Identifying a prognostic model and screening of potential natural compounds for acute myeloid leukemia
Highlight box
Key findings
• The predictive power of 5 genes (RHOBTB2, PLA2G4A, IL2RA, CSRP1, and OLFML2A) in combination with clinical features was better than clinical data alone for AML, and the stable docking of diosgenin-PLA2G4A, beta-sitosterol-IL2RA, and 3,4-di-O-caffeoylquinic acid-OLFML2A indicated that natural compounds might be new options for the treatment of AML.
What is known and what is new?
• RHOBTB2, PLA2G4A, IL2RA, CSRP1 and OLFML2A are involved in the development of AML.
• A prognostic model combining 5 genes with clinical features guided the prognosis of AML patients, and natural compounds targeting PLA2G4A, IL2RA and OLFML2A in AML were screened (Guadi, Fangji and Beiliujinu).
What is the implication, and what should change now?
• The predictive model consisting of combined clinical features of RHOBTB2, PLA2G4A, IL2RA, CSRP1, and OLFML2A is vital for guiding the prognosis of AML patients. Molecular docking screening of natural compounds with possible efficacy in AML provides new directions for subsequent drug development in AML.
Introduction
Acute myeloid leukemia (AML) is a heterogeneous hematologic malignancy characterized by clonal proliferation of abnormally differentiated or undifferentiated myeloid cells in the bone marrow and peripheral blood. The main clinical manifestations are anemia, bleeding, and infection. Most patients have poor prognoses, especially those with poor prognostic karyotypes or mutated genes (1-3). In recent years, with the development of chemotherapy, hematopoietic stem cell transplantation, bio-immunotherapy, cell therapy, and gene-targeted therapy, the complete remission rate and relapse-free survival rate of AML patients have been improved. However, most patients are still drug-resistant and relapse after remission (4-6). Different genetic characteristics of AML patients are often associated with various clinical prognostic features; it is of great significance to further elucidate the potential genes related to the prognosis of AML. Recently, different prognostic signatures with transcriptome profiles have been proposed for survival prediction including a 3-gene signature (7), a 5-gene signature (8), a 10-gene signature (9), an 85-gene signature (10), and a 17-gene leukemia stem cell (LSC) score (11). However, accurate prognostic stratification remains an unsolved problem in AML, along with the need for appropriate clinical treatment measures.
Network pharmacology is an approach to drug design that incorporates systems biology, network analysis, and genetic pleiotropy to understand drug-organism interactions and guide new drug discovery from a holistic perspective that improves or restores the balance of biological networks. Based on this, an approach to Traditional Chinese Medicine Systemic Pharmacological (TCMSP) was established to predict the targeting characteristics and pharmacological effects of herbal compounds, to screen multiple compounds from herbal formulations in a high-throughput manner, and to transform traditional Chinese medicine (TCM) from empirical medicine to an evidence-based medical system, which will accelerate the discovery of TCM and improve the current treatment options for diseases (12-15). Since its first appearance in the mid-1970s, molecular docking has represented a unique computer tool for drug design and discovery. It docks new natural compounds of potential therapeutic interest and predicts ligand-target interactions at the molecular level (16).
In this study, a predictive model of transcriptomic data combined with clinical features was developed to better predict the prognosis of AML patients through different bioinformatics tools and public databases. In addition, drugs with possible efficacy in AML through hub genes were identified by network pharmacology and validated by molecular docking. A new direction for subsequent basic research and drug development was provided. We present this article in accordance with the TRIPOD reporting checklist (available at https://tcr.amegroups.com/article/view/10.21037/tcr-22-2500/rc).
Methods
Data collection
RNA sequencing (RNA-seq) data on AML were obtained from The Cancer Genome Atlas (TCGA) database (https://portal.gdc.cancer.gov/). The complete clinical information of patients was downloaded from TCGA, and a total of 243 AML patients met the criteria at clinical information screening step, excluding samples with less than 30 days of follow-up. In addition, the GSE9476 dataset was downloaded from the Gene List module of the Gene Expression Omnibus (GEO) database for analysis. The study was conducted in accordance with the Declaration of Helsinki (as revised in 2013). Since the data involved in this study were obtained from the TCGA and GEO databases and in strict accordance with TCGA and GEO publication guidelines, no ethics committee approval was required.
Differentially expressed genes (DEGs) screening
The study identified TCGA and GSE9476 by the GEO2R online analysis tool, adjusted for P<0.05 and positive log fold change (FC) as cut-off criteria for DEGs screening. Statistical analysis and visualization were performed using R language (version 3.6.3), GEOquery package for data collation and download, limma package for gene variance analysis, ggplot2 package for gene volcano map, gene variance ranking and Wayne plot visualization, ComplexHeatmap package for row heat map visualization, the pROC package and ggplot2 package performed receiver operator characteristic (ROC) curve analysis of critical genes. The ggalluvial package analyzed the internal association of TCGA, GSE9476, and Vene intersection genes, and the ggalluvial package analyzed the inner association of TCGA, GSE9476, and Vene intersection genes.
Construction of protein-protein interaction (PPI) network
PPI networks were constructed using Cytoscape software for TCGA dataset, significantly DEGs of GSE9476 (P<0.05, logFC ≥2) and Vene intersection genes, and visualized using String online database for Vene intersection genes.
Functional enrichment analysis
Gene set enrichment analysis (GSEA, http://www.broadinstitute.org/gsea/index.jsp) was applied to explain the functional enrichment of gene expression data. Functional enrichment of intersection genes with prognostic value was explored. We visualized the Gene Ontology (GO) and Kyoto Encyclopedia of Genes and Genomes (KEGG) pathways using the ggplot2 package and clusterProfiler package.
Construction of prognostic risk score model
A total of 2,340 DEGs were identified between AML and normal subjects by analyzing the dataset. The intersection of 2,153 highly expressed AML genes in the GSE9476 dataset with 187 highly expressed genes in TCGA included 33 hub genes. Thirty-three differentially expressed hub genes were included in the least absolute shrinkage and selection operator (LASSO) (glmnet package & survival package) regression model to obtain the risk coefficient and establish the risk score model.
Development of prognostic nomogram model
The rms and survival packages were used to construct a nomogram to predict survival in AML patients. The accuracy of the model was validated using the calibration curves (rms package and survival package), concordance index (C-index), and ROC time-dependent curves (timeROC package and ggplot2 package). We included the five DEGs in a multivariate Cox regression analysis.
Target-related drugs
Symptom mapping (symMap Version 2.0) database was used to predict the candidate herbs, which will target the hub gene. We chose herbs with a false discovery rate (FDR) less than 0.05. The constituents of the obtained drugs were analyzed using the TCMSP online database, and those with oral bioavailability (OB) ≥30% and drug-likeness (DL) ≥0.18 were selected for follow-up studies.
Molecular docking
Before docking both structures, ligand and receptor structures were needed to prepare. Therefore, critical protein backbone structures were obtained from the Protein Database (PDB: https://www.rcsb.org/) and small-molecule drug structures of compounds with the most significant OB values from the Pub Chemical database (https://pubchem.ncbi.nlm.nih.gov/). Finally, the RCSB PDB online tool (https://cadd.labshare.cn/cb-dock2/php/index.php) was used to perform the molecular docking procedure, and the one with the smallest docking score was selected for the study.
Statistical analysis
Survival curves were generated using the Kaplan-Meier method and compared with the Cox test. Statistical analysis was performed using R language (version 3.6). The prognostic value of hub genes was analyzed by Cox and LASSO’s regression. Differences were considered statistically significant when P<0.05.
Results
DEGs analysis
One hundred and fifty-one AML patients with clinical, prognostic and gene expression data were included in the TCGA dataset (alive =54, dead =97); GSE9476 included 38 healthy individuals and 26 AML patients. DEGs was established based on two datasets, ranked according to logFC fold difference from largest to smallest, to investigate relevant biomarkers that can effectively predict prognosis in AML (Figure 1A). In addition, it used the volcano figure for differences in gene screening (P<0.05, |logFC| ≥1), selecting 2,340 high-expressed genes, lower expression gene 1,097 (Figure 1B). Further, 2,153 highly expressed genes in GSE9476 and 187 highly expressed genes in TCGA were screened out, and 33 essential genes were obtained by the intersection of the highly expressed genes from the two datasets (Figure 1C). The Sankey diagram was used to analyze the dimensions of 33 essential genes, and it was found that they were correlated with the dimensions of the first 33 critical genes of TCGA and GSE9476 (Figure 1D). The vital genes were visualized by heat map (Figure 1E).
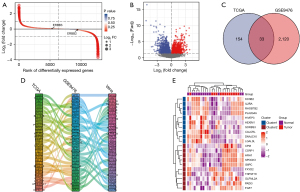
PPI network
Significant DEGs in TCGA and GSE9476 datasets were constructed for PPI networks. The results showed that these 33 differential genes acted as important components in PPI networks (Figure 2A). Network construction of hub genes also demonstrated some association (Figure 2B). Also, correlation heatmap analysis of 33 hub genes revealed that most were positively correlated (Figure 3).
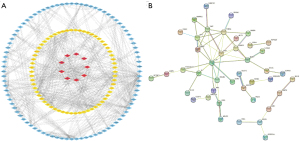
Functional analysis of critical genes
GO and KEGG analysis were performed on 33 DEGs as well as significant differential genes in the GSE9476 dataset and TCGA database, respectively hub genes identified in GSE9476 were mainly involved in cell adhesion molecule binding, pattern specification process, cell-substrate adherences junction, and phosphatidylinositol 3-kinase (PI3K)-Akt signaling pathway processes (Figure 4A), corresponding to GO: 0050839, GO: 0007389, GO: 0005924 and hsa04151, respectively (Figure 4B), see Table 1 for details. Hub genes found in TCGA were mainly involved in platelet alpha granule lumen, cytokine-cytokine receptor interaction and carbohydrate digestion and absorption processes (Figure 4C), they corresponded to GO: 0031093, hsa04060 and hsa04973, respectively (Figure 4D), and the detailed pathways involved are shown in Table 2. Thirty-three hub genes were mainly involved in carbohydrate digestion and absorption and galactose metabolism processes (Figure 4E). They corresponded to hsa04973 and hsa00052, respectively (Figure 4F), and see Table 3 for details. GESA enrichment analysis of 33 genes revealed that they were mainly enriched in NABA ECM Regulators, NABA Secreted Factors, Reactome Class A1 Rhodopsin Like Receptors, Reactome Degradation of the Extracellular Matrix, Reactome Extracellular Matrix Organization (Figure 4G).
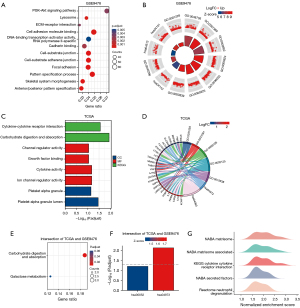
Table 1
Ontology | ID | Description | Gene ratio | Bg ratio | P value | P.adjust |
---|---|---|---|---|---|---|
BP | GO:0048705 | Skeletal system morphogenesis | 57/1,866 | 239/18,670 | 3.20e-10 | 1.12e-06 |
BP | GO:0007389 | Pattern specification process | 87/1,866 | 446/18,670 | 7.00e-10 | 1.12e-06 |
BP | GO:0009952 | Anterior/posterior pattern specification | 53/1,866 | 219/18,670 | 7.59e-10 | 1.12e-06 |
CC | GO:0005924 | Cell-substrate adherens junction | 77/1,915 | 408/19,717 | 8.85e-09 | 3.38e-06 |
CC | GO:0030055 | Cell-substrate junction | 77/1,915 | 412/19,717 | 1.37e-08 | 3.38e-06 |
CC | GO:0005925 | Focal adhesion | 76/1,915 | 405/19,717 | 1.42e-08 | 3.38e-06 |
MF | GO:0050839 | Cell adhesion molecule binding | 91/1,856 | 499/17,697 | 9.00e-08 | 1.00e-04 |
MF | GO:0045296 | Cadherin binding | 62/1,856 | 331/17,697 | 4.10e-06 | 0.002 |
MF | GO:0001228 | DNA-binding transcription activator activity, RNA polymerase II-specific | 75/1,856 | 439/17,697 | 1.40e-05 | 0.005 |
KEGG | hsa04142 | Lysosome | 36/977 | 128/8,076 | 6.57e-07 | 2.11e-04 |
KEGG | hsa04512 | ECM-receptor interaction | 25/977 | 88/8,076 | 2.75e-05 | 0.003 |
KEGG | hsa04151 | PI3K-Akt signaling pathway | 69/977 | 354/8,076 | 2.97e-05 | 0.003 |
Bg, background; BP, biological process; CC, cellular component; DEGs, differentially expressed genes; GO, Gene Ontology; MF, molecular function; KEGG, Kyoto Encyclopedia of Genes and Genomes.
Table 2
Ontology | ID | Description | Gene ratio | Bg ratio | P value | P.adjust |
---|---|---|---|---|---|---|
CC | GO:0031093 | Platelet alpha granule lumen | 5/146 | 67/19,717 | 1.39e-04 | 0.035 |
CC | GO:0031091 | Platelet alpha granule | 5/146 | 91/19,717 | 5.80e-04 | 0.072 |
MF | GO:0099106 | Ion channel regulator activity | 6/139 | 118/17,697 | 3.38e-04 | 0.067 |
MF | GO:0005125 | Cytokine activity | 8/139 | 220/17,697 | 3.51e-04 | 0.067 |
MF | GO:0019838 | Growth factor binding | 6/139 | 137/17,697 | 7.46e-04 | 0.091 |
MF | GO:0016247 | Channel regulator activity | 6/139 | 144/17,697 | 9.68e-04 | 0.091 |
MF | GO:0005201 | Extracellular matrix structural constituent | 6/139 | 163/17,697 | 0.002 | 0.091 |
KEGG | hsa04973 | Carbohydrate digestion and absorption | 5/73 | 47/8,076 | 6.00e-05 | 0.011 |
KEGG | hsa04060 | Cytokine-cytokine receptor interaction | 10/73 | 295/8,076 | 2.93e-04 | 0.028 |
Bg, background; CC, cellular component; DEGs, differentially expressed genes; GO, Gene Ontology; MF, molecular function; KEGG, Kyoto Encyclopedia of Genes and Genomes; TCGA, The Cancer Genome Atlas.
Table 3
Ontology | ID | Description | Gene ratio | Bg ratio | P value | P.adjust |
---|---|---|---|---|---|---|
KEGG | hsa04973 | Carbohydrate digestion and absorption | 3/16 | 47/8,076 | 9.81e-05 | 0.007 |
KEGG | hsa00052 | Galactose metabolism | 2/16 | 31/8,076 | 0.002 | 0.061 |
Bg, background; KEGG, Kyoto Encyclopedia of Genes and Genomes.
Establishment of critical gene prognostic models
To further explore key factors to guide the prognosis of AML patients, 33 key genes were included in the LASSO analysis (Figure 5A), combined with the equation of Figure 5B of the results. The model fitted best when the penalty coefficient was 5. The corresponding five related genes were selected to enter the model, which were Rho-related BTB domain containing 2 (RHOBTB2), phospholipase A2 (PLA2G4A), interleukin-2 receptor-α (IL2RA), cysteine and glycine-rich protein 1 (CSRP1), and olfactomedin-like 2A (OLFML2A). Risk factor analysis of these 5 hub genes revealed that the risk of death increased with increasing expression of the 5 genes (Figure 5C). In addition, multivariate cox regression analysis of the five hub genes revealed that these 5 hub genes were important prognostic factors, and CSRP1 and OLFML2A were independent risk factors for AML prognosis. Therefore, these five genes entered the equation as prognostic factors for AML (Table 4). Further analysis yielded the corresponding regression coefficients β1–β5, which were 0.075, 0.119, 0.069, 0.074, and 0.029, respectively. Based on the above formula, combined with the beta value of regression coefficient from the LASSO regression, the final predictive risk score model was established: risk score = 0.075 * RHOBTB2 + 0.119 * PLA2G4A + 0.069 * IL2RA + 0.074 * CSRP1 + 0.029 * OLFML2A.
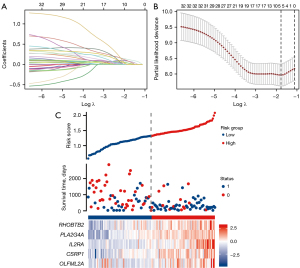
Table 4
Characteristics | N | Univariate analysis | Multivariate analysis | |||
---|---|---|---|---|---|---|
HR (95% CI) | P value | HR (95% CI) | P value | |||
Age (>60 years) | 61 | 3.333 (2.164–5.134) | <0.001 | 2.458 (1.503–4.019) | <0.001 | |
Cytogenetic risk (intermediate) | 76 | 2.957 (1.498–5.836) | 0.002 | 1.266 (0.570–2.811) | 0.563 | |
Cytogenetic risk (poor) | 31 | 4.157 (1.944–8.893) | <0.001 | 1.598 (0.675–3.781) | 0.286 | |
RHOBTB2 (high) | 69 | 2.437 (1.572–3.779) | <0.001 | 1.547 (0.961–2.492) | 0.073 | |
PLA2G4A (high) | 70 | 3.387 (2.143–5.355) | <0.001 | 1.690 (0.959–2.978) | 0.070 | |
IL2RA (high) | 71 | 2.027 (1.315–3.127) | 0.001 | 1.007 (0.630–1.611) | 0.976 | |
CSRP1 (high) | 71 | 2.356 (1.527–3.635) | <0.001 | 1.747 (1.109–2.751) | 0.016 | |
OLFML2A (high) | 69 | 2.362 (1.534–3.639) | <0.001 | 1.697 (1.057–2.724) | 0.029 |
CI, confidence interval; CSRP1, cysteine and glycine-rich protein 1; HR, hazard ratio; IL2RA, interleukin-2 receptor-α; OLFML2A, olfactomedin-like 2A; PLA2G4A, phospholipase A2; RHOBTB2, Rho-related BTB domain containing 2.
Clinical characteristics of 5 hub genes associated with poor prognosis in AML
Differential analysis revealed that RHOBTB2, PLA2G4A, IL2RA, CSRP1, and OLFML2A were all significantly more expressed in AML than in the normal group (Figure 6A), critical gene expression was positively correlated with age greater than 60 years (Figure 6B) and cytogenetic risk (Figure 6C), indicating that higher critical gene expression may have a worse prognosis.
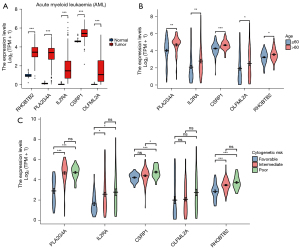
Five hub genes are associated with prognosis in AML
The ROC curves predicted the sensitivity and specificity of five hub genes, and the results showed that RHOBTB2 [area under the curve (AUC) =0.991], PLA2G4A (AUC =0.996), IL2RA (AUC =0.995), CSRP1 (AUC =0.880) and OLFML2A (AUC =0.977) all had a good prediction of AML prognosis sensitivity and specificity. (Figure 7A). Survival analysis of these 5 genes in AML showed that high expression of RHOBTB2 [hazard ratio (HR) =2.44, 95% CI: 1.57–3.78, P<0.001], PLA2G4A (HR =3.39, 95% CI: 2.14–5.36, P<0.001), IL2RA (HR =2.03, 95% CI: 1.31–3.13, P=0.001), CSRP1 (HR =2.36, 95% CI: 1.53–3.64, P<0.001), and OLFML2A (HR =2.36, 95% CI: 1.53–3.64, P<0.001) indicated poor prognosis (Figure 7B-7F).
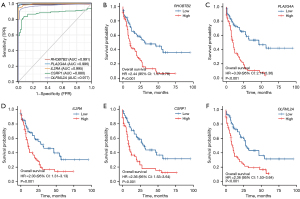
Construction of nomogram and evaluation of prognostic value
A nomogram containing multiple clinicopathological features were developed to evaluate the prognosis of AML patients. The nomogram has ten components: sex, age, peripheral blood (PB) blasts (%), cytogenetic risk, FLT3 mutation, and hub genes included in the risk score model (RHOBTB2, PLA2G4A, IL2RA, CSRP1, OLFML2A). The nomogram can be calculated and combined with each variable’s fraction to comprehensively predict AML patients’ prognosis (Figure 8A). The established nomogram C-index was 0.787. In summary, the predictive power of risk scores incorporating hub genes combined with clinical characteristics is more substantial than traditional clinical-only prediction approaches. The predictive accuracy of nomograms integrating multiple clinical information is the most pow robust. Similarly, predictive model fitting analysis (Figure 8B) and decision curve analysis (DCA) plot (Figure 8C) also demonstrated that our nomogram had better clinical application value in predicting the 1-, 3-, and 5-year prognosis of AML patients. The results indicated that the constructed nomogram model had better net benefits for AML patients.
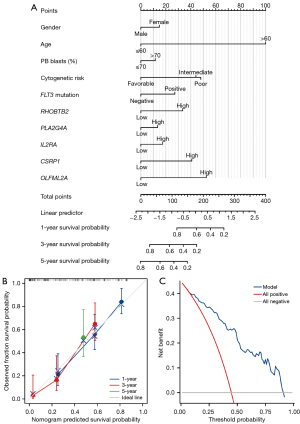
Specificity and sensitivity of hub genes in predicting 1, 3 or 5 years in AML patients
To assess the specificity and sensitivity of the five key genes in predicting 1, 3, and 5 years in AML patients, time-dependent ROC curve analysis was performed. The results showed that the 5 hub genes had good sensitivity and specificity in predicting 1-year prognosis (RHOBTB2, AUC =0.68; PLA2G4A, AUC =0.714; IL2RA, AUC =0.69; CSRP1, AUC =0.769; OLFML2A, AUC =0.718), 3-year prognosis (RHOBTB2, AUC =0.732; PLA2G4A, AUC =0.758; IL2RA, AUC =0.755; CSRP1, AUC =0.732; OLFML2A, AUC =0.682) and 5-year prognosis (RHOBTB2, AUC =0.802; PLA2G4A, AUC =0.851; IL2RA, AUC =0.78; CSRP1, AUC =0.763; OLFML2A, AUC =0.73) (Figure 9).
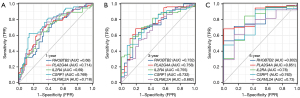
Molecular docking to search for drug molecules of 5 hub genes in AML
RHOBTB2, PLA2G4A, IL2RA, CSRP1, and OLFML2A molecules were targeted analysis to find effective drugs in AML patients, respectively, and found that drugs targeting these 5 hub genes may have some efficacy in AML (RHOBTB2—Piananghuang, PLA2G4A—Guadi, Huomaren, Difuzi, IL2RA—Fangji, Difengpi, Baiguo, CSRP1—Juye, Guijia, Biejia, OLFML2A—Fengfang, Mingdangshen, etc.) (Table S1). Further, drugs corresponding to hub genes as targets were selected in AML for analysis (PLA2G4A—Guadi, IL2RA—Fangji, CSRP1—Juye, and OLFML2A—Beiliujinu), respectively. The chemical composition of Guadi, Fangji, Juye, and Beiliujinu were obtained by analysis in the TCMSP database (Table 5). The drug with the largest OB (%) value was selected for molecular docking to validate the drug and target possibility. As a result, diosgenin could dock well with PLA2G4A (Figure 10A), beta-sitosterol could dock well with IL2RA (Figure 10B), and 3,4-di-O-caffeoylquinic acid could dock well with OLFML2A (Figure 10C). These reveals that these natural compounds may be efficacious in AML patients and provide appropriate targets.
Table 5
Gene | Medicine | Mol ID | Molecule name | MW | AlogP | OB (%) | Caco-2 | DL | FASA− | HL |
---|---|---|---|---|---|---|---|---|---|---|
PLA2G4A | Guadi | MOL004355 | Spinasterol | 412.77 | 7.64 | 42.98 | 1.44 | 0.76 | 0.21 | 5.32 |
MOL000546 | Diosgenin | 414.69 | 4.63 | 80.88 | 0.82 | 0.81 | 0.19 | 4.14 | ||
IL2RA | Fangji | MOL002333 | Tetraneurin A | 322.39 | 0.7 | 35.4 | 0.04 | 0.31 | 0.31 | 4.54 |
MOL000358 | Beta-sitosterol | 414.79 | 8.08 | 36.91 | 1.32 | 0.75 | 0.23 | 5.36 | ||
MOL002341 | Hesperetin | 302.3 | 2.28 | 70.31 | 0.37 | 0.27 | 0.33 | 15.78 | ||
CSRP1 | Juye | MOL005100 | 5,7-dihydroxy-2-(3-hydroxy-4-methoxyphenyl) chroman-4-one | 302.3 | 2.28 | 47.74 | 0.28 | 0.27 | 0.31 | 16.51 |
OLFML2A | Beiliujinu | MOL001733 | Eupatorin | 344.34 | 2.55 | 30.23 | 0.7 | 0.37 | 0.21 | 15.21 |
MOL000358 | Beta-sitosterol | 414.79 | 8.08 | 36.91 | 1.32 | 0.75 | 0.23 | 5.36 | ||
MOL000006 | Luteolin | 286.25 | 2.07 | 36.16 | 0.19 | 0.25 | 0.39 | 15.94 | ||
MOL008135 | 3,4-di-O-caffeoylquinic acid | 516.49 | 1.56 | 49.62 | −0.96 | 0.69 | 0.40 | 4.14 | ||
MOL008127 | Ermanin | 314.31 | 2.09 | 58.95 | 0.57 | 0.3 | 0.31 | 16.53 |
AlogP, lipid/water partition coefficient; Caco-2, intestinal epithelial permeability; CSRP1, cysteine and glycine-rich protein 1; DL, drug-likeness; FASA−, fractional water accessible surface area of all atoms with negative partial charge; HL, drug half-life; IL2RA, interleukin-2 receptor-α; MW, molecular weight; Mol, molecular; OB, oral bioavailability; OLFML2A, olfactomedin-like 2A; PLA2G4A, phospholipase A2.
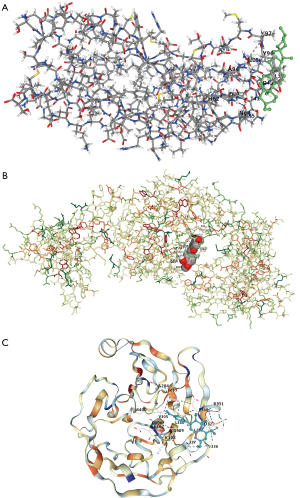
Discussion
AML, the most common acute leukemia in adults, accounts for approximately 80% of this group of diseases. In the United States, the incidence of AML is 3 to 5 per 100,000 people, and the incidence of AML increases with age (17). Combined chemotherapy, demethylation, hematopoietic stem cell transplantation, and targeted therapy are currently the primary treatment modalities based on patients’ clinical and genetic characteristics. Although advances in AML treatment have improved outcomes in younger patients, the prognosis of the elderly remains very poor, which accounts for the majority of new cases. Mutations in genes such as NPM1, CEBPA, RUNX1, FLT3, TP53, and ASXL1 play a vital role in the diagnosis, treatment, and guiding prognosis of AML (18-20). Molecular diagnosis allows individualized evaluation and treatment options for AML patients with different genetic characteristics. For example, combining small molecule inhibitors of FLT3, IDH1/IDH2, and BCL-2 with standard treatment can enhance anti-tumor activity and reduce drug resistance while providing new options for relapsed and refractory patients (21,22). Therefore, discovering new targets and developing new therapies are essential for improving the prognostic stratification and clinical efficacy of AML patients.
This current study screened 33 DEGs highly expressed in AML in both TCGA and GSE9476 datasets by bioinformatics. Through GO/KEGG functional analysis, hub genes were mainly involved in lysosome, extracellular matrix (ECM)-receptor interaction, and PI3K-Akt signaling pathway processes. The PI3K-Akt-mammalian target of the rapamycin (mTOR) pathway appears to be constitutively activated in 60% of AML patients, and this activation seems to be associated with reduced overall survival. PI3K is frequently activated in AML and contributes to the proliferation of blasts and leukemic progenitors (23,24). The selected differential genes in this study were also likely to be involved in the development of leukemia through the PI3K-Akt signaling pathway. Further, LASSO regression and multivariate Cox regression analysis revealed that RHOBTB2, PLA2G4A, IL2RA, CSRP1, and OLFML2A were important factors affecting the prognosis of AML. Clinical correlation and predictive analysis showed that the expression of these 5 hub genes were positively correlated with age older than 60 years, cytogenetic risk, and high expression were associated with poor prognosis. When these 5 hub genes were combined with clinical features into the prediction model, they were found to be of high value in predicting AML patients at 1, 3, and 5 years. To discover which of these 5 hub genes act on AML through which drugs, natural compounds were excavated that may affect AML patients through network pharmacology and molecular docking. RHOBTB2 is a candidate tumor suppressor located on human chromosome 8p21, a region commonly found in cancer (25). RHOBTB2 is an atypical Rho-GTPase with a conserved Rho-GTPase domain at the N-terminus followed by 2BTB domains that may be involved in protein interactions (26). The RHOBTB has been identified as a tumor suppressor and is reduced, eliminated, or mutated in various solid tumors. Studies have confirmed that RHOBTB2 plays an essential role in breast and colon cancer occurrence and development (27-29), and studies have also shown that high RHOBTB2 expression is associated with poor prognosis in AML patients (30), so drugs targeting RHOBTB2 have specific therapeutic prospects for treating AML patients. PLA2G4A belongs to the group IV phospholipase A2 family and hydrolyzes phospholipids, providing arachidonic acid as a rate-limiting substrate for prostaglandin production. Hassan et al. identified PLA2G4A as a poor prognostic marker and potential therapeutic target in HOXA9 and MEIS1-dependent AML (31), which is consistent with the results of the current study. At the same time, a natural compound in Guadi (diosgenin) was found that could perform molecular docking well with PLA2G4A, suggesting that the natural compound diosgenin may act through PLA2G4A in AML patients. L2RA is a low-affinity receptor for interleukin-2 (IL-2) that regulates proliferation, differentiation, apoptosis, stem cell-related properties, and leukemogenesis and is a potential therapeutic target for AML (32,33). This study found beta-sitosterol to be well-docked to IL2RA, providing a new option for targeting IL2RA therapy. Similarly, CSRP1 and OLFML2A play a role in the development of tumors, and in addition, overexpression of OLFML2A is associated with poor prognosis of extramedullary infiltration in AML (34-36). 3,4-di-O-caffeoylquinic acid also docked well with OLFML2A. Network pharmacology and molecular docking play a vital role in the drug development of natural compounds. The stable combination of natural compounds selected in this study with their corresponding targets PLA2G4A, IL2RA, and OLFML2A promotes the development and research of AML drugs. However, these 5 hub genes have different roles in different cancers, and only PLA2G4A and IL2RA have been validated for their function in AML (31,33).
This study finds that a predictive model composed of selected hub genes RHOBTB2, PLA2G4A, IL2RA, CSRP1, and OLFML2A is vital for guiding AML patient prognosis. It brings new ideas for the individualized treatment of AML patients. In addition, natural compounds with potential efficacy against AML were selected by molecular docking, which also provides new possibilities for the selection of subsequent drug studies.
However, there are some limitations to this study. First, the establishment and validation of this model are based on an existing public database, and more prospective studies are required to validate its clinical application. Second, further experiments need to explore the role of related molecules and corresponding natural compounds in AML. Overall, these finds need further confirmation in larger experimental and clinical studies.
Conclusions
In this study, a predictive model for AML patients was constructed based on public databases combined with bioinformatics, which has a high predictive value for the prognosis of AML. Natural compounds with potential efficacy against AML were discovered by molecular docking against selected hub genes. This study provides a new direction for establishing prediction models for AML patients and the research and development of precision medicine drugs.
Acknowledgments
Funding: None.
Footnote
Reporting Checklist: The authors have completed the TRIPOD reporting checklist. Available at https://tcr.amegroups.com/article/view/10.21037/tcr-22-2500/rc
Peer Review File: Available at https://tcr.amegroups.com/article/view/10.21037/tcr-22-2500/prf
Conflicts of Interest: All authors have completed the ICMJE uniform disclosure form (available at https://tcr.amegroups.com/article/view/10.21037/tcr-22-2500/coif). The authors have no conflicts of interest to declare.
Ethical Statement: The authors are accountable for all aspects of the work in ensuring that questions related to the accuracy or integrity of any part of the work are appropriately investigated and resolved. The study was conducted in accordance with the Declaration of Helsinki (as revised in 2013). Since the data involved in this study were obtained from the TCGA and GEO databases and in strict accordance with TCGA and GEO publication guidelines, no ethics committee approval was required.
Open Access Statement: This is an Open Access article distributed in accordance with the Creative Commons Attribution-NonCommercial-NoDerivs 4.0 International License (CC BY-NC-ND 4.0), which permits the non-commercial replication and distribution of the article with the strict proviso that no changes or edits are made and the original work is properly cited (including links to both the formal publication through the relevant DOI and the license). See: https://creativecommons.org/licenses/by-nc-nd/4.0/.
References
- Newell LF, Cook RJ. Advances in acute myeloid leukemia. BMJ 2021;375: [PubMed]
- Arber DA. The 2016 WHO classification of acute myeloid leukemia: What the practicing clinician needs to know. Semin Hematol 2019;56:90-5. [Crossref] [PubMed]
- Ferrara F, Schiffer CA. Acute myeloid leukaemia in adults. Lancet 2013;381:484-95. [Crossref] [PubMed]
- Craddock C. Acute myeloid leukaemia therapeutic innovation and clinical trials: past, present and future. Br J Haematol 2020;191:568-72. [PubMed]
- Thol F, Ganser A. Treatment of Relapsed Acute Myeloid Leukemia. Curr Treat Options Oncol 2020;21:66. [Crossref] [PubMed]
- Xin X, Xu Z, Wei J, et al. MiR-376a-3p increases cell apoptosis in acute myeloid leukemia by targeting MT1X. Cancer Biol Ther 2022;23:234-42. [Crossref] [PubMed]
- Wagner S, Vadakekolathu J, Tasian SK, et al. A parsimonious 3-gene signature predicts clinical outcomes in an acute myeloid leukemia multicohort study. Blood Adv 2019;3:1330-46. [Crossref] [PubMed]
- Sha K, Lu Y, Zhang P, et al. Identifying a novel 5-gene signature predicting clinical outcomes in acute myeloid leukemia. Clin Transl Oncol 2021;23:648-56. [Crossref] [PubMed]
- Walker CJ, Mrózek K, Ozer HG, et al. Gene expression signature predicts relapse in adult patients with cytogenetically normal acute myeloid leukemia. Blood Adv 2021;5:1474-82. [Crossref] [PubMed]
- Lai Y, Sheng L, Wang J, et al. A Novel 85-Gene Expression Signature Predicts Unfavorable Prognosis in Acute Myeloid Leukemia. Technol Cancer Res Treat 2021;20:15330338211004933. [Crossref] [PubMed]
- Ng SW, Mitchell A, Kennedy JA, et al. A 17-gene stemness score for rapid determination of risk in acute leukaemia. Nature 2016;540:433-7. [Crossref] [PubMed]
- Hopkins AL. Network pharmacology. Nat Biotechnol 2007;25:1110-1. [Crossref] [PubMed]
- Hopkins AL. Network pharmacology: the next paradigm in drug discovery. Nat Chem Biol 2008;4:682-90. [Crossref] [PubMed]
- Li S, Zhang B. Traditional Chinese medicine network pharmacology: theory, methodology and application. Chin J Nat Med 2013;11:110-20. [Crossref] [PubMed]
- Ru J, Li P, Wang J, et al. TCMSP: a database of systems pharmacology for drug discovery from herbal medicines. J Cheminform 2014;6:13. [Crossref] [PubMed]
- Pinzi L, Rastelli G. Molecular Docking: Shifting Paradigms in Drug Discovery. Int J Mol Sci 2019;20:4331. [Crossref] [PubMed]
- De Kouchkovsky I, Abdul-Hay M. 'Acute myeloid leukemia: a comprehensive review and 2016 update'. Blood Cancer J 2016;6:e441. [Crossref] [PubMed]
- Döhner H, Estey E, Grimwade D, et al. Diagnosis and management of AML in adults: 2017 ELN recommendations from an international expert panel. Blood 2017;129:424-47. [Crossref] [PubMed]
- Ranieri R, Pianigiani G, Sciabolacci S, et al. Current status and future perspectives in targeted therapy of NPM1-mutated AML. Leukemia 2022;36:2351-67. [Crossref] [PubMed]
- Romanova EI, Zubritskiy AV, Lioznova AV, et al. RUNX1/CEBPA Mutation in Acute Myeloid Leukemia Promotes Hypermethylation and Indicates for Demethylation Therapy. Int J Mol Sci 2022;23:11413. [Crossref] [PubMed]
- Döhner H, Wei AH, Löwenberg B. Towards precision medicine for AML. Nat Rev Clin Oncol 2021;18:577-90. [Crossref] [PubMed]
- Döhner H, Wei AH, Appelbaum FR, et al. Diagnosis and management of AML in adults: 2022 recommendations from an international expert panel on behalf of the ELN. Blood 2022;140:1345-77. [Crossref] [PubMed]
- Park S, Chapuis N, Tamburini J, et al. Role of the PI3K/AKT and mTOR signaling pathways in acute myeloid leukemia. Haematologica 2010;95:819-28. [Crossref] [PubMed]
- Nepstad I, Hatfield KJ, Grønningsæter IS, et al. The PI3K-Akt-mTOR Signaling Pathway in Human Acute Myeloid Leukemia (AML) Cells. Int J Mol Sci 2020;21:2907. [Crossref] [PubMed]
- Wilkins A, Ping Q, Carpenter CL. RhoBTB2 is a substrate of the mammalian Cul3 ubiquitin ligase complex. Genes Dev 2004;18:856-61. [Crossref] [PubMed]
- Freeman SN, Ma Y, Cress WD. RhoBTB2 (DBC2) is a mitotic E2F1 target gene with a novel role in apoptosis. J Biol Chem 2008;283:2353-62. [Crossref] [PubMed]
- Choi YM, Kim KB, Lee JH, et al. DBC2/RhoBTB2 functions as a tumor suppressor protein via Musashi-2 ubiquitination in breast cancer. Oncogene 2017;36:2802-12. [Crossref] [PubMed]
- Xu RS, Wu XD, Zhang SQ, et al. The tumor suppressor gene RhoBTB1 is a novel target of miR-31 in human colon cancer. Int J Oncol 2013;42:676-82. [Crossref] [PubMed]
- Zhang CS, Liu Q, Li M, et al. RHOBTB3 promotes proteasomal degradation of HIFα through facilitating hydroxylation and suppresses the Warburg effect. Cell Res 2015;25:1025-42. [Crossref] [PubMed]
- Liu P, Ma Q, Chen H, et al. Identification of RHOBTB2 aberration as an independent prognostic indicator in acute myeloid leukemia. Aging (Albany NY) 2021;13:15269-84. [Crossref] [PubMed]
- Hassan JJ, Lieske A, Dörpmund N, et al. A Multiplex CRISPR-Screen Identifies PLA2G4A as Prognostic Marker and Druggable Target for HOXA9 and MEIS1 Dependent AML. Int J Mol Sci 2021;22:9411. [Crossref] [PubMed]
- Flynn MJ, Hartley JA. The emerging role of anti-CD25 directed therapies as both immune modulators and targeted agents in cancer. Br J Haematol 2017;179:20-35. [Crossref] [PubMed]
- Nguyen CH, Schlerka A, Grandits AM, et al. IL2RA Promotes Aggressiveness and Stem Cell-Related Properties of Acute Myeloid Leukemia. Cancer Res 2020;80:4527-39. [Crossref] [PubMed]
- Weiskirchen R, Günther K. The CRP/MLP/TLP family of LIM domain proteins: acting by connecting. Bioessays 2003;25:152-62. [Crossref] [PubMed]
- Jin GH, Xu W, Shi Y, et al. Celecoxib exhibits an anti-gastric cancer effect by targeting focal adhesion and leukocyte transendothelial migration-associated genes. Oncol Lett 2016;12:2345-50. [Crossref] [PubMed]
- Lv C, Sun L, Guo Z, et al. Circular RNA regulatory network reveals cell-cell crosstalk in acute myeloid leukemia extramedullary infiltration. J Transl Med 2018;16:361. [Crossref] [PubMed]