Network pharmacology- and molecular docking-based investigation of the therapeutic potential and mechanism of daucosterol against multiple myeloma
Highlight box
Key findings
• In the PPI network, the target of daucosterol was significantly correlated with MM-related genes, indicating that it has therapeutic potential for MM.
• A total of 18 therapeutic targets for MM were obtained, which were significantly enriched in the FoxO signaling pathway, prostate cancer, the PI3K-Akt signaling pathway, insulin resistance, the AMPK signaling pathway, and pathways related to the regulation of TP53.
• The core targets were HSP90AA1, MDM2, GSK3B, AKT3, PRKAA1, and PRKAB1.
What is known and what is new?
• Some studies have shown that daucosterol has potential anti-tumor activity, but its therapeutic effect on MM has not been reported.
• This study highlights that daucosterol has promising therapeutic potential for MM treatment.
What is the implication, and what should change now?
• This study reflects the characteristics of pharmacological effects of natural products with “multiple targets and multiple pathways”, which lays a foundation for the subsequent in-depth study of daucosterol in the treatment of MM and provides a basis for its application in the clinical treatment of MM.
Introduction
Multiple myeloma (MM) is a kind of malignant proliferative disease derived from monoclonal plasma cells. It is typically characterized by the production of a large amount of monoclonal immunoglobulin, which eventually leads to end-stage impairment of multiple organ function (1,2). MM is common among middle-aged and elderly patients, predominantly in elderly male patients (over 65 years old). The incidence of MM shows an increasing trend year by year (1,3), and currently ranks the second among hematological malignancies, second only to non-Hodgkin’s lymphoma (4). In the past 30 years, high-dose chemotherapy, immunomodulatory drugs (IMiDs), proteasome inhibitors (PIs), anti-CD38 monoclonal antibody, autologous hematopoietic stem cell transplantation, and chimeric antigen receptor T cells (CAR-T) have greatly improved the quality of life of patients, but MM currently remains incurable (5-7). During the treatment, patients may experience side effects such as diarrhea, thrombocytopenia, peripheral neuritis, pulmonary infection, and thrombosis (8,9). Clonal evolution of MM cells and an immunosuppressive tumor microenvironment are the main causes of relapse, progression, and drug resistance in MM (10,11). First-line drugs such as bortezomib are effective but expensive. Natural compounds with low side effects are cheap, easy to obtain, and have great potential application value in the treatment of various malignant tumors, including MM, and have attracted increasing attention (12,13).
Daucosterol is a natural small molecule compound. Relevant studies have reported its anti-tumor effect on liver cancer (14), breast cancer (15), prostate cancer (16), and non-small cell lung cancer (17). For example, it has obvious inhibitory effect on tumor cell proliferation, recurrence, and metastasis processes. The relevant mechanisms that have been studied include regulation of the JNK pathway (18), Wnt/β-catenin pathway (14), cell cycle (19), and oxidative stress (17). However, no previous study has investigated daucosterol in treatment of MM.
In recent years, network pharmacology has provided an efficient research strategy to elucidate the mechanism of action, discover new targets, and reposition new indications from the perspective of biological network balance (20-23). In this study, we preliminarily investigated the potential effect and mechanism of daucosterol against MM using network pharmacology and bioinformatics (Figure 1), laying the foundation for further exploration of its potential clinical application value. We present the following article in accordance with the MDAR reporting checklist (available at https://tcr.amegroups.com/article/view/10.21037/tcr-23-456/rc).
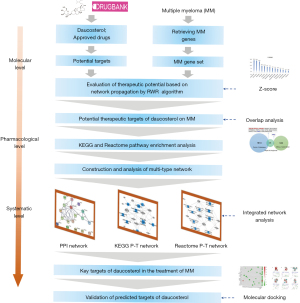
Methods
Acquisition of potential target profiles of daucosterol and the approved drugs for MM
To systematically evaluate the therapeutic effect daucosterol against MM, we searched the DrugBank database (https://go.drugbank.com/) to identify and retrieve the approved small molecules drugs for the treatment of MM as reference. A comprehensive target spectrum of daucosterol and marketed drugs was constructed as the base to explore mechanism of action (24). To improve reliability, the known targets and the putative targets were integrated to obtain the target profiles of the drugs (Table 1). We defined targets from (I) marketed drug databases such as DrugBank (25) and Therapeutic Target Database (TTD) (26), (II) activity assay databases such as the Chemogenic European Molecular Biology Laboratory (ChEMBL) (27) and PubChem (28) as known targets. In addition, targets from (III) literature mining databases such as Search Tool for interactions of CHemicals and proteins (STITCH) (29), and (IV) target prediction tools such as Similarity Ensemble Approach (SEA) (30), TargetNet (31), SwissTargetPrediction (32), ChEMBL_prediction tool (33), and Bioinformatics Analysis Tool for Molecular mechANism of Traditional Chinese Medicine (BATMAN-TCM) (34) were defined as putative targets. All the known targets were kept. Only the putative targets which could be predicted in at least 2 prediction models and validated by literature mining source at the same time were preserved. All of the targets were normalized by the UniProt database (http://www.uniprot.org) (35) and only those belonging to “Homo sapiens” were reserved for further analysis. In this study, we used the known target data as the validation data of the prediction target to verify the accuracy of the prediction method and ensure the reliability of the final compound target spectrum.
Table 1
Target source | Interaction type | Target classification | Reference |
---|---|---|---|
DrugBank | Known interaction | Known target | (25) |
TTD | Known interaction | Known target | (26) |
ChEMBL | Known interaction | Known target | (27) |
PubChem | Known interaction | Known target | (28) |
STITCH | Text mining interaction | Text mining target | (29) |
SEA | Putative interaction | Putative target | (30) |
TargetNet | Putative interaction | Putative target | (31) |
SwissTargetPrediction | Putative interaction | Putative target | (32) |
CHEMBL prediction | Putative interaction | Putative target | (33) |
BATMAN-TCM | Putative interaction | Putative target | (34) |
TTD, Therapeutic Target Database; ChEMBL, Chemogenic European Molecular Biology Laboratory; STITCH, Search Tool for interactions of CHemicals and proteins; SEA, Similarity Ensemble Approach; BATMAN-TCM, Bioinformatics Analysis Tool for Molecular mechANism of Traditional Chinese Medicine.
Construction of specific gene set of MM
We aimed to systematically construct specific molecular network highly related to the pathogenesis of MM. In order to achieve this goal, we integrated the published scattered scientific research results, which were collected from DisGeNET (36), Open Target Platform (37), MalaCards (38), Online Mendelian Inheritance in Man (OMIM) (39), GeneCards (40), and the Comparative Toxicogenomics Database (CTD) (41). To ensure the credibility of MM gene set, we only retained genes that appeared in at least 4 data sources.
In addition, we integrated differentially expressed genes (DEGs) analyzed from GSE125361 by the GEO2R tool in the Gene Expression Omnibus (GEO) database (https://www.ncbi.nlm.nih.gov/geo/) (screening threshold: P<0.05 and |FC| ≥1.2) (42). The gene symbol was standardized based on the Database for Annotation, Visualization, and Integrated Discovery (DAVID) knowledgebase (v2022q2) (https://david.abcc.ncifcrf.gov/) (43).
Evaluation of drug efficacy in view of network propagation
In order to systematically and comprehensively evaluate the efficacy of a daucosterol and marketed drug to MM, we computed the correlation of the drug’s targets and the genes associated with the disease in view of network propagation. First, we took drug targets and disease genes as seed nodes respectively to run random walk with restart (RWR) algorithm (44) in the background network obtained from the Search Tool for the Retrieval of Interacting Genes/Proteins (STRING) database version 11.5 (https://cn.string-db.org/) (45). In this way, we obtained the influence score vector of the 2 seed sets against all nodes in the background network, respectively. Further, the Pearson correlation coefficient of the 2 score vectors (Cor) was calculated and the significance was evaluated the by Z-score. The higher the value, the stronger the correlation between the drug and disease, and significance was generally considered if the value was greater than 3:
where E (Cor) and δ (Cor) were mean and standard deviation of the Pearson correlation coefficients between influence score vector of drug targets and those of 1,000 groups of random contrast disease genes, each of which contained the same number of randomly selected proteins as the disease seed nodes. The RWR algorithm was performed in R 3.5.2 by package dnet (version 1.1.4; R Foundation fr Statistical Computing, Vienna, Austria), and the restart probability was set as the default value 0.75.
Identification of potential therapeutic targets of daucosterol against MM
The targets of daucosterol that overlapped with genes of MM were selected as the candidate targets with therapeutic effects on MM.
Enrichment analysis
For the sake of interpreting the mechanisms of daucosterol against MM from a systematic perspective, clusterProfiler Version 4.0.3, an R Bioconductor package (46) was used to perform Kyoto Encyclopedia of Genes and Genomes (KEGG) and Reactome pathway enrichment analysis (47).
Networks construction
Based on the relationship between the potential target of daucosterol and the gene sets related to MM, we constructed a component-target-disease network to demonstrate the potential mechanism of action of daucosterol in the treatment of MM. Cytoscape v3.7.1 was used to visualized the network (48). To further identify the key targets of daucosterol in the regulation of MM, we constructed a protein-protein interaction (PPI) network through STRING (45). It contains information on more than 14,000 species, more than 60 million proteins, and more than 20 billion interactions. These protein interactions include both direct physical interactions and indirect functional correlations.
To verify that the interactions between them were statistically valid, PPI interaction enrichment tests were conducted. Whole genome was assumed as the statistical background. The hub nodes in the networks were evaluated by the crucial topological parameters, namely degree (49). The NetworkAnalyzer plugin (50) of Cytoscape was used to compute the degree. The degree of a node was the combined number of neighbor nodes (49).
Verification of interaction by molecular docking
The molecular docking method was used to predict the binding affinity between daucosterol and the potential therapeutic targets for the treatment of MM, which could verify the predicted interaction relationship at the molecular level and provide a basis for further experimental verification. AutoDock Vina 1.1.2 (https://vina.scripps.edu/) was used to conduct the docking task (51). The spatial data files (SDFs) of compounds were downloaded from the PubChem database (https://pubchem.ncbi.nlm.nih.gov/) (28) and converted to MOL2 format by Open Babel 2.4.1 (52). We obtained 3-dimensional (3D) structures of the proteins from the Protein Data Bank (PDB) database (https://www.rcsb.org/) (53). The ligands and receptors were prepared according to the tutorial of AutoDock Vina 1.1.2. For each structure, we deleted the water molecules, added non-polar hydrogen, calculated the Gasteiger charge, and saved them as Protein Data Bank, partial charge (Q) and Atom Type (T) (PDBQT) format. The lower the binding energy, the more stable the ligand-receptor binding conformation (threshold: ≤−5.0 kJ/mol). The conformation with the lowest affinity was used as the best docking conformation and protein-ligand interaction profiler (PLIP) was taken to visualize the interaction mode (54).
Results
Target profile of daucosterol and the approved drugs for MM
A total of 13 approved drugs reported to treat MM were retrieved from the DrugBank database (Table 2). Multiple types of target data sources were used to obtain the target spectra of daucosterol and the approved drugs. The detailed targets, data sources, and target types are listed in https://cdn.amegroups.cn/static/public/tcr-23-456-1.xlsx, and the number of potential targets corresponding to each drug is listed in Table 3.
Table 2
# | Compound | CID | CHEMBL ID | Molecular formula | Molecular weight (g/mol) |
---|---|---|---|---|---|
1 | Cyclophosphamide | 2907 | CHEMBL88 | C7H15Cl2N2O2P | 261.08 |
2 | Lidocaine | 3676 | CHEMBL79 | C14H22N2O | 234.34 |
3 | Pamidronic acid | 4674 | CHEMBL834 | C3H11NO7P2 | 235.07 |
4 | Thalidomide | 5426 | CHEMBL468 | C13H10N2O4 | 258.23 |
5 | Dexamethasone | 5743 | CHEMBL384467 | C22H29FO5 | 392.5 |
6 | Vincristine | 5978 | CHEMBL90555 | C46H56N4O10 | 825 |
7 | Doxorubicin | 31703 | CHEMBL53463 | C27H29NO11 | 543.5 |
8 | Etoposide | 36462 | CHEMBL44657 | C29H32O13 | 588.6 |
9 | Zoledronic acid | 68740 | CHEMBL924 | C5H10N2O7P2 | 272.09 |
10 | Pomalidomide | 134780 | CHEMBL43452 | C13H11N3O4 | 273.24 |
11 | Lenalidomide | 216326 | CHEMBL848 | C13H13N3O3 | 259.26 |
12 | Bortezomib | 387447 | CHEMBL325041 | C19H25BN4O4 | 384.2 |
13 | Daucosterol | 5742590 | CHEMBL506678 | C35H60O6 | 576.8 |
14 | Selinexor | 71481097 | CHEMBL3545185 | C17H11F6N7O | 443.3 |
CID, Pubchem_ID.
Table 3
# | Compound | CID | Known target | Putative target | Known & putative target | Total |
---|---|---|---|---|---|---|
1 | Cyclophosphamide | 2907 | 10 | 44 | 0 | 54 |
2 | Lidocaine | 3676 | 13 | 173 | 1 | 187 |
3 | Pamidronic acid | 4674 | 3 | 47 | 2 | 52 |
4 | Thalidomide | 5426 | 16 | 87 | 3 | 106 |
5 | Dexamethasone | 5743 | 20 | 74 | 6 | 100 |
6 | Vincristine | 5978 | 34 | 26 | 8 | 68 |
7 | Doxorubicin | 31703 | 55 | 20 | 11 | 86 |
8 | Etoposide | 36462 | 51 | 16 | 7 | 74 |
9 | Zoledronic acid | 68740 | 8 | 29 | 8 | 45 |
10 | Pomalidomide | 134780 | 2 | 74 | 4 | 80 |
11 | Lenalidomide | 216326 | 7 | 69 | 2 | 78 |
12 | Bortezomib | 387447 | 124 | 13 | 6 | 143 |
13 | Daucosterol | 5742590 | 8 | 27 | 0 | 35 |
14 | Selinexor | 71481097 | 4 | 23 | 0 | 27 |
CID, Pubchem_ID.
Gene set of MM
To make the study more rigorous, we obtained MM-related gene sets in 2 ways. The first was database retrieval. By searching multiple databases and integrating genes from at least 4 data sources, 1,810 pathogenic genes of MM were finally obtained (available online: https://cdn.amegroups.cn/static/public/tcr-23-456-2.xlsx). The second was by analyzing the omics data of MM (GSE125361). We obtained 1,469 DEGs, including 336 down-regulated and 1,133 down-regulated genes (screening threshold: P<0.05 and |FC| ≥1.2) (available online: https://cdn.amegroups.cn/static/public/tcr-23-456-3.xlsx). The gene sets obtained by the above 2 methods were used for subsequent research on the mechanism and targets of daucosterol in the treatment of MM.
Daucosterol showed the potential in the treatment of MM
In this study, we used a network-based approach to comprehensively evaluate the therapeutic potential of daucosterol for MM, using marketed drugs as controls (Figure 2). The results showed that targets profiles of daucosterol and MM-related genes were significantly correlated in the PPI background network, suggesting that daucosterol has the potential to treat MM.
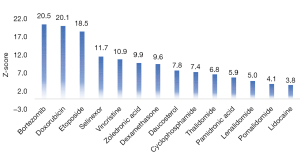
Potential therapeutic targets of daucosterol in the treatment of MM
By intersection analysis of daucosterol target profiles with MM-related gene sets from 2 sources, a total of 18 potential targets were obtained (Figure 3), including 3 known targets (GSK3B, PRKAA1, PRKAB1) and 15 novel predicted targets.
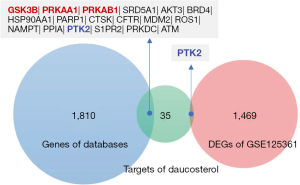
Core signaling pathways potentially regulated by daucosterol in the treatment of MM
To interpret the mechanisms of daucosterol against MM from a systematic perspective, KEGG and Reactome pathway enrichment analysis were conducted (Table 4). The top 5 significantly enriched KEGG pathways were the FoxO signaling pathway (P.adjust=0.0005), prostate cancer (P.adjust=0.0017), PI3K-Akt signaling pathway (P.adjust=0.0005), insulin resistance (P.adjust=0.0017), and the AMPK signaling pathway (P.adjust=0.0017). The top 5 significantly enriched Reactome pathways were AKT phosphorylates targets in the cytosol (P.adjust=0.0003), regulation of TP53 activity (P.adjust=0.0004), constitutive signaling by AKT1 E17K in cancer (P.adjust=0.0006), regulation of TP53 activity through phosphorylation (P.adjust=0.0006), and regulation of TP53 degradation (P.adjust=0.001).
Table 4
ID | Pathway name | P.adjust | Gene symbol |
---|---|---|---|
hsa04068 | FoxO signaling pathway | 0.0005 | PRKAA1/PRKAB1/AKT3/MDM2/ATM |
hsa05215 | Prostate cancer | 0.0017 | GSK3B/AKT3/HSP90AA1/MDM2 |
hsa04151 | PI3K-Akt signaling pathway | 0.0017 | GSK3B/PRKAA1/AKT3/HSP90AA1/MDM2/PTK2 |
hsa04931 | Insulin resistance | 0.0017 | GSK3B/PRKAA1/PRKAB1/AKT3 |
hsa04152 | AMPK signaling pathway | 0.0018 | PRKAA1/PRKAB1/AKT3/CFTR |
hsa05131 | Shigellosis | 0.0018 | GSK3B/AKT3/MDM2/PTK2/ATM |
hsa04110 | Cell cycle | 0.0018 | GSK3B/MDM2/PRKDC/ATM |
hsa04210 | Apoptosis | 0.0018 | AKT3/PARP1/CTSK/ATM |
hsa04910 | Insulin signaling pathway | 0.0018 | GSK3B/PRKAA1/PRKAB1/AKT3 |
R-HSA-198323 | AKT phosphorylates targets in the cytosol | 0.0003 | GSK3B/AKT3/MDM2 |
R-HSA-5633007 | Regulation of TP53 Activity | 0.0004 | PRKAA1/PRKAB1/AKT3/MDM2/ATM |
R-HSA-5674400 | Constitutive signaling by AKT1 E17K in cancer | 0.0006 | GSK3B/AKT3/MDM2 |
R-HSA-6804756 | Regulation of TP53 activity through phosphorylation | 0.0006 | PRKAA1/PRKAB1/MDM2/ATM |
R-HSA-6804757 | Regulation of TP53 degradation | 0.0010 | AKT3/MDM2/ATM |
R-HSA-6806003 | Regulation of TP53 expression and degradation | 0.0010 | AKT3/MDM2/ATM |
R-HSA-3700989 | Transcriptional regulation by TP53 | 0.0059 | PRKAA1/PRKAB1/AKT3/MDM2/ATM |
R-HSA-5628897 | TP53 regulates metabolic genes | 0.0086 | PRKAA1/PRKAB1/AKT3 |
R-HSA-3371556 | Cellular response to heat stress | 0.0086 | GSK3B/HSP90AA1/ATM |
KEGG, Kyoto Encyclopedia of Genes and Genomes.
Identification of key targets of daucosterol in the treatment of MM
A PPI network of 18 potential functional targets of daucosterol for MM treatment was constructed based on the STRING database (Figure 4), and the network parameters are listed in Table 5. The PPI network consisted of 18 nodes and 32 edges. The average node degree and the average local clustering coefficient were 3.56 and 0.606, respectively. PPI interaction enrichment result indicated that this PPI sub-network has significantly more interactions than what would be expected for a random set of proteins of similar size, drawn from the genome (P=1.07e-07). This means that these proteins are closely connected biologically as a group. The degree of nodes in the network was calculated (Table 6).
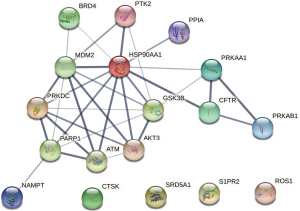
Table 5
Network statistical parameters | Value |
---|---|
Number of nodes | 18 |
Number of edges | 32 |
Average node degree | 3.56 |
Avg. local clustering coefficient | 0.606 |
Expected number of edges | 11 |
PPI enrichment P value | 1.07e-07 |
PPI, protein-protein interaction; Avg., average.
Table 6
Gene symbol | Degree in PPI network | Degree in KEGG P-T network | Degree in Reactome P-T network |
---|---|---|---|
HSP90AA1 | 11 | 2 | 1 |
MDM2 | 8 | 5 | 7 |
GSK3B* | 7 | 6 | 3 |
PARP1 | 6 | 1 | 0 |
PRKDC | 6 | 1 | 0 |
AKT3 | 5 | 8 | 7 |
ATM | 5 | 4 | 6 |
PRKAA1* | 4 | 5 | 4 |
CFTR | 3 | 1 | 0 |
PTK2 | 3 | 2 | 0 |
BRD4 | 2 | 0 | 0 |
PRKAB1* | 2 | 4 | 4 |
NAMPT | 1 | 0 | 0 |
PPIA | 1 | 0 | 0 |
CTSK | 0 | 1 | 0 |
ROS1 | 0 | 0 | 0 |
S1PR2 | 0 | 0 | 0 |
SRD5A1 | 0 | 0 | 0 |
*, the known targets. PPI, protein-protein interaction; P-T, pathway-target; KEGG, Kyoto Encyclopedia of Genes and Genomes.
In order to accurately locate the core targets of daucosterol in the treatment of MM, we integrated the results of pathway enrichment analysis and selected the top 5 signaling pathways to construct pathway-target network (P-T network) (Figure 5). It is generally believed that targets involved in multiple signaling pathways play an important role in cross-talk between different signaling pathways.
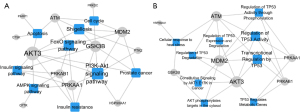
By integrating PPI network and P-T network data, it is very easy to select the core targets of daucosterol in the treatment of MM, which occupy an important network topological position in the PPI network and play the role of cross-talk in multiple signaling pathways, including HSP90AA1, MDM2, GSK3B, AKT3, PRKAA1, and PRKAB1 (Table 6).
Daucosterol might have potential direct regulatory effects on target proteins closely related to MM
Molecular docking was conducted to verify the predicted interaction relationship between daucosterol and the potential therapeutic targets. If the binding free energy is lower than the threshold (≤−5.0 kJ/mol), it indicates that the compound has the potential of forming stable binding conformation with the target protein (Figure 6). The binding free energy between daucosterol and 13 of the 18 targets was lower than the threshold, indicating that they could easily form stable binding conformations. Among them, the binding free energy of 6 targets with daucosterol was lower than that with co-crystallized molecules, including BRD4 (affinity =−10 kcal/mol), GSK3B (affinity =−7.9 kcal/mol), GSK3B (affinity =−7.9 kcal/mol), CTSK (affinity =−7.4 kcal/mol), PRKAA1 (affinity =−7.3 kcal/mol), MDM2 (affinity =−6.5 kcal/mol), and PPIA (affinity =−6.3 kcal/mol). These results demonstrated the reliability of daucosterol’s potential target profile and its therapeutic potential with MM.
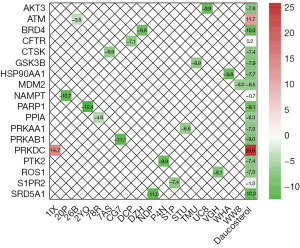
Interaction patterns between daucosterol and the 6 targets with the lowest binding free energy were visualized (Figure 7). The interaction modes with the other 7 targets can be seen in Figure S1. The results showed that daucosterol could successfully dock with the active pocket of the core targets in the treatment of MM, revealing a stable docking model with a specific binding site, binding distance, and binding atom. For example, the SRD5A1-daucosterol complex was stabilized at amino acid residue GLU-54, ARG-90, and THR-219 by 3 hydrogen bonds. Daucosterol formed 5 hydrogen bonds with PRO-95, ASP-96, TYR-139, ASN-140, and ASP-144 in BRD4. PARP1 and daucosterol were bound at TYR-1224 by 2 hydrogen bonds. The PRKAB1-daucosterol complex was stabilized by 3 hydrogen bonds at amino acid residue LYS-29, ASN-48, and ARG-83. Daucosterol formed 1 hydrogen bond with SER-203 in GSK3B. HSP90AA1 and daucosterol were bound at ASN-51, GLY-135, and TYR-139 by 3 hydrogen bonds. The sugar ring structure in daucosterol plays a key role in the formation of hydrogen bonds.
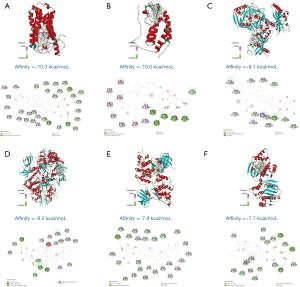
Discussion
Relevant studies have shown that daucosterol has anti-tumor potential, but no studies related to MM have been reported. Therefore, in this study, the therapeutic potential and the mechanism of action of daucosterol were evaluated using network pharmacology and molecular docking methods.
To systematically evaluate the therapeutic potential of daucosterol for MM, a network-based RWR algorithm was applied. It adapted a global distance measure to define similarity between genes within this interaction network, which is better suited to capture relationships between drug targets and disease proteins than overlapping genes, direct interactions, or shortest paths between them (44). This step ensures that subsequent analysis is meaningful. It is highly necessary for network pharmacological analysis. In addition, this study innovatively took marketed drugs as the control. The results showed that 13 reported drugs showed significant correlations with MM, which validated the reliability of the method. Among them, bortezomib, a PI with the highest correlation (Z-score =20.5) with MM, is the current first-line treatment drug for MM, which again proves the reliability of this analysis method. The number of candidate targets for drugs varies, which may affect the ranking. Daucosterol and bortezomib, for example, have 35 and 143 candidate targets, respectively. The imbalance of target number may be the main reason for the low ranking of daucosterol, but it does not affect its significant correlation with MM gene.
Pathway enrichment analysis allows us to provide some reference for exploring the mechanism of disease occurrence and the mechanism of drug action from a systematic perspective. KEGG and Reactome pathway analysis showed that pharmacological effect of daucosterol against MM may be mainly through regulating of the FoxO signaling pathway, prostate cancer, PI3K-Akt signaling pathway, insulin resistance, AMPK signaling pathway, and pathways related to the regulation of TP53. A multitude of related studies have shown that these pathways are closely related to the occurrence and development of MM (55-60). At present, the mechanism of daucosterol to treat tumors mainly involves the JNK pathway (18), Wnt/β-catenin pathway (14), cell cycle (19), oxidative stress (17), and so on. The high-quality data in this study lay the foundation and highlight a new direction for the follow-up mechanism research of daucosterol in the treatment of MM.
Through integrated analysis of multiple types of networks, 6 core targets of daucosterol in the treatment of MM were identified, including HSP90AA1, MDM2, GSK3B, AKT3, PRKAA1, and PRKAB1. These targets are at the core position of the PPI network and play an important cross-talking role in multiple signaling pathways. Their intervention can maximize the impact of MM pathophysiology. Research has clearly demonstrated that HSPs might be novel tumor antigens for immunotherapy of myeloma (61). MDM2 is elevated in MM and is key to MM growth and survival (62). Targeting an MDM2/MYC axis could overcome drug resistance in MM (63). GSK3βcould activate the βcatenin pathway in MM cells and promotes the malignancy of MM (64,65). AKT3 overexpression is closely related to MM progression (66). PRKAB1 participates in the AMPK pathway, and the activation of AMPK pathway is associated with survival of MM cells (67). PRKAA1 is involved in PI3K-Akt signaling pathway, and the activated PI3K-AKT pathway could accelerate cellular proliferation of MM (68). Studies have shown that daucosterol could significantly increase the levels of GSK3B, PRKAA1, and PRKAB1, and promote the inducting of GSK3-beta phosphorylation and AMPK phosphorylation respectively (69). GSK3B plays a key role in the Wnt/β-catenin pathway. Our previous research showed that daucosterol could inhibit cell migration and invasion in hepatocellular carcinoma cells (HCC) cells via the Wnt/β-catenin signaling pathway (14). Therefore, it is necessary to conduct similar studies in MM-related cell or animal models in subsequent studies to explore the mechanism of daucosterol in the treatment of MM.
The reliability of the interaction relationships between 18 predicted anti-MM targets of daucosterol was verified by molecular docking, and the results showed that daucosterol could form stable binding conformations with 13 of them (affinity <−5 kcal/mol). This indicates that the strategy used in this study to obtain the target profiles of compounds is very reliable and can be used by other researchers to obtain the potential target profiles of compounds of interest. The complex formed between daucosterol and BRD4, GSK3B, GSK3B, CTSK, PRKAA1, MDM2, and PPIA has a lower binding free energy than the complex formed by the molecules crystallized together with these targets. These results suggest that the binding stability of daucosterol in these targets is better than that of co-crystallized molecules, and it may have better activity than them. The effect of daucosterol on the activity of these targets is worthy of further study. Analysis of the interaction modes between daucosterol and 13 candidate targets suggested that the sugar ring structure in daucosterol was very important and involved in the formation of multiple hydrogen bonds stabilizing conformation with targets. This is an important reminder for the subsequent research on drug development and design modification.
Conclusions
In this study, the therapeutic potential and mechanism of action of daucosterol in the treatment of MM were investigated by network pharmacological and molecular docking technology. On the basis of obtaining high quality therapeutic target profiles of daucosterol and MM gene sets, the therapeutic potential of daucosterol against MM was systematically evaluated through a network-based approach. The pharmacological effect of daucosterol against MM may be mainly through regulation of the FoxO signaling pathway, prostate cancer, PI3K-Akt signaling pathway, insulin resistance, AMPK signaling pathway, and pathways related to the regulation of TP53. Key targets involved in multiple pathways include HSP90AA1, MDM2, GSK3B, AKT3, PRKAA1, and PRKAB1. This study reflects the characteristics of pharmacological effects of natural products with “multiple targets and multiple pathways”, which lays a foundation for the subsequent in-depth study of daucosterol in the treatment of MM and provides a basis for its application in the clinical treatment of MM.
Acknowledgments
We also wish to extend our thanks to all who assisted in the conduction of this study.
Funding: This research was supported by the
Footnote
Reporting Checklist: The authors have completed the MDAR reporting checklist. Available at https://tcr.amegroups.com/article/view/10.21037/tcr-23-456/rc
Peer Review File: Available at https://tcr.amegroups.com/article/view/10.21037/tcr-23-456/prf
Conflicts of Interest: All authors have completed the ICMJE uniform disclosure form available at https://tcr.amegroups.com/article/view/10.21037/tcr-23-456/coif). The authors have no conflicts of interest to declare.
Ethical Statement: The authors are accountable for all aspects of the work in ensuring that questions related to the accuracy or integrity of any part of the work are appropriately investigated and resolved.
Open Access Statement: This is an Open Access article distributed in accordance with the Creative Commons Attribution-NonCommercial-NoDerivs 4.0 International License (CC BY-NC-ND 4.0), which permits the non-commercial replication and distribution of the article with the strict proviso that no changes or edits are made and the original work is properly cited (including links to both the formal publication through the relevant DOI and the license). See: https://creativecommons.org/licenses/by-nc-nd/4.0/.
References
- Cowan AJ, Green DJ, Kwok M, et al. Diagnosis and Management of Multiple Myeloma: A Review. JAMA 2022;327:464-77. [Crossref] [PubMed]
- van de Donk NWCJ, Pawlyn C, Yong KL. Multiple myeloma. Lancet 2021;397:410-27. [Crossref] [PubMed]
- Turesson I, Bjorkholm M, Blimark CH, et al. Rapidly changing myeloma epidemiology in the general population: Increased incidence, older patients, and longer survival. Eur J Haematol 2018; Epub ahead of print. [Crossref] [PubMed]
- Dehghanifard A, Kaviani S, Abroun S, et al. Various Signaling Pathways in Multiple Myeloma Cells and Effects of Treatment on These Pathways. Clin Lymphoma Myeloma Leuk 2018;18:311-20. [Crossref] [PubMed]
- Voorhees PM, Kaufman JL, Laubach J, et al. Daratumumab, lenalidomide, bortezomib, and dexamethasone for transplant-eligible newly diagnosed multiple myeloma: the GRIFFIN trial. Blood 2020;136:936-45. [Crossref] [PubMed]
- Mikkilineni L, Kochenderfer JN. CAR T cell therapies for patients with multiple myeloma. Nat Rev Clin Oncol 2021;18:71-84. [Crossref] [PubMed]
- Holstein SA. Current Frontline Treatment of Multiple Myeloma. Oncology (Williston Park) 2022;36:430-41. [PubMed]
- Delmore JE, Issa GC, Lemieux ME, et al. BET bromodomain inhibition as a therapeutic strategy to target c-Myc. Cell 2011;146:904-17. [Crossref] [PubMed]
- Evan G, Harrington E, Fanidi A, et al. Integrated control of cell proliferation and cell death by the c-myc oncogene. Philos Trans R Soc Lond B Biol Sci 1994;345:269-75. [Crossref] [PubMed]
- Neumeister P, Schulz E, Pansy K, et al. Targeting the Microenvironment for Treating Multiple Myeloma. Int J Mol Sci 2022;23:7627. [Crossref] [PubMed]
- Davis LN, Sherbenou DW. Emerging Therapeutic Strategies to Overcome Drug Resistance in Multiple Myeloma. Cancers (Basel) 2021;13:1686. [Crossref] [PubMed]
- Capalbo A, Lauritano C. Multiple Myeloma: Possible Cure from the Sea. Cancers (Basel) 2022;14:2965. [Crossref] [PubMed]
- Das S, Juliana N, Yazit NAA, et al. Multiple Myeloma: Challenges Encountered and Future Options for Better Treatment. Int J Mol Sci 2022;23:1649. [Crossref] [PubMed]
- Zeng J, Liu X, Li X, et al. Daucosterol Inhibits the Proliferation, Migration, and Invasion of Hepatocellular Carcinoma Cells via Wnt/β-Catenin Signaling. Molecules 2017;22:862. [Crossref] [PubMed]
- Nguedia MY, Tueche AB, Yaya AJG, et al. Daucosterol from Crateva adansonii DC (Capparaceae) reduces 7,12-dimethylbenz(a)anthracene-induced mammary tumors in Wistar rats. Environ Toxicol 2020;35:1125-36. [Crossref] [PubMed]
- Zingue S, Gbaweng Yaya AJ, Michel T, et al. Bioguided identification of daucosterol, a compound that contributes to the cytotoxicity effects of Crateva adansonii DC (capparaceae) to prostate cancer cells. J Ethnopharmacol 2020;247:112251. [Crossref] [PubMed]
- Rajavel T, Banu Priya G, Suryanarayanan V, et al. Daucosterol disturbs redox homeostasis and elicits oxidative-stress mediated apoptosis in A549 cells via targeting thioredoxin reductase by a p53 dependent mechanism. Eur J Pharmacol 2019;855:112-23. [Crossref] [PubMed]
- Gao P, Huang X, Liao T, et al. Daucosterol induces autophagic-dependent apoptosis in prostate cancer via JNK activation. Biosci Trends 2019;13:160-7. [Crossref] [PubMed]
- Rajavel T, Mohankumar R, Archunan G, et al. Beta sitosterol and Daucosterol (phytosterols identified in Grewia tiliaefolia) perturbs cell cycle and induces apoptotic cell death in A549 cells. Sci Rep 2017;7:3418. [Crossref] [PubMed]
- Hopkins AL. Network pharmacology: the next paradigm in drug discovery. Nat Chem Biol 2008;4:682-90. [Crossref] [PubMed]
- Li S, Zhang B. Traditional Chinese medicine network pharmacology: theory, methodology and application. Chin J Nat Med 2013;11:110-20. [Crossref] [PubMed]
- Zheng Y, Liu Z, Cai A, et al. Study on the mechanism of Ginseng-Gegen for mesenteric lymphadenitis based on network pharmacology. Transl Pediatr 2022;11:1534-43. [Crossref] [PubMed]
- Song T, Wang G, Ding M, et al. Network-Based Approaches for Drug Repositioning. Mol Inform 2022;41:e2100200. [Crossref] [PubMed]
- Rix U, Superti-Furga G. Target profiling of small molecules by chemical proteomics. Nat Chem Biol 2009;5:616-24. [Crossref] [PubMed]
- Wishart DS, Feunang YD, Guo AC, et al. DrugBank 5.0: a major update to the DrugBank database for 2018. Nucleic Acids Res 2018;46:D1074-82. [Crossref] [PubMed]
- Wang Y, Zhang S, Li F, et al. Therapeutic target database 2020: enriched resource for facilitating research and early development of targeted therapeutics. Nucleic Acids Res 2020;48:D1031-41. [PubMed]
- Gaulton A, Hersey A, Nowotka M, et al. The ChEMBL database in 2017. Nucleic Acids Res 2017;45:D945-54. [Crossref] [PubMed]
- Kim S, Thiessen PA, Bolton EE, et al. PubChem Substance and Compound databases. Nucleic Acids Res 2016;44:D1202-13. [Crossref] [PubMed]
- Szklarczyk D, Santos A, von Mering C, et al. STITCH 5: augmenting protein-chemical interaction networks with tissue and affinity data. Nucleic Acids Res 2016;44:D380-4. [Crossref] [PubMed]
- Keiser MJ, Roth BL, Armbruster BN, et al. Relating protein pharmacology by ligand chemistry. Nat Biotechnol 2007;25:197-206. [Crossref] [PubMed]
- Yao ZJ, Dong J, Che YJ, et al. TargetNet: a web service for predicting potential drug-target interaction profiling via multi-target SAR models. J Comput Aided Mol Des 2016;30:413-24. [Crossref] [PubMed]
- Gfeller D, Grosdidier A, Wirth M, et al. SwissTargetPrediction: a web server for target prediction of bioactive small molecules. Nucleic Acids Res 2014;42:W32-8. [Crossref] [PubMed]
- Bosc N, Atkinson F, Felix E, et al. Large scale comparison of QSAR and conformal prediction methods and their applications in drug discovery. J Cheminform 2019;11:4. [Crossref] [PubMed]
- Liu Z, Guo F, Wang Y, et al. BATMAN-TCM: a Bioinformatics Analysis Tool for Molecular mechANism of Traditional Chinese Medicine. Sci Rep 2016;6:21146. [Crossref] [PubMed]
- UniProt: a worldwide hub of protein knowledge. Nucleic Acids Res 2019;47:D506-15. [Crossref] [PubMed]
- Piñero J, Ramírez-Anguita JM, Saüch-Pitarch J, et al. The DisGeNET knowledge platform for disease genomics: 2019 update. Nucleic Acids Res 2020;48:D845-55. [PubMed]
- Carvalho-Silva D, Pierleoni A, Pignatelli M, et al. Open Targets Platform: new developments and updates two years on. Nucleic Acids Res 2019;47:D1056-65. [Crossref] [PubMed]
- Rappaport N, Twik M, Plaschkes I, et al. MalaCards: an amalgamated human disease compendium with diverse clinical and genetic annotation and structured search. Nucleic Acids Res 2017;45:D877-87. [Crossref] [PubMed]
- Amberger JS, Bocchini CA, Scott AF, et al. OMIM.org: leveraging knowledge across phenotype-gene relationships. Nucleic Acids Res 2019;47:D1038-43. [Crossref] [PubMed]
- Safran M, Dalah I, Alexander J, et al. GeneCards Version 3: the human gene integrator. Database (Oxford) 2010;2010:baq020. [Crossref] [PubMed]
- Davis AP, Grondin CJ, Johnson RJ, et al. The Comparative Toxicogenomics Database: update 2019. Nucleic Acids Res 2019;47:D948-54. [Crossref] [PubMed]
- Farswan A, Gupta A, Gupta R, et al. Imputation of Gene Expression Data in Blood Cancer and Its Significance in Inferring Biological Pathways. Front Oncol 2020;9:1442. [Crossref] [PubMed]
- Dennis G Jr, Sherman BT, Hosack DA, et al. DAVID: Database for Annotation, Visualization, and Integrated Discovery. Genome Biol 2003;4:3. [Crossref] [PubMed]
- Köhler S, Bauer S, Horn D, et al. Walking the interactome for prioritization of candidate disease genes. Am J Hum Genet 2008;82:949-58. [Crossref] [PubMed]
- Szklarczyk D, Morris JH, Cook H, et al. The STRING database in 2017: quality-controlled protein-protein association networks, made broadly accessible. Nucleic Acids Res 2017;45:D362-8. [Crossref] [PubMed]
- Yu G, Wang LG, Han Y, et al. clusterProfiler: an R package for comparing biological themes among gene clusters. OMICS 2012;16:284-7. [Crossref] [PubMed]
- Kanehisa M, Furumichi M, Tanabe M, et al. KEGG: new perspectives on genomes, pathways, diseases and drugs. Nucleic Acids Res 2017;45:D353-61. [Crossref] [PubMed]
- Shannon P, Markiel A, Ozier O, et al. Cytoscape: a software environment for integrated models of biomolecular interaction networks. Genome Res 2003;13:2498-504. [Crossref] [PubMed]
- Karbalaei R, Allahyari M, Rezaei-Tavirani M, et al. Protein-protein interaction analysis of Alzheimer`s disease and NAFLD based on systems biology methods unhide common ancestor pathways. Gastroenterol Hepatol Bed Bench 2018;11:27-33. [PubMed]
- Assenov Y, Ramírez F, Schelhorn SE, et al. Computing topological parameters of biological networks. Bioinformatics 2008;24:282-4. [Crossref] [PubMed]
- Trott O, Olson AJ. AutoDock Vina: improving the speed and accuracy of docking with a new scoring function, efficient optimization, and multithreading. J Comput Chem 2010;31:455-61. [PubMed]
- O'Boyle NM, Banck M, James CA, et al. Open Babel: An open chemical toolbox. J Cheminform 2011;3:33. [Crossref] [PubMed]
- Karuppasamy MP, Venkateswaran S, Subbiah P. PDB-2-PBv3.0: An updated protein block database. J Bioinform Comput Biol 2020;18:2050009. [Crossref] [PubMed]
- Salentin S, Schreiber S, Haupt VJ, et al. PLIP: fully automated protein-ligand interaction profiler. Nucleic Acids Res 2015;43:W443-7. [Crossref] [PubMed]
- Bloedjes TA, de Wilde G, Maas C, et al. AKT signaling restrains tumor suppressive functions of FOXO transcription factors and GSK3 kinase in multiple myeloma. Blood Adv 2020;4:4151-64. [Crossref] [PubMed]
- Heinemann L, Möllers KM, Ahmed HMM, et al. Inhibiting PI3K-AKT-mTOR Signaling in Multiple Myeloma-Associated Mesenchymal Stem Cells Impedes the Proliferation of Multiple Myeloma Cells. Front Oncol 2022;12:874325. [Crossref] [PubMed]
- Ragbourne SC, Maghsoodi N, Streetly M, et al. The Association between Metabolic Syndrome and Multiple Myeloma. Acta Haematol 2021;144:24-33. [Crossref] [PubMed]
- Medina EA, Oberheu K, Polusani SR, et al. PKA/AMPK signaling in relation to adiponectin's antiproliferative effect on multiple myeloma cells. Leukemia 2014;28:2080-9. [Crossref] [PubMed]
- Rojas EA, Corchete LA, De Ramón C, et al. Expression of p53 protein isoforms predicts survival in patients with multiple myeloma. Am J Hematol 2022;97:700-10. [Crossref] [PubMed]
- Feng X, Guo J, An G, et al. Genetic Aberrations and Interaction of NEK2 and TP53 Accelerate Aggressiveness of Multiple Myeloma. Adv Sci (Weinh) 2022;9:e2104491. [Crossref] [PubMed]
- Li R, Qian J, Zhang W, et al. Human heat shock protein-specific cytotoxic T lymphocytes display potent antitumour immunity in multiple myeloma. Br J Haematol 2014;166:690-701. [Crossref] [PubMed]
- Manier S, Huynh D, Shen YJ, et al. Inhibiting the oncogenic translation program is an effective therapeutic strategy in multiple myeloma. Sci Transl Med 2017;9:eaal2668. [Crossref] [PubMed]
- Faruq O, Zhao D, Shrestha M, et al. Targeting an MDM2/MYC Axis to Overcome Drug Resistance in Multiple Myeloma. Cancers (Basel) 2022;14:1592. [Crossref] [PubMed]
- Lv M, Liu Q. JMJD2C triggers the growth of multiple myeloma cells via activation of β catenin. Oncol Rep 2021;45:1162-70. [Crossref] [PubMed]
- Zhou Y, Uddin S, Zimmerman T, et al. Growth control of multiple myeloma cells through inhibition of glycogen synthase kinase-3. Leuk Lymphoma 2008;49:1945-53. [Crossref] [PubMed]
- Jiang F, Tang X, Tang C, et al. HNRNPA2B1 promotes multiple myeloma progression by increasing AKT3 expression via m6A-dependent stabilization of ILF3 mRNA. J Hematol Oncol 2021;14:54. [Crossref] [PubMed]
- De Veirman K, Menu E, Maes K, et al. Myeloid-derived suppressor cells induce multiple myeloma cell survival by activating the AMPK pathway. Cancer Lett 2019;442:233-41. [Crossref] [PubMed]
- Zhang Y, Deng Z, Sun S, et al. NAT10 acetylates BCL-XL mRNA to promote the proliferation of multiple myeloma cells through PI3K-AKT pathway. Front Oncol 2022;12:967811. [Crossref] [PubMed]
- Ha do T. Palbinone and triterpenes from Moutan Cortex (Paeonia suffruticosa, Paeoniaceae) stimulate glucose uptake and glycogen synthesis via activation of AMPK in insulin-resistant human HepG2 Cells. Bioorg Med Chem Lett 2009;19:5556-9. [Crossref] [PubMed]
(English Language Editor: J. Jones)