CT-based three-dimensional invasiveness analysis of adenocarcinoma presenting as pure ground-glass nodules
Highlight box
Key findings
• This study found a significant correlation between CT density and histological invasiveness in pure GGNs, indicating that CT density may serve as a useful tool for predicting the invasiveness of these nodules.
What is known and what is new?
• It is known that pure GGNs can be either minimally invasive adenocarcinoma or invasive adenocarcinoma. This study adds to the existing knowledge by identifying CT density as a potential predictor of histological invasiveness in pure GGNs, which can inform clinical decision-making.
What is the implication, and what should change now?
• Clinicians may use CT density as a tool for predicting the invasiveness of pure GGNs, which can help guide the decision to perform surgery or follow up with imaging. However, further research is needed to validate.
Introduction
Lung cancer remains the leading cause of cancer and cancer mortality worldwide (1). Ground glass nodules (GGNs) are increasingly detected during low-dose computed tomography (LDCT) lung cancer screening. The National Lung Screening Trial Research Team reported that the screening adherence rates were >90% and the positivity rates 24.2% (2). GGNs can be divided into part-solid and pure GGNs on chest CT. Part-solid GGNs exhibit both GG opacity and solid component but pure GGNs lack the latter components (3). Several guidelines are used to manage GGNs evident on CT. For pure GGNs, the guidelines of the American College of Chest Physicians recommend annual chest CT surveillance of at least 3 years >5 mm in size and note that early follow-up (at 3 months) may be indicated for pure GGNs >10 mm in size. If nodules persist during follow-up, nonsurgical biopsy or surgical resection is recommended (4). These suggestions are consistent with the Fleischner Society recommendations (5). The Japanese Society for CT Screening recommends definitive diagnosis of GGNs >15 mm in maximal diameter on CT (6). Such lesions may be non-invasive adenocarcinomas (NIAs) [such as adenocarcinomas in situ (AIS)] or precancerous lesions (such as atypical adenomatous hyperplasia). However, some are diagnosed as invasive adenocarcinomas (IAs) or as lesions that will develop into IAs. Such lesions should be followed-up via LDCT or resected for definitive diagnosis or treatment (7).
In histological studies, about 12% to 39% of pure GGNs were IAs (8-11). Studies seeking risk factors for IA among pure GGNs reported that the maximal GGN diameter was only one of several predictors (8,12). It was emphasized that surgical resection should be considered; this affords both an excellent prognosis and an accurate diagnosis. The risk of an IA is high when a pure GGN is >10 mm in size.
It is important to differentiate IAs from noninvasive lesions prior to surgery; this determines the extent of surgery. Percutaneous CT-guided transthoracic needle biopsy (CTNB) is commonly used for preoperative diagnosis of lung cancer. However, the accuracy is only about 50% for lesions <10 mm in size (13,14). Frozen sections are evaluated intraoperatively; Walts et al. examined 224 such sections. The accuracy of frozen section diagnoses was 59% for AIS and 46% for minimally IA (MIA) (15).
The optimal management of pure GGNs remains unclear; no preoperative differential diagnosis of invasiveness has yet been established. Recently, preoperative three-dimensional (3D) analyses have become widely used, especially in the lung cancer context (16,17). However, only a few parameters (such as mass size) have been employed to predict the invasiveness of adenocarcinomas presenting as pure GGNs. We thus sought 3D differences between NIAs and IAs radiologically presenting as pure GGNs in patients who had undergone surgical resection. We used a 3D analysis system to this end. We present the following article in accordance with the STARD reporting checklist (available at https://tcr.amegroups.com/article/view/10.21037/tcr-22-2327/rc).
Methods
Patients
We included patients who underwent surgical resection of pure GGNs from January 2013 to December 2019 in our institution. All subjects were evaluated and screened for study eligibility by the first author (Z.P.) prior to study enrollment. The medical records were retrospectively reviewed and the pathological and CT data were prospectively re-assessed and analyzed. The surgical indications for pure GGNs in our center are: (I) A biopsy-proven pre-malignant lesion or malignancy; (II) a size >15 mm; (III) a size 5–10 mm with an increase in size during follow-up; (IV) a nodule >8 mm in size that does not disappear on follow-up. The study was conducted in accordance with the Declaration of Helsinki (as revised in 2013). The study was approved by the Institutional Review Board of the Chungnam National University Hospital (approval No. 2019-08-034) and individual consent of this retrospective analysis was waived.
Nodule localization and surgical procedure
It is difficult to find small pure GGNs during minimally invasive lung surgery. Commencing in 2017, we used electromagnetic navigation bronchoscopy (ENB) to localize pure GGNs in 32 patients. To find the target lesions, after induction of patient anesthesia in the operating room, we inject indigo carmine under ENB guidance employing a superDimension Navigation System (Medtronic, Minneapolis, MN, USA). We usually use an 8.00-Fr single-lumen E-tube. After the target is located, we change to a double-lumen endotracheal tube (to allow single lung ventilation) and perform surgical resection via single-port or conventional three-port video-assisted thoracoscopic surgery (VATS). Before ENB was available, we anatomically resected all pure GGNs. Today, we prefer to perform ENB-guided limited resection; we perform on-site pathological evaluation (frozen section biopsy) of all cases. If significant evidence of invasiveness is apparent, we prefer to convert to anatomical resection rather than segmentectomy with lymph node sampling.
Nodule assessment
Pathological assessment
Surgical specimens were fixed in 10% (v/v) formalin and embedded in paraffin. Two pathologists blinded to the clinical and radiological findings reviewed the pathological results based on hematoxylin-and-eosin (H&E) and immunohistochemical staining. Pathological evaluation and TNM staging were based on the 2015 World Health Organization classification (18) and the eighth edition of the American Joint Committee on Cancer (AJCC) guidelines (19). Pure GGNs were divided into pathologically NIAs (AISs or MIAs) and IAs.
CT and image assessment
All patients underwent high-resolution CT (HRCT) (SOMATOM Definition Flash, Siemens, Germany) at full inspiration within 2 months before surgery. Axial sections (1.0-mm-thick) of the whole lungs were obtained. The images were viewed using a standard lung window setting [−700 Hounsfield units (HUs) width 1,500 HU], and a mediastinal window setting (50 HU; width 250 HU). A pure GGN was defined as a nodule lacking any solid component in both the lung and mediastinal windows. Two thoracic surgeons and two radiologists re-assessed and analyzed the HRCT scans.
The Synapse Vincent system (Fujifilm Corporation, Tokyo, Japan) was used to analyze the images of pure GGNs. Digital imaging and communication in medicine (DICOM) files were obtained and transferred to the 3D analysis system. Figure 1A shows the CT image of a pure GGN in the right upper lobe, Figure 1B shows the pure GGN in the 3D analysis system. The radiologists manually eliminated vascular and bronchial shadows, and ribs, when the automated measurements contained these structures. The CT density histogram is depicted in Figure 2; this shows the density profile across the tumor. We analyzed CT nodule size and volume, and also derived the maximum, minimum, mean and standard deviations of the CT densities of the 3D image analysis system. We extracted a new CT feature from the CT density histogram: the proportion of regions with high CT densities. We defined a threshold high-CT as θHU. In a pure GGN, volumes with CT densities higher and lower than θHU are denoted vH and vL respectively. Then, the high-density CT volume proportion is:
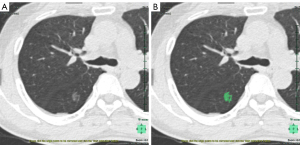
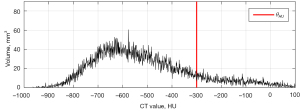
γ can be computed for each pure GGN nodule. For example, in Figure 2, θHU is set to −300 HU and vHand vLare 225.5 and 1,619.4 mm³ respectively; γ is thus 12.2%. We observed that the γ was closely related to the invasiveness of pure GGNs. Therefore, we defined a new parameter, θγ; this is the cutoff used to separate invasive from noninvasive GGNs.
Statistical analysis
SPSS software (ver. 24.0; DDR3 RDIMM; SPSS Inc., Chicago, IL, USA) was used for statistical analysis. The normalities of distributions were explored using the Shapiro-Wilk test. Clinicopathological NIA and IA parameters were compared using the Student’s t-test when they were normally distributed and the Mann-Whitney U-test otherwise. Normally distributed data (factors) are expressed as means ± standard deviations. Non-normally distributed data are expressed as medians with the [25%, 75% quartiles]. A P value <0.05 was considered statistically significant.
θHU, which maximizes the separability of γ between the NIA and IA groups, was determined via receiver operating curve (ROC) analysis. The area under the ROC curve (AUC) and the 95% confidence interval (CI) were calculated. Using θHU, the high-density CT volume proportion was computed for all pure GGNs. Finally, the Youden index was used to determine the optimal cut-off point (θγ) that distinguished invasive from noninvasive GGNs; we evaluated the γ values.
Results
Forty-eight pure GGN lesions were surgically resected in 45 patients treated in our hospital during the study period; three patients (6.7%) underwent resection of two lesions. Of the 48 pure GGNs, 40 were pathologically diagnosed as non-small cell lung cancers (NSCLCs) and 8 as benign lesions (AAH, 6; inflammation, 2). Patient age ranged from 41 to 81 years; 24 (53.3%) had a smoking history. Fifteen (37.5%) nodules were removed via wedge resection, 7 (17.5%) via segmentectomy, and 18 (45%) via lobectomy.
Of the 40 pure GGNs with NSCLCs, 20 (50%) were diagnosed as NIAs (4 AISs and 16 MIAs) and 20 (50%) as IAs. Sublobar resection was performed on 13 (65%) cases in the NIA and 9 (45%) cases in the IA group; all patients underwent R0 resection. In the IA group, nine cases underwent sublobar resections, of which four were wedge resections. Although the pathologies of the first operations (wedge resections) revealed invasive IAs, the second operations (for anatomical resection) could not be completed because of poor lung function (two cases) or patient refusal (two cases). The operative methods and the clinical characteristics (gender, age, and smoking history) are summarized in Table 1. None of gender, age, or smoking history differed between the two groups. There was no significant difference in the GGN maximal diameter (15.1±8.6 vs. 17.1±7.3 mm, P=0.459) or lesional volume (3,018 vs. 2,765 mm3, P=0.894) between the two groups (Table 2). Figure 3 shows the distributions of the 3D densities of two nodules, one from each of the NIA and IA groups. The red and blue curves are the density distributions of an invasive and a noninvasive nodule respectively. We calculated the minimum and maximum densities, the means and standard deviations, and the high-density volume proportions. We used the 3D density histograms to perform these calculations and to analyze the differences between the two groups.
Table 1
Variable | NIA (N=20) | IA (N=20) | P value |
---|---|---|---|
Female, n [%] | 11 [55] | 9 [45] | 0.539 |
Age (years), mean ± SD | 63±9.9 | 62.8±9.5 | 0.949 |
Never smoker, n [%] | 11 [55] | 9 [45] | 0.539 |
Operative method, n [%] | 0.057 | ||
Lobectomy | 7 [35] | 11 [55] | |
Segmentectomy | 2 [10] | 5 [25] | |
Wedge resection | 11 [55] | 4 [20] |
NIA, non-invasive adenocarcinoma; IA, invasive adenocarcinoma; SD, standard deviation.
Table 2
Variable | NIA (N=20) | IA (N=20) | P value |
---|---|---|---|
CT size (mm) | 15.1±8.6 | 17.1±7.3 | 0.459 |
≤10 | 6 | 4 | |
10–19 | 10 | 11 | |
≥20 | 4 | 5 | |
Distance from visceral pleura (mm) | 10.3±10.7 | 8±6.7 | 0.442 |
Lesional volume (mm3) | 3,018 [361, 3,249] | 2,765 [754, 2,977] | 0.894 |
HU max | −102.8±239.4 | 83.6±178.7 | 0.010 |
HU min | −914.0 [−975.8, −871.3] | −900.5 [−959.5, −809.8] | 0.644 |
HU mean | −651.8±76.9 | −520.5±95.6 | <0.005 |
HU SD | 120.1±32.1 | 173.8±34.2 | <0.005 |
Data were presented as mean ± SD, number, or median [range]. NIA, non-invasive adenocarcinoma; IA, invasive adenocarcinoma; CT, computed tomography; HU, Hounsfield unit; SD, standard deviation.
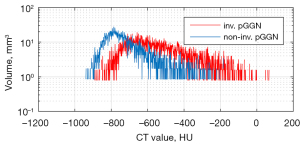
The CT features of the 3D volume histogram are listed in Table 2. We found significant differences in the maximum, and the means and standard deviations, of the CT HU value between NIA and IA GGNs (HU max −102.8±239.4 vs. 83.6±178.7, P=0.01; HU mean −651.8±76.9 vs. −520.5±95.6, P<0.005; HU SD 120.1±32.1 vs. 173.8±34.2, P<0.005). However, there was no significant difference in the minimum CT HU value between the two groups.
Finally, we evaluated the newly proposed CT parameter, thus the proportion of GGN voxels with high CT values. This is denoted by γ, and it changes with the chosen high-CT threshold value θHU. To determine the optimal θHU that differentiates NIA and IA, the γ parameters for different θHU values of each pure GGN were investigated. The γ distributions for the IA and NIA groups at various thresholds were analyzed. The tested thresholds were:
At each , the high-CT value ratios were calculated for all GGNs; the distributions of γ are shown in Table 3. The γ values of the NIA group averaged lower than those of the IA group. The performances at different thresholds were analyzed via ROC analysis. Thus, the AUC of each and the 95% confidence limits were calculated (Table 3). Table 4 shows that ROC analysis afforded a maximum AUC of 0.933 (95% CI: 0.856–1.000) at a θHU of −300 HU; thus, the optimal threshold distinguishing the IA and NIA groups in terms of γ was −300 HU. The ROC curve for prediction of invasiveness by θHU is shown in Figure 4A. Based on this optimal threshold, the best cutoff for dichotomization of the γ values of the two groups was determined using the Youden index. The Youden functions of all possible cut-points are shown in Figure 4B; the optimal cutoff maximizing the Youden function was 5.41%, associated with a sensitivity of 85% and a specificity of 95%.
Table 3
θHU value (HU) | γ of NIA (N=20), [95% CI] | γ of IA (N=20), [95% CI] | P value |
---|---|---|---|
−500 | 14.78 [4.35, 29.06] | 40.46 [27.24, 58.19] | <0.001 |
−450 | 9.03 [2.45, 14.60] | 32.57 [21.34, 49.00] | <0.001 |
−400 | 5.60 [1.10, 9.61] | 25.67 [15.54, 40.64] | <0.001 |
−350 | 3.24 [0.67, 5.11] | 19.87 [11.16, 31.33] | <0.001 |
−300 | 1.96 [0.23, 2.55] | 14.66 [7.72, 19.95] | <0.001 |
−250 | 1.21 [0.00, 1.49] | 10.85 [5.26, 13.95] | <0.001 |
−200 | 0.73 [0.00, 0.95] | 7.61 [2.90, 8.77] | 0.001 |
−150 | 0.38 [0.00, 0.55] | 5.07 [1.36, 5.24] | 0.006 |
−100 | 0.27 [0.00, 0.39] | 3.10 [0.79, 3.13] | 0.023 |
θHU is the threshold CT volume; volumes greater than θHU are vH values; volumes less than θHU are vL values. γ is (vH/vH + vL)×100 [%]. HU, Hounsfield unit; NIA, non-invasive adenocarcinoma; IA, invasive adenocarcinoma.
Table 4
θHU value (HU) | AUC | 95% CI |
---|---|---|
–500 | 0.855 | 0.742–0.968 |
–450 | 0.898 | 0.804–0.991 |
–400 | 0.915 | 0.831–0.999 |
–350 | 0.930 | 0.853–1.000 |
–300 | 0.933 | 0.856–1.000 |
–250 | 0.913 | 0.813–1.000 |
–200 | 0.919 | 0.822–1.000 |
–150 | 0.919 | 0.822–1.000 |
–100 | 0.875 | 0.755–0.995 |
θHU is the threshold computed tomography value. HU, Hounsfield unit; AUC, area under the curve; CI, confidence interval.
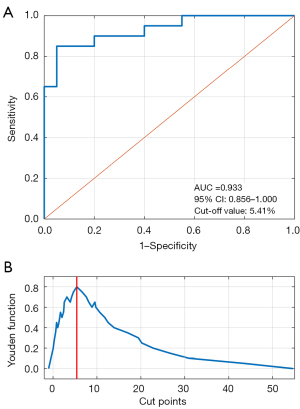
To analyze further the optimal cutoff points for pure GGNs of different sizes, the 40 pure GGNs were divided into three groups: A (0–9 mm), B (10–19 mm), and C (≥20 mm), and the predictive accuracies of the cutoff points were calculated. For Group A (10 pure GGNs), the predictive accuracy was 90%; for Group B (21 pure GGNs), the figure was 95.2%; for Group C (9 pure GGNs), the figure was 77.8%.
Discussion
As more pure GGNs are detected, the optimal treatment of such patients has become a matter of concern (20). The extent of surgical resection is determined by the degree of pathological invasiveness. Pure GGNs are thought to be radiological surrogate markers of pathological non-invasiveness, but this cannot be guaranteed. Sakurai et al. evaluated 291 patients with resected lung cancers ≤1.0 cm in diameter, including 50 pure GGNs. Of the latter, 16% were IAs (21). Thus, a small pure GGN is not necessarily non-invasive. In our present study, 50% (n=20) of pure GGNs were pathological IAs, as were 40% (n=4) of pure GGNs of size ≤1 cm. Therefore, it is very important to predict accurately the invasiveness of pure GGNs.
Here, we developed a new quantitative CT parameter, γ, to predict the invasiveness of pure GGNs. Unlike other studies, we calculated the proportion of the total GGN volume of density >−300 HU and used this to predict pure GGN invasiveness; we then determined the optimal cutoff θγ via ROC curve analysis. Based on the Youden index, θγwas 5.41%, affording a high sensitivity (85%) and specificity (95%). In other words, in a pure GGN, if the volume proportion that is denser than −300 HU is >5.41%, the GGN is more likely to be an IA than not, and lobectomy could be considered. In contrast, in a pure GGN, if the proportion of that volume is <5.41%, it is more likely than not to be an NIA and sublobar resection could be considered.
Other studies have used certain CT parameters to predict the invasiveness of pure GGNs. Several studies found that GGN CT size significantly distinguished preinvasive lesions from IAs (12,22). However, we found that four lesions (20%) <10 mm in size were IAs and 14 (70%) lesions >10 mm in size were NIAs. Thus, lesional size did not predict invasiveness. Unlike the two-dimensional (2D) systems used in previous studies, we employed a 3D system and used the volume proportion >−300 HU to predict the invasiveness of pure GGNs. This reduces the errors associated with 2D radiological evaluations, during which some values are over-emphasized when measuring small lesions. Our predictive accuracies were 90, 95.2, and 77.8% for lesions 0–9, 10–19, and ≥20 mm in size. Our method appropriately evaluates lesions of all sizes, especially those <10 mm.
Ichinose et al. evaluated 180 patients with pure GGNs who underwent surgical resection, and found a that higher maximum CT value (≥−300 HU) usefully predicted histological invasiveness (23). Another study on 66 NIA and 30 IA cases found the mean 3D attenuation value distinguished pre-invasive lesions and MIAs from IAs. The AUC for IA prediction at a mean 3D CT attenuation of 0.838 exhibited a cutoff of −489 HU (24). This method, combined with our method, would improve the detection of pure GGN IAs.
Scholten et al. semiautomatically evaluated 115 non-solid and part-solid nodules and found that an HU threshold of −300 HU afforded a sensitivity of 90%, a specificity of 88%, a positive predictive value of 96%, and a negative predictive value of 72% (25). Another study analyzed 127 surgically resected lungs with subsolid nodules using a United Imaging workstation; the presence of a solid component with a threshold of −300 HU predicted pathological malignancy (26). We evaluated only pure GGNs; the −300 HU threshold was also applicable. We hope that our work will aid the clinical diagnosis and surgical management of pure GGNs.
Recently, less-invasive methods such as electromagnetic navigation and bronchoscope-guided target lesion localization have allowed surgeons to perform minimally invasive limited resections that preserve lung function in patients with early-stage lung adenocarcinomas (27). As adenocarcinoma invasiveness is reflected by the CT features of pure GGNs, it is now possible to perform more limited resections in patients with NIA.
Our work has certain limitations. First, this was a retrospective study performed in a single center. Second, the number of lesions was rather small and we included only patients undergoing surgery. Third, the GGN voxels measured automatically by the 3D image analysis system contained vascular and bronchial shadows, which the radiologists manually eliminated. However, small shadows could not be completely removed; it is possible that these affected the GGN HU values.
Conclusions
In conclusion, analyses of CT volumes using the 3D system suggest a relationship between such volumes and pure GGN invasiveness. The pure GGN volume proportion of density >−300 HU may predict histological invasiveness; a cutoff of 5.41% affords high sensitivity and specificity. Further, multicenter prospective studies on pure GGNs are required to determine the clinical utility of the metric.
Acknowledgments
Funding: This work was supported by
Footnote
Reporting Checklist: The authors have completed the STARD reporting checklist. Available at https://tcr.amegroups.com/article/view/10.21037/tcr-22-2327/rc
Data Sharing Statement: Available at https://tcr.amegroups.com/article/view/10.21037/tcr-22-2327/dss
Peer Review File: Available at https://tcr.amegroups.com/article/view/10.21037/tcr-22-2327/prf
Conflicts of Interest: All authors have completed the ICMJE uniform disclosure form (available at https://tcr.amegroups.com/article/view/10.21037/tcr-22-2327/coif). The authors have no conflicts of interests to declare.
Ethical Statement: The authors are accountable for all aspects of the work and in ensuring that questions related to the accuracy or integrity of any part of the work are appropriately investigated and resolved. The study was conducted in accordance with the Declaration of Helsinki (as revised in 2013). The study was approved by Institutional Review Board of the Chungnam National University Hospital (approval No. 2019-08-034) and individual consent of this retrospective analysis was waived.
Open Access Statement: This is an Open Access article distributed in accordance with the Creative Commons Attribution-NonCommercial-NoDerivs 4.0 International License (CC BY-NC-ND 4.0), which permits the non-commercial replication and distribution of the article with the strict proviso that no changes or edits are made and the original work is properly cited (including links to both the formal publication through the relevant DOI and the license). See: https://creativecommons.org/licenses/by-nc-nd/4.0/.
References
- Khazaei Z, Jarrahi AM, Momenabadi V, et al. Global cancer statistics 2018: GLOBOCAN estimates of incidence and mortality worldwide stomach cancers and their relationship with the human development index (HDI). World Cancer Res J 2019;6:e1257.
- National Lung Screening Trial Research Team. Reduced lung-cancer mortality with low-dose computed tomographic screening. N Engl J Med 2011;365:395-409. [Crossref] [PubMed]
- Kim HY, Shim YM, Lee KS, et al. Persistent pulmonary nodular ground-glass opacity at thin-section CT: histopathologic comparisons. Radiology 2007;245:267-75. [Crossref] [PubMed]
- Gould MK, Donington J, Lynch WR, et al. Evaluation of individuals with pulmonary nodules: when is it lung cancer? Diagnosis and management of lung cancer, 3rd ed: American College of Chest Physicians evidence-based clinical practice guidelines. Chest 2013;143:e93S-e120S.
- MacMahon H, Naidich DP, Goo JM, et al. Guidelines for Management of Incidental Pulmonary Nodules Detected on CT Images: From the Fleischner Society 2017. Radiology 2017;284:228-43. [Crossref] [PubMed]
- Nawa T, Nakagawa T, Mizoue T, et al. Low-dose computed tomography screening in Japan. J Thorac Imaging 2015;30:108-14. [Crossref] [PubMed]
- Koike T, Kitahara A, Sato S, et al. Lobectomy Versus Segmentectomy in Radiologically Pure Solid Small-Sized Non-Small Cell Lung Cancer. Ann Thorac Surg 2016;101:1354-60. [Crossref] [PubMed]
- Lim HJ, Ahn S, Lee KS, et al. Persistent pure ground-glass opacity lung nodules ≥ 10 mm in diameter at CT scan: histopathologic comparisons and prognostic implications. Chest 2013;144:1291-9. [Crossref] [PubMed]
- Ichinose J, Kohno T, Fujimori S, et al. Invasiveness and malignant potential of pulmonary lesions presenting as pure ground-glass opacities. Ann Thorac Cardiovasc Surg 2014;20:347-52. [Crossref] [PubMed]
- Heidinger BH, Anderson KR, Nemec U, et al. Lung Adenocarcinoma Manifesting as Pure Ground-Glass Nodules: Correlating CT Size, Volume, Density, and Roundness with Histopathologic Invasion and Size. J Thorac Oncol 2017;12:1288-98. [Crossref] [PubMed]
- She Y, Zhao L, Dai C, et al. Preoperative nomogram for identifying invasive pulmonary adenocarcinoma in patients with pure ground-glass nodule: A multi-institutional study. Oncotarget 2017;8:17229-38. [Crossref] [PubMed]
- Lee SM, Park CM, Goo JM, et al. Invasive pulmonary adenocarcinomas versus preinvasive lesions appearing as ground-glass nodules: differentiation by using CT features. Radiology 2013;268:265-73. [Crossref] [PubMed]
- Ohno Y, Hatabu H, Takenaka D, et al. CT-guided transthoracic needle aspiration biopsy of small (< or = 20 mm) solitary pulmonary nodules. AJR Am J Roentgenol 2003;180:1665-9. [Crossref] [PubMed]
- Shimizu K, Ikeda N, Tsuboi M, et al. Percutaneous CT-guided fine needle aspiration for lung cancer smaller than 2 cm and revealed by ground-glass opacity at CT. Lung Cancer 2006;51:173-9. [Crossref] [PubMed]
- Walts AE, Marchevsky AM. Root cause analysis of problems in the frozen section diagnosis of in situ, minimally invasive, and invasive adenocarcinoma of the lung. Arch Pathol Lab Med 2012;136:1515-21. [Crossref] [PubMed]
- Ikeda N, Yoshimura A, Hagiwara M, et al. Three dimensional computed tomography lung modeling is useful in simulation and navigation of lung cancer surgery. Ann Thorac Cardiovasc Surg 2013;19:1-5. [Crossref] [PubMed]
- Hagiwara M, Shimada Y, Kato Y, et al. High-quality 3-dimensional image simulation for pulmonary lobectomy and segmentectomy: results of preoperative assessment of pulmonary vessels and short-term surgical outcomes in consecutive patients undergoing video-assisted thoracic surgery†. Eur J Cardiothorac Surg 2014;46:e120-6. [Crossref] [PubMed]
- Travis WD, Brambilla E, Nicholson AG, et al. The 2015 World Health Organization Classification of Lung Tumors: Impact of Genetic, Clinical and Radiologic Advances Since the 2004 Classification. J Thorac Oncol 2015;10:1243-60. [Crossref] [PubMed]
- Amin MB, Greene FL, Edge SB, et al. The Eighth Edition AJCC Cancer Staging Manual: Continuing to build a bridge from a population-based to a more "personalized" approach to cancer staging. CA Cancer J Clin 2017;67:93-9.
- Zhao H, Marshall HM, Yang IA, et al. Screen-detected subsolid pulmonary nodules: long-term follow-up and application of the PanCan lung cancer risk prediction model. Br J Radiol 2016;89:20160016. [Crossref] [PubMed]
- Sakurai H, Nakagawa K, Watanabe S, et al. Clinicopathologic features of resected subcentimeter lung cancer. Ann Thorac Surg 2015;99:1731-8. [Crossref] [PubMed]
- Yu Y, Cheng JJ, Li JY, et al. Determining the invasiveness of pure ground-glass nodules using dual-energy spectral computed tomography. Transl Lung Cancer Res 2020;9:484-95. [Crossref] [PubMed]
- Ichinose J, Kawaguchi Y, Nakao M, et al. Utility of Maximum CT Value in Predicting the Invasiveness of Pure Ground-Glass Nodules. Clin Lung Cancer 2020;21:281-7. [Crossref] [PubMed]
- Kitazawa S, Saeki Y, Kobayashi N, et al. Three-dimensional mean CT attenuation value of pure and part-solid ground-glass lung nodules may predict invasiveness in early adenocarcinoma. Clin Radiol 2019;74:944-9. [Crossref] [PubMed]
- Scholten ET, Jacobs C, van Ginneken B, et al. Detection and quantification of the solid component in pulmonary subsolid nodules by semiautomatic segmentation. Eur Radiol 2015;25:488-96. [Crossref] [PubMed]
- Li Q, Gu YF, Fan L, et al. Effect of CT window settings on size measurements of the solid component in subsolid nodules: evaluation of prediction efficacy of the degree of pathological malignancy in lung adenocarcinoma. Br J Radiol 2018;91:20180251. [Crossref] [PubMed]
- Piao Z, Han SJ, Cho HJ, et al. Feasibility of electromagnetic navigation bronchoscopy-guided lung resection for pulmonary ground-glass opacity nodules. J Thorac Dis 2020;12:2467-73. [Crossref] [PubMed]