Integrated analysis of coexpression and a tumor-specific ceRNA network revealed a potential prognostic biomarker in breast cancer
Highlight box
Key findings
• A potential prognostic marker for breast cancer was identified.
What is known and what is new?
• The ceRNA plays a role in the molecular biological mechanisms related to the occurrence and development of cancer.
• We identified the HOTAIR-miR-130a-3p-HMGB3 axis as a potential prognostic marker in breast cancer.
What is the implication, and what should change now?
• Further experimental studies are needed to confirm the underlying biological regulatory mechanism.
Introduction
As one of the most common malignant tumors, breast cancer ranks first as a cause of death for women aged 20 to 59 years (1). According to the 2021 statistics from the World Health Organization, the incidence of breast cancer has reached 11.7%, accounting for 6.9% of total global deaths (2). Currently, the prognosis of breast cancer still remains poor despite advances in surgical techniques, chemotherapy, and radiotherapy (3). Therefore, it is of increasing urgency to identify novel molecules for tumor intervention and further improve the prognosis and treatment for those with breast cancer.
With more than 200 nucleotides, long non-coding RNAs (lncRNAs) constitute a group of important regulatory RNAs of low or no protein-coding capacity (4). They act as regulators in many intracellular biological processes (BPs), including tumor cell metastasis, proliferation, apoptosis, and immune response (5-8). In addition, lncRNA’s interactions with messenger RNAs (mRNAs) or microRNAs (miRNAs) also have an important effect on tumorigenesis and tumor biological features (9-12). The mechanism of competing endogenous RNAs (ceRNA), proposed by Salmena et al., asserts that when competing for shared miRNAs, transcripts may cross-regulate each other (13). This hypothesis has been proven by experiments on many malignancies, including liver, colon, lung, pancreas, and breast cancers (14-18).
Weighted gene coexpression network analysis (WGCNA) has gained popularity as a systematic biological method due to its ability to locate the highly correlated gene modules through the construction of a free-scale gene coexpression network (19) and the correlation of selected module of significant genes with clinical traits, which is useful for identifying potential biomarkers. Thus far, many researches have applied this method in both neoplastic and nonneoplastic diseases, such as cancer and system diseases of the heart and nerves (20-22). Xu et al. revealed the commonness between bladder cancer and breast cancer through comprehensive analysis of mRNA, miRNA, and lncRNA maps (23). Li et al. analyzed the multi-level expression profile of bladder urothelial carcinoma (BUC) using the TCGA database, constructed a ceRNA regulatory network by integrating information on tumor progression and prognosis, and determined a lncRNA-miRNA-mRNA regulated ceRNA network, including 2 lncRNAs, 1 miRNA, and 14 mRNA (24). Previous studies have demonstrated that WGCNA can be used to identify genes associated with the progression and prognosis of BRCA. For example, Yao et al. downloaded the LncRNA, mRNA and miRNA expression profiles of breast cancer from the TCGA database, and used weighted gene co-expression network analysis to generate 23 and 5 modules, respectively. According to the green module and the blue module, five lncRNAs related to BRCA progress were identified, which were identified as important prognostic factors for cancer patients (25).
In this study, breast cancer expression profiles of lncRNAs, miRNAs, and mRNAs and their corresponding clinical data were extracted from The Cancer Genome Atlas (TCGA) (26) and Genotype-Tissue Expression (GTEx) databases (27). Following this, the cancer-associated modules were recognized through WGCNA. Together with the differential expression results, univariate Cox analysis was used to select the common RNAs related to the overall survival (OS) of patients, and these RNA were then used to construct a breast cancer-associated ceRNA network. Finally, we constructed a multivariable Cox regression model and identified the HOX antisense intergenic RNA (HOTAIR)-miR-130a-3p-high mobility group-box 3 (HMGB3) axis as a potential biomarker for OS, which may provide management and surveillance information for breast cancer. We present the following article in accordance with the TRIPOD reporting checklist (available at https://tcr.amegroups.com/article/view/10.21037/tcr-23-313/rc).
Methods
Data acquisition and preprocessing
The RNA-sequencing (RNA-seq; via an Illumina HiSeq RNA-Seq platform) and miRNA expression profiles (via an Illumina HiSeq miRNA-Seq platform) of breast cancer were acquired from TCGA database (https://portal.gdc.cancer.gov/). The study was conducted in accordance with the Declaration of Helsinki (as revised in 2013). To solve the problem of limited normal samples in TCGA, the RNA-seq data sets were combined with GTEx, which comprises normal breast tissues from deceased noncancerous individuals. To improve the compatibility of data from different sources, the University of California, Santa Cruz (UCSC), Xena project (https://xena.ucsc.edu/), which recomputed and normalized all expression raw data based on a standard pipeline, was used to download the RNA-seq data with expectation maximization (RSEM) and transcripts per million (TPM) expression values (28,29). Subsequently, the RNA-seq data were transformed into mRNAs (protein coding) and lncRNAs (antisense; long intergenic non-coding RNAs (ncRNAs); macro lncRNAs; noncoding, 3’ overlapping ncRNAs; sense intronic, sense overlapping, bidirectional promoter lncRNAs; and processed transcripts) with a human gene annotation file from GENCODE (https://www.gencodegenes.org/human/) (30). The RNA-seq cohort was composed of 1,098 breast cancer and 291 normal tissues. Meanwhile, the miRNA dataset of raw count values including 103 pairs of breast tumor tissues and their paracarcinoma tissues was downloaded through the gdc-client tool (TCGA; https://portal.gdc.cancer.gov/). All clinical data from the corresponding patients were also collected via gdc-client.
Construction of a weighted gene coexpression network
The TPM expression values of mRNAs and lncRNAs were first transformed with log2(TPM +0.001). Then, unqualified RNAs were removed with the “goodSamplesGenes” function (https://www.rproject.org). Based on squared Euclidean distance, a sample network was then constructed, and Z.k <−2.5 was used as the threshold to filter out unqualified samples for subsequent studies. Furthermore, mRNAs and lncRNAs with over 60% variance across the remaining samples were put through WGCNA. Next, based on a scale-free topology criterion of R2>0.85, a proper soft-threshold power (β) was chosen with the “pickSoftThreshold” function (https://www.rproject.org), and the weighted adjacency matrix was established. After this, the topological overlap matrix (TOM) was reconstructed. The dynamic tree cut method was adopted to generate the modules of coexpressed RNAs with 3 major parameters in WGCNA: a minModuleSize of 30, a mergeCutHeight of 0.25, and a maxBlockSize of 12,000. Finally, these mRNAs and lncRNAs with high correlations were separately grouped into coexpression modules.
Differential expression analysis of RNAs
To increase the accuracy of this study, the three popular R packages (The R Foundation of Statistical Computing, USA), “limma” (version 3.42.0), “edgeR” (version 3.28.0), and “DESeq2” (version 1.26.0), were selected to discern the differentially expressed (DE)RNAs (31-33). The RSEM expected counts for mRNAs and lncRNAs and the raw counts for miRNAs were passed to the three R packages with the criteria of false discovery rate (FDR) <0.05 and |log2fold change (FC)| >1. After the RNAs with low expression values were filtered out, the DE RNAs were displayed in Venn plots, and the overlapping RNAs of the three packages were considered suitable for further analysis.
Function and pathway enrichment analysis
To perform the Gene Ontology (GO) and Kyoto Encyclopedia of Genes and Genomes (KEGG) enrichment analysis, the R package “clusterProfiler” (version 3.14.0) was used for determining the mRNA overlap according to WGCNA and the differential expression analysis (34). The three GO aspects included BP, molecular function (MF), and cellular component (CC). A pathway with an adjusted P value <0.05 was considered reasonable.
Univariate Cox analysis of RNAs
To maintain the reliability of the study, 26 samples without full clinical survival information were removed from all 1,098 TCGA patients with breast cancer. After intersecting the results of WGCNA and DERNAs, we used univariate Cox regression analysis to determine the correlation between the RNAs and the OS of 1,072 patients. The OS was considered to be the period between first-visit diagnosis and the most recent follow-up or death of any cause. The R package “survival” (version 3.2.3) was used to identify the prognosis-associated RNAs at a P value <0.05.
Construction of lncRNA-miRNA-mRNA ceRNA network
Based on the results of univariate Cox analysis, a 3-stage ceRNA network was constructed with prognosis-related mRNAs, lncRNAs and miRNAs. (I) In order to interact mRNAs and miRNAs in a reliable way, the R package “multiMiR” (version 1.10.0) was employed, which compiled nearly 50 million records and contains 14 validated and forecasted databases, including miRTarBase, TargetScan, and miRDB (35-38). (II) To obtain the miRNA-targeted lncRNAs, the miRNATarget data from starBase (version 2.0) was downloaded (https://starbase.sysu.edu.cn/) (39). (III) To construct the ceRNA network, the mRNA-miRNA and miRNA-lncRNA pairs were visualized with Cytoscape software (version 3.8.0) (40).
Construction and assessment of the prognostic risk model
First, the 1,072 patients with full clinical data were randomly divided in a 1:1 ratio into two groups (the training set =536; validation set =536). Next, for model construction, the expression level of the survival-related RNAs in the training set were used to establish a prognostic risk formula by means of multivariable Cox regression analysis. The risk signal of each patient was calculated using the regression coefficient-weighted expression, and a risk score formula was devised as follows: (41). In this formula, n is the number of selected RNAs, Expi is the expression value of each RNA, and Coei is the multivariate Cox regression coefficient. The effectiveness of this model was roughly estimated by calculating the concordance index (C-index). Finally, for detailed model assessment, all patients of both the training set and validation set were divided into high- and low-risk groups according to the median risk score. The OS curves were generated by Kaplan-Meier analysis according to the low-risk and high-risk groups as mentioned above. A P value less than 0.05 was considered to be statistically significant. The expression profiles of key RNAs in the high-risk and low-risk groups were plotted using a risk heatmap. To estimate the diagnostic accuracy on the basis of the risk score for 3- and 5-year OS probability, a time-dependent receiver operating characteristic (ROC) curve was adopted.
Investigation of the RNA expression and DNA methylation level in the prognostic risk model
To verify the RNA expression in breast cancer, RNA-seq and miRNA-seq expression data were downloaded from the National Center for Biotechnology Information (NCBI) Gene Expression Omnibus (GEO) (https://gtexportal.org/home/). The GSE65216 data set includes the mRNA and lncRNA expression data from 11 normal and 167 breast cancer samples, and the GSE26659 data set includes the miRNA expression data from 11 normal and 77 breast cancer samples. To explore the expression changes between tumor and normal samples in other cancer types, UCSC Xena and gdc-client were used for RNA-seq and miRNA-seq respectively to retrieve pan-cancer count data from TCGA, which were further normalized with log2(count +1). Additionally, using gdc-client, we acquired TCGA somatic mutation and DNA methylation data to clarify the mechanism of the RNA expression changes. The R package “maftools” was used to detect mRNA mutation in the patients with breast cancer (42). For DNA methylation analysis, we conducted a t-test to check if the methylation beta values of mRNA and lncRNA were lower in tumors and to calculate the Pearson correlation coefficient between RNA expression and the DNA methylation level.
Statistical analysis
All statistical analyses were performed under R version 3.5.3. Classification variables are expressed in numbers and percentages. The differences between groups were compared using chi-square test. Continuous variables are expressed as median or mean standard deviation. The survival time distribution was evaluated using the Kaplan Meier method, and the logarithmic rank test was used for comparison. The Cox proportional risk model was used for univariate and multivariate analysis to determine the contribution of these variables. Predicted mRNA and lncRNA were tested using hypergeometric and Pearson correlation tests. The difference of P<0.05 was statistically significant.
Results
Clinical patient characteristics
The workflow for our study is illustrated in Figure 1, while Table 1 summarizes the detailed clinical and pathological features of the study population. All 1,098 patients were pathologically diagnosed with breast cancer, the median age was 58.0 (interquartile range, 49–67) years, and 69.1% of the patients were White. An absolute female predominance was observed (98.9% female).
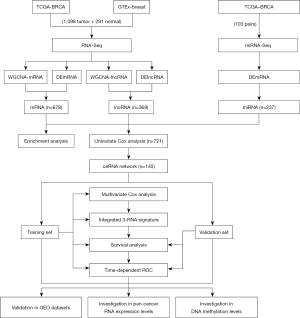
Table 1
Characteristics | Alive (n=944) | Deceased (n=154) | Overall (n=1,098) |
---|---|---|---|
Gender, n (%) | |||
Female | 933 (98.8) | 153 (99.4) | 1,086 (98.9) |
Male | 11 (1.2) | 1 (0.6) | 12 (1.1) |
Age (years) | |||
Mean (SD) | 58.0 (12.8) | 60.8 (15.2) | 58.4 (13.2) |
Median [min, max] | 58.0 [26.0, 90.0] | 62.0 [26.0, 90.0] | 58.0 [26.0, 90.0] |
Missing, n (%) | 1 (0.1) | 0 (0.0) | 1 (0.1) |
Race, n (%) | |||
White | 645 (68.3) | 114 (74.0) | 759 (69.1) |
Black | 153 (16.2) | 30 (19.5) | 183 (16.7) |
Other | 146 (15.5) | 10 (6.5) | 156 (14.2) |
Stage, n (%) | |||
0 | 7 (0.7) | 4 (2.6) | 11 (1.0) |
I | 166 (17.6) | 16 (10.4) | 182 (16.6) |
II | 554 (58.7) | 68 (44.2) | 622 (56.6) |
III | 206 (21.8) | 44 (28.6) | 250 (22.8) |
IV | 5 (0.5) | 15 (9.7) | 20 (1.8) |
X | 6 (0.6) | 7 (4.5) | 13 (1.2) |
SD, standard deviation.
Identification of key modules with WGCNA
After removal of the unqualified samples and outlying genes, WGCNA was performed on the top 60% of the variances of mRNAs (11,772 mRNAs) and lncRNAs (8,814 lncRNAs). When the scale-free topology fit index was over 0.85, the soft-power thresholding was set to 5 and 3 for mRNAs and lncRNAs respectively (Figure 2A,2B). Ultimately, in the mRNA and lncRNA coexpression network, there were a total of 16 and 12 modules generated, respectively (Figure 2C,2D). Additionally, it is worth noting that we calculated and plotted the relation of each module with its corresponding clinical traits, which is shown in Figure 2E,2F. It is clear that the most specific mRNA network is the blue module, with a correlation coefficient of 0.8 (P=5×10−309); the magenta and brown modules in the lncRNAs network had a correlation coefficient of 0.73 (P=2×10−225) and 0.67 (P=2×10−175), respectively.
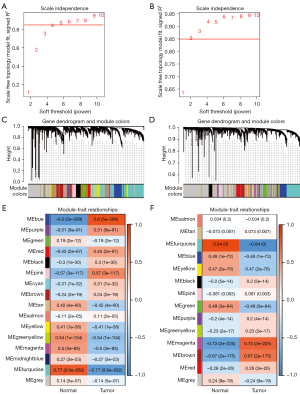
Differential RNA expression analysis
We used three popular methods to compare breast cancer with normal tissues so as to identify the DEmRNAs, DElncRNAs, and DEmiRNAs. To improve the reliability of the study, we chose the overlapping results with the R packages “DESeq2”, “edgeR”, and “limma”. As shown in Figure 3A-3C, we obtained 4,352 overlapping DEmRNAs (2,287 upregulated and 2,065 downregulated), 1,883 overlapping DElncRNAs (778 upregulated and 1,105 downregulated), and 237 overlapping DEmiRNAs (136 upregulated and 101 downregulated).
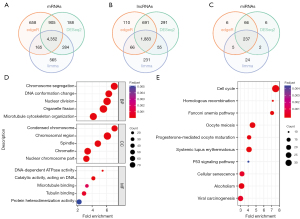
Functional annotation of mRNAs overlapping between the WGCNA and differential expression analysis
In total, 678 mRNAs were intersected between the WGCNA and differential expression analysis. To further explore the potential biological functions of mRNAs in breast cancer, we performed GO and KEGG pathway analysis using the R package “clusterProfiler”. Based on the GO analysis, we identified that mRNAs were mostly enriched in nuclear division and chromosome segregation of BP; the chromosome region the most enriched term of CC; adenosine triphosphatases (ATPases) activity was the most enriched term of MF (Figure 3D). Moreover, in the KEGG analysis, cell cycle, cellular senescence, and p53 signaling were enriched as the important pathways for common mRNAs (Figure 3E).
Construction and analysis of the ceRNA network
We built a lncRNA-miRNA-mRNA (ceRNA) network to understand how lncRNA regulates mRNA through combination with miRNA in human breast cancer. First, we performed univariate Cox regression analysis and a log-rank test to estimate the prognostic relationship between the RNA expression profiles and OS of 1,072 patients. Second, we selected 180 lncRNAs, 120 miRNAs, and 421 mRNAs with a P value <0.05, among which we found that 418 mRNAs interacted with 109 miRNAs in the ceRNA network according to the R package “multiMiR”. Meanwhile, a total of 14 miRNA-targeted lncRNAs based on the 32 miRNAs was predicted through starBase. Consequently, we performed hypergeometric and Pearson correlation tests on the predicted mRNAs and lncRNAs. Finally, 9 lncRNA nodes, 110 mRNA nodes, 26 miRNA nodes, and 416 edges were preserved in our network (Figure 4).
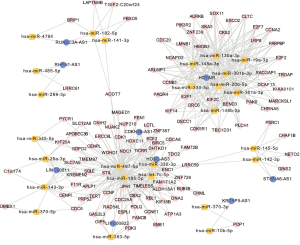
Prognostic risk model construction
Based on the training set, we first used multivariable Cox regression analysis on all 770 interactions among the lncRNAs, miRNAs, and mRNAs in the ceRNA network and obtained three RNAs (HMGB3, HOTAIR, and miR-130a-3p) as potential prognostic biomarkers for patients with breast cancer. Afterward, we selected the three RNAs to construct a risk factor prediction model, with the risk scores of the individual samples being calculated with the following formula: risk score = (0.297 × expression value of HMGB3) + (0.076 × expression value of HOTAIR) + (−0.135 × expression value of miR-130a-3p). Next, the boxplot and Kaplan-Meier plot for the three RNAs showed that HMGB3 and HOTAIR were upregulated and associated with poor prognosis (Figure 5A,5B), while miR-130a-3p was downregulated and associated with good prognosis (Figure 5C). The hazard ratios (HRs) for the integrated 3-RNA model are shown in Figure 5D. The HR results showed that HMGB3 (HR =1.3462) and HOTAIR (HR =1.0786) were hazardous factors, while miR-130a-3p (HR =0.8736) was a protective one. The value of the C-index was 0.722 [95% confidence interval (CI): 0.632–0.812], indicating that the HOTAIR-miR-130a-3p-HMGB3 model may be effective biomarker in the prognosis of breast cancer.
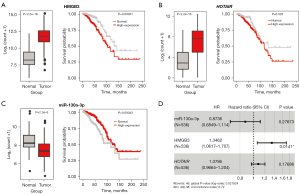
Assessment of the prognostic risk model in the training and validation sets
In order to prove the robustness of the prognosis model for patients with breast cancer, we created Kaplan-Meier curves of OS in the training set. It was found that the median cutoff risk score could best differentiate between the low-risk and high-risk groups, and the two groups were thus categorized. Kaplan-Meier analysis showed that the high-risk (n=268) patients differed significantly from low-risk patients (n=268), in that the high-risk group tended to have a lower OS rate (log-rank test, P value =0.0065; Figure 6A). The risk score panel demonstrated the risk stratification, survival information, and expression values of the three RNAs in the training set. It was observed that patients in the high-risk group had the overexpression of HMGB3 and HOTAIR and the under expression of miR-130a-3p (Figure 6B). The prognostic ability of the 3-RNA prediction model was assessed by calculating the area under the ROC curve (AUC); a higher AUC indicated the better performance of the model. The 3- and 5-year survival AUCs of the three RNA biomarkers in the training set were 0.686 and 0.653, respectively, indicating a good sensitivity and specificity of the prediction model (Figure 6C). The validation set also showed distinctive differences between the high-risk group and low-risk group, including survival curves (Figure 6D), the risk score panel (Figure 6E), and the time-ROC curve (Figure 6F). As expected, the results in the validation set were consistent with those in the training set, indicating the robustness of the 3-RNA prognostic risk model.
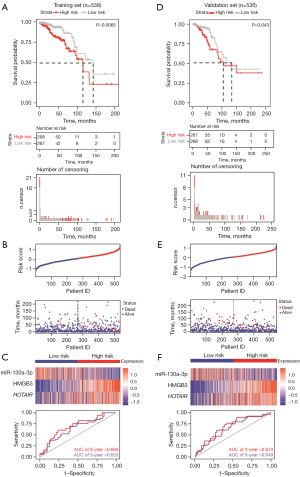
Investigation of RNA expression and DNA methylation level for the HOTAIR-miR-130a-3p-HMGB3 axis
To verify the expression of the HOTAIR-miR-130a-3p-HMGB3 axis in breast cancer, we downloaded two GEO data sets and found both HOTAIR and HMGB3 were upregulated while miR-130a-3p was downregulated, indicating consistency with our previous result (43). Next, for the 3 RNAs, we drew boxplots of the global expression in 22 other TCGA cancer types. This revealed that the expression pattern of the HOTAIR-miR-130a-3p-HMGB3 axis in invasive breast carcinoma (BRCA) also occurred in kidney renal papillary cell carcinoma (KIRP) (Figure S1). According to the mutation analysis result, only 3 in 1,098 patients had the HMGB3 somatic mutation, so we excluded the assumption that a somatic mutation directly leads to the expression changes of RNA. In addition, we investigated the DNA methylation level of HMGB3 and HOTAIR, which contained 16 and 37 valid CpG sites according to the Illumina Human DNA Methylation 450K microarray, respectively. The DNA methylation result demonstrated that 11 cytosine-phosphate-guanine (CpG) sites for HMGB3 and 4 CpG sites for HOTAIR were significantly hypomethylated (Figure S2A), and they were negatively correlated with RNA expression (Figure S2B), indicating that the upregulation of HMGB3 and HOTAIR expression in our prognostic risk model may be attributable to hypomethylation.
Discussion
As one of the most common malignancies in the world, breast cancer has a poorer prognosis and a higher rate of distant metastasis compared with other cancer types. Due to a lack of effective therapeutic targets and biomarkers, the OS rate of breast cancer patients remains low. Thus, we endeavored to locate novel markers to help the early screening and prognosis of breast cancer.
Recently, a growing number of ceRNA network studies exploring the process of diseases, particularly on human tumors, have been conducted. The ceRNA hypothesis proposes that in order to compete for shared miRNAs, lncRNAs can bind with the miRNAs like a “sponge”, and thus indirectly regulate the downstream target genes of miRNAs (13). The RoR-miR-145-ARF6 axis was found to be one of the more important ceRNA networks related to triple-negative breast cancer (TNBC) by Eades et al., and it contributes to tumor invasion and metastasis through disrupting cell-cell adhesion (44). The lncRNA TINCR was shown to modulate oncogenic KLF4 expression via competing with miR-7 and thus promoting the formation and progression of breast cancer (45). An oncogenic lncRNA, PTENP1, was found to upregulate p53 and PTEN via interacting with miR-19b and to downregulate p-AKT (46). Chou et al. found that like a sponge of miR-1, MALAT1 regulated the expression of its target gene CDC42 and reduced the migration and invasion of breast cancer cells (47). Peng et al. demonstrated that for patients with metastasized breast cancer tumors and poor OS, H19, and LIN28 were remarkably overexpressed both in vivo and in vitro (48).
DNA methylation modification is a hot research topic in epigenetics. It is involved in regulating gene expression, gene silencing, DNA damage repair, and cancer, and other important BPs, gene methylation is mainly in the areas rich in GC base sequence of the CpG, gene under the action of the corresponding methylation transferase regulate the expression of cancer gene, oncogene and DNA damage repair, etc. (49).
In order to explore the potential biomarkers linked with breast cancer, WGCNA, the integrated bioinformatics method, was used to identify the modules related with tumor, including 1 lncRNA/mRNA module (blue) and 2 miRNA modules (brown and magenta). Following this, depending on the lncRNA-miRNA-mRNA interactions, we built a ceRNA network and found that, among the 9 lncRNAs, 110 mRNAs, and 26 miRNAs, the HOTAIR-miR-130a-3p-HMGB3 axis might be the potential prognostic biomarker for patients with breast cancer.
HOTAIR, localized in chromosome 12, interacts with polycomb repressive complex 2 (PRC2) to reprogram the chromatin state and induce cancer metastasis (50,51). Studies have demonstrated that there is a close correlation between HOTAIR and EZH2 expression levels in breast tumor tissues and that a higher HOTAIR level is associated with worse prognosis (52,53). In relevant research of breast cancer, Xue et al. found that HOTAIR accumulated in nuclei and its expression was increased in tamoxifen-resistant breast tumor cells compared to primary, hormone-naive tumor cells (54). Additionally, HOTAIR was shown to be able to sequester miR-206 at the posttranscription level, thus increasing Bcl-2-like protein 2 (Bcl-w) expression and ultimately promoting the growth of breast tumor (55).
Previous studies have shown that miR-130a-3p is directly involved in the development of chemoresistance (56,57), and its abnormal expression was linked to a variety of tumors. In patients with esophageal squamous cell carcinoma, both up- and downregulation of miR-130a-3p significantly increased the sensitivity toward chemotherapy (58). According to the report by Wang et al., miR-130a-3p suppressed cell migration and invasion in gastric carcinoma by directly targeting and decreasing TBL1XR1 and the subsequent epithelial-mesenchymal transition process (59). A relevant study showed that the overexpression of miR-130a-3p in breast cancer stem cells inhibited cellular proliferation, migration, and invasion, while the silencing of miR-130a-3p had the opposite effects. Furthermore, miR-130a-3p was associated with lymph node metastasis and advanced TNM stage (60).
HMGB3, also known as HMG2a, belongs to the family of chromatin-binding proteins and is categorized into the HMGB subfamily with HMGB1 and HMGB2 (61). It was reported that HMGB3 is involved in the progression of certain cancers, including gastric cancer, prostate cancer, hepatocarcinogenesis, and non-small cell lung cancer (62-65). A recent study showed that the high expression of HMGB3 was associated with the proliferation and mammosphere formation of breast tumor cells, with HMGB3 acting as an oncogene to regulate drug resistance (66,67).
Although it has been established that these RNAs are essential in many BPs, little is known about the mechanistic interactions among HOTAIR, miR-130a-3p, and HMGB3. Hu et al. found that miR-130a-3p could take part in a HOTAIR-addressed mechanism in hepatocellular carcinoma (68). He et al. found that HOTAIR promotes the proliferation and invasion/metastasis of breast cancer cells by targeting the miR-130a-3p/Suv39H1 axis (69). According to the PubMed database, records about the interaction of HMGB3 and miR-130a-3p in cancer are not available. We speculate that LncRNA HOTAIR promotes the proliferation and invasion/metastasis of breast cancer (BC) cells by targeting the miR-130a-3p-HMGB3 axis. The greatest advantage of the prognostic risk model in this study is that this is the first study to identify, via public databases and modeling, the HOTAIR-miR-130a-3p-HMGB3 axis as a prognosis-related biomarker. This axis may be a potential prognostic biomarker, providing a new research direction for the prognosis research of breast cancer. At the same time, the model in this study can also be used as a reference for screening other tumor prognostic biomarkers. Compared with many previous ceRNA studies, we conducted further studies in geographic data sets and TCGA pan-cancer cohort, and verified the up-regulation of HOTAIR and HMGB3 and the down-regulation of miR-130a-3p. In addition, we hypothesized that hypomethylation might up-regulate the expression of HOTAIR and HMGB3.
Unavoidably, some limitations are present in our work. The biggest problem is the lack of experimental verification in vivo and in vitro. Furthermore, the molecular mechanism underlying the upregulated expression of HOTAIR and HMGB3 was not fully accessed. In the subsequent experiment, we should further explore and verify the expression of selected prognostic markers in cancer and its predictive role on survival and prognosis of patients by combining with immunohistochemistry, Western blot, cell function and other tests. To validate the findings of our study, we propose to use both in vitro and in vivo models. In vitro, we plan to use cell culture systems, including both gain-of-function and loss-of-function experiments, to investigate the effect of HOTAIR-miR-130a-3p-HMGB3 axis on cell proliferation, migration, invasion, and apoptosis. Specifically, we will use techniques such as transfection of siRNA, plasmid DNA, and lentivirus vectors to knockdown or overexpress HOTAIR, miR-130a-3p, and HMGB3 in cell lines. We will then assess the phenotypic changes by performing assays such as cell viability, colony formation, wound healing, transwell invasion, and flow cytometry. In vivo, we plan to use established human cancer cell lines that represent the type of cancer we studied in our research. We will knockdown or overexpress the genes of interest in these cells and then inject them into immunodeficient mice. We will monitor tumor growth over time, measure tumor volume, and perform histological and immunohistochemical analyses to assess the effects of the HOTAIR-miR-130a-3p-HMGB3 axis on tumorigenesis. This may provide candidate targets for the treatment of breast cancer patients, thereby reducing the mortality rate and improving the prognosis of patients.
Conclusions
A breast cancer-specific ceRNA regulatory network was built using bioinformatics analysis. Our research identified a prognostic risk model with the HOTAIR-miR-130a-3p-HMGB3 axis that may serve as a reliable prognostic biomarker for patients with breast cancer. Further experimental studies are needed to confirm the underlying biological regulatory mechanism in the future.
Acknowledgments
Funding: This work was supported by the
Footnote
Reporting Checklist: The authors have completed the TRIPOD reporting checklist. Available at https://tcr.amegroups.com/article/view/10.21037/tcr-23-313/rc
Peer Review File: Available at https://tcr.amegroups.com/article/view/10.21037/tcr-23-313/prf
Conflicts of Interest: All authors have completed the ICMJE uniform disclosure form (available at https://tcr.amegroups.com/article/view/10.21037/tcr-23-313/coif). The authors have no conflicts of interest to declare.
Ethical Statement: The authors are accountable for all aspects of the work in ensuring that questions related to the accuracy or integrity of any part of the work are appropriately investigated and resolved. The study was conducted in accordance with the Declaration of Helsinki (as revised in 2013).
Open Access Statement: This is an Open Access article distributed in accordance with the Creative Commons Attribution-NonCommercial-NoDerivs 4.0 International License (CC BY-NC-ND 4.0), which permits the non-commercial replication and distribution of the article with the strict proviso that no changes or edits are made and the original work is properly cited (including links to both the formal publication through the relevant DOI and the license). See: https://creativecommons.org/licenses/by-nc-nd/4.0/.
References
- Giaquinto AN, Sung H, Miller KD, et al. Breast Cancer Statistics, 2022. CA Cancer J Clin 2022;72:524-41. [Crossref] [PubMed]
- Sung H, Ferlay J, Siegel RL, et al. Global Cancer Statistics 2020: GLOBOCAN Estimates of Incidence and Mortality Worldwide for 36 Cancers in 185 Countries. CA Cancer J Clin 2021;71:209-49. [Crossref] [PubMed]
- Lee K, Kruper L, Dieli-Conwright CM, et al. The Impact of Obesity on Breast Cancer Diagnosis and Treatment. Curr Oncol Rep 2019;21:41. [Crossref] [PubMed]
- Wilusz JE, Sunwoo H, Spector DL. Long noncoding RNAs: functional surprises from the RNA world. Genes Dev 2009;23:1494-504. [Crossref] [PubMed]
- Mercer TR, Dinger ME, Mattick JS. Long non-coding RNAs: insights into functions. Nat Rev Genet 2009;10:155-9. [Crossref] [PubMed]
- Ulitsky I, Bartel DP. lincRNAs: genomics, evolution, and mechanisms. Cell 2013;154:26-46. [Crossref] [PubMed]
- Shi X, Sun M, Liu H, et al. Long non-coding RNAs: a new frontier in the study of human diseases. Cancer Lett 2013;339:159-66. [Crossref] [PubMed]
- Huarte M. LncRNAs have a say in protein translation. Cell Res 2013;23:449-51. [Crossref] [PubMed]
- Ye J, Zhu J, Chen H, et al. A novel lncRNA-LINC01116 regulates tumorigenesis of glioma by targeting VEGFA. Int J Cancer 2020;146:248-61. [Crossref] [PubMed]
- Pan J, Fang S, Tian H, et al. lncRNA JPX/miR-33a-5p/Twist1 axis regulates tumorigenesis and metastasis of lung cancer by activating Wnt/β-catenin signaling. Mol Cancer 2020;19:9. [Crossref] [PubMed]
- Shi L, Hong X, Ba L, et al. Long non-coding RNA ZNFX1-AS1 promotes the tumor progression and metastasis of colorectal cancer by acting as a competing endogenous RNA of miR-144 to regulate EZH2 expression. Cell Death Dis 2019;10:150. [Crossref] [PubMed]
- López-Urrutia E, Bustamante Montes LP, Ladrón de Guevara Cervantes D, et al. Crosstalk Between Long Non-coding RNAs, Micro-RNAs and mRNAs: Deciphering Molecular Mechanisms of Master Regulators in Cancer. Front Oncol 2019;9:669. [Crossref] [PubMed]
- Salmena L, Poliseno L, Tay Y, et al. A ceRNA hypothesis: the Rosetta Stone of a hidden RNA language? Cell 2011;146:353-8. [Crossref] [PubMed]
- Wang YG, Wang T, Ding M, et al. hsa_circ_0091570 acts as a ceRNA to suppress hepatocellular cancer progression by sponging hsa-miR-1307. Cancer Lett 2019;460:128-38. [Crossref] [PubMed]
- Zhao Y, Du T, Du L, et al. Long noncoding RNA LINC02418 regulates MELK expression by acting as a ceRNA and may serve as a diagnostic marker for colorectal cancer. Cell Death Dis 2019;10:568. [Crossref] [PubMed]
- Chen X, Wang Z, Tong F, et al. lncRNA UCA1 Promotes Gefitinib Resistance as a ceRNA to Target FOSL2 by Sponging miR-143 in Non-small Cell Lung Cancer. Mol Ther Nucleic Acids 2020;19:643-53. [Crossref] [PubMed]
- Han Q, Li J, Xiong J, et al. Long noncoding RNA LINC00514 accelerates pancreatic cancer progression by acting as a ceRNA of miR-28-5p to upregulate Rap1b expression. J Exp Clin Cancer Res 2020;39:151. [Crossref] [PubMed]
- Kong X, Duan Y, Sang Y, et al. LncRNA-CDC6 promotes breast cancer progression and function as ceRNA to target CDC6 by sponging microRNA-215. J Cell Physiol 2019;234:9105-17. [Crossref] [PubMed]
- Langfelder P, Horvath S. WGCNA: an R package for weighted correlation network analysis. BMC Bioinformatics 2008;9:559. [Crossref] [PubMed]
- Xu BF, Liu R, Huang CX, et al. Identification of key genes in ruptured atherosclerotic plaques by weighted gene correlation network analysis. Sci Rep 2020;10:10847. [Crossref] [PubMed]
- Zhang Z, Liu R, Jin R, et al. Integrating Clinical and Genetic Analysis of Perineural Invasion in Head and Neck Squamous Cell Carcinoma. Front Oncol 2019;9:434. [Crossref] [PubMed]
- Niemira M, Collin F, Szalkowska A, et al. Molecular Signature of Subtypes of Non-Small-Cell Lung Cancer by Large-Scale Transcriptional Profiling: Identification of Key Modules and Genes by Weighted Gene Co-Expression Network Analysis (WGCNA). Cancers (Basel) 2019;12:37. [Crossref] [PubMed]
- Xu W, Hua L, Xia H. Integrative analysis of mRNA, miRNA and lncRNA profiles reveals the commonness between bladder cancer and breast cancer. Transl Cancer Res 2020;9:1070-90. [Crossref] [PubMed]
- Li Y, Zu X, Hu X, et al. Competing endogenous RNA network analysis reveals pivotal ceRNAs in bladder urothelial carcinoma. Transl Androl Urol 2021;10:797-808. [Crossref] [PubMed]
- Yao Y, Zhang T, Qi L, et al. Integrated analysis of co-expression and ceRNA network identifies five lncRNAs as prognostic markers for breast cancer. J Cell Mol Med 2019;23:8410-9. [Crossref] [PubMed]
- Kim SY, Kawaguchi T, Yan L, et al. Clinical Relevance of microRNA Expressions in Breast Cancer Validated Using the Cancer Genome Atlas (TCGA). Ann Surg Oncol 2017;24:2943-9. [Crossref] [PubMed]
- Carithers LJ, Ardlie K, Barcus M, et al. A Novel Approach to High-Quality Postmortem Tissue Procurement: The GTEx Project. Biopreserv Biobank 2015;13:311-9. [Crossref] [PubMed]
- Tang Z, Li C, Kang B, et al. GEPIA: a web server for cancer and normal gene expression profiling and interactive analyses. Nucleic Acids Res 2017;45:W98-W102. [Crossref] [PubMed]
- Li B, Dewey CN. RSEM: accurate transcript quantification from RNA-Seq data with or without a reference genome. BMC Bioinformatics 2011;12:323. [Crossref] [PubMed]
- Harrow J, Frankish A, Gonzalez JM, et al. GENCODE: the reference human genome annotation for The ENCODE Project. Genome Res 2012;22:1760-74. [Crossref] [PubMed]
- Law CW, Chen Y, Shi W, et al. voom: Precision weights unlock linear model analysis tools for RNA-seq read counts. Genome Biol 2014;15:R29. [Crossref] [PubMed]
- Robinson MD, McCarthy DJ, Smyth GK. edgeR: a Bioconductor package for differential expression analysis of digital gene expression data. Bioinformatics 2010;26:139-40. [Crossref] [PubMed]
- Love MI, Huber W, Anders S. Moderated estimation of fold change and dispersion for RNA-seq data with DESeq2. Genome Biol 2014;15:550. [Crossref] [PubMed]
- Yu G, Wang LG, Han Y, et al. clusterProfiler: an R package for comparing biological themes among gene clusters. OMICS 2012;16:284-7. [Crossref] [PubMed]
- Hsu SD, Lin FM, Wu WY, et al. miRTarBase: a database curates experimentally validated microRNA-target interactions. Nucleic Acids Res 2011;39:D163-9. [Crossref] [PubMed]
- Agarwal V, Bell GW, Nam JW, et al. Predicting effective microRNA target sites in mammalian mRNAs. Elife 2015;4:e05005. [Crossref] [PubMed]
- Wang X. miRDB: a microRNA target prediction and functional annotation database with a wiki interface. RNA 2008;14:1012-7. [Crossref] [PubMed]
- Ru Y, Kechris KJ, Tabakoff B, et al. The multiMiR R package and database: integration of microRNA-target interactions along with their disease and drug associations. Nucleic Acids Res 2014;42:e133. [Crossref] [PubMed]
- Li JH, Liu S, Zhou H, et al. starBase v2.0: decoding miRNA-ceRNA, miRNA-ncRNA and protein-RNA interaction networks from large-scale CLIP-Seq data. Nucleic Acids Res 2014;42:D92-7. [Crossref] [PubMed]
- Shannon P, Markiel A, Ozier O, et al. Cytoscape: a software environment for integrated models of biomolecular interaction networks. Genome Res 2003;13:2498-504. [Crossref] [PubMed]
- Lin T, Fu Y, Zhang X, et al. A seven-long noncoding RNA signature predicts overall survival for patients with early stage non-small cell lung cancer. Aging (Albany NY) 2018;10:2356-66. [Crossref] [PubMed]
- Mayakonda A, Lin DC, Assenov Y, et al. Maftools: efficient and comprehensive analysis of somatic variants in cancer. Genome Res 2018;28:1747-56. [Crossref] [PubMed]
- Barrett T, Wilhite SE, Ledoux P, et al. NCBI GEO: archive for functional genomics data sets--update. Nucleic Acids Res 2013;41:D991-5. [Crossref] [PubMed]
- Eades G, Wolfson B, Zhang Y, et al. lincRNA-RoR and miR-145 regulate invasion in triple-negative breast cancer via targeting ARF6. Mol Cancer Res 2015;13:330-8. [Crossref] [PubMed]
- Liu Y, Du Y, Hu X, et al. Up-regulation of ceRNA TINCR by SP1 contributes to tumorigenesis in breast cancer. BMC Cancer 2018;18:367. [Crossref] [PubMed]
- Li RK, Gao J, Guo LH, et al. PTENP1 acts as a ceRNA to regulate PTEN by sponging miR-19b and explores the biological role of PTENP1 in breast cancer. Cancer Gene Ther 2017;24:309-15. [Crossref] [PubMed]
- Chou J, Wang B, Zheng T, et al. MALAT1 induced migration and invasion of human breast cancer cells by competitively binding miR-1 with cdc42. Biochem Biophys Res Commun 2016;472:262-9. [Crossref] [PubMed]
- Peng F, Li TT, Wang KL, et al. H19/let-7/LIN28 reciprocal negative regulatory circuit promotes breast cancer stem cell maintenance. Cell Death Dis 2017;8:e2569. [Crossref] [PubMed]
- Moore LD, Le T, Fan G. DNA methylation and its basic function. Neuropsychopharmacology 2013;38:23-38. [Crossref] [PubMed]
- Gupta RA, Shah N, Wang KC, et al. Long non-coding RNA HOTAIR reprograms chromatin state to promote cancer metastasis. Nature 2010;464:1071-6. [Crossref] [PubMed]
- Gupta S, Iljin K, Sara H, et al. FZD4 as a mediator of ERG oncogene-induced WNT signaling and epithelial-to-mesenchymal transition in human prostate cancer cells. Cancer Res 2010;70:6735-45. [Crossref] [PubMed]
- Chisholm KM, Wan Y, Li R, et al. Detection of long non-coding RNA in archival tissue: correlation with polycomb protein expression in primary and metastatic breast carcinoma. PLoS One 2012;7:e47998. [Crossref] [PubMed]
- Sørensen KP, Thomassen M, Tan Q, et al. Long non-coding RNA HOTAIR is an independent prognostic marker of metastasis in estrogen receptor-positive primary breast cancer. Breast Cancer Res Treat 2013;142:529-36. [Crossref] [PubMed]
- Xue X, Yang YA, Zhang A, et al. LncRNA HOTAIR enhances ER signaling and confers tamoxifen resistance in breast cancer. Oncogene 2016;35:2746-55. [Crossref] [PubMed]
- Ding W, Ren J, Ren H, et al. Long Noncoding RNA HOTAIR Modulates MiR-206-mediated Bcl-w Signaling to Facilitate Cell Proliferation in Breast Cancer. Sci Rep 2017;7:17261. [Crossref] [PubMed]
- Sorrentino A, Liu CG, Addario A, et al. Role of microRNAs in drug-resistant ovarian cancer cells. Gynecol Oncol 2008;111:478-86. [Crossref] [PubMed]
- Acunzo M, Visone R, Romano G, et al. miR-130a targets MET and induces TRAIL-sensitivity in NSCLC by downregulating miR-221 and 222. Oncogene 2012;31:634-42. [Crossref] [PubMed]
- Eichelmann AK, Matuszcak C, Lindner K, et al. Complex role of miR-130a-3p and miR-148a-3p balance on drug resistance and tumor biology in esophageal squamous cell carcinoma. Sci Rep 2018;8:17553. [Crossref] [PubMed]
- Wang S, Han H, Hu Y, et al. MicroRNA-130a-3p suppresses cell migration and invasion by inhibition of TBL1XR1-mediated EMT in human gastric carcinoma. Mol Carcinog 2018;57:383-92. [Crossref] [PubMed]
- Kong X, Zhang J, Li J, et al. MiR-130a-3p inhibits migration and invasion by regulating RAB5B in human breast cancer stem cell-like cells. Biochem Biophys Res Commun 2018;501:486-93. [Crossref] [PubMed]
- Nemeth MJ, Curtis DJ, Kirby MR, et al. Hmgb3: an HMG-box family member expressed in primitive hematopoietic cells that inhibits myeloid and B-cell differentiation. Blood 2003;102:1298-306. [Crossref] [PubMed]
- Gong Y, Cao Y, Song L, et al. HMGB3 characterization in gastric cancer. Genet Mol Res 2013;12:6032-9. [Crossref] [PubMed]
- Yamada Y, Nishikawa R, Kato M, et al. Regulation of HMGB3 by antitumor miR-205-5p inhibits cancer cell aggressiveness and is involved in prostate cancer pathogenesis. J Hum Genet 2018;63:195-205. [Crossref] [PubMed]
- Song N, Liu B, Wu JL, et al. Prognostic value of HMGB3 expression in patients with non-small cell lung cancer. Tumour Biol 2013;34:2599-603. [Crossref] [PubMed]
- Zheng WJ, Yao M, Fang M, et al. Abnormal expression of HMGB-3 is significantly associated with malignant transformation of hepatocytes. World J Gastroenterol 2018;24:3650-62. [Crossref] [PubMed]
- Gu J, Xu T, Huang QH, et al. HMGB3 silence inhibits breast cancer cell proliferation and tumor growth by interacting with hypoxia-inducible factor 1α. Cancer Manag Res 2019;11:5075-89. [Crossref] [PubMed]
- Li X, Wu Y, Liu A, et al. MiR-27b is epigenetically downregulated in tamoxifen resistant breast cancer cells due to promoter methylation and regulates tamoxifen sensitivity by targeting HMGB3. Biochem Biophys Res Commun 2016;477:768-73. [Crossref] [PubMed]
- Hu M, Fu Q, Jing C, et al. LncRNA HOTAIR knockdown inhibits glycolysis by regulating miR-130a-3p/HIF1A in hepatocellular carcinoma under hypoxia. Biomed Pharmacother 2020;125:109703. [Crossref] [PubMed]
- He W, Li D, Zhang X. LncRNA HOTAIR promotes the proliferation and invasion/metastasis of breast cancer cells by targeting the miR-130a-3p/Suv39H1 axis. Biochem Biophys Rep 2022;30:101279. [Crossref] [PubMed]