Development and validation of a predictive nomogram for the specific mortality risk of luminal B breast cancer patients: a competing risk model based on real populations
Highlight box
Key findings
• We constructed a predictive nomogram of the competitive risk model. The nomogram has ideal accuracy and calibration for the specific death of patients with luminal B breast cancer.
What is known and what is new?
• There is a prediction model for all-cause death of breast cancer patients, and the overall prediction C statistic is between 0.70 and 0.72.
• A predictive nomogram of luminal B breast cancer was constructed for the first time, and Estrogen Receptor and Progesterone Receptor status of patients were included in the analysis.
What is the implication, and what should change now?
• This study indicated that attention should be paid to different Estrogen Receptor and Progesterone Receptor status and to the selection of treatment protocols. The predictive model can provide clinicians with the ability to assess the prognosis and formulate more optimal treatment options.
Introduction
Breast cancer is the most common malignant tumor in women. According to the American Cancer Society, 290,560 new cases occurred in 2022, accounting for 30% of diagnosed female cancer cases, and the numbers are growing at an annual rate of about 0.5% (1). According to its molecular classification, breast cancer is divided into luminal A, luminal B, human epidermal growth factor receptor 2 (HER2)+, and triple-negative breast cancer (TNBC). Among these, luminal B type accounts for about 15% of breast cancer (2). Luminal B type is characterized by the increased expression of HER2, which is considered highly invasive. Five years after the diagnosis is considered the peak period of recurrence. At the same time, Luminal B typle is extremely prone to distant metastasis, such as bone metastasis (3). Compared with other subtypes, chromosome aberration and high levels of DNA amplification are more common in luminal B type, and the level of recurrent genome copy number abnormalities is quite high, which is related to the poor efficacy of clinical treatments (4). Therefore, the treatment and prevention strategies for luminal B breast cancer have attracted extensive attention. With the development of medical technology, targeted therapy and endocrine therapy have become one of the greatest advances in treatment of breast cancer. These treatments have been thought to significantly improve the prognosis of early stage, particularly in patient with luminal B type breast cancer (3). The use of aromatase inhibitors and tamoxifen can reduce the risk of disease recurrence and death by about 30% (5,6). However, the expression of hormone receptors in each patient is different, meaning that some patients cannot benefit from systemic therapy (7-9). The benefits of the treatments vary because of a lack of clarity about adverse risk factors. Therefore, for clinical treatment plans and follow-ups, predicting the prognostic factors of luminal B breast cancer patients and establishing a reasonable predictive model are of great significance.
For the survival status of tumor patients, specific death may have more clinical significance than all-cause death because, in all-cause deaths, many other causes of death are unpredictable, such as accidents, other diseases, and emergencies. This part of the population may reduce the accuracy of the predictive model for all-cause mortality. Therefore, when it comes to cancer patients, specific death is crucial for clinical decision-making. Recently, some researchers have also explored Luminal B breast cancer mortality and related risk factors (10-13). Patient age, tumor size, tumor stage, and lymph node status were the major prognostic factors for breast cancer (12). Besides, a study has shown that the proteolytic activity of proteasome is one of the factors affecting the prognosis of Luminal B breast cancer, because researchers have found the chymotrypsin-like activity and the caspase-like activity coefficients of proteasome are related to the 5-year metastasis-free survival rate in patients with Luminal B HER-2 negative breast cancer (14). However, these studies are based on traditional survival analysis, the number of cases is relatively small, the clinical characteristics are limited, and deaths from other causes must be excluded to some extent, which will cause deviation of prediction results.
SEER database covers a wealth of tumor diseases, and regularly includes clinical retrospective data such as basic information of the patient's population, clinical pathological data, survival state and cause of death. With a large sample size of cases, it has strong statistical efficiency (15). Nomograms integrate different prognostic variables to generate an accurate result (16,17). Balachandran et al. believed that one of the advantages of nomogramas was the ability to predict personalized risks according to the disease conditions of different cancer patients, and promoting the development of personalized clinical treatments (16). Therefore, our research aims to explore the independent risk factors of the death of breast cancer patients and develop a predictive nomogram based on the Surveillance, Epidemiology, and End Results (SEER) Program database. The results of this study can be used as a tool to evaluate specific death. We present the following article in accordance with the TRIPOD reporting checklist (available at https://tcr.amegroups.com/article/view/10.21037/tcr-23-484/rc).
Methods
Data source and data extraction
In this study, we collected patients who were histologically confirmed as luminal B breast cancer patients between 2010 and 2015 from SEER, a database established by the National Cancer Institute of the United States. The SEER database collects corresponding demographic information, clinical pathological information, and follow-up information from 18 registries in the United States, covering about 25% of the United States population. The study was conducted in accordance with the Declaration of Helsinki (as revised in 2013).
From the database, we collected demographic characteristics (race, age, sex, marital status, age of diagnosis), clinical case characteristics (tumor size, laterality, estrogen receptor (ER) status, progesterone receptor (PR) status, histologic tumor grade, TNM stages, lymph node status, metastasis), treatment characteristics (surgery, radiotherapy, chemotherapy), and survival data of all luminal B breast cancer patients. Patients were included if they had a pathological diagnosis of luminal B breast cancer (ICD-O-3 breast cancer) and the following statuses: Her (+), ER (−/+), and PR (−/+). The exclusion criteria were: (I) patients with missing or incomplete data from SEER; (II) patients aged less than 18 years old; and (III) patients with a survival time of less than 1 month.
Construction and validation of the competing risk model
Compared with the traditional risk model, the competing risk model can more accurately evaluate the cumulative incidence of multiple results (18). Therefore, we adopted the competing risk model for our evaluation. We used the primary variables “COD to site rec KM” and “SeerCause-Specific Death Class” in the database to determine the patient’s cause of death, and defined deaths from other causes [other cause mortality (OCM)] as competing events. We defined cases where the patients were still alive at the end of follow-up as right-censored events. The deaths attributed to breast cancer [cancer-specific mortality (CSM)] were labeled as interest events or specific events. Follow-up time was based on the start of patient discharge. Patients who died in hospital were excluded from our study. After data cleansing, all patients were randomized into a training cohort (70%) or a validation cohort (30%). In the single-factor competitive risk model, the variable P<0.05 was included in the multi-factor competitive risk model, and the variable P<0.05 was screened as an independent factor. Based on these independent predictors, a nomogram of specific death was constructed. At the same time, we used the overall C-statistics and the area under the receiver operating characteristic (ROC) curve (AUC) at different time points to evaluate the sensitivity of the nomogram. We used the calibration curve to evaluate the calibration of the predictive nomogram.
Statistical analysis
Continuous data are expressed in the form of mean ± standard deviation, and the differences between the 2 groups were compared using a one-way Analysis of Variance (ANOVA). The categorical data are expressed as n (%), and the differences between groups are compared using the chi-squared test.
We adopted a ratio of 7:3 to divide the included cases into the training and validation cohorts according to the random sampling method. In the training cohort, the influencing factors of luminal B breast cancer–specific death were analyzed using single-factor and multi-factor competing risk models, and a predictive nomogram based on the competing risk model was constructed. In the training and validation cohorts, the accuracy and calibration of the predictive nomogram were verified using the concordance index (c-index) and the area under the ROC curve at different time points. It was generally believed that C-index =0.5 indicated that the model was in a completely random classification, and C-index >0.7 indicated that the model had the ideal accuracy. In addition, we also compared the cumulative specific mortality of the traditional survival analysis and the Fine-Gary method at different time points.
The whole modeling process was completed in R language, and all analyses and statistics were conducted in SPSS 23.0 (IBM Corp., Armonk, NY, USA). A P value of less than 0.05 was considered statistically significant.
Results
Clinical characteristics
According to the inclusion and exclusion criteria, 30,419 luminal B breast cancer cases were included in the analysis. After random sampling, the patients were assigned to the training cohort (n=21,293) or the validation cohort (n=9,126). The average age of the patients was 57.91±13.64 years in the training cohort and 58.23±13.73 years in the validation cohort. The follow-up time for the training and validation cohorts was 61.38±24.561 and 61.31±25.123, respectively. The number of specific deaths was 1,996 (9.37%) in the training cohort and 867 (9.5%) in the validation cohort. The specific distribution of other indicators is shown in Table 1.
Table 1
Factors | Train (n=21,293) | Test (n=9,126) | All (n=30,419) | Statistics (P value) |
---|---|---|---|---|
Age, years | 57.91±13.64 | 58.23±13.73 | 58.01±13.67 | −2.079 (0.038) |
Marriage | 5.47 (0.065) | |||
Married | 12,629 (59.31) | 5,283 (57.89) | 17,912 (58.88) | |
Single | 3,654 (17.16) | 1,607 (17.61) | 5,261 (17.3) | |
Other | 5,010 (23.53) | 2,236 (24.5) | 7,246 (23.82) | |
Race | 0.295 (0.587) | |||
White | 16,529 (77.63) | 7,110 (77.91) | 23,639 (77.71) | |
Other | 4,764 (22.37) | 2,016 (22.09) | 6,780 (22.29) | |
Laterality | 0.878 (0.349) | |||
Right | 10,839 (50.9) | 4,699 (51.49) | 15,538 (51.08) | |
Left | 10,454 (49.1) | 4,427 (48.51) | 14,881 (48.92) | |
Primary site | 10.78 (0.013) | |||
C50.4 | 8,784 (41.25) | 3,723 (40.8) | 12,507 (41.12) | |
C50.2 | 3,589 (16.86) | 1,532 (16.79) | 5,121 (16.83) | |
C50.8 | 4,802 (22.55) | 2,201 (24.12) | 7,003 (23.02) | |
Other | 4,118 (19.34) | 1,670 (18.3) | 5,788 (19.03) | |
Pathological behavior | 0.376 (0.54) | |||
Behav1 | 19,059 (89.51) | 8,147 (89.27) | 27,206 (89.44) | |
Other | 2,234 (10.49) | 979 (10.73) | 3,213 (10.56) | |
Grade | 3.13 (0.209) | |||
I | 1,410 (6.62) | 655 (7.18) | 2,065 (6.79) | |
II | 8,905 (41.82) | 3,787 (41.5) | 12,692 (41.72) | |
III/IV | 10,978 (51.56) | 4,684 (51.33) | 15,662 (51.49) | |
Stage | 1.665 (0.645) | |||
I | 8,593 (40.36) | 3,745 (41.04) | 12,338 (40.56) | |
II | 8,391 (39.41) | 3,582 (39.25) | 11,973 (39.36) | |
III | 3,122 (14.66) | 1,300 (14.25) | 4,422 (14.54) | |
IV | 1,187 (5.57) | 499 (5.47) | 1,686 (5.54) | |
T | 2.306 (0.511) | |||
T1 | 10,620 (49.88) | 4,595 (50.35) | 15,215 (50.02) | |
T2 | 8,004 (37.59) | 3,381 (37.05) | 11,385 (37.43) | |
T3 | 1,615 (7.58) | 720 (7.89) | 2,335 (7.68) | |
T4 | 1,054 (4.95) | 430 (4.71) | 1,484 (4.88) | |
N | 10.106 (0.018) | |||
N0 | 12,728 (59.78) | 5,582 (61.17) | 18,310 (60.19) | |
N1 | 6,144 (28.85) | 2,546 (27.9) | 8,690 (28.57) | |
N2 | 1,516 (7.12) | 584 (6.4) | 2,100 (6.9) | |
N3 | 905 (4.25) | 414 (4.54) | 1,319 (4.34) | |
M | 0.139 (0.709) | |||
M0 | 20,106 (94.43) | 8,627 (94.53) | 28,733 (94.46) | |
M1 | 1,187 (5.57) | 499 (5.47) | 1,686 (5.54) | |
Surgery | 3.384 (0.336) | |||
Surg1 | 1,558 (7.32) | 716 (7.85) | 2,274 (7.48) | |
Surg2 | 9,307 (43.71) | 4,000 (43.83) | 13,307 (43.75) | |
Surg3 | 5,946 (27.92) | 2,540 (27.83) | 8,486 (27.9) | |
Surg4 | 4,482 (21.05) | 1,870 (20.49) | 6,352 (20.88) | |
Other surgical | 0.57 (0.45) | |||
Yes | 20,842 (97.88) | 8,945 (98.02) | 29,787 (97.92) | |
None | 451 (2.12) | 181 (1.98) | 632 (2.08) | |
Radiation | 5.109 (0.024) | |||
Yes | 11,235 (52.76) | 4,944 (54.17) | 16,179 (53.19) | |
None | 10,058 (47.24) | 4,182 (45.83) | 14,240 (46.81) | |
Chemotherapy | 0.002 (0.969) | |||
Yes | 15,530 (72.93) | 6,658 (72.96) | 22,188 (72.94) | |
None | 5,763 (27.07) | 2,468 (27.04) | 8,231 (27.06) | |
LNAxillary | 6.023 (0.049) | |||
Negative | 11,422 (53.64) | 5,006 (54.85) | 16,428 (54.01) | |
Positive | 7,493 (35.19) | 3,078 (33.73) | 10,571 (34.75) | |
No examined | 2,378 (11.17) | 1,042 (11.42) | 3,420 (11.24) | |
Bone | 0 (0.984) | |||
Yes | 20,566 (96.59) | 8,814 (96.58) | 29,380 (96.58) | |
None | 727 (3.41) | 312 (3.42) | 1,039 (3.42) | |
Brain | 4.003 (0.045) | |||
Yes | 21,221 (99.66) | 9,081 (99.51) | 30,302 (99.62) | |
None | 72 (0.34) | 45 (0.49) | 117 (0.38) | |
Liver | 0.093 (0.761) | |||
Yes | 20,898 (98.14) | 8,952 (98.09) | 29,850 (98.13) | |
None | 395 (1.86) | 174 (1.91) | 569 (1.87) | |
Lung | 0.005 (0.946) | |||
Yes | 20,936 (98.32) | 8,972 (98.31) | 29,908 (98.32) | |
None | 357 (1.68) | 154 (1.69) | 511 (1.68) | |
Size | 0.005 (0.997) | |||
<2 cm | 9,843 (46.23) | 4,216 (46.2) | 14,059 (46.22) | |
2–5 cm | 8,864 (41.63) | 3,803 (41.67) | 12,667 (41.64) | |
>5 cm | 2,586 (12.14) | 1,107 (12.13) | 3,693 (12.14) | |
Malignant | 0.009 (0.924) | |||
1 | 16,518 (77.57) | 7,084 (77.62) | 23,602 (77.59) | |
>1 | 4,775 (22.43) | 2,042 (22.38) | 6,817 (22.41) | |
Estrongen receptor | 0.193 (0.66) | |||
+ | 20,724 (97.33) | 8,874 (97.24) | 29,598 (97.3) | |
− | 569 (2.67) | 252 (2.76) | 821 (2.7) | |
Progesterone receptor | 2.682 (0.101) | |||
+ | 15,620 (73.36) | 6,777 (74.26) | 22,397 (73.63) | |
− | 5,673 (26.64) | 2,349 (25.74) | 8,022 (26.37) | |
Status | 3.694 (0.158) | |||
Alive | 18,043 (84.74) | 7,671 (84.06) | 25,714 (84.53) | |
Specific mortality | 1,996 (9.37) | 867 (9.5) | 2,863 (9.41) | |
Other mortality | 1,254 (5.89) | 588 (6.44) | 1,842 (6.06) | |
Follow-up time | 61.38±24.561 | 61.31±25.123 | 61.36±24.731 | 0.225 (0.822) |
Data are shown as mean ± standard deviation or number (percentage). LNAxillary, lymph node Axillary metastasis status; Behav1, 8500/3: infiltrating duct carcinoma, no otherwise specified; 8521/3: infiltrating ductular carcinoma; 8522/3: infiltrating duct and lobular carcinoma; 8523/3: infiltrating duct mixed with other types of carcinoma. Surg1: no surgery was performed, and the surgery code in SEER is 0; Surg2: Local tumor destruction or Partial mastectomy, and the surgery code in SEER is 19-24; Surg3: Subcutaneous Mastectomy or Total (SIMPLE) Mastectomy, and the surgery code in SEER is 30–48; Surg4: Other radical surgery, and the surgery code in SEER is 50–90. SEER, Surveillance, Epidemiology, and End Results.
Analysis of the influencing factors
All variables were screened by single-factor analysis. The results showed a significant difference (P<0.05) in the following factors: married, primary site, grade, T stage, N stage, surgery, radiotherapy, chemotherapy, LNAxillary, metastatic status, size, malignant, ER, and PR. These factors may be the influencing factors of specific death. Therefore, these variables were put into the multi-factor competing risk model to analyze and evaluate the independent predictors of mortality risk of luminal B breast cancer. The results showed that married, primary site, grade, T stage, N stage, surgery, radiation, chemotherapy, LNAxillary, bone, brain, liver, lung, ER, and PR were independent predictors of specific death risk of luminal B breast cancer (Table 2).
Table 2
Factors | Single-factor analysis | Multi-factor analysis | |||
---|---|---|---|---|---|
HR (95% CI) | Z (P value) | HR (95% CI) | Z (P value) | ||
Age | 1.024 (1.020–1.028) | 12.821 (<0.001) | 1.016 (1.012–1.02) | 7.825 (0) | |
Marriage | |||||
Married | Ref | NA | Ref | NA | |
Single | 1.610 (1.433–1.808) | 8.015 (<0.001) | 1.272 (1.126–1.436) | 3.881 (0) | |
Other | 1.951 (1.766–2.155) | 13.154 (<0.001) | 1.252 (1.118–1.401) | 3.905 (0) | |
Race | |||||
White | Ref | NA | |||
Other | 1.095 (0.988–1.214) | 1.728 (0.084) | |||
Laterality | |||||
Right | Ref | NA | |||
Left | 0.967 (0.886–1.056) | −0.740 (0.46) | |||
Primary site | |||||
C50.4 | Ref | NA | Ref | NA | |
C50.2 | 1.038 (0.905–1.189) | 0.530 (0.600) | 1.203 (1.045–1.386) | 2.568 (0.01) | |
C50.8 | 1.163 (1.032–1.312) | 2.467 (0.014) | 1.06 (0.934–1.202) | 0.895 (0.37) | |
Other | 1.891 (1.694–2.111) | 11.328 (<0.001) | 1.232 (1.095–1.385) | 3.48 (0.001) | |
Pathological behavior | |||||
Behav1 | Ref | NA | |||
Other | 1.073 (0.933–1.234) | 0.992 (0.32) | |||
Grade | |||||
I | Ref | NA | Ref | NA | |
II | 2.337 (1.755–3.112) | 5.810 (<0.001) | 1.744 (1.305–2.331) | 3.756 (0) | |
III/IV | 3.364 (2.536–4.460) | 8.423 (<0.001) | 2.172 (1.627–2.899) | 5.263 (0) | |
Stage | |||||
I | Ref | NA | Ref | NA | |
II | 2.675 (2.304–3.107) | 12.900 (<0.001) | 1.699 (1.361–2.121) | 4.688 (0) | |
III | 7.271 (6.257–8.449) | 25.900 (<0.001) | 3.18 (2.432–4.158) | 8.458 (0) | |
IV | 26.293 (22.606–30.581) | 42.413 (<0.001) | 4.675 (3.422–6.387) | 9.685 (0) | |
T | |||||
T1 | Ref | NA | Ref | NA | |
T2 | 2.694 (2.404–3.018) | 17.070 (<0.001) | 1.183 (0.919–1.524) | 1.302 (0.19) | |
T3 | 5.350 (4.638–6.171) | 23.024 (<0.001) | 1.22 (0.898–1.656) | 1.271 (0.2) | |
T4 | 10.337 (8.993–11.881) | 32.880 (<0.001) | 1.275 (0.967–1.682) | 1.721 (0.085) | |
N | |||||
N0 | Ref | NA | Ref | NA | |
N1 | 2.678 (2.411–2.976) | 18.343 (<0.001) | 1.158 (0.958–1.399) | 1.512 (0.13) | |
N2 | 4.488 (3.930–5.131) | 21.996 (<0.001) | 1.29 (1.02–1.63) | 2.126 (0.033) | |
N3 | 7.245 (6.287–8.349) | 27.368 (<0.001) | 1.617 (1.27–2.058) | 3.898 (0) | |
M | |||||
M0 | Ref | NA | |||
M1 | 10.038 (9.100–11.077) | 45.891 (<0.001) | |||
Surgery | |||||
Surg1 | Ref | NA | Ref | NA | |
Surg2 | 0.086 (0.076–0.098) | −37.461 (<0.001) | 0.508 (0.421–0.613) | –7.07 (0) | |
Surg3 | 0.125 (0.109–0.142) | −30.895 (<0.001) | 0.579 (0.485–0.692) | –5.999 (0) | |
Surg4 | 0.286 (0.255–0.320) | −21.458 (<0.001) | 0.735 (0.621–0.87) | –3.578 (0) | |
Other surgical | |||||
Yes | 1.136 (0.853–1.511) | 0.872 (0.38) | |||
None | Ref | NA | |||
Radiation | |||||
Yes | 0.633 (0.578–0.693) | −9.917 (<0.001) | 0.848 (0.761–0.946) | –2.967 (0.003) | |
None | Ref | NA | Ref | NA | |
Chemotherapy | |||||
Yes | Ref | NA | Ref | NA | |
None | 1.550 (1.414–1.699) | 9.357 (<0.001) | 2.055 (1.829–2.31) | 12.084 (0) | |
LNAxillary | |||||
Negative | Ref | NA | Ref | NA | |
Positive | 4.401 (3.916–4.946) | 24.856 (<0.001) | 1.642 (1.336–2.019) | 4.709 (0) | |
No examined | 8.748 (7.682–9.961) | 32.712 (<0.001) | 2.18 (1.833–2.592) | 8.82 (0) | |
Bone | |||||
Yes | 10.315 (9.213–11.549) | 40.670 (<0.001) | 1.618 (1.316–1.991) | 4.555 (0) | |
None | Ref | NA | Ref | NA | |
Brain | |||||
Yes | 17.583 (13.135–23.537) | 19.266 (<0.001) | 2.318 (1.596–3.368) | 4.413 (0) | |
None | Ref | NA | Ref | NA | |
Liver | |||||
Yes | 9.950 (8.571–11.551) | 30.181 (<0.001) | 1.39 (1.136–1.702) | 3.192 (0.001) | |
None | Ref | NA | Ref | NA | |
Lung | |||||
Yes | 11.104 (9.495–12.985) | 30.145 (<0.001) | 1.395 (1.142–1.705) | 3.257 (0.001) | |
None | Ref | NA | Ref | NA | |
Size | |||||
<2 cm | Ref | NA | Ref | NA | |
2–5 cm | 2.682 (2.394–3.006) | 16.982 (<0.001) | 1.102 (0.86–1.412) | 0.77 (0.44) | |
>5 cm | 6.254 (5.424–7.081) | 28.945 (<0.001) | 1.203 (0.91–1.59) | 1.301 (0.19) | |
Malignant | |||||
1 | Ref | NA | Ref | NA | |
>1 | 1.267 (1.148–1.398) | 4.705 (<0.001) | 1.16 (1.041–1.293) | 2.678 (0.007) | |
Estrongen receptor | |||||
+ | Ref | NA | Ref | NA | |
− | 1.367 (1.075–1.739) | 2.554 (0.011) | 1.386 (1.08–1.78) | 2.563 (0.01) | |
Progesterone receptor | |||||
+ | Ref | NA | Ref | NA | |
− | 1.544 (1.409–1.693) | 9.264 (<0.001) | 1.382 (1.251–1.526) | 6.386 (0) |
Behav1, 8500/3: infiltrating duct carcinoma, no otherwise specified; 8521/3: infiltrating ductular carcinoma; 8522/3: infiltrating duct and lobular carcinoma; 8523/3: infiltrating duct mixed with other types of carcinoma. Surg1: no surgery was performed, and the surgery code in SEER is 0; Surg2: Local tumor destruction or Partial mastectomy, and the surgery code in SEER is 19-24; Surg3: Subcutaneous Mastectomy or Total (SIMPLE) Mastectomy, and the surgery code in SEER is 30-48; Surg4: Other radical surgery, and the surgery code in SEER is 50-90. HR, hazard ratio; CI, confidence interval; NA, not acception; SEER, Surveillance, Epidemiology, and End Results.
Construction and validation of the predictive nomogram
The predictive nomogram based on the competing risk model, covering all the above independent predictors, was used to determine the risk stratification of specific death of luminal B patients (Figure 1). See Supplementary materials (Figures S1-S6) for the cumulative mortality rate under different levels of each predictor. In the training cohort, the overall C statistic of the predictive nomogram was 0.858 (SE: 0.001016844), and the AUC for predicting specific death at 1, 3, and 5 years was 0.891 (95% CI: 0.870–0.912), 0.864 (95% CI: 0.852–0.875), and 0.845 (95% CI: 0.834–0.856), respectively (Figure 2). In the validation cohort, the overall C statistic was 0.8620 (SE: 001520709), and the AUC of specific death at 1, 3, and 5 years was 0.888 (95% CI: 0.859–0.917), 0.872 (95% CI: 0.856–0.889), and 0.849 (95% CI: 0.833–0.865) respectively, which also showed that the model had good discrimination (Figure 3).
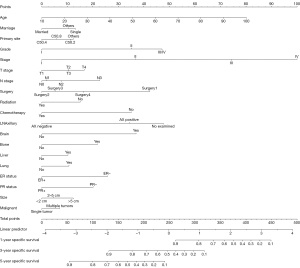
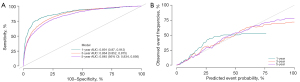
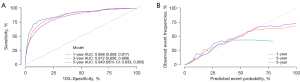
In the training and validation cohorts, the calibration curves of the model showed that the predicted values of the model were consistent with the actual observed values, indicating that the model had good accuracy and calibration (Figures 2,3).
Specific mortality rate estimates
We calculated the cumulative mortality according to the traditional survival analysis and the competing risk model at the same time points. The results of both calculations demonstrated differences at each time point. Moreover, compared with the CSM by the competitive risk model, the CSM by the traditional survival analysis were high, indicating that the predictive nomogram could predict luminal B breast cancer more accurately than the traditional TNM staging system (Table 3).
Table 3
Time (year) | Specific mortality calculated using traditional survival analysis (%) | Competitive risk model | |
---|---|---|---|
Specific mortality (%) | Other causes of death (%) | ||
1 | 1.71 | 1.61 | 0.93 |
2 | 3.51 | 3.29 | 1.92 |
3 | 5.78 | 5.43 | 3.02 |
4 | 7.79 | 7.3 | 4.14 |
5 | 9.49 | 8.88 | 5.23 |
6 | 10.97 | 10.23 | 6.52 |
7 | 12.25 | 11.4 | 7.78 |
8 | 13.56 | 12.59 | 8.99 |
Discussion
Compared with other breast cancer subtypes, luminal B breast cancer has a poor disease-free survival rate, a high early recurrence rate, and is prone to metastasis, leading to a worse survival outcome (19). Therefore, efforts should be made to improve the early diagnosis rate and develop effective treatments to help patients’ prognoses. Using the data of 30,419 patients extracted from the SEER database, we constructed a competing risk model for specific death of luminal B breast cancer, identified 17 independent factors that were significantly related to overall survival (OS), and developed a nomogram to predict the 1-, 3-, and 5-year survival rates. The results showed that the nomogram had great accuracy, with a C statistic of 0.858, a high degree of calibration, and could provide solid guidance for prognosis prediction and disease treatment and management.
There are already many predictive models for the all-cause death of breast cancer based on the SEER database. We found that their overall prediction C statistic was between 0.70 and 0.72 (20,21). In addition, we found that, when their all-cause mortality models were constructed, the SEER predictors did not include some of the basic diseases of the patients and some regular indicators at the time of hospitalization. Therefore, in tumors with a high proportion of deaths from other causes, models based on the population on which SEER data are based cannot guarantee an effective prediction. At the same time, we also found a few studies that constructed specific death models for breast cancer (20,22), and they excluded patients [who died from other causes (such as other diseases and emergencies] when constructing their specific death models. However, in our research, we found that this part of the population accounted for about 20% of the deaths. This way of processing causes deviation to the predictors and poses certain challenges, especially in clinical applications.
According to our single-factor and multi-factor analyses, the factors of marital status, primary site, grade, T stage, N stage, surgery method, radiotherapy, chemotherapy, LNAxillary, bone metastasis, brain metastasis, liver metastasis, lung metastasis, ER,and PR were significantly related to the prognosis of luminal B breast cancer. In our study, being single or being divorced patients have worse prognosis than being married patients. Epidemiology findings support our results (23). Ding et al. (24) evaluated the prognostic factors of 700 luminal B patients, including age, tumor size, TNM stage, and lymph node involvement. Similarly, our results also showed that patients with higher staging and bigger tumor sizes had a heavier disease burden, which was a key factor affecting the prognosis. We also found that the primary tumor location related to the specific death of luminal B breast cancer and that, when the tumor originated from the nipple, the center, and the axillary tail, the risks were higher (P<0.05). These findings are consistent with the conclusions of recent studies (25,26). Wu et al. found that the OS of tumors located in the lower inner quadrant of axillary in 5 years and 10 years was significantly lower than other groups (such as the upper outer quadrant group) (26).
Lymph node metastasis was considered a key factor in influencing breast cancer treatment decisions (27). Although it is unknown whether luminal B breast cancer is more likely than the other molecular subtypes to have lymph node metastasis, compared with luminal A breast cancer, the association between luminal B tumors and lymph node involvement was more statistically significant (P<0.001) (11). Our study results showed that patients with axillary lymph node metastasis had a higher risk of death than those without axillary lymph node metastasis. Therefore, axillary lymph node biopsy and imaging evaluation are necessary for clinical work. According to reports, Luminal B breast cancer is highly aggressive and easily metastasizes to distant sites, such as bone (3). Approximately 5 million people die each year from metastatic breast cancer because of the poor quality of life of breast cancer patients with distant metastasis and the poor efficacy of treatment (28). We found that patients with bone, liver, lung, and other metastases had an increased risk of a poor prognosis. The study by Li et al. (29) collected 258 cases of luminal B breast cancer patients and found that the proportion of bone metastasis and the recurrence risk were higher than that of patients in the non-luminal B cancer. These results suggest that clinicians could use imaging examinations to monitor prognosis and treatment as early as possible.
According to a literature review, mastectomy (MTS) was usually used as the primary treatment for breast cancer (30). However, with the development of medicine, a recent study has shown that the risk of recurrence in MTS was higher than that in breast-conserving surgery group, and the 10-year specific survival rate (BCSS) of patients who undergo breast-conserving surgery is also significantly higher than that of the MTS group (96.6% vs. 88.3%; P<0.001) (31). After adjusting the clinical factors and demographic information, the prognosis risk of all types of MTS was still high compared with that of the BCT group (32). Our results also found that different surgical methods had different outcomes. In addition, in actual clinical work, some female patients have a strong desire for breast conservation and lactation demand. This suggesting that clinical work will need to carefully decide on the surgical method through a comprehensive evaluation of the patient’s needs and the condition.
Currently, chemotherapy has become the latest treatment strategy for luminal B breast cancer (33). The results of several retrospective analyses and randomized clinical trials also showed that, in luminal B breast cancer, neoadjuvant chemotherapy could reduce the long-term 10 years recurrence rate by an average of 27.6% by killing or inhibiting cancer cells and reducing tumor volume (34). Our multi-factor analysis showed that patients who received chemotherapy had a better prognosis than those who did not receive chemotherapy; these patients had longer survival periods than those who did not receive chemotherapy. The indicates that patients receiving chemotherapy benefit from treatment. Therefore, the specific regimen of adjuvant chemotherapy and adjuvant chemotherapy can be determined as early as possible according to the stages of patients.
The prognosis of different ER and PR levels is also controversial (35,36). In a randomized trial, patients with negative ER and PR were found to have worse prognoses after receiving letrozole and tamoxifen (35). However, Dowsett et al. (36) stated in their retrospective analysis that the recurrence time of ER+/PgR+ and ER+/PgR− patients was prolonged after treatment with anastrozole, but the treatment benefit of the ER+/PgR− group was significantly greater than that of the ER+/PgR+ group. In our study, multi-factor analysis showed that ER+ and PR+ had a positive effect on improving the prognosis of patients. This finding may be due to the different therapeutic drugs used or the differences in clinicopathological features between patients, so the effect of ER and PR on CSM of patients with luminal B breast cancer needs to be discussed in more studies.
Radiotherapy, one of the classic adjuvant therapies, provides patients with surgery opportunities or tumor cleaning after surgery. Consistent with Li et al’s. study, we found that patients with adjuvant radiotherapy had a better prognosis than those who did not undergo radiotherapy (37). However, the results in 2 randomized trials conflicts with our study (38,39). Sjöström et al. found that while radiotherapy reduced the recurrence rate of breast cancer with triple-negative tumors in the first 10 years, other subtypes did not have obvious effects. And in any subtype, radiotherapy did not reduce the death from any cause (39). The difference may have occurred because no Her-patients were included in the population of the Sjöström’s study. This conflicting result suggests that further validation may be needed by conducting multi-center, large-data randomized trials.
We also compared the traditional survival analysis and the competing risk model. The results of the assessment of cumulative mortality at different time points showed that the proportion of other causes of death increased significantly with the increase of time. More importantly, the traditional analysis shows that the mortality rates at 1, 5, and 8 years were 1.71%, 9.49%, and 13.56%, while the mortality rates assessed using the competing risk model was lower, with the results of 1.61%, 8.88%, and 12.59%. Our study showed that there were differences between the cumulative specific mortality estimated by the competitive risk model and the Cox regression, and Cox regression seemed to overestimate the cumulative specific mortality at different time points.
We constructed a predictive nomogram of luminal B breast cancer for the first time. Our nomogram has good accuracy and rationality and can provide a basis for the development and innovation of clinical scoring tools. However, there were some limitations. First, other effective predictors of specific death (such as hypertension and other comorbidities, radiotherapy and chemotherapy methods, response to radiotherapy and chemotherapy) are lacking in SEER database, which limits the accuracy of the model to some extent. In the future, we will include more comprehensive clinical data, such as immunotherapy, gene test, and tumor markers. Second, data from other institutions were not used, leading to our inclusion case limitations. Additional institutional data will be included to add clinical samples from different regions to supplement and further validate the model.
Conclusions
The nomogram we developed has a satisfactory predictive effect. It can be applied to early clinical-specific death risk stratification and can guide the future development and validation of subsequent scoring tools. Our nomogram can also be used in the clinical development of personalized treatments and follow-up strategies for patients with luminal B breast cancer.
Acknowledgments
Funding: None.
Footnote
Reporting Checklist: The authors have completed the TRIPOD reporting checklist. Available at https://tcr.amegroups.com/article/view/10.21037/tcr-23-484/rc
Peer Review File: Available at https://tcr.amegroups.com/article/view/10.21037/tcr-23-484/prf
Conflicts of Interest: All authors have completed the ICMJE uniform disclosure form (available at https://tcr.amegroups.com/article/view/10.21037/tcr-23-484/coif).The authors have no conflicts of interest to declare.
Ethical Statement: The authors are accountable for all aspects of the work in ensuring that questions related to the accuracy or integrity of any part of the work are appropriately investigated and resolved. The study was conducted in accordance with the Declaration of Helsinki (as revised in 2013).
Open Access Statement: This is an Open Access article distributed in accordance with the Creative Commons Attribution-NonCommercial-NoDerivs 4.0 International License (CC BY-NC-ND 4.0), which permits the non-commercial replication and distribution of the article with the strict proviso that no changes or edits are made and the original work is properly cited (including links to both the formal publication through the relevant DOI and the license). See: https://creativecommons.org/licenses/by-nc-nd/4.0/.
References
- Siegel RL, Miller KD, Fuchs HE, et al. Cancer statistics, 2022. CA Cancer J Clin 2022;72:7-33. [Crossref] [PubMed]
- Waks AG, Winer EP. Breast Cancer Treatment: A Review. JAMA 2019;321:288-300. [Crossref] [PubMed]
- Ades F, Zardavas D, Bozovic-Spasojevic I, et al. Luminal B breast cancer: molecular characterization, clinical management, and future perspectives. J Clin Oncol 2014;32:2794-803. [Crossref] [PubMed]
- Chin K, DeVries S, Fridlyand J, et al. Genomic and transcriptional aberrations linked to breast cancer pathophysiologies. Cancer Cell 2006;10:529-41. [Crossref] [PubMed]
- Early Breast Cancer Trialists' Collaborative Group (EBCTCG). Effects of chemotherapy and hormonal therapy for early breast cancer on recurrence and 15-year survival: an overview of the randomised trials. Lancet 2005;365:1687-717. [Crossref] [PubMed]
- Coombes RC, Hall E, Gibson LJ, et al. A randomized trial of exemestane after two to three years of tamoxifen therapy in postmenopausal women with primary breast cancer. N Engl J Med 2004;350:1081-92. [Crossref] [PubMed]
- von Minckwitz G, Procter M, de Azambuja E, et al. Adjuvant Pertuzumab and Trastuzumab in Early HER2-Positive Breast Cancer. N Engl J Med 2017;377:122-31. [Crossref] [PubMed]
- Bardou VJ, Arpino G, Elledge RM, et al. Progesterone receptor status significantly improves outcome prediction over estrogen receptor status alone for adjuvant endocrine therapy in two large breast cancer databases. J Clin Oncol 2003;21:1973-9. [Crossref] [PubMed]
- Coombes RC, Kilburn LS, Snowdon CF, et al. Survival and safety of exemestane versus tamoxifen after 2-3 years' tamoxifen treatment (Intergroup Exemestane Study): a randomised controlled trial. Lancet 2007;369:559-70. [Crossref] [PubMed]
- Haybittle JL, Blamey RW, Elston CW, et al. A prognostic index in primary breast cancer. Br J Cancer 1982;45:361-6. [Crossref] [PubMed]
- Cheang MC, Chia SK, Voduc D, et al. Ki67 index, HER2 status, and prognosis of patients with luminal B breast cancer. J Natl Cancer Inst 2009;101:736-50. [Crossref] [PubMed]
- Hashmi AA, Aijaz S, Khan SM, et al. Prognostic parameters of luminal A and luminal B intrinsic breast cancer subtypes of Pakistani patients. World J Surg Oncol 2018;16:1. [Crossref] [PubMed]
- Rajc J, Fröhlich I, Mrčela M, et al. Prognostic impact of low estrogen and progesterone positivity in luminal b (HER2 negative) breast cancer. Acta Clin Croat 2018;57:425-33. [Crossref] [PubMed]
- Sereda EE, Kolegova ES, Kakurina GV, Korshunov DA, Sidenko EA, Doroshenko AV, Slonimskaya EM, Kondakova IV. Five-year survival in luminal breast cancer patients: relation with intratumoral activity of proteasomes. Transl Breast Cancer Res 2022;3:23. [Crossref]
- Doll KM, Rademaker A, Sosa JA. Practical Guide to Surgical Data Sets:Surveillance, Epidemiology, and End Results (SEER) Database. JAMA Surg 2018;153:588-9. [Crossref] [PubMed]
- Balachandran VP, Gonen M, Smith JJ, et al. Nomograms in oncology: more than meets the eye. Lancet Oncol 2015;16:e173-80. [Crossref] [PubMed]
- Iasonos A, Schrag D, Raj GV, et al. How to build and interpret a nomogram for cancer prognosis. J Clin Oncol 2008;26:1364-70. [Crossref] [PubMed]
- Putter H, Fiocco M, Geskus RB. Tutorial in biostatistics: competing risks and multi-state models. Stat Med 2007;26:2389-430. [Crossref] [PubMed]
- Tran B, Bedard PL. Luminal-B breast cancer and novel therapeutic targets. Breast Cancer Res 2011;13:221. [Crossref] [PubMed]
- Tu Q, Hu C, Zhang H, et al. Establishment and Validation of Novel Clinical Prognosis Nomograms for Luminal A Breast Cancer Patients with Bone Metastasis. Biomed Res Int 2020;2020:1972064. [Crossref] [PubMed]
- Tang Z, Ji Y, Min Y, et al. Prognostic Factors and Models for Elderly (≥70 Years Old) Primary Operable Triple-Negative Breast Cancer: Analysis From the National Cancer Database. Front Endocrinol (Lausanne) 2022;13:856268. [Crossref] [PubMed]
- Liu R, Xiao Z, Hu D, et al. Cancer-Specific Survival Outcome in Early-Stage Young Breast Cancer: Evidence From the SEER Database Analysis. Front Endocrinol (Lausanne) 2022;12:811878. [Crossref] [PubMed]
- Logan WP. Marriage and childbearing in relation to cancer of the breast and uterus. Lancet 1953;265:1199-202. [Crossref] [PubMed]
- Ding NH, Liu CF, Hu C, et al. Prognostic Factors for Luminal B-like Breast Cancer. Curr Med Sci 2019;39:396-402. [Crossref] [PubMed]
- Yang J, Tang S, Zhou Y, et al. Prognostic implication of the primary tumor location in early-stage breast cancer: focus on lower inner zone. Breast Cancer 2018;25:100-7. [Crossref] [PubMed]
- Wu S, Zhou J, Ren Y, et al. Tumor location is a prognostic factor for survival of Chinese women with T1-2N0M0 breast cancer. Int J Surg 2014;12:394-8. [Crossref] [PubMed]
- Arroyo-Crespo JJ, Armiñán A, Charbonnier D, et al. Characterization of triple-negative breast cancer preclinical models provides functional evidence of metastatic progression. Int J Cancer 2019;145:2267-81. [Crossref] [PubMed]
- Cardoso F, Spence D, Mertz S, et al. Global analysis of advanced/metastatic breast cancer: Decade report (2005-2015). Breast 2018;39:131-8. [Crossref] [PubMed]
- Li ZH, Hu PH, Tu JH, et al. Luminal B breast cancer: patterns of recurrence and clinical outcome. Oncotarget 2016;7:65024-33. [Crossref] [PubMed]
- Zurrida S, Veronesi U. Milestones in breast cancer treatment. Breast J 2015;21:3-12. [Crossref] [PubMed]
- Li W, Zheng Y, Wu H, et al. Breast-conserving therapy versus mastectomy for breast cancer: a ten-year follow-up single-center real-world study. Gland Surg 2022;11:1148-65. [Crossref] [PubMed]
- Chu QD, Hsieh MC, Yi Y, et al. Outcomes of Breast-Conserving Surgery Plus Radiation vs Mastectomy for All Subtypes of Early-Stage Breast Cancer: Analysis of More Than 200,000 Women. J Am Coll Surg 2022;234:450-64. [Crossref] [PubMed]
- Goldhirsch A, Wood WC, Coates AS, et al. Strategies for subtypes--dealing with the diversity of breast cancer: highlights of the St. Gallen International Expert Consensus on the Primary Therapy of Early Breast Cancer 2011. Ann Oncol 2011;22:1736-47. [Crossref] [PubMed]
- Paik S, Tang G, Shak S, et al. Gene expression and benefit of chemotherapy in women with node-negative, estrogen receptor-positive breast cancer. J Clin Oncol 2006;24:3726-34. [Crossref] [PubMed]
- Viale G, Regan MM, Maiorano E, et al. Prognostic and predictive value of centrally reviewed expression of estrogen and progesterone receptors in a randomized trial comparing letrozole and tamoxifen adjuvant therapy for postmenopausal early breast cancer: BIG 1-98. J Clin Oncol 2007;25:3846-52. [Crossref] [PubMed]
- Dowsett M, Cuzick J, Wale C, et al. Retrospective analysis of time to recurrence in the ATAC trial according to hormone receptor status: an hypothesis-generating study. J Clin Oncol 2005;23:7512-7. [Crossref] [PubMed]
- Li Z, Shi Y, Wu L, et al. Establishment and verification of a nomogram to predict tumor-specific mortality risk in triple-negative breast cancer: a competing risk model based on the SEER cohort study. Gland Surg 2022;11:1961-75. [Crossref] [PubMed]
- Wickberg Å, Magnuson A, Holmberg L, et al. Influence of the subtype on local recurrence risk of breast cancer with or without radiation therapy. Breast 2018;42:54-60. [Crossref] [PubMed]
- Sjöström M, Lundstedt D, Hartman L, et al. Response to Radiotherapy After Breast-Conserving Surgery in Different Breast Cancer Subtypes in the Swedish Breast Cancer Group 91 Radiotherapy Randomized Clinical Trial. J Clin Oncol 2017;35:3222-9. [Crossref] [PubMed]
(English Language Editor: C. Mullens)