma by Integrated Bioinformatics Analysis. Front Oncol 2021;11:726655. [Crossref] [PubMed]
- Wang J, Wang X, Lin S, et al. Identification of kininogen-1 as a serum biomarker for the early detection of advanced colorectal adenoma and colorectal cancer. PLoS One 2013;8:e70519. [Crossref] [PubMed]
- Xu J, Fang J, Cheng Z, et al. Overexpression of the Kininogen-1 inhibits proliferation and induces apoptosis of glioma cells. J Exp Clin Cancer Res 2018;37:180. [Crossref] [PubMed]
- Nayak A, Pednekar L, Reid KB, et al. Complement and non-complement activating functions of C1q: a prototypical innate immune molecule. Innate Immun 2012;18:350-63. [Crossref] [PubMed]
- Zhang L, Jiang H, Xu G, et al. iTRAQ-based quantitative proteomic analysis reveals potential early diagnostic markers of clear-cell Renal cell carcinoma. Biosci Trends 2016;10:210-9. [Crossref] [PubMed]
- Omeis IA, Hsu YC, Perin MS. Mouse and human neuronal pentraxin 1 (NPTX1): conservation, genomic structure, and chromosomal localization. Genomics 1996;36:543-5. [Crossref] [PubMed]
- Hsu YC, Perin MS. Human neuronal pentraxin II (NPTX2): conservation, genomic structure, and chromosomal localization. Genomics 1995;28:220-7. [Crossref] [PubMed]
- Kirkpatrick LL, Matzuk MM, Dodds DC, et al. Biochemical interactions of the neuronal pentraxins. Neuronal pentraxin (NP) receptor binds to taipoxin and taipoxin-associated calcium-binding protein 49 via NP1 and NP2. J Biol Chem 2000;275:17786-92. [Crossref] [PubMed]
- Tsui CC, Copeland NG, Gilbert DJ, et al. Narp, a novel member of the pentraxin family, promotes neurite outgrowth and is dynamically regulated by neuronal activity. J Neurosci 1996;16:2463-78. [Crossref] [PubMed]
- Lee SJ, Wei M, Zhang C, et al. Presynaptic Neuronal Pentraxin Receptor Organizes Excitatory and Inhibitory Synapses. J Neurosci 2017;37:1062-80. [Crossref] [PubMed]
- von Roemeling CA, Radisky DC, Marlow LA, et al. Neuronal pentraxin 2 supports clear cell renal cell carcinoma by activating the AMPA-selective glutamate receptor-4. Cancer Res 2014;74:4796-810. [Crossref] [PubMed]
- Dunaway GA, Kasten TP, Sebo T, et al. Analysis of the phosphofructokinase subunits and isoenzymes in human tissues. Biochem J 1988;251:677-83. [Crossref] [PubMed]
- Wang J, Zhang P, Zhong J, et al. The platelet isoform of phosphofructokinase contributes to metabolic reprogramming and maintains cell proliferation in clear cell renal cell carcinoma. Oncotarget 2016;7:27142-57. [Crossref] [PubMed]
- Nie H, Li J, Yang XM, et al. Mineralocorticoid receptor suppresses cancer progression and the Warburg effect by modulating the miR-338-3p-PKLR axis in hepatocellular carcinoma. Hepatology 2015;62:1145-59. [Crossref] [PubMed]
- Nguyen A, Loo JM, Mital R, et al. PKLR promotes colorectal cancer liver colonization through induction of glutathione synthesis. J Clin Invest 2016;126:681-94. [Crossref] [PubMed]
- Colell A, Green DR, Ricci JE. Novel roles for GAPDH in cell death and carcinogenesis. Cell Death Differ 2009;16:1573-81. [Crossref] [PubMed]
- Sheokand N, Malhotra H, Kumar S, et al. Moonlighting cell-surface GAPDH recruits apotransferrin to effect iron egress from mammalian cells. J Cell Sci 2014;127:4279-91. [PubMed]
- Caradec J, Sirab N, Revaud D, et al. Is GAPDH a relevant housekeeping gene for normalisation in colorectal cancer experiments? Br J Cancer 2010;103:1475-6. [Crossref] [PubMed]
- Nakajima H, Amano W, Fujita A, et al. The active site cysteine of the proapoptotic protein glyceraldehyde-3-phosphate dehydrogenase is essential in oxidative stress-induced aggregation and cell death. J Biol Chem 2007;282:26562-74. [Crossref] [PubMed]