Correlation of gene expression profiles to identify pancreatic cancer cell lines that best model primary human tumors
Highlight box
Key findings
• The gene expression profiles of PAAD cell lines correlate weakly with primary pancreatic tumors, we provide a new strategy for choosing the appropriate cell lines.
What is known and what is new?
• Empirical selection of cancer cell lines may not be adequate for in vitro cancer studies, especially for pancreatic cancer.
• We compared the genetic similarity between PAAD cell lines and human tissue to choose the most appropriate cell lines.
What is the implication, and what should change now?
• Not all PAAD cell lines behave like human tumor tissue in in vitro cancer research. We could choose a more optimal PAAD cell line based on the genetic similarity between them.
Introduction
Cancer cell lines have had an increasingly important role in the study of cancer biology, and they are an invaluable model system for in vitro cancer research (1-5). A vast number of different cancer cell lines have been developed, yet only a few can be used in any given study due to financial and experimental constraints. As little information is available on how well the behavior of a particular cell line matches that of the primary tumor, most investigators choose a cell line based on empirical evidence or simply choose the most commonly used cell line (6-8). Unfortunately, empirical methods may not be adequate. For example, the commonly-used ovarian cell line SKOV3 has less genomic fidelity to patient tumors than the 2 less-described cell lines KURAMOCHI and OVSAHO (9). In two commonly used cell lines BXPC-3 and PANC-1, genes involved in epithelial-mesenchymal transition (EMT) and carbohydrate metabolism are quite different (10,11). Therefore, understanding and quantifying the genetic similarity between cell lines and patient tumors, and choosing the most appropriate cell lines is of critical importance for in vitro cancer studies.
Several recent studies have compared the messenger RNA (mRNA) expression profiles of cell lines and primary tumors of various cancers (12-16); the genetic similarity between cell lines and primary tissues was found to be tumor dependent. Cell lines with moderately similar gene expression to primary tumors were reported to have a median correlation coefficient of 0.6 in the Cancer Cell Line Encyclopedia (CCLE) project (15). However, pancreatic adenocarcinoma (PAAD) was found to exhibit a weaker correlation between cell lines and primary tumors, with a correlation coefficient of only 0.347 (15). At the same time, Deer et al. compared phenotype and genotype of 11 PAAD cell lines, revealing that sufficient discrepancies exist in them (17). As an extremely poor correlation between PAAD primary tumors and their cell lines was found, the empirical model of cell lines selection, and even the least accurate 1 cell line fitted all study, may be challenged. For example, if the role of KRAS mutation in pancreatic tumor is studied, then it is not reasonable to choose BxPC-3, KP-4 and Panc 10.05 cell lines; if gemcitabine-resistance pancreatic tumor is studied, the gemcitabine-sensitive cell lines BxPC-3, CFPAC and SU86.86 are not suitable (18). A more reasonable method would be to warrant scrutiny during cell line selection, to compare the gene expression profiles of a number of different cell lines to that of the patient tissues, with a focus on the most relevant pathways, in order to select the one that best matches the in vivo situation.
The aforementioned studies conducted by Domcke and Shuaichen had a leading find of genetic similarity between cancer cell lines and patient tumor tissue (12,14). However, more detailed information, such as correlation of individual cancer-related pathways or cancer-related functions, should be provided to suggest appropriate cell lines for in vitro study of the corresponding cancer. Therefore, in the present study, we conducted a comprehensive analysis of the similarity of cancer-related pathways covering 33 commonly used human PAAD cell lines and 892 patient samples. We found that the gene expression profiles of PAAD cell lines correlate weakly with those of primary pancreatic tumors. Up to 50% cancer-related pathways are not strongly recommended in PAAD cell lines, and a small proportion of cancer-related functions (12–17%) are extremely poorly correlated with PAAD cell lines. Based on the similarity of genetic profiles and bioinformatics analyses, we provide a strategy for choosing the appropriate PAAD cell line. We present the following article in accordance with the MDAR reporting checklist (available at https://tcr.amegroups.com/article/view/10.21037/tcr-23-173/rc).
Methods
Cell lines
The human PAAD cell lines are available at following cell line collections:
- American Type Culture Collection (ATCC; Manassas, VA, USA; http://www.atcc.org/);
- Leibniz Institute (DSMZ; Braunschweig, Germany; http://www.dsmz.de/);
- European Collection of Authenticated Cell Cultures (ECACC; Salisbury, UK; http://www.hpacultures.org.uk/collections/ecacc.jsp);
- Health Sciences Research Resources Bank (HSRRB; Tokyo, Japan; http://www.jhsf.or.jp/English/hsrrb.html);
- RIKEN of Japan (Tokyo, Japan; http://www.brc.riken.jp/lab/cell/english/);
- Interlab Cell Line Collection (ICLC; Genoa, Italy; http://www.iclc.it/Listanuova.html);
- Korean Cell Line Bank (KCLB; Seoul, Korea; http://cellbank.snu.ac.kr/english/index.php);
- China Infrastructure of Cell Line Resource (CICR; Beijing, China; http://www.cellresource.cn/).
Private cell lines were excluded. The characteristics of cell lines were checked in database of Cellosaurus (https://web.expasy.org/cellosaurus). Further details are shown in Table S1.
mRNA expression data
Microarray data
Data on mRNA expression in PAAD cell lines and patient tumor tissues were obtained from the Gene Expression Omnibus (GEO; http://www.ncbi.nlm.nih.gov/geo/). GEO datasets uploaded to the database on or before 30 June 2017 were refined using the following search terms: (I) cancer: pancreatic carcinoma, pancreatic cancer, or pancreatic tumor; OR (II) cell lines: AsPC-1, BxPC-3, PANC-1, Capan-1, Capan-2, CFPAC-1, DAN-G, Hs 766T, HuP-T3, HuP-T4, KP-2, KP-3,KP-4, MIA PaCa-2, PK-45H, PK-59, PSN1, SNU-213, SNU-324, SU.86.86, SNU-410, SUIT-2, SW1990, T3M-4, YAPC, HPAC, Panc 02.13, Panc 03.27, Panc 04.03, Panc 05.04, Panc 08.13, Panc 10.05, and PK-1. The datasets were independently inspected and included. The study type was limited to “Expression profiling by array”, and species was defined as “Homo sapiens”. All datasets were independently inspected by two review authors (Gaoqi Xu and Jiao Sun), who checked the data for tumor tissues from therapy-naive patients or for untreated cell lines. Further details are shown in Figure S1. The study was conducted in accordance with the Declaration of Helsinki (as revised in 2013).
Preprocessing of microarray data
Affymetrix microarray datasets were downloaded as raw. CEL files and preprocessed using the robust multi-array average (RMA) algorithm in the Bioconductor package “affy”. Datasets from other microarray platforms were downloaded as a series matrix file with normalized data.
If a gene was detected with multiple probes in an array, the expression level was taken as the average value of all probes. Datasets from the same cell lines or from PAAD patients were pooled. Batch effect correction was performed using the function ComBat from the Bioconductor package sva to control for batch effects between different microarray datasets.
Cell line to patient tumor comparison
The similarity of cell lines and patient tumors was evaluated by compared the pooled data of each cell line with PAAD patients’ tumors based on the top 2,000 gene with largest interquartile range (IQR) or cancer-related pathways.
Similarity of general genes
The correlation based on general gene-profile was evaluated according to a previously reported algorithm. The average fold-changes for each gene ranked among the top 2,000 IQR genes between cell lines and tumor samples of PAAD patients were calculated by a previously fitting linear model for microarray data (limma) (15).
Similarity of specific pathways
Text mining search of cancer-related genes
An online text mining search engine, DiGseE (developed and available by Data Mining & Computational Biology Laboratory, Gwangju Institute of Science and Technology, Gwangju, South Korea, at http://gcancer.org/digsee), was used to collect the genes related to cancer from Medline abstracts for evidence sentences describing in literature (19).
Cancer-related pathways enriched via Kyoto Encyclopedia of Genes and Genomes (KEGG) pathway analysis
All the reported cancer-related genes were analyzed via the Database for Annotation, Visualization, and Integrated Discovery (DAVID) Bioinformatics Resources 6.8 (available at https://david.ncifcrf.gov). The cancer-related pathways and each pathway-involved gene were obtained. A P value <0.10 was considered to have statistical significance and to achieve significant enrichment.
Similarity evaluation of cancer-related pathways
The correlation of each pathway between an individual cell line and PAAD patient tumors was calculated by comparing normalized-expression levels of genes involved in each pathway using pairwise Pearson’s correlation analysis according to the algorithm previously described (10), respectively. The pathway-similarity profiles of cell lines to human tumors were compared based on correlation coefficients of all 134 pathways using Mann-Whitney U-test. The pathways were grouped into highly (r>0.60), moderately (r=0.30–0.60), and poorly (r<0.30) consistent pathways of each cell line, whereas the highly, moderately, and poorly consistent cell lines of each pathway were also collected.
Similarity evaluation of cancer-related functions
The correlation of each function between an individual cell line and PAAD patient tumors was calculated by comparing normalized-expression levels of genes involved in each function using pairwise Pearson’s correlation analysis according to the algorithm previously described, respectively. The function-similarity profiles of cell lines to human tumors were compared based on correlation coefficients of all 504 functions using the Mann-Whitney U-test. The functions were grouped into highly (r>0.60), moderately (r=0.30–0.60), and poorly (r<0.30) consistent functions of each cell line, and the highly, moderately, and poorly consistent cell lines of each function were also collected.
Results
A total of 479 of potentially related datasets series was found in GEO, of which 86, 48, 226, and 12 datasets were excluded for treated cell line, duplicate data-series, unavailable detail information, and too many missed data, respectively. Ultimately, 107 data series were included, among which there were 33 cell lines (AsPC-1, BxPC-3, PANC-1, Capan-1, Capan-2, CFPAC-1, DAN-G, Hs 766T, HuP-T3, HuP-T4, KP-2, KP-3, KP-4, MIA PaCa-2, PK-45H, PK-59, PSN1, SNU-213, SNU-324, SU.86.86, SNU-410, SUIT-2, SW1990, T3M-4, YAPC, HPAC, Panc 02.13, Panc 03.27, Panc 04.03, Panc 05.04, Panc 08.13, Panc 10.05, and PK-1), and 1,358 samples, respectively, as listed in Tables S1,S2 and Figure S1.
Cell line to primary tumor comparison
As is shown in Figure 1 and Table S1, high genetic divergence was observed between each PAAD cell line; and the correlation between PAAD cell lines and tumor tissue were extremely poor (correlation coefficient: median, 0.137; range, −0.416 to 0.511).
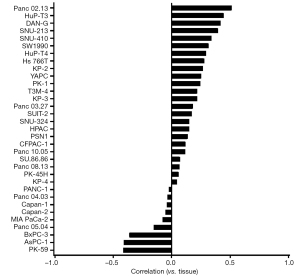
The correlation coefficients of cancer-related pathways in various pancreatic cancer cell lines are displayed in Figure 2. Several key cancer-related pathways showed poor consistency between most PAAD cell lines and patient tissues, including Toll-like receptor, PI3K-Akt, NF kappa B, cAMP, p53, focal adhesion, HIF-1, Wnt, Foxo, and so on. Moreover, according to the Cellosaurus database, PAAD cell lines were divided into two groups: cell lines of primary lesion and metastatic lesion. The top 3 poor consistency PAAD cell lines with tumor tissue were all cell lines of metastatic lesion in several cancer-related pathways, such as endometrial cancer, renal cell carcinoma, and small cell lung cancer.
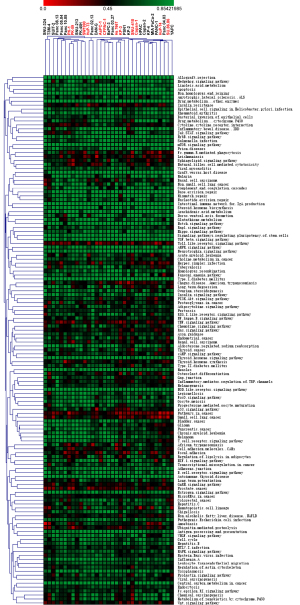
Although they all belonged to the same group, the genomic similarity between cell lines and tumor tissue varied greatly in the same pathway. For example, SW1990, Hup-T3, CFPAC-1, Hs766T, Capan-1, and ASPC-1 are cell lines of metastatic lesions, among which, ASPC-1 cells were poorly correlated with the HIF-1 pathway, whereas the others were highly correlated with the HIF-1 pathway. Similarly, DAN-G and Panc 10.05 were PAAD cell lines of primary lesions, the consistent value of DAN-G in the p53 pathway was 0.12, whereas that of Panc 10.05 in the p53 pathway was 0.83. In addition, there were pathways, such as mTOR, the NOD like receptor signaling pathway, and Allograft rejection, upon which the chosen of PAAD cell line had little effect. Based on these observations, we recommended the most suitable cancer-related pathways for each PAAD cell line (Table 1 and Table S3). For pan-pathway analysis, the cell line showing the highest genetic correlation to patient tumors was Panc 03.27 for PAAD cell lines from primary lesion sites, and CFPAC-1 for PAAD cell lines from metastatic lesion sites.
Table 1
PAAD cell lines | Highly correlated pathways (top 3) |
---|---|
Panc 02.13 | DNA ligation involved in DNA repair, negative regulation of acute inflammatory response, B cell proliferation |
HuP-T3 | Atrioventricular valve morphogenesis; positive regulation of cell adhesion mediated by integrin; microglial cell activation |
DAN-G | DNA ligation involved in DNA repair; B cell proliferation; liver regeneration |
HUP-T4 | Positive regulation of tyrosine phosphorylation of STAT1 protein; natural killer cell activation involved in immune response; mammary gland epithelial cell proliferation |
PK-59 | Cell migration in hindbrain; Blood coagulation intrinsic pathway; DNA dependent DNA replication maintenance of fidelity |
AsPC-1 | DNA ligation involved in DNA repair; cell migration in hindbrain; positive regulation of tyrosine phosphorylation of STAT1 protein |
SNU-213 | Positive regulation of tyrosine phosphorylation of STAT1 protein; DNA ligation involved in DNA repair; dendritic cell chemotaxis |
BxPC-3 | DNA ligation involved in DNA repair; negative regulation of chondrocyte differentiation; positive regulation of cell adhesion mediated by integrin |
SNU-410 | DNA ligation involved in DNA repair; negative regulation of osteoblast differentiation; atrioventricular valve morphogenesis |
SW1990 | Positive regulation of tyrosine phosphorylation of STAT1 protein; osteoblast development; atrioventricular valve morphogenesis |
HuP-T4 | Natural killer cell activation involved in immune response; positive regulation of tyrosine phosphorylation of STAT1 protein; blood coagulation intrinsic pathway |
Hs 766T | Blood coagulation intrinsic pathway; negative regulation of acute inflammatory response; negative regulation of osteoblast differentiation |
KP-2 | Cell migration in hindbrain; positive regulation of tyrosine phosphorylation of STAT1 protein; wound healing spreading of epidermal cells |
YAPC | Negative regulation of acute inflammatory response; atrioventricular valve morphogenesis; negative regulation of cell cycle arrest |
PK-1 | Mammary gland epithelial cell proliferation; negative regulation of chondrocyte differentiation; liver regeneration |
T3M-4 | Liver regeneration; DNA ligation involved in DNA repair; cellular response to fluid shear stress |
KP-3 | Cell migration in hindbrain; blood coagulation intrinsic pathway; positive regulation of tyrosine phosphorylation of STAT1 protein |
Panc 03.27 | Fibrinolysis; cell migration in hindbrain; DNA ligation involved in DNA repair |
SUIT-2 | DNA ligation involved in DNA repair; positive regulation of tyrosine phosphorylation of STAT1 protein; negative regulation of acute inflammatory response |
Panc 05.04 | Cell migration in hindbrain; wound healing spreading of epidermal cells; dendritic cell chemotaxis |
SNU-324 | Dendritic cell chemotaxis; cellular response to fluid shear stress; DNA ligation involved in DNA repair |
HPAC | DNA ligation involved in DNA repair; atrioventricular valve morphogenesis; bone mineralization |
PSN1 | Positive regulation of tyrosine phosphorylation of STAT1 protein; liver regeneration; negative regulation of chondrocyte differentiation |
CFPAC-1 | Blood coagulation intrinsic pathway; positive regulation of tyrosine phosphorylation of STAT1 protein; DNA ligation involved in DNA repair |
Panc 10.05 | Cell migration in hindbrain; regulation of cytokine secretion involved in immune response; atrioventricular valve morphogenesis |
MIA PaCa-2 | DNA ligation involved in DNA repair; positive regulation of tyrosine phosphorylation of STAT1 protein; Protein homotrimerization |
SU.86.86 | Positive regulation of tyrosine phosphorylation of STAT1 protein; DNA ligation involved in DNA repair; Blood coagulation intrinsic pathway |
Panc 08.13 | Natural killer cell activation involved in immune response; cell migration in hindbrain; positive regulation of tyrosine phosphorylation of STAT1 protein |
PK-45H | Protein homotrimerization; positive regulation of tyrosine phosphorylation of STAT1 protein; fibrinolysis |
Capan-2 | Dendritic cell chemotaxis; cellular response to fluid shear stress; osteoblast development |
KP-4 | Natural killer cell activation involved in immune response; DNA ligation involved in DNA repair; Positive regulation of tyrosine phosphorylation of STAT1 protein |
Capan-1 | Cell migration in hindbrain; blood coagulation intrinsic pathway; DNA ligation involved in DNA repair |
Panc 04.03 | DNA ligation involved in DNA repair; cellular response to fluid shear stress; cell migration in hindbrain |
PANC-1 | Positive regulation of tyrosine phosphorylation of STAT1 protein; cell migration in hindbrain; blood coagulation intrinsic pathway |
PAAD, pancreatic adenocarcinoma.
In Figure S2, although about 20% of the functions were not very different in each PAAD cell, more than 50% of the functions varied greatly between PAAD cell lines and tumor tissue, including Epithelial to mesenchymal transition, Negative regulation of fat cell differentiation, and Cell aging, among others. To investigate these functions, selection of propriate cell lines was quite necessary. So, we listed the top 3 suitable functions for each PAAD cell line (Table 2), to create a reference to minimize selection of an inappropriate cell line. In pan-function analysis, the cell line showing the highest genetic correlation to patient tumors was Panc 03.27 for PAAD cell lines from primary lesion sites and Capan-1 for PAAD cell lines from metastatic lesion sites.
Table 2
Cell lines | Highly correlated functions (top 3) |
---|---|
Panc 02.13 | DNA ligation involved in DNA repair, negative regulation of acute inflammatory response, B cell proliferation |
HuP-T3 | Atrioventricular valve morphogenesis; positive regulation of cell adhesion mediated by integrin; microglial cell activation |
DAN-G | DNA ligation involved in DNA repair; B cell proliferation; liver regeneration |
HUP-T4 | Positive regulation of tyrosine phosphorylation of STAT1 protein; natural killer cell activation involved in immune response; mammary gland epithelial cell proliferation |
PK-59 | Cell migration in hindbrain; blood coagulation intrinsic pathway; DNA dependent DNA replication maintenance of fidelity |
AsPC-1 | DNA ligation involved in DNA repair; cell migration in hindbrain; positive regulation of tyrosine phosphorylation of STAT1 protein |
SNU-213 | Positive regulation of tyrosine phosphorylation of STAT1 protein; DNA ligation involved in DNA repair; dendritic cell chemotaxis |
BxPC-3 | DNA ligation involved in DNA repair; negative regulation of chondrocyte differentiation; positive regulation of cell adhesion mediated by integrin |
HPDE | DNA ligation involved in DNA repair; positive regulation of cardiac muscle cell proliferation; positive regulation of tyrosine phosphorylation of STAT1 protein |
SNU-410 | DNA ligation involved in DNA repair; negative regulation of osteoblast differentiation; atrioventricular valve morphogenesis |
SW1990 | Positive regulation of tyrosine phosphorylation of STAT1 protein; osteoblast development; atrioventricular valve morphogenesis |
HuP-T4 | Natural killer cell activation involved in immune response; positive regulation of tyrosine phosphorylation of STAT1 protein; blood coagulation intrinsic pathway |
Hs 766T | Blood coagulation intrinsic pathway; negative regulation of acute inflammatory response; negative regulation of osteoblast differentiation |
KP-2 | Cell migration in hindbrain; positive regulation of tyrosine phosphorylation of STAT1 protein; wound healing spreading of epidermal cells |
YAPC | Negative regulation of acute inflammatory response; atrioventricular valve morphogenesis; negative regulation of cell cycle arrest |
PK-1 | Mammary gland epithelial cell proliferation; negative regulation of chondrocyte differentiation; liver regeneration |
T3M-4 | Liver regeneration; DNA ligation involved in DNA repair; cellular response to fluid shear stress |
KP-3 | Cell migration in hindbrain; blood coagulation intrinsic pathway; positive regulation of tyrosine phosphorylation of STAT1 protein |
Panc 03.27 | Fibrinolysis; cell migration in hindbrain; DNA ligation involved in DNA repair |
SUIT-2 | DNA ligation involved in DNA repair; positive regulation of tyrosine phosphorylation of STAT1 protein; negative regulation of acute inflammatory response |
Panc 05.04 | Cell migration in hindbrain; wound healing spreading of epidermal cells; dendritic cell chemotaxis |
SNU-324 | Dendritic cell chemotaxis; cellular response to fluid shear stress; DNA ligation involved in DNA repair |
HPAC | DNA ligation involved in DNA repair; atrioventricular valve morphogenesis; bone mineralization |
PSN1 | Positive regulation of tyrosine phosphorylation of STAT1 protein; liver regeneration; negative regulation of chondrocyte differentiation |
CFPAC-1 | Blood coagulation intrinsic pathway; positive regulation of tyrosine phosphorylation of STAT1 protein; DNA ligation involved in DNA repair |
Panc 10.05 | Cell migration in hindbrain; regulation of cytokine secretion involved in immune response; atrioventricular valve morphogenesis |
MIA PaCa-2 | DNA ligation involved in DNA repair; Positive regulation of tyrosine phosphorylation of STAT1 protein; protein homotrimerization |
SU.86.86 | Positive regulation of tyrosine phosphorylation of STAT1 protein; DNA ligation involved in DNA repair; blood coagulation intrinsic pathway |
Panc 08.13 | Natural killer cell activation involved in immune response; cell migration in hindbrain; positive regulation of tyrosine phosphorylation of STAT1 protein |
PK-45H | Protein homotrimerization; positive regulation of tyrosine phosphorylation of STAT1 protein; fibrinolysis |
Capan-2 | Dendritic cell chemotaxis; Cellular response to fluid shear stress; osteoblast development |
KP-4 | Natural killer cell activation involved in immune response; DNA ligation involved in DNA repair; positive regulation of tyrosine phosphorylation of STAT1 protein |
Capan-1 | Cell migration in hindbrain; blood coagulation intrinsic pathway; DNA ligation involved in DNA repair |
Panc 04.03 | DNA ligation involved in DNA repair; cellular response to fluid shear stress; cell migration in hindbrain |
PANC-1 | Positive regulation of tyrosine phosphorylation of STAT1 protein; cell migration in hindbrain; blood coagulation intrinsic pathway |
PAAD, pancreatic adenocarcinoma.
Discussion
After the first cancer cells, Hela, were established by Gey in 1951 (20), cancer cell lines have provided a relatively homogeneous model for in vitro cancer research. The first pancreatic cancer cell line was the CaPa strain established in 1963 (21). To date, more than 60 pancreatic cancer cell lines have been reported.
The use of immortalized cancer cell lines is an easy method for cancer research, not only due to the high degree of control over experimental variables, but also the role in high-throughput screening for anti-cancer drug discovery and research on the mechanisms of disease (22,23).
Although cancer cell lines provide a convenient tool for the study of cancer biology in vitro, there are still some limitations. First, cancer cell lines have originated from parts of primary tumors, and have been cultured in vitro for a long time, which renders them prone to genetic drift. Second, some cancer cell lines have been derived from primary lesions and others derived from metastatic lesions; how this difference will affect the results of the specific research is unknown. Third, due to significant differences in both genomic alterations and expression, the selected cancer lines may not be an accurate representation or model system of primary tumors. Fourthly, PAAD cell lines cannot mimic the real immune environment in primary pancreatic tumors because not only tumor cells but also stroma, fibroblast and various immune cells are present in tumors (24). Thus, it is urgent to establish an appropriate method to compare the gene expression profiles of numbers of different cell lines to those of tumor tissues, with a focus on the most relevant pathways or function, in order to select the cell line that best matches the in vivo situation.
Recent study reported huge heterogeneity between primary pancreatic tumors and metastatic disease: primary tumors contained 7 major cell populations while the metastatic lesions contained only 3 major cell populations; Tumor cells from primary lesions showed mesenchymal phenotype while the metastatic ones showed little mesenchymal characteristics (25). Thus in our study, PAAD cell lines are also divided into two groups according to the Cellosaurus database: primary lesion and metastatic lesion. We evaluated the similarities based on total gene profiles, the top 2,000 genes with largest IQRs, and significantly deferential genes in expression between human tumor samples and PAAD cell lines. The results indicated poor correlations between PAAD cell lines and primary tumor tissues.
In 134 cancer-related pathways which were identified based on KEGG analysis of gene enrichment in publications from Medline, 50% of cancer-related pathways were not recommended in neither cell lines of primary lesion nor cell lines of metastatic lesion, such as the Toll-like receptor pathway. In the pancreatic cancer pathway, all 33 cell lines were not poorly correlated with the tumor (r>0.3), and they could be chosen for studying the pathway of pancreatic cancer. In addition, among 504 cancer-related functions, up to about 15% of functions were poorly correlated (r<0.3) in all cell lines, including Regulation of circadian rhythm and Insulin receptor signaling pathway, among others. According to statistics, SW1990, Panc 08.13, and Panc 02.13 were the top3 PAAD cell lines that best matched to the overall cancer-related pathways, and Panc 03.27, Capan-1, and Panc 10.05 were the top3 PAAD cell lines that best matched to the overall cancer-related functions.
Correlation between PAAD cell lines and tumor tissue are varies greatly in some pathways, for example, African trypanosomiasis, Cytokine cytokine receptor interaction, Long term depression, Allograft rejection, Non homologous end joining and Type I diabetes mellitus pathways, there were no obvious selectivity tendencies among all PAAD cell lines. In Toll like receptor signaling pathway and pathways in cancer, all PAAD cell lines were poorly correlated with tumor tissue.
The same situation was observed in cancer-related functions between PAAD cell lines and tumor tissue. For example, PAAD cell lines were highly correlated in Positive regulation of tyrosine phosphorylation of stat1 protein, Response to zinc ion, and Response to fluid shear stress, among others, with tumor tissue, and were poorly correlated in Cellular response to insulin stimulus, Positive regulation of gene expression, and Regulation of circadian rhythm et al. with tumor tissue, and varied greatly in Smad protein signal transduction, Negative regulation of erk1 and erk2 cascade, and Positive regulation of DNA replication and so on.
Our research provides a new strategy for selecting suitable cell lines, but it also leaves a question unanswered. Can genetic correlation between cell lines and tumor tissues reflect the “real behavioral similarity” among them? It is yet to be verified that the selected PAAD cell line would reliably mimic the biologic behavior of pancreatic cancer.
Conclusions
The gene expression profiles of PAAD cell lines correlate weakly with those of primary pancreatic tumors. We have provided a strategy for choosing the appropriate PAAD cell line, and compared the genetic similarity between PAAD cell lines and human tumor tissue.
Acknowledgments
Funding: This research was funded by
Footnote
Reporting Checklist: The authors have completed the MDAR reporting checklist. Available at https://tcr.amegroups.com/article/view/10.21037/tcr-23-173/rc
Peer Review File: Available at https://tcr.amegroups.com/article/view/10.21037/tcr-23-173/prf
Conflicts of Interest: All authors have completed the ICMJE uniform disclosure form (available at https://tcr.amegroups.com/article/view/10.21037/tcr-23-173/coif). The authors have no conflicts of interest to declare.
Ethical Statement: The authors are accountable for all aspects of the work in ensuring that questions related to the accuracy or integrity of any part of the work are appropriately investigated and resolved. The study was conducted in accordance with the Declaration of Helsinki (as revised in 2013).
Open Access Statement: This is an Open Access article distributed in accordance with the Creative Commons Attribution-NonCommercial-NoDerivs 4.0 International License (CC BY-NC-ND 4.0), which permits the non-commercial replication and distribution of the article with the strict proviso that no changes or edits are made and the original work is properly cited (including links to both the formal publication through the relevant DOI and the license). See: https://creativecommons.org/licenses/by-nc-nd/4.0/.
References
- Bytautaite M, Petrikaite V. Comparative Study of Lipophilic Statin Activity in 2D and 3D in vitro Models of Human Breast Cancer Cell Lines MDA-MB-231 and MCF-7. Onco Targets Ther 2020;13:13201-9. [Crossref] [PubMed]
- Ellem SJ, De-Juan-Pardo EM, Risbridger GP. In vitro modeling of the prostate cancer microenvironment. Adv Drug Deliv Rev 2014;79-80:214-21. [Crossref] [PubMed]
- Kuenzi BM, Park J, Fong SH, et al. Predicting Drug Response and Synergy Using a Deep Learning Model of Human Cancer Cells. Cancer Cell 2020;38:672-684.e6. [Crossref] [PubMed]
- Monks A, Scudiero D, Skehan P, et al. Feasibility of a high-flux anticancer drug screen using a diverse panel of cultured human tumor cell lines. J Natl Cancer Inst 1991;83:757-66. [Crossref] [PubMed]
- Scatozza F, Moschella F, D'Arcangelo D, et al. Nicotinamide inhibits melanoma in vitro and in vivo. J Exp Clin Cancer Res 2020;39:211. [Crossref] [PubMed]
- Kim JY, Choi YJ, Kim HJ. Determining the effect of ellagic acid on the proliferation and migration of pancreatic cancer cell lines. Transl Cancer Res 2021;10:424-33. [Crossref] [PubMed]
- Zhou C, Qian W, Li J, et al. High glucose microenvironment accelerates tumor growth via SREBP1-autophagy axis in pancreatic cancer. J Exp Clin Cancer Res 2019;38:302. [Crossref] [PubMed]
- Braun LM, Lagies S, Guenzle J, et al. Metabolic Adaptation during nab-Paclitaxel Resistance in Pancreatic Cancer Cell Lines. Cells 2020;9:1251. [Crossref] [PubMed]
- Elias KM, Emori MM, Papp E, et al. Beyond genomics: critical evaluation of cell line utility for ovarian cancer research. Gynecol Oncol 2015;139:97-103. [Crossref] [PubMed]
- Sinha A, Cherba D, Bartlam H, et al. Mesenchymal-like pancreatic cancer cells harbor specific genomic alterations more frequently than their epithelial-like counterparts. Mol Oncol 2014;8:1253-65. [Crossref] [PubMed]
- Tataranni T, Agriesti F, Ruggieri V, et al. Rewiring carbohydrate catabolism differentially affects survival of pancreatic cancer cell lines with diverse metabolic profiles. Oncotarget 2017;8:41265-81. [Crossref] [PubMed]
- Shuaichen L, Guangyi W. Relating pancreatic ductal adenocarcinoma tumor samples and cell lines using gene expression data in translational research. Cell Mol Biol (Noisy-le-grand) 2018;64:157-62. [Crossref] [PubMed]
- Jiang G, Zhang S, Yazdanparast A, et al. Comprehensive comparison of molecular portraits between cell lines and tumors in breast cancer. BMC Genomics 2016;17:525. [Crossref] [PubMed]
- Domcke S, Sinha R, Levine DA, et al. Evaluating cell lines as tumour models by comparison of genomic profiles. Nat Commun 2013;4:2126. [Crossref] [PubMed]
- Barretina J, Caponigro G, Stransky N, et al. The Cancer Cell Line Encyclopedia enables predictive modelling of anticancer drug sensitivity. Nature 2012;483:603-7. [Crossref] [PubMed]
- Emdadi A, Eslahchi C. Clinical drug response prediction from preclinical cancer cell lines by logistic matrix factorization approach. J Bioinform Comput Biol 2022;20:2150035. [Crossref] [PubMed]
- Deer EL, González-Hernández J, Coursen JD, et al. Phenotype and genotype of pancreatic cancer cell lines. Pancreas 2010;39:425-35. [Crossref] [PubMed]
- Arumugam T, Ramachandran V, Fournier KF, et al. Epithelial to mesenchymal transition contributes to drug resistance in pancreatic cancer. Cancer Res 2009;69:5820-8. [Crossref] [PubMed]
- Kim J, So S, Lee HJ, et al. DigSee: Disease gene search engine with evidence sentences (version cancer). Nucleic Acids Res 2013;41:W510-7. [Crossref] [PubMed]
- Scherer WF, Syverton JT, Gey GO. Studies on the propagation in vitro of poliomyelitis viruses. IV. Viral multiplication in a stable strain of human malignant epithelial cells (strain HeLa) derived from an epidermoid carcinoma of the cervix. J Exp Med 1953;97:695-710. [Crossref] [PubMed]
- Dobrynin YV. Establishment and characteristics of cell strains from some epithelial tumors of human origin. J Natl Cancer Inst 1963;31:1173-95. [PubMed]
- Qiu Z, Li H, Zhang Z, et al. A Pharmacogenomic Landscape in Human Liver Cancers. Cancer Cell 2019;36:179-193.e11. [Crossref] [PubMed]
- Bagati A, Kumar S, Jiang P, et al. Integrin αvβ6-TGFβ-SOX4 Pathway Drives Immune Evasion in Triple-Negative Breast Cancer. Cancer Cell 2021;39:54-67.e9. [Crossref] [PubMed]
- Hutton C, Heider F, Blanco-Gomez A, et al. Single-cell analysis defines a pancreatic fibroblast lineage that supports anti-tumor immunity. Cancer Cell 2021;39:1227-1244.e20. [Crossref] [PubMed]
- Lin W, Noel P, Borazanci EH, et al. Single-cell transcriptome analysis of tumor and stromal compartments of pancreatic ductal adenocarcinoma primary tumors and metastatic lesions. Genome Med 2020;12:80. [Crossref] [PubMed]
(English Language Editor: J. Jones)