Ferula sinkiangensis against gastric cancer: a network pharmacology, molecular docking and cell experiment study
Highlight box
Key findings
• This study showed that ethyl acetate extract of F. sinkiangensis weakened the proliferation, migration, and invasion of human gastric cancer SGC7901 cells, and systematically explained the potential mechanism of F. sinkiangensis in treating GC using network pharmacology.
What is known and what is new?
• Research on the anticancer inhibitor effects of Ferula has gained significant momentum.
• This study systematically explained the potential mechanism of F. sinkiangensis in treating GC using network pharmacology, molecular docking, and bioinformatics analysis technology for the first time.
What is the implication, and what should change now?
• In this study, the results are only predictions after all. And for the next step, we intend to use F. sinkiangensis to treat GC in both in vivo and in vitro experimental models, in order to analyze the express level of protein and the mRNA level by transcriptomics and proteomics studies.
Introduction
Gastric cancer (GC) is a malignant tumor threatening human health globally. According to the most recent statistics from the journal CA: A Cancer Journal for Clinicians, more than 1 million new cases of GC are reported every year. GC reportedly kills about 760,000 people annually and is the fourth most common types of cancer (1). GC morbidity and mortality rates in China are among the highest globally, accounting for roughly 40% of global GC cases (2). Notwithstanding that the past decade had witnessed significant inroads in surgical treatment, adjuvant radiotherapy, chemotherapy, and targeted therapy for GC patients, the 5-year survival rate for advanced GC has not improved significantly, reported to a range between 30% and 50% (3).
Ferula is a member of the Peucedanum family of Umbelliferae, with over 150 species found primarily in Central Asia, including Iran, Pakistan, Turkey, and the former Soviet Union. In China, 31 species are grown, with 25 species in the Xinjiang province. Ferula was first reported in the Newly Revised Materia Medica of the Tang Dynasty in China. According to the 2020 edition of the Chinese Pharmacopoeia, the resin of Ferula sinkiangensis (F. sinkiangensis) or Ferula fukanensis has the effect of eliminating food, removing blood stasis, dispersing a lump in the abdomen, and killing insects. An ancient saying goes as follows, “there is no fake in gold, and there is no truth in Ferula”, implying that Ferula is difficult to come by and is regarded as a precious resource known as the “Gobi Treasure” and “National Treasure of Western Regions”.
F. sinkiangensis is a traditional Chinese medicine used in China for thousands of years to treat stomach ailments. It has been documented in the Materia Medica of all dynasties. According to the Compendium of Materia Medica, “Ferula can eliminate meat accumulation and kill insects; therefore, it can detoxify and ward off evil spirits”. F. sinkiangensis is classified as a dietary supplement or an insecticide. Xinjiang people have the habit of eating freshly grown leaves of F. sinkiangensis, which is believed to have the effects of invigorating the stomach, expelling parasites, and eliminating abdominal distension and abdominal pain. Over the past five years, research on the anticancer, anti-angiogenesis and P-glycoprotein inhibitor effects of Ferula has gained significant momentum, especially in Gharaei et al. reported that Ferula gummosa could inhibit proliferation and induce apoptosis on GC cell lines (4). Zhang et al. of the Chinese Academy of Medical Sciences reported that F. sinkiangensis could induce apoptosis and G0/G1 cycle arrest of GC cells, mediated by the Wnt signaling pathway (5,6). Over the years, our research group has published 21 papers on the antitumor and antioxidation properties of F. sinkiangensis since 2011 (7-10).
Network pharmacology is a new interdisciplinary field that uses a systematic network model to analyze the interaction between “traditional Chinese medicine compounds-disease-targets-pathways” and reveals the functioning mechanisms behind for drugs at the molecular level. It is now a comprehensive and effective method for conducting research on the pharmacological mechanisms of traditional Chinese medicine. At the moment, network pharmacology is constrained by various aspects of technology, such as limited information from the database, inability to accurately reflect the status of the patients, etc. However, as more researchers pay attention to network pharmacology, the research of its related disciplines is also deepening, with growing number of data related to diseases and drugs, and the continuous improvement of computer technology and calculation and analysis software. Network pharmacology is anticipated to become more prevalent in the pharmacy industry in the coming years.
Herein, network pharmacology was used to predict the mechanism of F. sinkiangensis' antitumor activity on the biological, molecular level, and the findings were validated using molecular docking technology and bioinformatics analysis technology to provide the foothold for future studies for the development of pharmacodynamic materials based on its active compounds. We present the following article in accordance with the MDAR reporting checklist (available at https://tcr.amegroups.com/article/view/10.21037/tcr-22-2292/rc).
Methods
The study was conducted in accordance with the Declaration of Helsinki (as revised in 2013).
Active compounds and target genes prediction of F. sinkiangensis
The main chemical compounds of F. sinkiangensis were determined in a previous chemical compound separation experiment of F. sinkiangensis conducted by our research group and a literature review (11-18). The Simplified Molecular Input Line Entry System (SMILES) of chemical compounds was obtained from the PubChem database, and the active compounds of F. sinkiangensis were screened by the SwissADME database (19). Metabolites with high gastrointestinal absorption and at least two “YES” for Druglikeness indexes Lipinski, Ghose, Veber, Egan, and Muegge were selected in SwissADME. The potential target genes of each active compound were predicted with the help of the PharmMapper platform, and the target genes were screened with “NormFit” greater than 0.6 (20). The screened target genes were introduced into the Uniprot database to obtain the official gene name, and the predictive target genes of active compounds could be obtained after removing repetitive genes (21).
Collection of GC-related target genes
The relevant target genes were retrieved from the GeneCards database using the keyword “Gastric Cancer” and standardized by the Uniprot database (22). The genes obtained were GC’s predictive target genes after excluding repetitive and false positive genes. If too many GC target genes are detected, those with a “Relevance Score” greater than 2 times the median were set as potential GC target genes.
Drug-Compound-Target-Disease (D-C-T-D) network construction
A Venn plot was generated to obtain the intersection of the predicted drug-related and disease-related target genes. Next, complex information networks were constructed based on the interactions of drugs (F. sinkiangensis), active compounds, intersected target genes, and disease (GC). Finally, Cytoscape 3.7.2 software was used to visualize and analyze the D-C-T-D network (23). The first five active compounds of degree were selected as the core active compounds.
Protein-protein interaction (PPI) network construction
The STRING online database was used to obtain PPI data of the previously overlapping targets in the network (24). The object was selected as “Homo sapiens,” and confidence scores were greater than 0.900. An R package was used to screen the key targets with the top 30 degree values for visualization.
Gene Ontology (GO) and Kyoto Encyclopedia of Genes and Genomes (KEGG) pathway enrichment analyses
GO enrichment and KEGG pathway enrichment of the targets of F. sinkiangensis in the treatment of GC were analyzed using the R package clusterProfiler. After the screening, significantly enriched GO terms and KEGG signaling pathways with P<0.05 were chosen.
Expression of the core genes in stomach adenocarcinoma (STAD) and paracancerous gastric tissues
mRNA expression of core genes in STAD and paracancerous gastric tissues
STAD is a malignant neoplasm in the glandular epithelium of the stomach. The incidence of STAD accounts for 95% of malignant gastric tumors. According to KEGG enrichment pathway analyses, the mechanism of F. sinkiangensis in treating GC is related to the glycolysis/gluconeogenesis, Pentose phosphate pathway and so on. Using GEPIA database, we analyzed the mRNA expression of seven core genes of PPI network related to energy metabolism in tumor cells (25). The target gene was entered, and the core target gene mRNA expression was obtained by clicking GEPIA after selecting the cancer species to be studied as STAD. The mRNA expression of the core target genes was analyzed using the UALCAN database (26). After the selection of the Cancer Genome Atlas (TCGA) module, the entering of the name of the core target gene, where “stomach adenocarcinoma” is selected, the mRNA expression data of the target gene in STAD could be obtained.
Immunohistochemical analysis of the core target genes in STAD and paracancerous gastric tissues
The Human Protein Atlas (HPA) database was retrieved for all core target genes whose mRNA were highly expressed in STAD (27). To obtain immunohistochemical results of the expression of the above target genes in stomach cancer tissues, “Stomach Cancer” during pathological analysis was chosen. “Stomach” was selected in the tissue option to obtain the immunohistochemical results of the above target genes in normal gastric tissue. ImageProPlus 6.0 was used to calculate the average optical density [AOD = integral optical density (IOD) sum/area sum]. The AOD of each pixel in the projected or cross-sectional image of the tested cell was referred to as AOD (28). In this study, AOD referred to the intracellular concentration of the measured protein and the staining depth.
Survival analysis of highly expressed core target genes in GC
KM plotter database includes 1,065 GC samples with follow-up data. The median overall survival (OS) was 28.9 months, and the median progression-free survival (PFS) was 18.3 months (29). The associations of the core genes verified by GEPIA, UALCAN, and HPA with survival rates of GC patients were explored by KM Plotter; the core genes for which higher expression indicated significantly worse survival were selected as potential immunotherapy biomarkers for GC. A P value <0.05 was statistically significant.
Molecular docking simulation
By docking the active compounds with the targets, the network pharmacology screening results were validated using the molecular docking software AutoDock 1.1.2 (Scripps Research, San Diego, CA, USA). Via computer simulations, small-molecule ligands were placed on the binding region of large-molecule receptors, and the physical and chemical parameters were calculated to predict the binding affinity. The gene crystal complexes of five PPI network core targets (ERBB2, GAPDH, GPI, TKT, GLYCTK) were downloaded in the PDB format from the RCSB PDB database, and PyMOL software (DeLano Scientific LLC, San Carlos, CA, USA) was used to remove water molecules and ligands (30). Through the use of the Chem3D software (Cambridgesoft Corporation, Cambridge, MA, USA), the sdf file of the core active compounds obtained from the PubChem database was converted into a mol 2 file with the lowest free energy. Finally, Ligands and receptors were processed with AutoDock Tools 1.5.6 and used AutoDock Vina 1.1.2 for molecular docking and analysis of docking results (31). PyMOL was used to visualize the results, and the hydrogen bonds and their binding sites were examined. The docking energy value was computed using the consistency score function of the ligand-receptor affinity (32). The affinity between the active compound and the related target was assessed by measuring the active compound's binding free energy to the target. The stronger the affinity, the lower the binding free energy required for docking.
Cell culture
SGC7901 cell lines (Shanghai Qingqi Biological Co., Ltd., China) were cultured in RPMI-1640 (Hyclone, Logan, UT, USA) (containing 10% fetal bovine serum, 100 U/mL penicillin, and 100 µg/mL streptomycin). All the cells were cultured in an incubator at 37 ℃ with 5% CO2 and were subcultured after the cells grew to 70% to 80%. The cells used in the experiment were all logarithmic growth cells.
MTT assays
According to the standard of antineoplastic effect of natural drugs, the inhibition rate of plant crude extract on tumor cell IC50 less than 30 or 100 mg/L is about 80%, which can be preliminarily confirmed to have a certain anti-tumor effect. Therefore, SGC7901 cells were treated with five concentrations of ethyl acetate extract (250, 125, 62.5, 31.2 and 15.6 mg/L) from F. sinkiangensis for 48 hours. MTT assays analyzed the proliferation of SGC7901 cells. About 2×105 cells were plated in 96-well plates and incubated for 24 hours. To assess cell viability, the cells were cultured with MTT solution (Solarbio, Beijing, China) (5 mg/mL) and incubated for 4 hours, and 150 µL DMSO (Sigma, St. Louis, MO, USA) was applied to treat the cells. The optical density value at 490 nm was detected with a microplate analyze (Thermo, Waltham, MA, USA).
Transwell assay
SGC7901 cells were seeded in a 6-well plate, and two concentrations (12.5, 6.25 mg/L) of ethyl acetate extract of F. sinkiangensis and positive control drug cisplatin (Jiangsu Hansoh Pharmaceutical Group Co., Ltd., Lianyungang, China) were added. After treatment for 48 hours, SGC7901 cells were collected, resuspended in serum-free cell culture medium, and were added to the upper chamber of the Transwell chamber (Corning, Corning, NY, USA). The chamber precoated with Matrigel (Corning) was used for the invasion assay and that without Matrigel was used for the migration assay. Cell culture medium containing 10% fetal bovine serum was added to the lower chamber. After culturing for 72 hours, the cells in the lower Transwell chamber were fixed with paraformaldehyde (4%) for 30 minutes and stained for 30 minutes. Finally, the number of migrated and invaded cells in each group was counted under the microscope.
Wound healing assay
SGC7901 cells were treated with ethyl acetate extract of F. sinkiangensis (12.5, 6.25 mg/L) and positive control drug cisplatin (12.5, 6.25 mg/L) for 48 hours. Approximately 2×105 SGC7901 cells were plated into the 24-well plates and incubated overnight to reach a fully confluent monolayer. A 20-µL pipette tip was applied to slowly cut a straight line across the well. The well was washed by phosphate buffered saline (PBS) three times and the medium was changed to serum-free medium and culture was continued. At 24 and 48 hours of incubation, the scratch distance (width between scratch lines) was measured, respectively. The wound healing percentage was calculated.
Statistical analysis
The SPSS17.0 statistical software (IBM, Armonk, NY, USA) was used, and the data are expressed as mean ± standard deviation (SD). One-way ANOVA was used for multiple comparisons followed by pairwise comparison with the least significant difference (LSD) method. A P value <0.05 indicates that the difference is statistically significant.
Results
Active compounds and target genes prediction of F. sinkiangensis
Based on our previous experiments on the separation of chemical compounds of F. sinkiangensis and a literature review, 56 main chemical compounds of F. sinkiangensis were identified. 23 active compounds of F. sinkiangensis were screened by SwissADME (Table 1). The potential target genes of 23 active compounds were predicted by the PharmMapper platform, and a total of 288 target genes were obtained by deleting repetitive values.
Table 1
F. sinkiangensis compounds | Compound CID | GI absorption | Lipinski | Druglikeness | Bioavailability score |
---|---|---|---|---|---|
Asacoumarin B | 4220856 | High | Yes | 4 | 0.56 |
Assafoetidin | 131751454 | High | Yes | 5 | 0.55 |
Auraptene | 1550607 | High | Yes | 4 | 0.55 |
Badrakemone | 101793077 | High | Yes | 5 | 0.55 |
Diversin | 13800313 | High | Yes | 5 | 0.55 |
Polyanthinin | 7002233 | High | Yes | 2 | 0.55 |
Umbelliferone | 5281426 | High | Yes | 3 | 0.55 |
Badrakemin | 1771505 | High | Yes | 4 | 0.55 |
Farnesiferol B | 1779468 | High | Yes | 4 | 0.55 |
Farnesiferol C | 15559239 | High | Yes | 4 | 0.55 |
Farnesiferol A | 7067262 | High | Yes | 5 | 0.55 |
Fekrynol acetate | 59052606 | High | Yes | 2 | 0.55 |
Feshurin | 11873225 | High | Yes | 5 | 0.55 |
Galbanic acid | 11873225 | High | Yes | 4 | 0.56 |
Gummosin | 7092581 | High | Yes | 5 | 0.55 |
Isofeterin | 16093742 | High | Yes | 5 | 0.55 |
Isosamarcandin angelate | 6442630 | High | Yes | 3 | 0.55 |
Lehmannolol | 16093743 | High | Yes | 4 | 0.55 |
Lehmannolone | 101418600 | High | Yes | 4 | 0.55 |
Karatavicinol | 44386968 | High | Yes | 2 | 0.55 |
Methyl galbanate | 7075765 | High | Yes | 4 | 0.55 |
Sinkianone | 101418599 | High | Yes | 4 | 0.55 |
Ferulsinaic acid | 102469382 | High | Yes | 4 | 0.56 |
GI, gastrointestinal.
GC-related target genes
A total of 10,433 GC target genes were identified after removing repetitive and false positive genes from the GeneCards database and standardizing them with the Uniprot database. 2,727 GC target genes were screened based on the criteria “Relevance Score” greater than 2 times the median.
D-C-T-D network construction
By using Venny 2.1 to obtain the target gene set of GC and active compounds, 189 target genes were obtained (Figure 1A). Cytoscape 3.7.2 software was used to establish the D-C-T-D network, and the degree values of compounds-target genes were calculated, as shown in Figure 1B). The higher the degree, the closer the relationship between compounds and target genes, and the more important the compounds are in this network. According to the analysis of network topology parameters, active compounds with the first five of degree were screened as the core active compounds, and the five core active compounds are listed in Table 2.
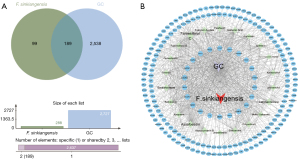
Table 2
No. | Core compounds | Degree | Molecular structure |
---|---|---|---|
01 | Farnesiferol C | 65 | ![]() |
02 | Assafoetidin | 49 | ![]() |
03 | Lehmannolone | 39 | ![]() |
04 | Badrakemone | 38 | ![]() |
05 | Feshurin | 38 | ![]() |
PPI network analysis
To further explore the possible relationship between the intersected target genes and better understand the therapeutic mechanism of F. sinkiangensis for GC, we constructed a PPI network composed of 189 nodes and 130 edges, with an average connection degree of 1.38, as shown in Figure 2A and the top intersected 30 target genes are shown in Figure 2B and Table 3. The nodes represent the target genes, and the edges represent the relationship between proteins and proteins. In this PPI network, the degree of the target gene is proportional to its importance. As shown in Figure 2B, the target genes related to energy metabolism of tumor cells might be the key to the therapeutic effect of F. sinkiangensis against GC.
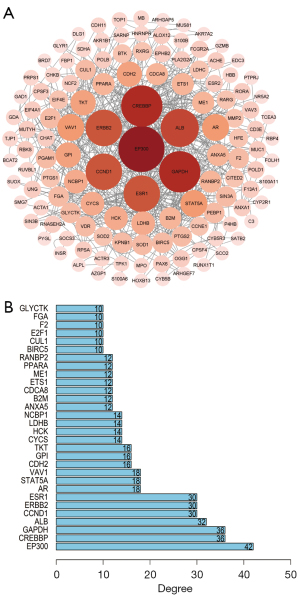
Table 3
Target gene | Target protein | Degree |
---|---|---|
EP300 | Histone acetyltransferase p300 | 42 |
CREBBP | CREB-binding protein | 36 |
GAPDH | Glyceraldehyde-3-phosphate dehydrogenase | 36 |
ALB | Serum albumin | 32 |
CCND1 | G1/S-specific cyclin-D1 | 30 |
ERBB2 | Receptor tyrosine-protein kinase erbB-2 | 30 |
ESR1 | Estrogen receptor | 30 |
AR | Androgen receptor | 18 |
STAT5A | Signal transducer and activator of transcription 5A | 18 |
VAV1 | Proto-oncogene vav | 18 |
CDH2 | Cadherin-2 | 16 |
GPI | Glucose-6-phosphate isomerase | 16 |
TKT | Transketolase | 16 |
CYCS | Cytochrome c | 14 |
HCK | Tyrosine-protein kinase HCK | 14 |
LDHB | L-lactate dehydrogenase B chain | 14 |
NCBP1 | Nuclear cap-binding protein subunit 1 | 14 |
ANXA5 | Annexin A5 | 12 |
B2M | Beta-2-microglobulin | 12 |
CDCA8 | Borealin | 12 |
ETS1 | Protein C-ets-1 | 12 |
ME1 | NADP-dependent malic enzyme | 12 |
PPARA | Peroxisome proliferator-activated receptor alpha | 12 |
RANBP2 | E3 SUMO-protein ligase RanBP2 | 12 |
BIRC5 | Baculoviral IAP repeat-containing protein 5 | 10 |
CUL1 | Cullin-1 | 10 |
E2F1 | Transcription factor E2F1 | 10 |
F2 | Prothrombin | 10 |
FGA | Fibrinogen alpha chain | 10 |
GLYCTK | Glycerate kinase | 10 |
Go enrichment analysis
We used the clusterProfiler package to perform GO enrichment analysis on 29 target genes. Only GO terms with P<0.05 were significant, and the top GO 10 terms were visualized. A total of 474 items were significantly enriched in the biological process analysis, including response to steroid hormone, monosaccharide metabolic process, hexose metabolic process, multi-multicellular organism process, transcription initiation from RNA polymerase II promoter, pyridine nucleotide metabolic process, nicotinamide nucleotide metabolic process, female pregnancy, pyridine−containing compound metabolic process, and monosaccharide biosynthetic process (Figure 3).
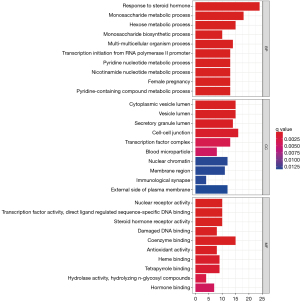
For cellular component analysis, 37 items were enriched, including cell−cell junction, cytoplasmic vesicle lumen, vesicle lumen, secretory granule lumen, transcription factor complex, nuclear chromatin, external side of the plasma membrane, membrane region, blood microparticle, and immunological synapse (Figure 3).
In terms of molecular function, 77 items were significantly enriched, including coenzyme binding, nuclear receptor activity, transcription factor activity, direct ligand regulated sequence−specific DNA binding, steroid hormone receptor activity, heme binding, tetrapyrrole binding, damaged DNA binding, antioxidant activity, hormone binding, and hydrolase activity, hydrolyzing N−glycosyl compounds (Figure 3).
KEGG enrichment analysis
The R package was used to perform KEGG enrichment analysis on 189 target genes. Only KEGG terms with P<0.05 were considered significant, yielding 104 relevant pathways. The top 15 KEGG pathways are displayed in Table 4 and Figure 4. Significantly enriched pathways for F. sinkiangensis in GC included carbon metabolism, pentose phosphate pathway, chemical carcinogenesis-receptor activation, Glucagon signaling pathway, HIF-1 signaling pathway, glycolysis/gluconeogenesis, and biosynthesis of amino acids. These findings suggest that 7 energy metabolism-related pathways are significantly related to F. sinkiangensis’s anti-GC activity, implying that F. sinkiangensis's therapeutic activity is mediated by inhibition of the tumor cell energy metabolism and promoting tumor cell apoptosis. According to KEGG enrichment pathway analyses, the mechanism of F. sinkiangensis in treating GC is related to the glycolysis/gluconeogenesis, pentose phosphate pathway, etc.
Table 4
Pathway | Name | Number of target genes | p.adjust |
---|---|---|---|
hsa01200 | Carbon metabolism | 11 | 0.001054302 |
hsa00030 | Pentose phosphate pathway | 6 | 0.001288682 |
hsa05207 | Chemical carcinogenesis-receptor activation | 14 | 0.001331695 |
hsa04922 | Glucagon signaling pathway | 9 | 0.005706446 |
hsa03410 | Base excision repair | 5 | 0.011869038 |
hsa04066 | HIF-1 signaling pathway | 8 | 0.023980785 |
hsa05219 | Bladder cancer | 5 | 0.023980785 |
hsa00010 | Glycolysis/gluconeogenesis | 6 | 0.03218902 |
hsa04520 | Adherens junction | 6 | 0.03227022 |
hsa05215 | Prostate cancer | 7 | 0.03227022 |
hsa05223 | Non-small cell lung cancer | 6 | 0.03227022 |
hsa05166 | Human T-cell leukemia virus 1 infection | 11 | 0.03227022 |
hsa00270 | Cysteine and methionine metabolism | 5 | 0.03227022 |
hsa01230 | Biosynthesis of amino acids | 6 | 0.033186575 |
hsa00640 | Propanoate metabolism | 4 | 0.036450369 |
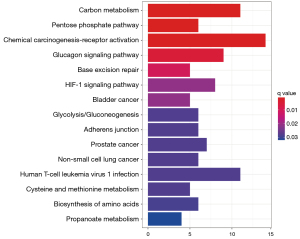
Expression of core genes before and after STAD
mRNA expression of core genes in STAD and paracancerous stomach tissues
Through the use of the GEPIA database, the TCGA analysis data of seven core genes were retrieved, and the mRNA expression data of core genes in STAD and paracancerous stomach tissues were studied. As shown in Figure 5A, six core genes ERBB2, GAPDH, GPI, TKT, GLYCTK, and ME1 exhibited significantly higher mRNA levels in STAD tissues than in paracancerous stomach tissues (P<0.05). The mRNA expression of the six core genes was explored further by the UALCAN database. As shown in Figure 5B, except for ME1, the mRNA expression of the other five core genes was substantially higher in STAD tissues than in paracancerous stomach tissues by UALCAN (P<0.01). These findings showed that the five core genes play a key role in the occurrence and progression of GC and are important target genes for GC diagnosis, intervention, and treatment.
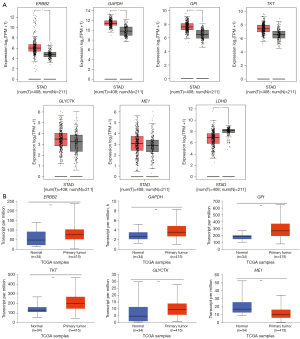
Core target gene immunohistochemistry in STAD and paracancerous tissues
The HPA database was used to obtain immunohistochemical images of GPI, TKT, GLYCTK, ERBB2, and GAPDH in STAD and paracancerous stomach tissues, and the changes in protein expression in the core genes were analyzed. As shown in Figure 6, the findings revealed that the expression of these five core genes in STAD was significantly higher than in paracancerous stomach tissues at the protein level (P<0.01). Our findings suggested that the five core genes play a key role in the occurrence and progression of GC and are important target genes for GC diagnosis, intervention, and treatment.
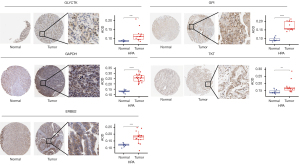
Prognostic value of highly expressed core genes in GC
GPI, TKT, GLYCTK, ERBB2, and GAPDH expressions in GC patients were found in the database (Figure 7). From the survival curves, we found that high mRNA expression of GPI (HR: 1.79, 95% CI: 1.47–2.17, P=2e-09), TKT (HR: 2.48, 95% CI: 1.98–3.1, P=2.8e-16), ERBB2 (HR: 1.36, 95% CI: 1.13–1.64, P=0.0011), GAPDH (HR: 1.79, 95% CI: 1.49–2.14, P=1.7e-10), GLYCTK (HR: 1.26, 95% CI: 1.02–1.56, P=0.036) were associated with a poor prognosis.
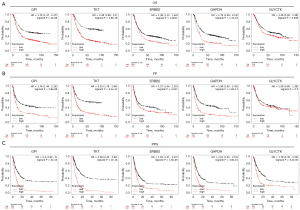
Furthermore, our results showed that the overexpression of GPI (HR: 1.76, 95% CI: 1.44–2.15, P=2.9e-08), TKT (HR: 2.25, 95% CI: 1.79–2.84, P=2.1e-12), ERBB2 (HR: 1.27, 95% CI: 1.04–1.55, P=0.021), GAPDH (HR: 1.98, 95% CI: 1.62–2.42, P=1.6e-11) were related to poor FP (First Progression), whereas overexpression of GLYCTK (HR: 1.08, 95% CI: 0.85–1.38, P=0.5) was not related to FP. At the same time, the high expression of GPI (HR: 2.4, 95% CI: 1.92–3, P=3e-15), TKT (HR: 3.09, 95% CI: 2.44–3.92, P<1e-16), ERBB2 (HR: 1.65, 95% CI: 1.31–2.07, P=1.6e-05) and GAPDH (HR: 2.01, 95% CI: 1.61–2.51, P=3.8e-10), GLYCTK (HR: 1.78, 95% CI: 1.35–2.34, P=3.4e-05) were correlated with shorter post-progression survival (PPS).
In conclusion, this study provided novel insights into the role of GPI, TKT, GLYCTK, ERBB2, and GAPDH in GC and identified potential diagnostic and prognostic biomarkers. Indeed, GPI, TKT, GLYCTK, ERBB2, and GAPDH have huge prospects for application as immunotherapy biomarkers in GC patients.
Molecular docking simulation
The binding energy values were acquired using molecular docking studies. The docking details are provided in Figure 8, and the binding energies of the 5 core compounds in F. sinkiangensis with their core target genes were less than −5 kcal/mol, indicating high affinity; the less energy needed, the more stable the binding. To further study the interaction between compounds and target genes, the docking structure map and binding sites of each core compound and the target gene with the strongest affinity were visualized. As shown in Figure 9, the solid yellow line represents hydrogen bonding, and the dotted line represents hydrophobic interactions. Fewer amino acid residues were observed around the compound, mainly bound to the compound by electrostatic (hydrogen bonding) and hydrophobic interactions. The results of molecular docking revealed that Farnesiferol C, Assafoetidin, Lehmannolone, Badrakemone, and Feshurin were the main compounds of F. sinkiangensis in the treatment of GC.
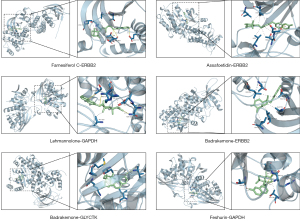
Ethyl acetate extract of F. sinkiangensis decreases proliferation of GC cells
The MTT assay was performed to analyze cell proliferation. Ethyl acetate extract of F. sinkiangensis repressed the viability of SGC7901 cells in a dose-dependent manner and 250 mg/L F. sinkiangensis extract had a greater effect. As shown in Figure 10, F. sinkiangensis extract (125, 250 mg/L) markedly reduced proliferation of SGC7901 cells, suggesting that F. sinkiangensis extract decreases proliferation of GC cells.
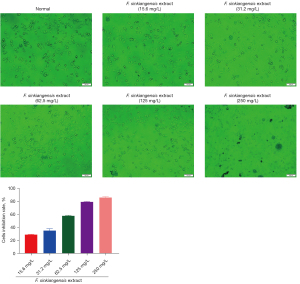
Ethyl acetate extract of F. sinkiangensis reduces invasion and migration of GC cells
We further measured the effect of ethyl acetate extract of F. sinkiangensis on the migration and invasion of GC cells. As shown in Figure 11A,11B, transwell assays indicated that the migration and invasion of SGC7901 cells were markedly decreased by F. sinkiangensis extract (6.25, 12.5 mg/L). Consistently, the treatment of F. sinkiangensis extract (6.25, 12.5 mg/L) significantly repressed wound healing in SGC7901 cells (Figure 11C), indicating that F. sinkiangensis extract is able to attenuate the migration and invasion of GC cells.
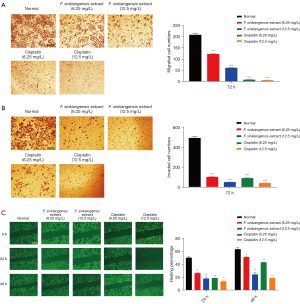
Discussion
In this study, F. sinkiangensis was found to have an antitumor effect in in vitro models, inhibiting the proliferation, migration, and invasion of GC cells, corroborating that F. sinkiangensis has a clear anti-GC effect and is worth further research and development.
The material basis and mechanism of F. sinkiangensis in the treatment of GC were investigated using network pharmacology and molecular docking techniques in this study. 23 putative active compounds and 189 related target genes were screened. When the drug-compound-target-disease network was established, 5 core active compounds of F. sinkiangensis in treating GC were identified, including Assafoetidin, Badrakemone, Farnesiferol C, Feshurin, Lehmannolone. These 5 active ingredients were Ferula-specific sesquiterpenes and coumarins. These chemical compounds have been associated with many properties, including anticancer, P-glycoprotein inhibition, and antiinflammation. Kasaian et al. reported that Farnesiferol A, B, and C have tumor cytotoxicity, apoptosis promotion, reversal of multidrug resistance, and antimutagenic activity (33-35). Hasanzadeh et al. discovered that in the MCF-7 cell line, farnesiferol C causes cell cycle arrest and apoptosis that is mediated by oxidative stress (36). Li et al. discovered that chemicals extracted from the seeds of F. sinkiangensis, such as Lehmannolone, Lehmannolol, Sinkianone, and Fekrynol, inhibits the growth of cervical cancer HeLa cells, with IC50 values ranging from 20.4 to 226.2 µmol.L−1 (37). Kamoldinov et al. reported that Feshurin showed high inhibitory activity against mino human lymphocyte cell with IC50 values of 7.88±0.60 µM (38). In non-small-cell lung cancers (NSCLCs), Jung JH found that Farnesiferol C increases the antitumor effects of puromycin or doxorubicin and induces apoptosis and G1 arrest through the regulation of ribosomal protein L11 and c-Myc (39).
PPI network analysis of the key target genes of F. sinkiangensis in anti-GC showed significant enrichment in biological processes such as glycolysis metabolism and Pentose phosphate pathway. 15 signaling pathways significantly related to the treatment of GC by F. sinkiangensis were screened using GO and KEGG pathway enrichment analyses with P<0.05 as the criteria, including the glycolysis/gluconeogenesis, glucagon signaling pathway, carbon metabolism, pentose phosphate pathway, cysteine and methionine metabolism, HIF-1 signaling pathway, biosynthesis of amino acids, propanoate metabolism, chemical carcinogenesis-receptor activation, base excision repair, bladder cancer, adherens junction, prostate cancer, NSCLC, human T-cell leukemia virus 1 infection. These findings suggest that 7 energy metabolism-related pathways are significantly related to F. sinkiangensis’s anti-GC activity, implying that F. sinkiangensis’s therapeutic activity is mediated by inhibition of the tumor cell energy metabolism and promoting tumor cell apoptosis. According to KEGG enrichment pathway analyses, the mechanism of F. sinkiangensis in treating GC is related to the glycolysis/gluconeogenesis, pentose phosphate pathway and so on.
OttoWarburg, Nobel Laureate in Physiology or Medicine, identified aberrant energy metabolism in cancer in 1931. Interestingly, even in the presence of plentiful oxygen, adenosinetriphosphate (ATP) synthesis in tumor cells does not result from oxidative phosphorylation of glucose but rather with a faster glycolysis rate, increasing the glucose intake and the lactic acid formation, which is called aerobic glycolysis, or the Warburg effect. One of the most basic characteristics of malignancies is the Warburg effect. It is widely acknowledged that high-rate glycolysis is the primary source of energy for rapidly growing tumor cells, which enhances tumor adaptability to hypoxia and other stressful environments and boosts the malignant potential of tumors (40). In recent years, tumor research has shifted its focus to targeted aerobic glycolysis.
In the glycolysis/gluconeogenesis process, F. sinkiangensis inhibited the activity of six related enzymes, indicating its importance. Glycolysis involves the breaking down of glucose or glycogen into pyruvate in the cytoplasm without using oxygen and generating tiny amounts of ATP. Even when there is enough oxygen, ATP generation in tumor tissue comes from aerobic glycolysis, known as the Warburg effect. In this study, GPI can transform glucose-6-phosphate and fructose-6-phosphate into each other in the cytoplasm. Fructose-1,6-bisphosphatase 1 (FBP1) catalyzes the conversion of fructose-6-phosphate to fructose-1,6- diphosphate. GAPDH catalyzes the conversion of glyceraldehyde 3-phosphate to 1,3-diphosphoglyceric acid. Phosphoglycerate dismutase (PGAM) catalyzes 3-phospho-D-glyceric acid rearrangement to form 2-phosphoglyceric acid. It has been established that tumor cells rely on lactate dehydrogenase (LDHB) to produce lactic acid after glucose is metabolized to pyruvate by glycolysis, which alters the tumor cell microenvironment and aids tumor cell invasion, metastasis, and immune evasion (41). ErbB2 is a transmembrane receptor tyrosine kinase that governs cell physiological responses such as cell growth, division, differentiation, adhesion, function, and apoptosis. ErbB2 promoted glycolysis via heat shock factor 1 (HSF1)/lactate dehydrogenase A (LDHA) axis and ErbB2-mediated glycolysis was required for the growth of breast cancer cells (42).
The pentose phosphate pathway (PPP), which branches from glycolysis at the first committed step of glucose metabolism, is required for the synthesis of ribonucleotides and is a major source of NADPH (43). By providing cells with both ribose-5-phosphate and NADPH for the detoxification of intracellular reactive oxygen species, reductive biosynthesis, and ribose biogenesis, PPP plays a crucial role in controlling the growth of cancer cells. As a result, changes to the PPP directly affect cell growth, survival, and senescence (44).
In the PPP, it appears that F. sinkiangensis works by inhibiting the activity of three related enzymes. To meet the unlimited and exuberant growth needs of tumor cells, the metabolic pentose phosphate pathway, which is rarely used in normal cells, is activated in tumor cells. In this study, transketolase (TKT) was found to be a rate-limiting enzyme in the non-oxidative part of the PPP that is responsible for maintaining ribose 5-phosphate levels. For cell proliferation to continue, TKT is required (45,46). Ribose-phosphate pyrophosphokinase 1 (PRPS1) and ribokinase (RBKS) are involved in nucleotide biosynthesis and subsequent purine and pyrimidine biosynthesis (47).
Herein, bioinformatics analysis was used to confirm the five core target genes: GPI, TKT, GLYCTK, ERBB2, and GAPDH. The five core target genes were highly expressed in GC tissues, which were potential biomarkers for the diagnosis and prognosis of GC and played an important role in the pathogenesis and treatment of GC, according to mRNA, protein expression, and survival time. Using molecular docking, the five active compounds were tightly correlated to GC-related core target genes. These findings demonstrate that F. sinkiangensis may inhibit GC cells from metabolizing energy, such as glycolysis and pentose phosphate pathways, by suppressing the expression of the GPI, TKT, GLYCTK, ERBB2 and GAPDH genes. This would inhibit GC cells from proliferating, migrating, and invading healthy tissue.
In this study, although the possible targets by F. sinkiangensis on GC was predicted with network pharmacology and molecular docking, the results are only predictions after all. And for the next step, we intend to use F. sinkiangensis to treat GC in both in vivo and in vitro experimental models, in order to analyze the express level of protein and the mRNA level by transcriptomics and proteomics studies.
Conclusions
To summarize, this study showed that ethyl acetate extract of F. sinkiangensis weakened the proliferation, migration, and invasion of human gastric cancer SGC7901 cells, and systematically explained the potential mechanism of F. sinkiangensis in treating GC using network pharmacology, molecular docking, and bioinformatics analysis technology. Assafoetidin, Badrakemone, Farnesiferol C, Feshurin, Lehmannolone, and other sesquiterpenes and coumarins are found to be the main active ingredients. F. sinkiangensis promotes tumor cell apoptosis and prevents energy metabolism by inhibiting the glycolysis/gluconeogenesis and pentose phosphate pathways. This study aims to provide the foothold for further studies on the pharmacodynamics of F. sinkiangensis and new ideas for improving the application of traditional Chinese medicine in treating GC. However, further studies are warranted to validate the putative mechanisms revealed by this research.
Acknowledgments
Funding: The work was supported by
Footnote
Reporting Checklist: The authors have completed the MDAR reporting checklist. Available at https://tcr.amegroups.com/article/view/10.21037/tcr-22-2292/rc
Conflicts of Interest: All authors have completed the ICMJE uniform disclosure form (available at https://tcr.amegroups.com/article/view/10.21037/tcr-22-2292/coif). The authors have no conflicts of interest to declare.
Ethical Statement: The authors are accountable for all aspects of the work in ensuring that questions related to the accuracy or integrity of any part of the work are appropriately investigated and resolved. This study was conducted in accordance with the Declaration of Helsinki (as revised in 2013).
Open Access Statement: This is an Open Access article distributed in accordance with the Creative Commons Attribution-NonCommercial-NoDerivs 4.0 International License (CC BY-NC-ND 4.0), which permits the non-commercial replication and distribution of the article with the strict proviso that no changes or edits are made and the original work is properly cited (including links to both the formal publication through the relevant DOI and the license). See: https://creativecommons.org/licenses/by-nc-nd/4.0/.
References
- Sung H, Ferlay J, Siegel RL, et al. Global Cancer Statistics 2020: GLOBOCAN Estimates of Incidence and Mortality Worldwide for 36 Cancers in 185 Countries. CA Cancer J Clin 2021;71:209-49. [Crossref] [PubMed]
- Global, regional, and national age-sex-specific mortality for 282 causes of death in 195 countries and territories, 1980-2017: a systematic analysis for the Global Burden of Disease Study 2017. Lancet 2018;392:1736-88. [Crossref] [PubMed]
- Eusebi LH, Telese A, Marasco G, et al. Gastric cancer prevention strategies: A global perspective. J Gastroenterol Hepatol 2020;35:1495-502. [Crossref] [PubMed]
- Gharaei R, Akrami H, Heidari S, et al. The suppression effect of Ferula gummosa Boiss. extracts on cell proliferation through apoptosis induction in gastric cancer cell line. Eur J Integr Med 2013;5:241-7. [Crossref]
- Zhang L, Si J, Li G, et al. Umbelliprenin and lariciresinol isolated from a long-term-used herb medicine Ferula sinkiangensis induce apoptosis and G0/G1 arresting in gastric cancer cells. RSC Adv 2015;5:91006-17. [Crossref]
- Zhang L, Sun X, Si J, et al. Umbelliprenin isolated from Ferula sinkiangensis inhibits tumor growth and migration through the disturbance of Wnt signaling pathway in gastric cancer. PLoS One 2019;14:e0207169. [Crossref] [PubMed]
- Yan L, Zhao S, Zhang H, et al. Effects of five kinds of Resina Ferulae in Xinjiang on cell proliferation inhibition and apoptosis of human gastric carcinoma SGC-7901 cells in vitro. China Journal of Traditional Chinese Medicine and Pharmacy 2020;35:5486-90.
- Zhang H, Li W, Zhou L, et al. Screening of Active Parts of Xinjiang Ferulae Resina with Anti-tumor Effects. Chinese Journal of Information on Traditional Chinese Medicine 2016;23:52-6.
- Luo F, Lu J, Zhang H, et al. Inhibitory Effect of Ethyl Acetate Part of Ferula sinkiangensis on S180 Solid Tumor in Mice. China Pharmacy 2016;27:4388-91.
- Yan L, Li W, Zhao S, et al. Study on GC-MS fingerprint of volatile oil from Ferula Soongarica and its anti-gastric cancer effect. Journal of Xinjiang Medical University 2020;43:957-61.
- Mei L, Wang X, Wang Y. Research Progress on Chemical constituents, Pharmacological Action and Toxicology of Ferula officinalis. China Pharmacy 2016;27:534-8.
- Wang L, Sun R, Xu M, et al. Research on the Development of Chemical Components, Pharmacological Activities and Toxicity of Resina Ferulae. World Chinese Medicine 2020;15:3887-94.
- Yang X. Bioactive Material Basis of Medicinal Plants in Genus Ferula. Modern Chinese Medicine 2018;20:123-44.
- Xing Y, Li N, Xue J, et al. Research Progress on Chemical constituents of Ferula. Journal of Shenyang Pharmaceutical University 2012;29:730-41.
- Patiguli F, Xieraili T, Gulishan M, et al. Clinical Application and Research Progress of Uighur Medicine Ferula in Uygur Medicine. Journal of Medicine and Pharmacy of Chinese Minorities 2011;17:61-4.
- Huang Y, Yue L, Shen X, et al. Chemical constituents and pharmacological activities of Ferula sinkiangensis K.M.Shen: research advances. Journal of International Pharmaceutical Research 2017;44:495-9.
- Zhao B, Gui H, Zhu Y, et al. Research Progress in Chemical Components, Pharmacological Effectiveness and Toxicity of Ferula assafoetid. Chinese Journal of Experimental Traditional Medical Formulae 2011;17:279-81.
- Lin J, Jin M, Wu C, et al. Advances in studies on chemical constituents and pharmacological effects of Ferula. Strait Pharmaceutical Journal 2014;1-3.
- Daina A, Michielin O, Zoete V. SwissADME: a free web tool to evaluate pharmacokinetics, drug-likeness and medicinal chemistry friendliness of small molecules. Sci Rep 2017;7:42717. [Crossref] [PubMed]
- Liu X, Ouyang S, Yu B, et al. PharmMapper server: a web server for potential drug target identification using pharmacophore mapping approach. Nucleic Acids Res 2010;38:W609-14. [Crossref] [PubMed]
- UniProt: the universal protein knowledgebase. Nucleic Acids Res 2017;45:D158-69. [Crossref] [PubMed]
- Stelzer G, Rosen N, Plaschkes I, et al. The GeneCards Suite: From Gene Data Mining to Disease Genome Sequence Analyses. Curr Protoc Bioinformatics 2016;54:1.30.1-1.30.33.
- Shannon P, Markiel A, Ozier O, et al. Cytoscape: a software environment for integrated models of biomolecular interaction networks. Genome Res 2003;13:2498-504. [Crossref] [PubMed]
- Szklarczyk D, Morris JH, Cook H, et al. The STRING database in 2017: quality-controlled protein-protein association networks, made broadly accessible. Nucleic Acids Res 2017;45:D362-8. [Crossref] [PubMed]
- Tang Z, Li C, Kang B, et al. GEPIA: a web server for cancer and normal gene expression profiling and interactive analyses. Nucleic Acids Res 2017;45:W98-W102. [Crossref] [PubMed]
- Chandrashekar DS, Bashel B, Balasubramanya SAH, et al. UALCAN: A Portal for Facilitating Tumor Subgroup Gene Expression and Survival Analyses. Neoplasia 2017;19:649-58. [Crossref] [PubMed]
- Thul PJ, Lindskog C. The human protein atlas: A spatial map of the human proteome. Protein Sci 2018;27:233-44. [Crossref] [PubMed]
- Zhang R, Li R, Zhang Y, et al. Immunohistochemical identification and fertility assessment of a mouse model of thin endometrium. Chinese Journal of Comparative Medicine 2018;28:19-26.
- Szász AM, Lánczky A, Nagy Á, et al. Cross-validation of survival associated biomarkers in gastric cancer using transcriptomic data of 1,065 patients. Oncotarget 2016;7:49322-33. [Crossref] [PubMed]
- Berman HM, Westbrook J, Feng Z, et al. The Protein Data Bank. Nucleic Acids Res 2000;28:235-42. [Crossref] [PubMed]
- Trott O, Olson AJ. AutoDock Vina: improving the speed and accuracy of docking with a new scoring function, efficient optimization, and multithreading. J Comput Chem 2010;31:455-61. [PubMed]
- Yu JW, Yuan HW, Bao LD, et al. Interaction between piperine and genes associated with sciatica and its mechanism based on molecular docking technology and network pharmacology. Mol Divers 2021;25:233-48. [Crossref] [PubMed]
- Kasaian J, Mohammadi A. Biological activities of farnesiferol C: a review. J Asian Nat Prod Res 2018;20:27-35. [Crossref] [PubMed]
- Kasaian J, Mosaffa F, Behravan J, et al. Modulation of Multidrug Resistance Protein 2 Efflux in the Cisplatin Resistance Human Ovarian Carcinoma Cells A2780/RCIS by Sesquiterpene Coumarins. Phytother Res 2016;30:84-9. [Crossref] [PubMed]
- Kasaian J, Mosaffa F, Behravan J, et al. Reversal of P-glycoprotein-mediated multidrug resistance in MCF-7/Adr cancer cells by sesquiterpene coumarins. Fitoterapia 2015;103:149-54. [Crossref] [PubMed]
- Hasanzadeh D, Mahdavi M, Dehghan G, et al. Farnesiferol C induces cell cycle arrest and apoptosis mediated by oxidative stress in MCF-7 cell line. Toxicol Rep 2017;4:420-6. [Crossref] [PubMed]
- Li G, Li X, Cao L, et al. Sesquiterpene coumarins from seeds of Ferula sinkiangensis. Fitoterapia 2015;103:222-6. [Crossref] [PubMed]
- Kamoldinov K, Li J, Eshbakova K, et al. Sesquiterpene coumarins from Ferula samarkandica Korovin and their bioactivity. Phytochemistry 2021;187:112705. [Crossref] [PubMed]
- Jung JH, Kim MJ, Lee H, et al. Farnesiferol c induces apoptosis via regulation of L11 and c-Myc with combinational potential with anticancer drugs in non-small-cell lung cancers. Sci Rep 2016;6:26844. [Crossref] [PubMed]
- Zhao L, Li J. Hypoxia adaption in life——An analysis of Nobel Prize in Physiology or Medicine in 2019. Science & Technology Review 2020;38:79-85.
- Li L, Hou Z. MiR-125B-5p in Ovarian Cancer Tissue Reduces Tumor Energy Metabolism and Inhibits Tumor Cell Proliferation by Regulating Hexokinase-2. Chinese Journal of Cancer 2021;40:394-403.
- He L, Lv S, Ma X, et al. ErbB2 promotes breast cancer metastatic potential via HSF1/LDHA axis-mediated glycolysis. Med Oncol 2022;39:45. [Crossref] [PubMed]
- Patra KC, Hay N. The pentose phosphate pathway and cancer. Trends Biochem Sci 2014;39:347-54. [Crossref] [PubMed]
- Jiang P, Du W, Wu M. Regulation of the pentose phosphate pathway in cancer. Protein Cell 2014;5:592-602. [Crossref] [PubMed]
- Qin Z, Xiang C, Zhong F, et al. Transketolase (TKT) activity and nuclear localization promote hepatocellular carcinoma in a metabolic and a non-metabolic manner. J Exp Clin Cancer Res 2019;38:154. [Crossref] [PubMed]
- Li M, Zhao X, Yong H, et al. Transketolase promotes colorectal cancer metastasis through regulating AKT phosphorylation. Cell Death Dis 2022;13:99. [Crossref] [PubMed]
- Li Y, Liu F, Pang B, et al. Discussion on Staged Intervention of Chronic Colitis-Cancer Transformation with the Method of Reinforcing Healthy Qi and Resolving Toxins: from the Perspective of Tumor Energy Metabolism Imbalance. Journal of Traditional Chinese Medicine 2022;63:234-9.