Establishment and validation of nomograms for predicting survival of lung invasive adenocarcinoma based on the level of pathological differentiation: a SEER cohort-based analysis
Highlight box
Key findings
• This study analyzed clinicopathological features of invasive adenocarcinoma (IAC) and investigated the risk factors associated with prognosis. Nomogram models of different pathological stages of IAC were established to predict prognosis.
What is known and what is new?
• Nomograms are widely used in various tumors, and prediction models for IAC are emerging in an endless stream.
• The relationship between pathological differentiation and other clinicopathologic factors was analyzed in this study. IAC and the variants were classified into three groups: high, medium, and low differentiation, and models of each group were constructed respectively.
What is the implication, and what should change now?
• This study highlights the importance of pathological differentiation in IAC survival and provides clinical nomograms for IAC patients with different pathologies. The pathogenesis and genomic changes of IAC with different histology might be further explored in the future.
Introduction
Lung cancer remains the main reason for cancer-related death on a global scale and lung adenocarcinoma (LUAD) is the most common pathological type (1). To ameliorate this major global health problem, further research is needed to focus on the prognosis. Many reports were published on prognostic risk factors for LUAD: factors like age, sex, race, tumor-node-metastasis (TNM) stage, pathological grade, marital status, and therapeutic regimen are closely related to survival. The age of the patients is a confirmed risk factor for LUAD, and it always affects the type of therapeutic strategy (2,3). The majority of studies consider females are more likely to have a better prognosis, which may relate to the estrogen receptor beta (ER-β) pathway (2,4-7). The race is also considered to be an independent prognostic factor for LUAD, with Asians, Pacific Islanders, and Hispanics experiencing better survival (8,9). In tons of former studies, TNM stage, pathological grade, and therapeutic regimen are used as prognostic factors of LUAD, thus almost all lung cancer nomograms include these three factors (3,7,10-14). There are 4 histologic subtypes of LUAD according to the 2015 WHO classification: preinvasive lesions, minimally invasive adenocarcinoma (MIA), invasive adenocarcinoma (IAC), and adenocarcinoma variants (15,16). Compared with preinvasive lesions and MIA, five subtypes of IAC and its variants portend a significantly worse prognosis. But whether these prognostic factors affecting LUAD still have effects on IAC and its variants has not been confirmed.
The International Association for the Study of Lung Cancer (IASLC) pathology panel established a set of histologic standards to assess the outcomes of lung cancer. Invasive pulmonary adenocarcinoma was divided into three types according to the degree of differentiation: grade 1, lepidic predominant tumor only; grade 2, acinar or papillary predominant tumor; and grade 3, solid, micropapillary, or complex gland (including variants) (17). Motivated by this, the pathological types were classified into three broad categories based on the grade of differentiation: high differentiation (lepidic adenocarcinoma only; LEP), moderate differentiation (acinar adenocarcinoma or papillary adenocarcinoma; ACI/PAP) and low differentiation (micropapillary adenocarcinoma, solid adenocarcinoma or variants; MIP/SOL).
Contemporary therapeutic decisions for IAC patients are often based on the TNM stage, which does not account for different pathological subtypes. Several recent studies have constructed predicting nomogram models based on specific pathological subtypes such as invasive mucinous adenocarcinoma (18-20) and PAP (21). However, these models which focus on only one pathological type are not widely used. Some researchers have established a prognostic model totally based on pathologic features for IAC, which showed passable discriminative ability (22). The predictive accuracy of the models was satisfactory, nevertheless, they just considered the role of IAC pathology, which limited the scope of the model’s application. There were also no established nomograms to estimate the outcome of IAC patients including multiple variants. So, it is warranted to analyze the predictive value of pathological differentiation combined with other clinical predictors.
This study was to analyze clinicopathological features of IAC and investigate the risk factors associated with prognosis. Furthermore, the relationship between pathological differentiation and other risk factors were probed. Nomogram models were established to better predict IAC prognosis. We present the following article in accordance with the TRIPOD reporting checklist (available at https://tcr.amegroups.com/article/view/10.21037/tcr-22-2308/rc).
Methods
Study design
This study was to explore the mechanism by which the pathological differentiation affects IAC outcomes and establish differentiation-specific nomograms. By reviewing the data of the Surveillance, Epidemiology, and End Results (SEER) database, the overall characteristics of IAC patients could be obtained. All patients would be randomly divided in a ratio of 7:3 into a training cohort and a validation cohort. The training cohort was to screen risk factors of IAC and establish differentiation-specific nomograms. The training cohort was utilized for internal validation and the validation cohort was used for external validation. In view of the data collected from the SEER database, the follow-up procedures would be omitted. The methodology each step was described in detail in following subsections.
Data source and cohorts selection
The patient data were gathered from the SEER public use database SEER 8 Regs Custom Data, Nov 2021 Sub (1975–2019), which collects clinicopathologic data of cancer patients, including demographics, tumor pathology, stage at diagnosis, treatment protocols, and survival from 1975 to 2019.
IAC patients between 1975 and 2019 were identified using SEER*Stat software (version 8.4.0). The inclusion criteria included two items: tumor primary sites and histologic ICD-O-3 codes. The primary sites were employed to identify the following sites: C34.0-Main bronchus; C34.1-Upper lobe, lung; C34.2-Middle lobe, lung; C34.3-Lower lobe, lung; C34.8-Overlapping lesion of lung; C34.9-Lung, NOS. The histologic ICD-O-3 codes (International Classification of Diseases for Oncology, Third Edition) were employed to identify the following subtypes: lepidic adenocarcinoma (ICD-O-3 code 8250), ACI (ICD-O-3 code 8551), PAP (ICD-O-3 code 8260), MIP (ICD-O-3 code 8265), SOL (ICD-O-3 code 8230), invasive mucinous adenocarcinoma (ICD-O-3 code 8253), mixed invasive mucinous and nonmucinous adenocarcinoma (ICD-O-3 code 8254), colloid adenocarcinoma (ICD-O-3 code 8480), fetal adenocarcinoma (ICD-O-3 code 8333), and enteric adenocarcinoma (ICD-O-3 code 8144).
The exclusion criteria were as follows: (I) no histological confirmation was found for the diagnosis; (II) the survival time was unknown or less than one month; (III) multiple primary cancers were diagnosed but IAC was not the first one; (IV) type of reporting source was autopsy or death certificate; (V) disease-related detail is missing, such as unknown age, gender, race, grade, TNM stage, and so on; (VI) therapeutic regimen is missing.
Final results revealed 4,418 patients with IAC met the criteria for inclusion and exclusion. For further analysis, 70% of screened patients were randomly divided into a training cohort and 30% were classified into a validation cohort to externally verify the final nomogram (Figure 1). Two cohorts showed no significant discrepancy in demographics and clinical characteristics by chi-squared test (Table 1).
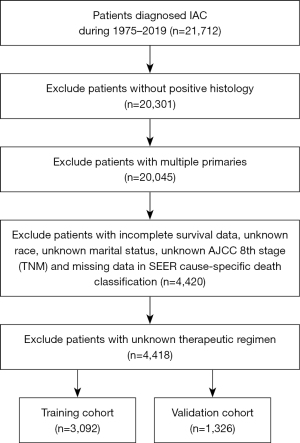
Table 1
Characteristic | High (n=1,001) | Median (n=1,866) | Low (n=1,551) | Total (N=4,418) | P value |
---|---|---|---|---|---|
Age, years | <0.001 | ||||
<60 | 128 (12.8) | 313 (16.8) | 284 (18.3) | 725 (16.4) | |
60–69 | 292 (29.2) | 671 (36.0) | 507 (32.7) | 1,470 (33.3) | |
70–79 | 395 (39.5) | 675 (36.2) | 540 (34.8) | 1,610 (36.4) | |
≥80 | 186 (18.6) | 207 (11.1) | 220 (14.2) | 613 (13.9) | |
Sex | <0.001 | ||||
Female | 667 (66.6) | 1,140 (61.1) | 841 (54.2) | 2,648 (59.9) | |
Male | 334 (33.4) | 726 (38.9) | 710 (45.8) | 1,770 (40.1) | |
Race | <0.001 | ||||
White | 821 (82.0) | 1,476 (79.1) | 1,273 (82.1) | 3,570 (80.8) | |
Black | 62 (6.2) | 165 (8.8) | 144 (9.3) | 371 (8.4) | |
Other | 118 (11.8) | 225 (12.1) | 134 (8.6) | 477 (10.8) | |
Marital status | <0.001 | ||||
Married | 594 (59.3) | 1,094 (58.6) | 927 (59.8) | 2,615 (59.2) | |
Divorced | 123 (12.3) | 239 (12.8) | 197 (12.7) | 559 (12.6) | |
Single | 103 (10.3) | 283 (15.2) | 214 (13.8) | 600 (13.6) | |
Widowed | 181 (18.1) | 250 (13.4) | 213 (13.7) | 644 (14.6) | |
Derived AJCC T stage | <0.001 | ||||
T1 | 579 (57.8) | 1,018 (54.6) | 613 (39.5) | 2,210 (50.0) | |
T2 | 179 (17.9) | 516 (27.7) | 389 (25.1) | 1,084 (24.4) | |
T3 | 41 (4.1) | 156 (8.4) | 148 (9.5) | 345 (7.8) | |
T4 | 202 (20.2) | 176 (9.4) | 401 (25.9) | 779 (17.6) | |
Derived AJCC N stage | <0.001 | ||||
N0 | 876 (87.5) | 1,513 (81.1) | 1,168 (75.3) | 3,557 (80.5) | |
N1 | 46 (4.6) | 131 (7.0) | 141 (9.1) | 318 (7.2) | |
N2 | 68 (6.8) | 177 (9.5) | 188 (12.1) | 433 (9.8) | |
N3 | 11 (1.1) | 45 (2.4) | 54 (3.5) | 110 (2.5) | |
Derived AJCC M stage | <0.001 | ||||
M0 | 887 (88.6) | 1,693 (90.7) | 1,272 (82.0) | 3,852 (87.2) | |
M1 | 114 (11.4) | 173 (9.3) | 279 (18.0) | 566 (12.8) | |
Derived AJCC stage | <0.001 | ||||
I | 683 (68.2) | 1,250 (67.0) | 765 (49.3) | 2,698 (61.1) | |
II | 64 (6.4) | 212 (11.4) | 192 (12.4) | 468 (10.6) | |
III | 140 (14.0) | 231 (12.4) | 315 (20.3) | 686 (15.5) | |
IV | 114 (11.4) | 173 (9.3) | 279 (18.0) | 566 (12.8) | |
Surgery | <0.001 | ||||
Yes | 689 (68.8) | 1,626 (87.1) | 1,143 (73.7) | 3,458 (78.3) | |
No | 312 (31.2) | 240 (12.9) | 408 (26.3) | 960 (21.7) | |
Death | <0.001 | ||||
Censored | 602 (60.1) | 1,606 (86.1) | 941 (60.7) | 3,149 (71.3) | |
Events | 399 (39.9) | 260 (13.9) | 610 (39.3) | 1,269 (28.7) | |
Cancer-specific death | <0.001 | ||||
Censored | 745 (74.4) | 1,671 (89.5) | 1,096 (70.7) | 3,512 (79.5) | |
Events | 256 (25.6) | 195 (10.5) | 455 (29.3) | 906 (20.5) |
Data are presented as n (%). IAC, invasive adenocarcinoma; AJCC, American Joint Committee on Cancer.
Study variables
Several demographic variables of patients, including age, sex, race, and marital status, were extracted from the SEER database. For a more concise analysis, the patients were segmented into four 4 groups: <60 years old, 60–69 years old, 70–79 years old, and ≥80 years old. Marital status was categorized as married, single, separated, divorced, and widowed according to the database. Divorced and separated patients were assigned into the divorced group which makes the results clear and credible.
Clinicopathologic variables captured from the SEER database encompassed pathological subtypes, tumor staging according to the 8th American Joint Committee on Cancer (AJCC) TNM stage, and surgery. Pathological subtypes were classified into 3 groups according to differentiation depending on the previously mentioned criteria. High differentiation included only adenocarcinoma. Median differentiation consisted of acinar or PAP. Low differentiation included MIP, SOL, invasive mucinous adenocarcinoma, mixed invasive mucinous and nonmucinous adenocarcinoma, colloid adenocarcinoma, fetal adenocarcinoma, and enteric adenocarcinoma.
From diagnosis to death for any reason, overall survival (OS) was the primary terminal point. As a secondary terminal point, cancer-specific survival (CSS) was defined as the period from diagnosis to death due to IAC.
Construction and verification of nomograms
Risk factors were identified by univariate analysis and multivariate analysis, and then the nomograms were constructed. The performance and accuracy of nomograms were assessed by receiver operating characteristics (ROC) curves, calibration plots, and decision curve analysis (DCA). More areas under the ROC curve (AUC) meant higher quality. Conventionally, 0.5 < AUC < 0.7 is considered low accuracy, 0.7 < AUC < 0.9 is medium accuracy, and AUC >0.9 is high accuracy. Most researchers believe that a qualified model should have an AUC greater than 0.7. Our calibrations were performed using bootstraps and 1,000 resamples to ensure a solid comparison of the predicted and observed OS and CSS over 1, 3, and 5 years. DCA curves showed benefits derived from constructed models.
Ethical statement
The study was conducted in accordance with the Declaration of Helsinki (as revised in 2013). Since the present study relied on the SEER database, which was freely available to the public (http://seer.cancer.gov/seerstat/), the ethical approval was waived.
Statistical analysis
The consistency between the training cohort and the testing cohort was checked by chi-squared tests. By using this statistical method, the baseline data of demographical and clinicopathological features in disparate differentiation groups were compared. OS and CSS survival analyses were performed using the Kaplan-Meier method and nonparametric group comparisons were performed using the log-rank test. For the training cohort, the independent prognostic factors of OS and CSS were determined using univariate and multivariate Cox proportional hazards regression models. All P values were two-sided, and statistical significance was determined by P<0.05. All statistical analyses and drawings were conducted using R software version 4.2.1.
Results
Baseline patient features
Four thousand four hundred and eighteen eligible cases from 1975 to 2019 were screened through SEER database searches. Information of the patients is displayed in Tables 1,2. As shown in Table 1, 1,001 cases were highly differentiated (22.7%), 1,866 cases were moderately differentiated (42.2%), and 1,551 cases were low differentiated (35.1%). The factors, age, gender, race, marital status, stage, surgery, death, and cancer-specific death were shown to significantly influence on pathological differentiation (P<0.001, chi-square test). In view of the difference in data composition of disparate pathological groups, differentiation-specific nomograms were applied to predict the OS and CSS. In general, there were more seniors over the age of 60 (83.6%), females (59.9%), whites (80.8%), and married people (59.2%). Most patients were in the early stages (T1: 50.0%, N0: 80.5%, M0: 87.2%, TNM stage I: 61.1%) and had surgery (78.3%). The high differentiation group had the highest proportion of T1 (57.8%), N0 (87.5%), TNM stage I (68.2%), suggesting a potential connection between high-differentiation and early TNM stage. The median differentiation group had the highest percentage of surgery (87.1%). A comparison of the training cohort and testing cohort showed no significant differences (P>0.05, chi-square test) in demographics or clinical characteristics (Table 2).
Table 2
Characteristic | Training (n=3,092) | Validation (n=1,326) | Total (N=4,418) | P value |
---|---|---|---|---|
Age, years | 0.104 | |||
<60 | 513 (16.6) | 212 (16.0) | 725 (16.4) | |
60–69 | 1,050 (34.0) | 420 (31.7) | 1,470 (33.3) | |
70–79 | 1,124 (36.4) | 486 (36.7) | 1,610 (36.4) | |
≥80 | 405 (13.1) | 208 (15.7) | 613 (13.9) | |
Sex | 1 | |||
Female | 1,853 (59.9) | 795 (60.0) | 2,648 (59.9) | |
Male | 1,239 (40.1) | 531 (40.0) | 1,770 (40.1) | |
Race | 0.497 | |||
White | 2,488 (80.5) | 1,082 (81.6) | 3,570 (80.8) | |
Black | 259 (8.4) | 112 (8.4) | 371 (8.4) | |
Other | 345 (11.2) | 132 (10.0) | 477 (10.8) | |
Marital status | 0.685 | |||
Married | 1,842 (59.6) | 773 (58.3) | 2,615 (59.2) | |
Divorced | 393 (12.7) | 166 (12.5) | 559 (12.6) | |
Single | 419 (13.6) | 181 (13.7) | 600 (13.6) | |
Widowed | 438 (14.2) | 206 (15.5) | 644 (14.6) | |
Derived AJCC T stage | 0.807 | |||
T1 | 1,536 (49.7) | 674 (50.8) | 2,210 (50.0) | |
T2 | 768 (24.8) | 316 (23.8) | 1,084 (24.4) | |
T3 | 246 (8.0) | 99 (7.5) | 345 (7.8) | |
T4 | 542 (17.5) | 237 (17.9) | 779 (17.6) | |
Derived AJCC N stage | 0.137 | |||
N0 | 2,504 (81.0) | 1,053 (79.4) | 3,557 (80.5) | |
N1 | 220 (7.1) | 98 (7.4) | 318 (7.2) | |
N2 | 285 (9.2) | 148 (11.2) | 433 (9.8) | |
N3 | 83 (2.7) | 27 (2.0) | 110 (2.5) | |
Derived AJCC M stage | 0.722 | |||
M0 | 2,700 (87.3) | 1,152 (86.9) | 3,852 (87.2) | |
M1 | 392 (12.7) | 174 (13.1) | 566 (12.8) | |
Derived AJCC stage | 0.902 | |||
I | 1,886 (61.0) | 812 (61.2) | 2,698 (61.1) | |
II | 334 (10.8) | 134 (10.1) | 468 (10.6) | |
III | 480 (15.5) | 206 (15.5) | 686 (15.5) | |
IV | 392 (12.7) | 174 (13.1) | 566 (12.8) | |
Differentiation | 0.678 | |||
High | 692 (22.4) | 309 (23.3) | 1,001 (22.7) | |
Median | 1,303 (42.1) | 563 (42.5) | 1,866 (42.2) | |
Low | 1,097 (35.5) | 454 (34.2) | 1,551 (35.1) | |
Surgery | 0.253 | |||
Yes | 2,435 (78.8) | 1,023 (77.1) | 3,458 (78.3) | |
No | 657 (21.2) | 303 (22.9) | 960 (21.7) | |
Death | 0.697 | |||
Censored | 2,198 (71.1) | 951 (71.7) | 3,149 (71.3) | |
Events | 894 (28.9) | 375 (28.3) | 1,269 (28.7) | |
Cancer-specific death | 1 | |||
Censored | 2,458 (79.5) | 1,054 (79.5) | 3,512 (79.5) | |
Events | 634 (20.5) | 272 (20.5) | 906 (20.5) |
Data are presented as n (%). AJCC, American Joint Committee on Cancer.
Exploration of prognostic risk factors associated with OS and CSS
By analyzing univariable and multivariable data, risk factors associated with the survival of IAC patients in the training cohort were identified. On univariate analysis, older age, male sex, white, lower differentiated, divorced or widowed, higher T/N/M stage, higher TNM stage, and no surgery predicted poorer OS and CSS (Table 3). Moreover, results are also presented in the form of Kaplan-Meier curves (Figures 2,3). All variables analyzed were significant and were included in multivariate analysis. In the multivariate analysis of both OS and CSS, variables including age, sex, race, differentiation, marital stage, TNM stage and surgery were all statistically significant (Table 4) and were determined as independent risk factors for OS and CSS of IAC. However, the differences between blacks and whites in race group were not significant. Analysis of multivariate data found that the prognosis improved in patients of younger age, female, other-race (Asians, Pacific Islanders and Hispanics), highly differentiated stage, lower TNM stage, and surgery.
Table 3
Variable | OS | CSS | |||||
---|---|---|---|---|---|---|---|
HR | 95% CI | P value | HR | 95% CI | P value | ||
Age, years | |||||||
<60 | Reference | Reference | |||||
60–69 | 1.030 | 0.853–1.245 | 0.756 | 0.932 | 0.755–1.151 | 0.51 | |
70–79 | 1.538 | 1.287–1.839 | <0.001 | 1.218 | 0.995–1.489 | 0.04 | |
≥80 | 2.490 | 2.056–3.016 | <0.001 | 1.982 | 1.594–2.465 | <0.001 | |
Sex | |||||||
Female | Reference | Reference | |||||
Male | 1.476 | 1.322–1.648 | <0.001 | 1.541 | 1.353–1.755 | <0.001 | |
Race | |||||||
White | Reference | Reference | |||||
Black | 0.741 | 0.593–0.9268 | 0.009 | 0.731 | 0.560–0.955 | 0.021 | |
Other | 0.680 | 0.559–0.827 | <0.001 | 0.733 | 0.584–0.919 | 0.007 | |
Differentiation | |||||||
High | Reference | Reference | |||||
Median | 0.734 | 0.626–0.863 | <0.001 | 0.791 | 0.654–0.958 | 0.016 | |
Low | 1.413 | 1.243–1.605 | <0.001 | 1.571 | 1.346–1.834 | <0.001 | |
Marital status | |||||||
Married | Reference | Reference | |||||
Divorced | 1.154 | 0.973–1.368 | 0.1 | 1.12 | 0.917–1.369 | 0.267 | |
Single | 0.815 | 0.675–0.984 | 0.03 | 0.742 | 0.591–0.933 | <0.001 | |
Widowed | 1.433 | 1.242–1.653 | <0.001 | 1.321 | 1.113–1.569 | <0.001 | |
Derived AJCC T stage | |||||||
T1 | Reference | Reference | |||||
T2 | 1.382 | 1.181–1.618 | <0.001 | 1.613 | 1.320–1.972 | <0.001 | |
T3 | 2.070 | 1.656–2.586 | <0.001 | 2.753 | 2.114–3.585 | <0.001 | |
T4 | 3.929 | 3.450–4.476 | <0.001 | 5.961 | 5.078–6.999 | <0.001 | |
Derived AJCC N stage | |||||||
N0 | Reference | Reference | |||||
N1 | 1.891 | 1.542–2.320 | <0.001 | 2.278 | 1.810–2.867 | <0.001 | |
N2 | 3.801 | 3.277–4.407 | <0.001 | 4.818 | 4.088–5.679 | <0.001 | |
N3 | 5.705 | 4.505–7.224 | <0.001 | 7.107 | 5.487–9.205 | <0.001 | |
Derived AJCC M stage | |||||||
M0 | Reference | Reference | |||||
M1 | 5.955 | 5.280–6.717 | <0.001 | 8.176 | 7.139–9.363 | <0.001 | |
Derived AJCC stage | |||||||
I | Reference | Reference | |||||
II | 1.473 | 1.169–1.857 | <0.001 | 1.634 | 1.209–2.209 | 0.001 | |
III | 2.656 | 2.296–3.071 | <0.001 | 3.894 | 3.256–4.656 | <0.001 | |
IV | 7.957 | 6.952–9.107 | <0.001 | 12.850 | 10.911–15.121 | <0.001 | |
Surgery | |||||||
Yes | Reference | Reference | |||||
No | 4.932 | 4.411–5.514 | <0.001 | 6.078 | 5.323–6.941 | <0.001 |
OS, overall survival; CSS, cancer-specific survival; HR, hazard ratio; CI, confidence interval; AJCC, American Joint Committee on Cancer.
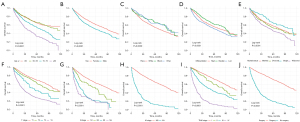
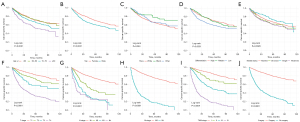
Table 4
Variable | OS | CSS | |||||
---|---|---|---|---|---|---|---|
HR | 95% CI | P value | HR | 95% CI | P value | ||
Age, years | |||||||
<60 | Reference | Reference | |||||
60–69 | 1.28 | 1.06–1.56 | 0.011 | 1.25 | 1.01–1.56 | <0.001 | |
70–79 | 1.75 | 1.45–2.11 | <0.001 | 1.49 | 1.21–1.84 | <0.001 | |
≥80 | 2.23 | 1.81–2.75 | <0.001 | 1.91 | 1.5–2.43 | <0.001 | |
Sex | |||||||
Female | Reference | Reference | |||||
Male | 1.48 | 1.32–1.67 | <0.001 | 1.51 | 1.31–1.73 | <0.001 | |
Race | |||||||
White | Reference | Reference | |||||
Black | 0.933 | 0.743–1.17 | 0.549 | 0.905 | 0.689–1.19 | 0.471 | |
Other | 0.728 | 0.597–0.887 | <0.001 | 0.765 | 0.608–0.962 | 0.022 | |
Differentiation | |||||||
High | Reference | Reference | |||||
Median | 0.909 | 0.772–1.07 | <0.001 | 0.97 | 0.799–1.18 | 0.76 | |
Low | 1.53 | 1.34–1.75 | <0.001 | 1.65 | 1.41–1.94 | <0.001 | |
Marital status | |||||||
Married | Reference | Reference | |||||
Divorced | 1.3 | 1.09–1.55 | 0.003 | 1.28 | 1.04–1.57 | 0.017 | |
Single | 1.02 | 0.845–1.24 | 0.803 | 0.971 | 0.768–1.23 | <0.001 | |
Widowed | 1.4 | 1.2–1.65 | <0.001 | 1.39 | 1.15–1.69 | <0.001 | |
Derived AJCC stage | |||||||
I | Reference | Reference | |||||
II | 1.44 | 1.14–1.82 | <0.001 | 1.59 | 1.17–2.16 | 0.003 | |
III | 2.37 | 2.04–2.75 | <0.001 | 3.39 | 2.82–4.06 | <0.001 | |
IV | 4.78 | 4.07–5.61 | <0.001 | 7.29 | 6.01–8.85 | <0.001 | |
Surgery | |||||||
Yes | Reference | Reference | |||||
No | 2.54 | 2.22–2.92 | <0.001 | 2.69 | 2.28–3.18 | <0.001 |
OS, overall survival; CSS, cancer-specific survival; HR, hazard ratio; CI, confidence interval; AJCC, American Joint Committee on Cancer.
Subgroup analysis of the effects of differentiation
The effects of differentiation on CSS and OS regarding other prognostic factors were evaluated (Figures 4,5). Differences in differentiation were significant in the 60–69 years group (P<0.0001, Figure 4B; P<0.0001, Figure 5B) and the 70–79 group (P<0.0001, Figure 4C; P<0.0001, Figure 5C). We previously speculated the effect of differentiation on prognosis should be more and more significant with the increase of age, but there was no remarkable difference in the ≥80 group (P=0.78, Figure 4D; P=0.98, Figure 5D), which may due to the limited cases in this group, and other higher-risk clinical conditions among the elderly. In addition, significant differences in female group (P<0.0001, Figure 4E; P<0.0001, Figure 5E), male group (P<0.0001, Figure 4F; P<0.0001, Figure 5F), white-race group (P<0.0001, Figure 4K; P<0.0001, Figure 5K), surgery group (P<0.0001, Figure 4R; P<0.0001, Figure 5R), and non-surgery group (P<0.0001, Figure 4S; P<0.0001, Figure 5S). To be noted, the results revealed that the contribution of differentiation to prognosis increased with higher TNM stage (Figures 4G-4J,5G-5J). Furthermore, the OS and CSS curves of high, moderate, and low differentiation groups did not make a distinction in stage I (P=0.097, Figure 4G; P=0.92, Figure 5G). In stage II, the 10-year CSS of lowly differentiated patients was just over half that of highly differentiated patients (Figure 5H). We also acknowledged that the OS and CSS of low-differentiation IAC patients were lower than that of the other two groups in stage III and IV (Figures 4I-4J,5I-5J).
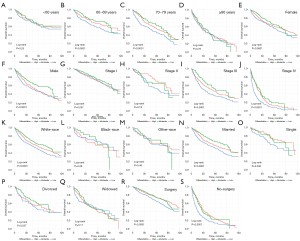
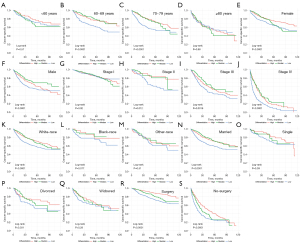
Construction of the nomogram for OS and CSS
All significant risk factors of Cox regression in the training group were included in the differentiation-specific prognostic nomograms. Besides, tumor size was added in nomograms due to the importance of IAC T-stage and forest maps were produced as a result of the final multivariate analysis (Figure 6). Based on these independent prognostic factors of high, median, and low differentiated IAC, the differentiation-specific nomogram models for 1-, 3- and 5-year IAC patients’ OS and CSS were constructed (Figure 7). The nomograms indicated that TNM stage was the strongest prognostic factor both in OS and CSS. Older age could indicate worse OS rather than CSS and we guess that old people are more likely to die from other diseases. The marital status played more important roles in high-differentiation nomograms (Figure 7A,7D) compared with others (Figure 7B,7C,7E,7F). Surgery had a great impact on each nomogram, while race played only a small role. The predicted probabilities of OS and CSS in 1, 3 and 5 years were obtained by consolidating the scores associated with each variable and converting them to the bottom scales.
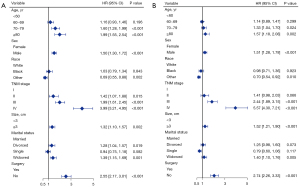
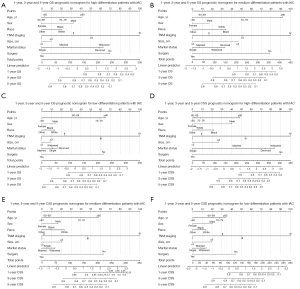
Validation of the nomograms
As a way to demonstrate the advantages of the nomogram models, ROC curves were plotted to identify accurate predictability for OS and CSS in the training and validation cohorts, respectively (Figure 8). AUC values of the ROC predicting the 1-, 3- and 5-year OS (Figure 8A-8C) and CSS (Figure 8D-8F) in training cohort were statistically satisfactory, as well as in the validation cohort (Figure 8G-8L). AUC values of OS and CSS in the training cohort were 0.817 [95% confidence interval (CI): 0.753–0.881] and 0.835 (95% CI: 0.774–0.896), while in the validation cohort were 0.784 (95% CI: 0.695–0.874) and 0.813 (95% CI: 0.722–0.905). Moreover, to investigate the discrimination of models constructed, patients were separated into high-risk and low-risk groups by median of model scores. As was revealed, the high-risk groups always had lower survival than the low-risk groups (P<0.001, Log-rank test) in both training cohorts (Figure 9A-9F) and validation cohorts (Figure 9G-9L). On each nomogram, a calibration curve was plotted to assess its accuracy. Calibration plot for predicting OS and CSS of 1-, 3- and 5-year demonstrated good predictability in Figure 10. Meanwhile, models for predicting 1-, 3- and 5-year survival of IAC were better superior to those in non-treated and all-treated groups in DCA curves (Figure 11).
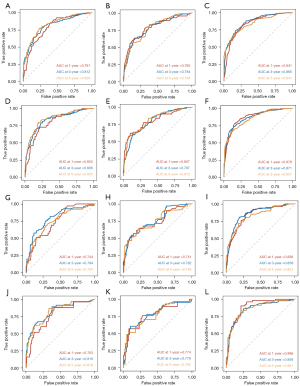
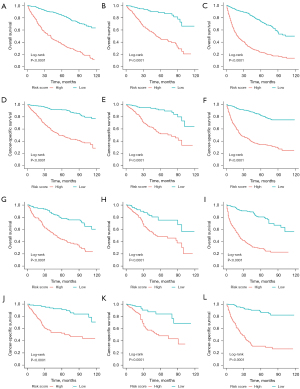
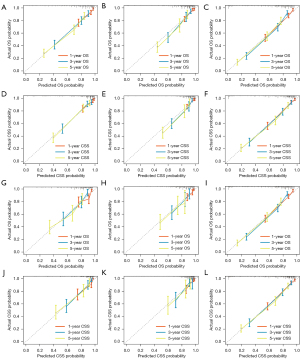
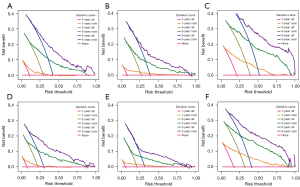
Discussion
Nowadays, lung cancer is still at the top place for cancer-related mortality, and LUAD is becoming more and more frequent (1,15). IAC plays an important role in LUAD but only a few researches have been conducted on IAC and its variants. In view of a few researches on the relationship between pathological grades and other factors of prognosis, this study focused on the connection and developed nomograms to estimate the outcomes of IAC, which could contribute to the development of a strategic treatment.
The study found that older patients, especially those over 80 years old, had distinctly lower OS and CSS. Simultaneously, age had a strong effect on nomograms (Figure 7), especially in median-differentiation nomogram models (Figure 7B,7E). Several studies have shown that advanced age has a detrimental effect on prognoses of IAC patients, which consisted with the findings in this study (2,3).
LUAD cases have increased dramatically over the past 20 years, especially among females (23). Therefore, the dataset of this study was composed mainly of female patients and the female gender could be a protective factor of IAC. Marital status has been shown to significantly influence the prognosis of LUAD patients since divorced patients are less likely to enjoy a relatively harmonious family environment (24). In this study, marital status for the first time was found to be an independent risk factor for CSS and OS of IAC, which was added to the nomogram. Studies have shown that white patients had the worst survival rate among IAC patients of different races (8,9), which consisted with our results. Moreover, as with most solid tumors, surgery remains the most effective treatment for IAC. According to our data, OS and CSS were significantly prolonged in surgical patients compared to non-surgical patients. Most patients undergoing surgery were in stage I (88.5%), II (90.9%), and III (75.9%). We speculated that the lower-staged patients were in better physical condition and at younger age, which may contribute to a better prognosis. Only 22.0% of stage IV patients opted for surgery and the prognosis (cancer-related deaths and non-cancer-related deaths) was poor in this group.
Histological patterns have long been recognized as an independent prognostic predictor of LUAD, closely related to biological characteristics and surgical outcomes (25-28). Our study divided the histological patterns into three types of differentiation and confirmed that the prognostic role of histological patterns still existed in IAC.
It is generally believed that lowly differentiated LUAD has a high recurrence rate and poor prognosis. Liu et al. (29) reported that patients with lowly differentiated LUAD had a higher risk of recurrence than those with highly differentiated LUAD throughout the follow-up. Notably, the recurrence hazard curve in low-differentiated patients showed a typical “double-peaked” pattern, which means the recurrence of patients was concentrated at 20–22 months and 5–6 years after surgery, while the curve of high-differentiated patients is relatively smooth after surgery. Some types of low-differentiated IAC, such as micropapillary and solid, are significantly associated with lymph node metastasis (30). Besides, pathological differentiation is an independent predictor for local recurrence, distant metastasis, chest recurrence and brain recurrence (26,29,31). IASLC pathology committee has specified a histology-based grading system for invasive pulmonary adenocarcinoma, which plays an important role in prognosis stratification (17,32-34). To be noted, IAC is histologically and molecularly heterogeneous even in patients with the same TNM stage, which affects their prognosis and treatment strategies (35). Therefore, to comprehensively predict the prognosis of ICA, other factors, such as TNM stage, age, and surgery should also be considered along with pathological differentiation. Patients with high- and moderate-differentiation had a better OS and CSS than low-differentiation patients at stage III and IV, while the differences was not significant in stage I patients. Some studies have explored the relationship between pathological components and prognosis after different types of surgery, revealing that the micropapillary/solid subtype indicates worse prognosis overall (36-38). Differentiation is correlated to almost all factors, except young, lower TNM-stage, non-white and widowed patients. Thus, for personalized medicine paradigm, clinicians can classify IAC patients by level of differentiation to predict prognosis. Histological subtypes have also been proved to be an independent factor of adjuvant chemotherapy, while micropapillary/solid subtype was considered as a negative predictor (28,39). Some doctors suggest patients with low differentiated IAC should receive postoperative adjuvant therapy (40). Due to the unavailability of chemoradiotherapy data, they are not included in our study.
Based on former studies, the combination of pathological differentiation and other factors has great influence on the prognosis of IAC, caused by complicated mechanisms. Many studies have focused on this aspect with no certain conclusion reached. However, there are some clues supporting pathogenesis, progression and prognosis of IAC are influenced by genomic alteration, especially in different pathological subtypes. Compared with high-differentiated IAC (LEP) and moderate-differentiated IAC (ACI/PAP), previous studies revealed that tumor mutational burden (TMB), fraction of genome altered (FGA), copy number amplifications, whole-genome doubling, rate of transversion and number of altered oncogenic pathways were higher in low-differentiated IAC (MIP/SOL and some variants) (41,42). The median of TMB and FGA increased as the subtypes became more aggressive. Besides, various altered genes have been found in distinct differentiated groups of lung cancer. Three genes (EGFR, RBM10, and TERT) are significantly altered in LEP, while 4 genes (TP53, SETD2, MGA, and SMARCA4) are significantly altered in MIP/SOL. Studies show that SOLs have the greatest amount of transversion/transition, while lepidic adenocarcinomas have the lowest amount. Mutations in PI3K pathways are closely related to recurrence and metastasis in ACI, PAP, MIP, and SOL subtypes (IAC with moderate and low differentiation). In pathologic subtypes that show higher aggression, EGFR mutations are less frequent, while the frequency of cell cycle pathway changes including TP53 mutations goes up (43). Some studies reported that KRAS and EGFR mutations are highly correlated with the invasiveness and inertia of tumor tissue, respectively (44). Adenocarcinomas and mucinous carcinomas were significantly characterized by KRAS mutations, the presence of tumor-infiltrating leukocytes and heavy smoking history. On the contrary, there was an association between EGFR mutations combined with non-smoking history and high/median-differentiation IAC, especially lepidic and papillary.
In recent years, immunotherapy has given new hope to lung cancer patients and have achieved good clinical results in some cases (45,46). Different pathological subtypes also shared disparate different immune profiles. Solid predominant IAC often showed the overexpression of PD-L1 and a high percentage of tumor-infiltrating lymphocytes, which provided a potential target for treatment-adjuvant PD-1 blockade immunotherapy. Dong et al. (42) claimed that the pathological subtype of IAC could serve as a potential predictor of adjuvant immunotherapy. Among IAC patients, the co-expression of PD-L1/CD47 has a prognostic effect, associated with an increase in CD8+ T-cells (47). Therefore, it is believed that PD-L1/CD47 co-expression could be used to predict the efficacy of dual-targeting immunotherapy. Based on the deepening understanding of different immune characteristics, pathology-specific immunotherapy may achieve good clinical results in the future.
Nomograms are graphical displays of mathematical models, which integrate biological and clinical variables to forecast the probability of certain clinical events. Although nomograms are a reliable tool for prognosis prediction, to some extent, the model is still not perfect in this study. First, this is a retrospective observational study, therefore inherent selection bias is inevitable, which suggests that further prospective comparative studies are needed. Second, the nomogram has not included some critical factors, such as smoking history, lymphovascular invasion, predominant growth patterns, and mixed pathological subtypes, due to the unavailability of this information in the SEER database. Rather than a distinct subtype of pathology, IACs are generally mixture of heterogeneous subtypes and the patterns are usually continuous (e.g., lepidic to papillary or acinar). The SEER database provides only one subtype, which could result in a decrease in the universality of the nomogram. In addition, many other factors that may affect prognosis, such as mutations of the KRAS and EGFR genes and expression of PD-L1. Third, the potential interaction terms are not considered in the nomogram to improve the conciseness and interpretability. Taking all potential interaction terms in model construction may lead to better predictive performance but this will make the model more complex and difficult to use in clinical practice. Despite validating the data, clinical trials could have provided more convincing evidence for the conclusion. In addition to the data of SEER database, the accuracy of models could be further judged by follow-up of patients with clear pathology.
Notably, this appears to be the first research to separate the prognostic factors of IAC based on differentiation level, followed by nomogram model construction and evaluation. Nomogram accuracy can be evaluated by using the AUCs of ROC curves and calibration curves. In our nomogram models, the majority of AUCs predicting the 1-, 3- and 5-year OS and CSS are higher than 0.75, suggesting high model accuracy. The calibration curve and DCA curves are also satisfactory, which means our nomogram models are highly reliable.
Conclusions
This study explored the impact of disparate pathological differentiation on the prognosis of IAC and developed differentiation-specific nomogram models to predict the OS and CSS in 1-, 3- and 5-year. Using these nomogram models, clinicians can accurately predict the prognosis and select appropriate treatment options for IAC patients. In future studies, we will further explore the pathogenesis and genomic changes of IAC with different pathological differentiation.
Acknowledgments
We acknowledge the Surveillance, Epidemiology, and End Results (SEER) Program tumor registries for their efforts to provide high quality open resources.
Funding: This work was supported by
Footnote
Reporting Checklist: The authors have completed the TRIPOD reporting checklist. Available at https://tcr.amegroups.com/article/view/10.21037/tcr-22-2308/rc
Conflicts of Interest: All authors have completed the ICMJE uniform disclosure form (available at https://tcr.amegroups.com/article/view/10.21037/tcr-22-2308/coif). All authors report that this study was supported by the Natural Science Foundation of Jiangsu Province (No. BK20210068), the Top Talent Support Program for Young and Middle-aged People of Wuxi Municipal Health Commission (No. HB2020003), Mega-project of Wuxi Commission of Health (No. Z202216), and the High-end Medical Expert Team of the 2019 Taihu Talent Plan (No. 2019-THRCTD1). The authors have no other conflicts of interest to declare.
Ethical Statement: The authors are accountable for all aspects of the work in ensuring that questions related to the accuracy or integrity of any part of the work are appropriately investigated and resolved. The study was conducted in accordance with the Declaration of Helsinki (as revised in 2013). Since the study relied on the SEER database, which was freely available to the public (http://seer.cancer.gov/seerstat/), the ethical approval was waived.
Open Access Statement: This is an Open Access article distributed in accordance with the Creative Commons Attribution-NonCommercial-NoDerivs 4.0 International License (CC BY-NC-ND 4.0), which permits the non-commercial replication and distribution of the article with the strict proviso that no changes or edits are made and the original work is properly cited (including links to both the formal publication through the relevant DOI and the license). See: https://creativecommons.org/licenses/by-nc-nd/4.0/.
References
- Global Burden of Disease Cancer Collaboration. Global, Regional, and National Cancer Incidence, Mortality, Years of Life Lost, Years Lived With Disability, and Disability-Adjusted Life-Years for 29 Cancer Groups, 1990 to 2017: A Systematic Analysis for the Global Burden of Disease Study. JAMA Oncol 2019;5:1749-68. [Crossref] [PubMed]
- Hu S, Zhang W, Guo Q, et al. Prognosis and Survival Analysis of 922,317 Lung Cancer Patients from the US Based on the Most Recent Data from the SEER Database (April 15, 2021). Int J Gen Med 2021;14:9567-88. [Crossref] [PubMed]
- You H, Teng M, Gao CX, et al. Construction of a Nomogram for Predicting Survival in Elderly Patients With Lung Adenocarcinoma: A Retrospective Cohort Study. Front Med (Lausanne) 2021;8:680679. [Crossref] [PubMed]
- Radkiewicz C, Dickman PW, Johansson ALV, et al. Sex and survival in non-small cell lung cancer: A nationwide cohort study. PLoS One 2019;14:e0219206. [Crossref] [PubMed]
- Huang Q, Zhang Z, Liao Y, et al. 17β-estradiol upregulates IL6 expression through the ERβ pathway to promote lung adenocarcinoma progression. J Exp Clin Cancer Res 2018;37:133. [Crossref] [PubMed]
- Chen W, Xin B, Pang H, et al. Downregulation of estrogen receptor β inhibits lung adenocarcinoma cell growth. Oncol Rep 2019;41:2967-74. [Crossref] [PubMed]
- Wen H, Lin X, Sun D. Gender-specific nomogram models to predict the prognosis of male and female lung adenocarcinoma patients: a population-based analysis. Ann Transl Med 2021;9:1654. [Crossref] [PubMed]
- Wu X, Wang Y, Lin X, et al. Racial and Ethnic Disparities in Lung Adenocarcinoma Survival: A Competing-Risk Model. Clin Lung Cancer 2020;21:e171-81. [Crossref] [PubMed]
- Clegg LX, Li FP, Hankey BF, et al. Cancer survival among US whites and minorities: a SEER (Surveillance, Epidemiology, and End Results) Program population-based study. Arch Intern Med 2002;162:1985-93. [Crossref] [PubMed]
- Zeng Y, Mayne N, Yang CJ, et al. A Nomogram for Predicting Cancer-Specific Survival of TNM 8th Edition Stage I Non-small-cell Lung Cancer. Ann Surg Oncol 2019;26:2053-62.
- Putila J, Remick SC, Guo NL. Combining clinical, pathological, and demographic factors refines prognosis of lung cancer: a population-based study. PLoS One 2011;6:e17493. [Crossref] [PubMed]
- Li G, Xie S, Hu F, et al. Segmentectomy or Wedge Resection in Stage IA Lung Squamous Cell Carcinoma and Adenocarcinoma? J Cancer 2021;12:1708-14. [Crossref] [PubMed]
- Komiya T, Chaaya G, Powell E. Addition of chemotherapy improves overall survival in patients with T2N0M0 non-small cell lung cancer undergoing definitive radiation therapy: An analysis of the SEER database. Radiother Oncol 2019;131:75-80. [Crossref] [PubMed]
- Zhang R, Li P, Li Q, et al. Radiotherapy improves the survival of patients with stage IV NSCLC: A propensity score matched analysis of the SEER database. Cancer Med 2018;7:5015-26. [Crossref] [PubMed]
- Kuhn E, Morbini P, Cancellieri A, et al. Adenocarcinoma classification: patterns and prognosis. Pathologica 2018;110:5-11. [PubMed]
- Travis WD, Brambilla E, Nicholson AG, et al. The 2015 World Health Organization Classification of Lung Tumors: Impact of Genetic, Clinical and Radiologic Advances Since the 2004 Classification. J Thorac Oncol 2015;10:1243-60. [Crossref] [PubMed]
- Moreira AL, Ocampo PSS, Xia Y, et al. A Grading System for Invasive Pulmonary Adenocarcinoma: A Proposal From the International Association for the Study of Lung Cancer Pathology Committee. J Thorac Oncol 2020;15:1599-610. [Crossref] [PubMed]
- Xie GD, Liu YR, Jiang YZ, et al. Epidemiology and survival outcomes of mucinous adenocarcinomas: A SEER population-based study. Sci Rep 2018;8:6117. [Crossref] [PubMed]
- Wang Y, Liu J, Huang C, et al. Development and validation of a nomogram for predicting survival of pulmonary invasive mucinous adenocarcinoma based on surveillance, epidemiology, and end results (SEER) database. BMC Cancer 2021;21:148. [Crossref] [PubMed]
- Zhang G, Wang X, Jia J, et al. Development and validation of a nomogram for predicting survival in patients with surgically resected lung invasive mucinous adenocarcinoma. Transl Lung Cancer Res 2021;10:4445-58. [Crossref] [PubMed]
- Zhang Y, Xie H, Zhang Z, et al. The Characteristics and Nomogram for Primary Lung Papillary Adenocarcinoma. Open Med (Wars) 2020;15:92-102. [Crossref] [PubMed]
- Liu A, Hou F, Qin Y, et al. Predictive value of a prognostic model based on pathologic features in lung invasive adenocarcinoma. Lung Cancer 2019;131:14-22. [Crossref] [PubMed]
- Bray F, Ferlay J, Soerjomataram I, et al. Global cancer statistics 2018: GLOBOCAN estimates of incidence and mortality worldwide for 36 cancers in 185 countries. CA Cancer J Clin 2018;68:394-424. [Crossref] [PubMed]
- Wu Y, Zhu PZ, Chen YQ, et al. Relationship between marital status and survival in patients with lung adenocarcinoma: A SEER-based study. Medicine (Baltimore) 2022;101:e28492. [Crossref] [PubMed]
- Sica G, Yoshizawa A, Sima CS, et al. A grading system of lung adenocarcinomas based on histologic pattern is predictive of disease recurrence in stage I tumors. Am J Surg Pathol 2010;34:1155-62. [Crossref] [PubMed]
- Zhao Y, Wang R, Shen X, et al. Minor Components of Micropapillary and Solid Subtypes in Lung Adenocarcinoma are Predictors of Lymph Node Metastasis and Poor Prognosis. Ann Surg Oncol 2016;23:2099-105. [Crossref] [PubMed]
- Warth A, Muley T, Meister M, et al. The novel histologic International Association for the Study of Lung Cancer/American Thoracic Society/European Respiratory Society classification system of lung adenocarcinoma is a stage-independent predictor of survival. J Clin Oncol 2012;30:1438-46. [Crossref] [PubMed]
- Tsao MS, Marguet S, Le Teuff G, et al. Subtype Classification of Lung Adenocarcinoma Predicts Benefit From Adjuvant Chemotherapy in Patients Undergoing Complete Resection. J Clin Oncol 2015;33:3439-46. [Crossref] [PubMed]
- Liu X, Sun K, Yang F, et al. Different pathologic types of early stage lung adenocarcinoma have different post-operative recurrence patterns. Thorac Cancer 2021;12:2205-13. [Crossref] [PubMed]
- Chang C, Sun X, Zhao W, et al. Minor components of micropapillary and solid subtypes in lung invasive adenocarcinoma (≤ 3 cm): PET/CT findings and correlations with lymph node metastasis. Radiol Med 2020;125:257-64. [Crossref] [PubMed]
- Hung JJ, Yeh YC, Wu YC, et al. Prognostic Factors in Completely Resected Node-Negative Lung Adenocarcinoma of 3 cm or Smaller. J Thorac Oncol 2017;12:1824-33. [Crossref] [PubMed]
- Jeon HW, Kim YD, Sim SB, et al. Significant difference in recurrence according to the proportion of high grade patterns in stage IA lung adenocarcinoma. Thorac Cancer 2021;12:1952-8. [Crossref] [PubMed]
- Kagimoto A, Tsutani Y, Kambara T, et al. Utility of Newly Proposed Grading System From International Association for the Study of Lung Cancer for Invasive Lung Adenocarcinoma. JTO Clin Res Rep 2020;2:100126. [Crossref] [PubMed]
- Rokutan-Kurata M, Yoshizawa A, Ueno K, et al. Validation Study of the International Association for the Study of Lung Cancer Histologic Grading System of Invasive Lung Adenocarcinoma. J Thorac Oncol 2021;16:1753-8. [Crossref] [PubMed]
- Cooper WA, Lam DC, O'Toole SA, et al. Molecular biology of lung cancer. J Thorac Dis 2013;5:S479-90. [PubMed]
- Wang Y, Zheng D, Zheng J, et al. Predictors of recurrence and survival of pathological T1N0M0 invasive adenocarcinoma following lobectomy. J Cancer Res Clin Oncol 2018;144:1015-23. [Crossref] [PubMed]
- Chen C, Chen ZJ, Li WJ, et al. Impact of minimal solid and micropapillary components on invasive lung adenocarcinoma recurrence. Ann Diagn Pathol 2022;59:151945. [Crossref] [PubMed]
- Morales-Oyarvide V, Mino-Kenudson M. High-grade lung adenocarcinomas with micropapillary and/or solid patterns: a review. Curr Opin Pulm Med 2014;20:317-23. [Crossref] [PubMed]
- Zhang Y, Li J, Wang R, et al. The prognostic and predictive value of solid subtype in invasive lung adenocarcinoma. Sci Rep 2014;4:7163. [Crossref] [PubMed]
- Motono N, Matsui T, Machida Y, et al. Prognostic significance of histologic subtype in pStage I lung adenocarcinoma. Med Oncol 2017;34:100. [Crossref] [PubMed]
- Caso R, Sanchez-Vega F, Tan KS, et al. The Underlying Tumor Genomics of Predominant Histologic Subtypes in Lung Adenocarcinoma. J Thorac Oncol 2020;15:1844-56. [Crossref] [PubMed]
- Dong ZY, Zhang C, Li YF, et al. Genetic and Immune Profiles of Solid Predominant Lung Adenocarcinoma Reveal Potential Immunotherapeutic Strategies. J Thorac Oncol 2018;13:85-96. [Crossref] [PubMed]
- Ahn B, Yoon S, Kim D, et al. Clinicopathologic and genomic features of high-grade pattern and their subclasses in lung adenocarcinoma. Lung Cancer 2022;170:176-84. [Crossref] [PubMed]
- Rekhtman N, Ang DC, Riely GJ, et al. KRAS mutations are associated with solid growth pattern and tumor-infiltrating leukocytes in lung adenocarcinoma. Mod Pathol 2013;26:1307-19. [Crossref] [PubMed]
- Tucker ZC, Laguna BA, Moon E, et al. Adjuvant immunotherapy for non-small cell lung cancer. Cancer Treat Rev 2012;38:650-61. [Crossref] [PubMed]
- Chalela R, Curull V, Enríquez C, et al. Lung adenocarcinoma: from molecular basis to genome-guided therapy and immunotherapy. J Thorac Dis 2017;9:2142-58. [Crossref] [PubMed]
- Yang Z, Peng Y, Guo W, et al. PD-L1 and CD47 co-expression predicts survival and enlightens future dual-targeting immunotherapy in non-small cell lung cancer. Thorac Cancer 2021;12:1743-51. [Crossref] [PubMed]