Nomogram for predicting preoperative axillary lymph node status in male breast carcinoma: a SEER population-based study
Highlight box
Key findings
• A model to predict ALNM was successfully established in MBC.
What is known and what is new?
• Many predictors of ALNM have been previously suggested in FBC.
• It can also predict ALNM by the preoperative clinical features in MBC.
What is the implication, and what should change now?
• This nomogram could serve as an acceptable and adoptable clinical tool in preoperative evaluation in MBC, especially for those who were clinically and radiologically node-negative to avoid an SLNB procedure safely. This can enhance the quality of life for patients without conceding the overall survival rate.
Introduction
Male breast carcinoma (MBC) is a rare disease that accounts for less than 1% of all cancers in men (1) and about less than 1% of all breast carcinoma (2). Due to its rarity, the limited information available regarding the prognosis of this disease, treatment, and epidemiology have been extrapolated from the protocols for breast carcinoma in women (3). Axillary lymph node (ALN) status is a key step in breast carcinoma because nodal status has been the standard of care to estimate prognosis and to guide the decision-making process for a systemic treatment plan. Many predictors of axillary lymph node metastasis (ALNM) have been previously suggested in female breast carcinoma (FBC) including age at diagnosis, tumor size, tumor location, pathological type, histologic grade, estrogen receptor (ER) status, progesterone receptor (PR) status, and HER2 status (4-7).
However, there are only a few predictive models for ALNM in MBC. Vaysse et al. (8) used two nomograms (validated in the female population) to demonstrate that the predictive factor of ALNM in FBC was not applicable to MBC. The possibility of building a prediction model of ALNM in MBC is hypothesized; of which can help identify patients with low risk of ALN involvement so as to improve the quality of life with no adverse effects on the survival rate. To verify the hypothesis, this study of applying the data from the Surveillance, Epidemiology, and End Results (SEER) database was conducted to explore the relationship between clinicopathologic features and ALN status. We present the following article in accordance with the TRIPOD reporting checklist (available at https://tcr.amegroups.com/article/view/10.21037/tcr-22-2516/rc).
Methods
Data were obtained from (SEER) (www.seer.cancer.gov) with the following search parameters. Incidence: SEER Research Data, 18 Registries, November 2020 Sub (2000 to 2018). The ethical approval of this study was exempted by the Ethics Committee of Affiliated Sanming First Hospital of Fujian Medical University as the data were from the publicly accessible database, SEER. The study was conducted in accordance with the Declaration of Helsinki (as revised in 2013).
A total of 2,610 male patients were selected according to the following inclusion and exclusion criteria between 2010 and 2018 in the investigation. Patients were included when meeting the following criteria: (I) male patients; (II) patients were diagnosed with primary breast cancer [International Classification of Diseases for Oncology, Third Edition (ICD-O-3)]; (III) patients aged 18 years old or above at diagnosis. Patients with the following criteria were excluded: (I) unknown clinical information including tumor size, American Joint Committee on Cancer (AJCC) stage, Tumor location (primary site-labeled), Pathological type, Histologic grade, estrogen receptor (ER) status, progesterone receptor (PR) status, and HER2 status; (II) regional nodes examined positive; (III) with metastatic or synchronous tumors.
The selected patients were randomly assigned in a 2:1 ratio to a training cohort and a validation cohort. The training cohort was used to construct the predictive risk model, and the validation cohort was applied to internal validation.
Variables including age at diagnosis, tumor location, AJCC tumor node metastasis (TNM) stage, AJCC T status, AJCC N status, pathological type, histologic grade, ER status, PR status, and HER2 status were gathered from the SEER database. Age was classified into four groups: ‘≤50’, ‘51–60’, ‘61–70’, ‘71–80’, and ‘>80’. Tumor location was classified as central portion of breast (Central), upper outer quadrant (UOQ), lower outer quadrant (LOQ), lower inner quadrant (LIQ), and upper inner quadrant (UIQ), nipple, overlapping lesion of breast (Overlapping), axillary tail of breast and Breast NOS (other). The pathological type was classified as invasive ductal carcinoma (IDC), invasive lobular carcinoma (ILC), adenocarcinomas (ADENO-CA), Paget’s disease (Paget), and others. ER, PR and HER2 were all classified as positive and negative (note: the SEER database began collecting HER-2 information in 2010). Histologic grade was classified as grades I, II, and III. AJCC TNM Stage was classified as stages I, II, and III. AJCC Tumor Status was classified as T1, T2, T3, and T4. AJCC Lymph Node Status was classified as positive and negative. The molecular subtype was classified as Luminal A, Luminal B, HER2+, and triple-negative (TN).
Statistical analysis
Baseline characteristics between the training cohort and the validation cohort were identified by using Pearson’s Chi-square or Fisher’s exact tests for categorical variables and Student’s t-test for quantitative variables. Using the clinical and pathological data of the training cohort, a univariate logistic regression analysis was performed to explore ALNM-related variables. To identify factors that were associated with ALN involvement, binary logistic regression analysis was used for multivariable analysis. Odds ratios (OR) were presented with 95% CI. A logistic regression model was used to structure the nomogram. The receiver operating characteristic (ROC) curve, the area under the curve (AUC), and the concordance index (C-index) were used to distinguish the effect of the prediction ability of the model. Calibration was assessed graphically by plotting the relationship between actual (observed) probabilities and predicted probabilities in both cohorts. Internal validation of performance was estimated with the bootstrapping method (1,000 replications). To further evaluate the clinical value of the model, certain cut-off values were considered for predicting the risk in patients in the validation cohort and the corresponding accuracy and the false-negative rate of the cut-off values were calculated to assess the screening indicators of patients with low ALNM risk. Statistical analyses were conducted using SPSS for Windows (version 26.0, SPSS Inc., Chicago, IL, USA) and the R programming language and environment version 3.4.1. A two-tailed P value <0.05 was considered statistically significant.
Results
Comparison of clinicopathological characteristics between the training cohort and the validation cohort
Based on the inclusion criteria, A total of 2,610 patients who had well-documented patient data were identified for analysis. According to the ratio of 2:1, 1,740 were included in the training cohort and 870 were included in the validation cohort. The clinical and pathological data of the patients between the two groups did not differ significantly (P>0.05) (Table 1).
Table 1
Characteristics | Training (n=1,740) | Validation (n=870) | P value |
---|---|---|---|
Age at diagnosis (years), mean ± SD | 67.57±11.70 | 67.64±12.03 | 0.895 |
Age (years), n (%) | 0.542 | ||
≤50 | 137 (7.9) | 79 (9.1) | |
51–60 | 336 (19.3) | 147 (16.9) | |
61–70 | 539 (31.0) | 269 (30.9) | |
71–80 | 456 (26.2) | 233 (26.8) | |
>80 | 272 (15.6) | 142 (16.3) | |
Tumor location#, n (%) | 0.716 | ||
Central | 744 (42.8) | 403 (46.3) | |
UIQ | 82 (4.7) | 35 (4.0) | |
LIQ | 32 (1.8) | 14 (1.6) | |
UOQ | 222 (12.8) | 100 (11.5) | |
LOQ | 65 (3.7) | 35 (4.0) | |
Nipple | 79 (4.5) | 39 (4.5) | |
Others | 228 (13.1) | 100 (11.5) | |
Overlapping | 288 (16.6) | 144 (16.6) | |
Tumor stage, n (%) | 0.808 | ||
T1 | 866 (49.8) | 421 (48.4) | |
T2 | 704 (40.5) | 360 (41.4) | |
T3 | 47 (2.7) | 21 (2.4) | |
T4 | 123 (7.1) | 68 (7.8) | |
Pathological type, n (%) | 0.334 | ||
IDC | 1,582 (90.9) | 789 (90.7) | |
ILC | 14 (0.8) | 8 (0.9) | |
ADENO-CA | 92 (5.3) | 39 (4.5) | |
Paget | 20 (1.1) | 8 (0.9) | |
Others* | 32 (1.8) | 26 (3.0) | |
Histologic grade, n (%) | 0.613 | ||
I | 251 (14.4) | 124 (14.3) | |
II | 936 (53.8) | 453 (52.1) | |
III | 553 (31.8) | 293 (33.7) | |
ER, n (%) | 0.620 | ||
Positive | 1,707 (98.1) | 851 (97.8) | |
Negative | 33 (1.9) | 19 (2.2) | |
PR, n (%) | 0.724 | ||
Positive | 1,595 (91.7) | 801 (92.1) | |
Negative | 145 (8.3) | 69 (7.9) | |
HER2 receptor status, n (%) | 0.899 | ||
Positive | 215 (12.4) | 106 (12.2) | |
Negative | 1,525 (87.6) | 764 (87.8) | |
AJCC stage, n (%) | 0.930 | ||
I | 837 (48.1) | 412 (47.4) | |
II | 626 (36.0) | 319 (36.7) | |
III | 277 (15.9) | 129 (16.0) | |
Lymph node status, n (%) | 0.976 | ||
Negative | 1,179 (67.8) | 590 (67.8) | |
Positive | 561 (32.2) | 280 (32.2) | |
Molecular subtype, n (%) | 0.730 | ||
Luminal A | 1,502 (86.3) | 748 (86.0) | |
Luminal B | 209 (12.0) | 104 (12.0) | |
HER2+ | 6 (0.3) | 2 (0.2) | |
TN | 23 (1.3) | 16 (1.8) |
#, tumor location: in cases of multifocal tumors or unifocal tumors that involved more than 1 quadrant, the tumor location was classified in the following order of location priority: central portion of breast, UOQ, LOQ, LIQ, and UIQ, nipple, overlapping lesion of breast (overlapping), Axillary tail of breast and Breast NOS (other). *, others: metaplastic carcinoma, medullary carcinoma, unspecified malignant neoplasms except for CNS, papillary carcinoma, infiltrating micropapillary carcinoma, tubular carcinoma, etc. (https://seer.cancer.gov/tools/solidtumor/Breast_STM.pdf). UIQ, upper inner quadrant; LIQ, lower inner quadrant; UOQ, upper outer quadrant; LOQ, lower outer quadrant; IDC, invasive ductal carcinoma; ILC, invasive lobular carcinoma; ADENO-CA, adenocarcinomas; ER, estrogen receptor; PR, progesterone receptor; TN, triple-negative; AJCC, American Joint Committee on Cancer; CNS, central nervous system.
Univariate logistic regression analysis of ALNM in the training cohort
Univariate logistic regression analysis was used to probe ALNM-related variables (Table 2) and showed that age, tumor location, tumor stage, pathological type, histologic grade, ER, PR, Her-2, and molecular subtypes were related to MBC ALNM (P<0.05).
Table 2
Variables | OR | 95% CI | P value |
---|---|---|---|
Age (years) | <0.001 | ||
≤50 | 1 (ref) | ||
51–60 | 0.896 | 0.601–1.337 | 0.592 |
61–70 | 0.572 | 0.390–0.838 | 0.004 |
71–80 | 0.452 | 0.304–0.670 | <0.001 |
>80 | 0.335 | 0.215–0.522 | <0.001 |
Tumor location | <0.001 | ||
Central | 1 (ref) | ||
UIQ | 0.380 | 0.216–0.668 | 0.001 |
LIQ | 0.361 | 0.147–0.888 | 0.027 |
UOQ | 0.528 | 0.377–0.740 | <0.001 |
LOQ | 0.600 | 0.3411–1.053 | 0.075 |
Nipple | 0.859 | 0.530–1.395 | 0.540 |
Other | 0.723 | 0.527–0.991 | 0.044 |
Overlapping | 0.551 | 0.408–0.745 | <0.001 |
Tumor stage | <0.001 | ||
T1 | 1 (ref) | ||
T2 | 6.065 | 4.703–7.823 | <0.001 |
T3 | 24.513 | 12.099–49.667 | <0.001 |
T4 | 53.929 | 30.248–96.150 | <0.001 |
Pathological type | 0.002 | ||
IDC | 1 (ref) | ||
ILC | 0.801 | 0.250–2.565 | 0.708 |
ADENO-CA | 0.359 | 0.201–0.641 | 0.001 |
Paget | 2.002 | 0.828–4.840 | 0.123 |
Other | 0.462 | 0.189–1.129 | 0.090 |
Histologic grade | <0.001 | ||
I | 1 (ref) | ||
II | 3.065 | 1.977–4.752 | <0.001 |
III | 10.642 | 6.815–16.617 | <0.001 |
ER | |||
ER+ | 1 (ref) | ||
ER− | 2.271 | 1.139–4.530 | 0.020 |
PR | |||
PR+ | 1 (ref) | ||
PR− | 1.541 | 1.089–2.181 | 0.015 |
HER2 | |||
HER2+ | 1 (ref) | ||
HER2− | 0.544 | 0.407–0.727 | <0.001 |
Molecular subtype | <0.001 | ||
LMA | 1 (ref) | ||
LMB | 1.809 | 1.348–2.429 | <0.001 |
HER2 | 4.602 | 0.840–25.216 | 0.079 |
TN | 1.770 | 0.771–4.066 | 0.178 |
ALNM, axillary lymph node metastasis; OR, odds ratio; CI, confidence interval; UIQ, upper inner quadrant; LIQ, lower inner quadrant; UOQ, upper outer quadrant; LOQ, lower outer quadrant; IDC, invasive ductal carcinoma; ILC, invasive lobular carcinoma; ADENO-CA, adenocarcinomas; ER, estrogen receptor; PR, progesterone receptor; TN, triple-negative.
Multivariate logistic regression analysis of ALNM in the training group
After adjusting the significant variables from the univariate analysis, the multivariate analysis found that age at diagnosis, tumor location, tumor stage, pathological type, and histologic grade stayed as independent predictive factors of ALNM (Table 3).
Table 3
Variables | OR | 95% CI | P value |
---|---|---|---|
Age (years) | <0.001 | ||
≤50 | 1 (ref) | ||
51–60 | 0.830 | 0.503–1.368 | 0.464 |
61–70 | 0.441 | 0.275–0.710 | 0.001 |
71–80 | 0.372 | 0.228–0.607 | <0.001 |
>80 | 0.198 | 0.113–0.347 | <0.001 |
Tumor location | 0.001 | ||
Central | 1 (ref) | ||
UIQ | 0.508 | 0.264–0.975 | 0.042 |
LIQ | 0.493 | 0.183–1.332 | 0.163 |
UOQ | 0.603 | 0.400–0.909 | 0.016 |
LOQ | 0.615 | 0.305–1.239 | 0.174 |
Nipple | 0.631 | 0.328–1.212 | 0.167 |
Other | 0.522 | 0.352–0.776 | 0.001 |
Overlapping | 0.497 | 0.342–0.722 | <0.001 |
Tumor stage | <0.001 | ||
T1 | 1 (ref) | ||
T2 | 5.877 | 4.463–7.739 | <0.001 |
T3 | 25.211 | 11.632–54.643 | <0.001 |
T4 | 60.418 | 32.409–112.631 | <0.001 |
Pathological type | 0.024 | ||
IDC | 1 (ref) | ||
ILC | 0.405 | 0.090–1.832 | 0.241 |
ADENO-CA | 0.355 | 0.176–0.716 | 0.004 |
Paget | 1.512 | 0.457–4.997 | 0.498 |
Other | 0.542 | 0.186–1.577 | 0.261 |
Histologic grade | <0.001 | ||
I | 1 (ref) | ||
II | 2.569 | 1.555–4.245 | <0.001 |
III | 7.240 | 4.335–12.093 | <0.001 |
Constant | 0.126 | <0.001 |
ALNM, axillary lymph node metastasis; OR, odds ratio; CI, confidence interval; UIQ, upper inner quadrant; LIQ, lower inner quadrant; UOQ, upper outer quadrant; LOQ, lower outer quadrant; IDC, invasive ductal carcinoma; ILC, invasive lobular carcinoma; ADENO-CA, adenocarcinomas.
Establishment of a prediction model for ALNM
A nomogram to predict ALNM was developed in the training cohort (Figure 1). The nomogram that incorporated the following independent predictors was shown to be associated with ALNM including age, pathological type, tumor location, histologic grade, and tumor stage. The weights of each variable in the nomogram corresponded to different points. Points for the following factors were added to the total points, which corresponded to the linear predictors and risk predictors of ALNM: age, histological (pathological type), Site (tumor location), Grade (histologic grade), and T-stage (tumor stage).
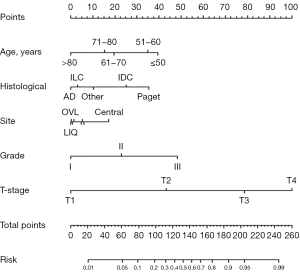
ROC curves of the training cohort are plotted in Figure 2A. AUC was 0.846 (95% CI: 0.825–0.867). The nomogram verified excellent accuracy for predicting ALN, with an unadjusted C-index of 0.848 (95% CI: 0.807–0.889). To test the prediction efficiency of the nomogram, 1,000 bootstrap resamplings were executed for verification through the calibration chart in the training cohort (Figure 2B). The calibration curve displayed a marked effect of the nomogram. These results testified that the nomogram was a suitable predictor of ALNM.
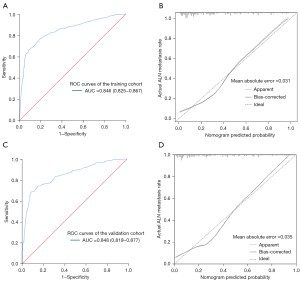
The predictive ability of nomograms in the validation cohort
This nomogram was prospectively used for patients in the validation cohort. It depicted the ROC curve (Figure 2C), and the AUC value calculated was 0.848 (95% CI: 0.819–0.877), indicating a good predictive ability. The nomogram was internally verified using the bootstrap (1,000 bootstrap resamples) validation method. Good calibration was observed for the probability of ALNM in the validation cohort (Figure 2D). To further evaluate the clinical value of the model under different risks of ALNM. We select 14 cut-offs according to the Jordan index. The number of patients, the number of patients with ALNM, sensitivity, specificity, accuracy, and negative predictive value of ALNM were calculated under different predicted risk values (Table 4). As can be seen from the table, this model is more accurate in predicting patients with negative ALN. There were 204 cases with a predicted risk of <10%, and only 14 (6.9%) had ALNM, indicating that the model was more accurate in predicting patients with a lower risk of ALNM. The reason for this result is related to the study design. Both the training cohort and the validation group were patients with clinically negative ALN, resulting in a more accurate prediction of negative lymph nodes and a poorer prediction of positive lymph nodes.
Table 4
Case No. | Predicted risk | No. of patients (%) | No. of patients with ALNM | Sensitivity (%) | Specificity (%) | Accuracy (%) | Negative predictive value (%) |
---|---|---|---|---|---|---|---|
1 | <0.051 | 76 (8.74) | 2 | 99.30 | 87.50 | 97.37 | 97.37 |
2 | <0.100 | 204 (23.45) | 14 | 95.00 | 67.80 | 93.14 | 93.14 |
3 | <0.150 | 307 (35.29) | 30 | 89.30 | 53.10 | 90.23 | 90.23 |
4 | <0.202 | 369 (42.41) | 39 | 86.10 | 44.10 | 89.43 | 89.43 |
5 | <0.248 | 437 (50.23) | 54 | 80.70 | 35.10 | 87.64 | 87.64 |
6 | <0.299 | 496 (57.01) | 62 | 77.90 | 26.40 | 87.50 | 87.50 |
7 | <0.353 | 553 (63.56) | 71 | 74.60 | 18.30 | 87.16 | 87.16 |
8 | <0.400 | 568 (65.29) | 76 | 72.90 | 16.60 | 86.62 | 86.62 |
9 | <0.452 | 616 (70.80) | 90 | 67.90 | 10.80 | 85.39 | 85.39 |
10 | <0.501 | 642 (73.79) | 94 | 66.40 | 7.10 | 85.36 | 85.36 |
11 | <0.603 | 708 (81.38) | 144 | 48.60 | 4.40 | 84.46 | 85.36 |
12 | <0.696 | 764 (87.82) | 189 | 32.50 | 2.50 | 84.16 | 85.36 |
13 | <0.803 | 792 (91.03) | 213 | 23.90 | 1.90 | 84.22 | 85.36 |
14 | <0.899 | 817 (93.91) | 230 | 17.90 | 0.50 | 83.72 | 85.36 |
ALNM, axillary lymph node metastasis.
Discussion
Sentinel lymph node biopsy (SLNB) has been recommended as a replacement for axillary dissection in MBC with clinical ALN-negative, as with the case in women (9). However, the morbidity after SLN biopsy is not negligible, short-term or long-term complications such as upper limb edema, numbness, and shoulder pain may also occur in FBC patients with SLNB (10,11). MBC usually has the same common presentation as FBC. Many models for predicting ALNM have been used in women with breast carcinoma (12,13). This study aimed to select patients with low risk of ALNM and to protect them from unnecessary axillary operations as in the case of women.
Most studies about MBC come with only small sample sizes as MBC is rarely seen, in view of this, the SEER database supplies a relatively large sample size for the study. In the study, we obtained 2,610 cases from the SEER database. The results provided evidence that analyzing the clinicopathological features can also be used to assist in the judgment of ALN status in MBC. A nomogram was successfully established for the prediction of ALNM in MBC. Age, pathological type, tumor location, histologic grade, and tumor stage were associated with ALNM. Applying this model to patients in the training cohort and the validation cohort in this study, the performance of the nomogram in these two groups was similar (AUC 0.846 vs. 0.848), and the nomogram showed good predictive value in both.
Zavagno et al. (14) findings showed that the youngest patients had the worst prognostic pattern, which improves as age increases, and is the best in patients over 75 years of age by looking at the influence of age on the pathologic and biological features of breast cancer. Many previous studies showed that young age was independently associated with a higher likelihood of one or more positive lymph nodes in FBC (15,16). There are few reports on the influence of age on ALNM in MBC. The research showed that age at diagnosis was an independent prognostic factor for the risk of ALNM in MBC, and the risk decreased with age.
The pathological types of MBC are mainly IDC, lobular carcinoma is rare, and other types, such as adenocarcinoma, papillary carcinoma, and Paget’s disease, have also been reported (2). Many researchers (12,15) have found that favorable histotypes (medullary, cribriform, tubular, mucinous) have a significantly lower risk of ALNM than ductal carcinoma in FBC. There is also little research on the relationship between pathological types and ALNM in MBC. The research also found that other pathological types of MBC, such as lobular carcinoma and adenocarcinoma, were less prone to ALNM than IDC. Paget’s disease had the highest risk of ALNM in MBC. Due to the lack of other clinical and pathological data provided by the SEER database, the causes were unable to further explore, which may be related to delayed treatment due to the patient’s misdiagnosed breast cancer as eczema of the nipple. Another reason may be the abundance of lymphatic vessels in the nipple and areola area, which is more prone to lymph node metastasis.
MBC is mostly manifested as a mass in the areola area, while the male breast is significantly smaller than the female breast. The tumor is close to the skin, nipple, areola, and chest wall. When the tumor occurs, the mammary lymphatic drainage system is easily being invaded at early stage and this leads to lymph node metastasis (17). Yang et al. (18) confirmed that central location was an independent predictor of ALNM in MBC. Tumors located in the central region are more likely to have ALNM than in other regions. The results also showed that lymph node metastasis was less likely to occur in tumors located elsewhere than in the central region.
The increasing tumor grade is associated with a higher risk for ALNM in FBC. This fact has been confirmed in some studies (16,19). A few studies (18-20) have found that the risk of ALNM increases with histological grade in MBC. It can be seen from the univariate analysis of our research that Grade 3 was associated with a 6.240-fold greater risk for lymphatic metastasis than grade 1, while grade 2 versus grade 1 had a 1.569-fold risk.
The T-stage is an objective performance criterium of the growth time and growth rate of the tumor. T-stage emerged as the most powerful independent predictor of SLNM. This fact has been confirmed in some research in FBC (20,21). Some researchers (22,23) support the opinion that the advanced tumor stage is associated with a higher risk of death in MBC. Due to the low incidence of MBC, the relationship between T-stage and ALNM is also rarely researched. The results showed that T-stage played a crucial role in ALNM in MBC. The risk of ALNM increases significantly with T-stage.
Although the nomogram showed conspicuously accuracy for predicting ALNM, there were still some limitations in the present study. First, the data analysis was based on SEER, with a lack of centralized pathology review, and limited information regarding the surgery. In addition, some important clinical information such as preoperative ultrasound and imaging examination, as well as some immunohistochemical index such as Ki67, was lacking. Second, the use of retrospective data introduced the possibility of selection bias. Third, the vast majority of patients in this study were white, so predictions for non-white patients might be less accurate. Prospective investigations and more in-depth research were needed to help establish the results.
Conclusions
In conclusion, this nomogram can be used as a suitable and applicable clinical tool for preoperative evaluation of MBC, especially for those who are of advanced age at diagnosis, have small tumor size, low malignancy, and are radiologically node-negative, to avoid the unnecessary axillary operation before sentinel lymph node biopsy. This can enhance the quality of life for patients without conceding the overall survival rate.
Acknowledgments
Funding: This work was supported by
Footnote
Reporting Checklist: The authors have completed the TRIPOD reporting checklist. Available at https://tcr.amegroups.com/article/view/10.21037/tcr-22-2516/rc
Peer Review File: Available at https://tcr.amegroups.com/article/view/10.21037/tcr-22-2516/prf
Conflicts of Interest: All authors have completed the ICMJE uniform disclosure form (available at https://tcr.amegroups.com/article/view/10.21037/tcr-22-2516/coif). The authors have no conflicts of interest to declare.
Ethical Statement: The authors are accountable for all aspects of the work and in ensuring that questions related to the accuracy or integrity of any part of the work are appropriately investigated and resolved. The ethical approval of this study was exempted by the Ethics Committee of Affiliated Sanming First Hospital of Fujian Medical University as the data were from the publicly accessible database, SEER. The study was conducted in accordance with the Declaration of Helsinki (as revised in 2013).
Open Access Statement: This is an Open Access article distributed in accordance with the Creative Commons Attribution-NonCommercial-NoDerivs 4.0 International License (CC BY-NC-ND 4.0), which permits the non-commercial replication and distribution of the article with the strict proviso that no changes or edits are made and the original work is properly cited (including links to both the formal publication through the relevant DOI and the license). See: https://creativecommons.org/licenses/by-nc-nd/4.0/.
References
- Siegel RL, Miller KD, Fuchs HE, et al. Cancer Statistics, 2021. CA Cancer J Clin 2021;71:7-33. [Crossref] [PubMed]
- Giordano SH, Cohen DS, Buzdar AU, et al. Breast carcinoma in men: a population-based study. Cancer 2004;101:51-7. [Crossref] [PubMed]
- Hassett MJ, Somerfield MR, Baker ER, et al. Management of Male Breast Cancer: ASCO Guideline. J Clin Oncol 2020;38:1849-63. [Crossref] [PubMed]
- Fehm T, Maul H, Gebauer S, et al. Prediction of axillary lymph node status of breast cancer patients by tumorbiological factors of the primary tumor. Strahlenther Onkol 2005;181:580-6. [Crossref] [PubMed]
- Reyal F, Rouzier R, Depont-Hazelzet B, et al. The molecular subtype classification is a determinant of sentinel node positivity in early breast carcinoma. PLoS One 2011;6:e20297. [Crossref] [PubMed]
- Guarnieri A, Neri A, Correale PP, et al. Prediction of lymph node status by analysis of prognostic factors and possible indications for elective axillary dissection in T1 breast cancers. Eur J Surg 2001;167:255-9. [Crossref] [PubMed]
- Yip CH, Taib NA, Tan GH, et al. Predictors of axillary lymph node metastases in breast cancer: is there a role for minimal axillary surgery? World J Surg 2009;33:54-7. [Crossref] [PubMed]
- Vaysse C, Sroussi J, Mallon P, et al. Prediction of axillary lymph node status in male breast carcinoma. Ann Oncol 2013;24:370-6. [Crossref] [PubMed]
- Gentilini O, Chagas E, Zurrida S, et al. Sentinel lymph node biopsy in male patients with early breast cancer. Oncologist 2007;12:512-5. [Crossref] [PubMed]
- Del Bianco P, Zavagno G, Burelli P, et al. Morbidity comparison of sentinel lymph node biopsy versus conventional axillary lymph node dissection for breast cancer patients: results of the sentinella-GIVOM Italian randomised clinical trial. Eur J Surg Oncol 2008;34:508-13. [Crossref] [PubMed]
- Rönkä R, von Smitten K, Tasmuth T, et al. One-year morbidity after sentinel node biopsy and breast surgery. Breast 2005;14:28-36. [Crossref] [PubMed]
- Zhang J, Li X, Huang R, et al. A nomogram to predict the probability of axillary lymph node metastasis in female patients with breast cancer in China: A nationwide, multicenter, 10-year epidemiological study. Oncotarget 2017;8:35311-25. [Crossref] [PubMed]
- Silverstein MJ, Skinner KA, Lomis TJ. Predicting axillary nodal positivity in 2282 patients with breast carcinoma. World J Surg 2001;25:767-72. [Crossref] [PubMed]
- Zavagno G, Meggiolaro F, Pluchinotta A, et al. Influence of age and menopausal status on pathologic and biologic features of breast cancer. Breast 2000;9:320-8. [Crossref] [PubMed]
- Viale G, Zurrida S, Maiorano E, et al. Predicting the status of axillary sentinel lymph nodes in 4351 patients with invasive breast carcinoma treated in a single institution. Cancer 2005;103:492-500. [Crossref] [PubMed]
- Rivadeneira DE, Simmons RM, Christos PJ, et al. Predictive factors associated with axillary lymph node metastases in T1a and T1b breast carcinomas: analysis in more than 900 patients. J Am Coll Surg 2000;191:1-6; discussion 6-8. [Crossref] [PubMed]
- Meng XL, Xie SN. Analysis of prognostic factors in male breast cancer patients. Chin J Clin Oncol 2002;29:74-5.
- Yang H, Chen M, Yang G, et al. Analysis of related factors of axillary lymph node metastasis in male breast cancer patients based on SEER database. Journal of Modern Oncology 2020;28:66-9.
- Sandoughdaran S, Malekzadeh M, Mohammad Esmaeil ME. Frequency and Predictors of Axillary Lymph Node Metastases in Iranian Women with Early Breast Cancer. Asian Pac J Cancer Prev 2018;19:1617-20. [PubMed]
- Wada N, Imoto S, Yamauchi C, et al. Predictors of tumour involvement in remaining axillary lymph nodes of breast cancer patients with positive sentinel lymph node. Eur J Surg Oncol 2006;32:29-33. [Crossref] [PubMed]
- Cutuli B, Velten M, Martin C. Assessment of axillary lymph node involvement in small breast cancer: analysis of 893 cases. Clin Breast Cancer 2001;2:59-65; discussion 66. [Crossref] [PubMed]
- Sun B, Zhang LN, Zhang J, et al. The prognostic value of clinical and pathologic features in nonmetastatic operable male breast cancer. Asian J Androl 2016;18:90-5. [Crossref] [PubMed]
- Foerster R, Foerster FG, Wulff V, et al. Matched-pair analysis of patients with female and male breast cancer: a comparative analysis. BMC Cancer 2011;11:335. [Crossref] [PubMed]