Establishment and validation of a prognostic nomogram for patients with renal cell carcinoma based on SEER and TCGA database
Highlight box
Key findings
• In the current study, a novel prognostic nomogram based on large populations from the public databases was constructed.
What is known and what is new?
• The function of a nomogram can help to formulate the clinical outcomes and assist clinicians on clinical decision-making, previous models have been developed and applied for risk-stratification of RCC patients, but they were limited by small sample size or no external validation.
• In this study, a novel prognostic nomogram based on the data of 40,154 RCC patients from the SEER database was constructed and external validation was performed by 1,188 patients form the TCGA database.
What is the implication, and what should change now?
• This prognostic model could be a useful tool for clinicians to counsel patients, develop follow-up regimes, and identify appropriate patients for clinical trials.
Introduction
Renal cell carcinoma (RCC) is a main pathological category and accounts for over 90% of all kidney malignancies. According to the most recent report, estimated new cases and deaths from kidney and renal cancer made up a total of 79,000 and 13,920 in the United States and 77,410 and 46,345 in China (1,2). Although more and more patients are being diagnosed with early-stage disease as a result of increased imaging examinations, a substantial number of patients with RCC still suffer tumor recurrence or dissemination after curative treatments like radical or partial nephrectomy (3). RCC is insensitive to adjuvant chemoradiotherapy, and postoperative recurrence or metastasis commonly signifies a poor prognostic outcome and limited survival time (4). In recent years, some molecular-targeted drugs like tyrosine kinase inhibitors and immune checkpoint inhibitors have been recommended as first- to third-line treatments for advanced or metastatic RCC, but the survival benefits of these agents still need to be confirmed by more high-quality clinical trials (5,6).
Some prognostic factors, such as anatomical factors like the well-known tumor, node, and metastasis (TNM) staging system; histological factors including pathological subtype, tumor grade, microvascular invasion, tissue necrosis or sarcomatoid features, and perirenal invasion; baseline characteristics like age and sex; and a number of molecular biomarkers are reported to be closely associated with the prognostic outcomes of RCC patients (5,7). Unfortunately, approximately 20% of cases are complicated by metastatic disease at initial diagnosis, and over 30% of patients with localized RCC experience inevitably experience post-surgical tumor progression eventually (7,8). Thus, conducting risk-stratification accurately is crucial for clinicians to develop an individualized surveillance paradigm and follow-up strategies because a systematic surveillance protocol can bring an obvious survival benefit to patients with RCC (9).
The prognostic nomogram is an effective tool to integrate significant clinicopathological parameters and formulize the clinical outcome. The predictive function of a nomogram can help to formulate the clinical outcomes and assist clinicians in clinical decision-making (10,11). On the basis of these aforementioned clinical factors, several risk models have been developed and applied for risk-stratification of RCC patients, but all of them have limitations and shortcomings, such as small sample size or no external validation (12). In the current study, a novel prognostic nomogram was constructed and validated for predicting the overall survival (OS) of RCC patients on the basis of a large population from the Surveillance, Epidemiology, and End Results (SEER) database. We present this article in accordance with the TRIPOD reporting checklist (available at https://tcr.amegroups.com/article/view/10.21037/tcr-22-2692/rc).
Methods
Data source and patient selection
Data of RCC patients were downloaded from the SEER database (http://seer.cancer.gov), which is the largest publicly available cancer dataset and consists of 18 population-based cancer registries. In this study, the case listing was retrieved from the incidence dataset “SEER Research Plus Data, 17 Registries, Nov 2021 Sub (2000 to 2019)”. Meanwhile, we used SEER*Stat version 8.4.0 (http://www.seer.cancer.gov/seerstat) from the U.S. National Cancer Institute to review on the collected data. We searched for RCC patients diagnosed between 2010 to 2015 based on the 7th edition of the American Joint Committee on Cancer (AJCC) Cancer Staging Manual. Patients were selected for enrollment according to a series of inclusion criteria and exclusion criteria. The inclusion criteria were (I) the AYA site recode (2020 revision) was “carcinoma of kidney”, (II) the patient was diagnosed between 2010 to 2015, (III) the patient had complete AJCC stage and complete TNM stage information, and (IV) the patient had complete OS information. Separately. The exclusion criteria were (I) unknown race, (II) the surgical approach was coded 00, 10, 11, 12, 13, 14, 15, 16, 20, 21, 22, 23, 24, 25, 26, 27, 90, or 99, (III) the tumor size was unknown, (IV) the survival information was unknown. Finally, 40,154 patients who complied with the inclusion criteria were selected and included in the study. The study was conducted in accordance with the Declaration of Helsinki (as revised in 2013).
External validation cohort from The Cancer Genome Atlas (TCGA) database
The external validation information was downloaded from TCGA database (https://xenabrowser.net/datapages/). The download data includes: Genomic Data Commons (GDC) TCGA kidney chromophobe, GDC TCGA kidney clear cell carcinoma and GDC TCGA kidney papillary cell carcinoma. Only patients having adequate clinical information were selected. Eventually, 1,188 eligible patients were regarded as the external validation cohort. The selection flow diagram of all the patients is presented in Figure S1. Approval by the medical ethics committee and informed consent were waived in this study.
Definition of clinical variables
To establish an accurate predictive model, we collected the potential clinical variables associated with RCC prognosis in the SEER database. Demographic characteristics included age at diagnosis, gender, and race. Cancer information included tumor grade, AJCC stage, tumor size, pathological types. Survival information included survival months and survival status classification. Continuous variables including age and tumor size were transformed into the following categorical variables: age at diagnosis (<45, 45–59, 60–74, or ≥75 years) and tumor size (≤4, 4–7, or >7 cm). The other variables of interest were as follows: (I) race (white; black; or others, including American Indian, Alaskan Native, Asian, and Pacific Islander), (II) sex (male or female), (III) tumor grade (grades I and II or grades III and IV), (IV) AJCC stage (I, II, III, or IV), (V) pathological types (papillary, chromophobe, and clear cell). The primary endpoint was OS. Survival time was defined as the time from the initial diagnosis to death caused by any reasons or to the last follow-up.
Construction and validation of the nomogram
The development cohort (n=40,154) was adopted to establish the prognostic nomogram and risk-classification system and to perform the Kaplan-Meier subgroup survival analysis. First, univariate and multivariate Cox regression analyses were used to decide the prognostic factors in the development group. All results of Cox regression analysis were shown as hazards ratios (HRs) and 95% confidence intervals (CIs). Then, the independent prognostic factors were used to construct the nomogram and risk-classification system. Meanwhile, the validation cohort (n=1,188) was used for external validation. Finally, we used the Kaplan-Meier curves to analysis the prognosis of the subgroups. The nomogram was established to predict the 3- and 5-year OS. The discrimination was assessed by C-index and area under the receiver operating characteristic (ROC) curve values, and calibration was assessed by plotting validation curves in both the development cohort and validation cohort for 3- and 5-year survival, respectively. Eventually, patients were classified into low-, intermediate-, and high-risk groups based on their total scores of prognostic variables according to the nomogram, and survival analysis was also performed.
Statistical analysis
Summary statistics were used to describe the basic characteristics of selected RCC patients. The categorical differences between the development and validation groups were examined with the chi-squared test. Statistical analysis was completed using SPSS version 25.0 (IBM Corporation, Armonk, NY, USA) and R version 4.1.1 (R Foundation for Statistical Computing, Vienna, Austria). The R packages used in our study included the foreign, rms, survival, and ggplot2 packages. Statistical significance was determined as P<0.05 for both sides.
Results
Demographic characteristics of the selected patients
After screening, a total of 40,154 patients from SEER database met the inclusion criteria were regarded as development cohort, 1,188 patients from TCGA database were used as validation cohort. The median follow-up time was 69 [interquartile range (IQR), 52–91] months, and 39 (IQR, 19–65) months in the development cohort, and validation cohort respectively. In development cohort, nearly two-thirds of all patients were male (63.88%), the majority of patients were white (82.63%), and tumor grades I and II accounted for 63.79% of all RCC cases, the AJCC stage of 67.75% patients was stage I. In addition, almost 80% of patients were diagnosed with clear cell RCC both in SEER and TCGA database. The demographic characteristics of the development cohort, and validation cohort are displayed in Table 1.
Table 1
Parameters | Development cohort, n (%) | Validation cohort, n (%) | P value |
---|---|---|---|
Total number | 40,154 (100.00) | 1,188 (100.00) | |
Age | 0.001 | ||
<45 years | 4,121 (10.26) | 137 (11.53) | |
45–59 years | 13,392 (33.35) | 436 (36.70) | |
60–74 years | 17,422 (43.39) | 444 (37.37) | |
≥75 years | 5,219 (13.00) | 171 (14.39) | |
Sex | 0.255 | ||
Male | 25,650 (63.88) | 778 (65.49) | |
Female | 14,504 (36.12) | 410 (34.51) | |
Race | <0.001 | ||
Others* | 2,821 (7.03) | 25 (2.10) | |
White | 33,179 (82.63) | 1,063 (89.48) | |
Black | 4,154 (10.35) | 100 (8.42) | |
Tumor grade | <0.001 | ||
I and II | 25,614 (63.79) | 574 (48.32) | |
III and IV | 14,540 (36.21) | 614 (51.68) | |
AJCC stage | <0.001 | ||
I | 27,203 (67.75) | 608 (51.18) | |
II | 3,831 (9.54) | 129 (10.86) | |
III | 6,828 (17.00) | 278 (23.40) | |
IV | 2,292 (5.71) | 173 (14.56) | |
Tumor size (cm) | NA | ||
≤4 | 19,361 (48.22) | NA | |
>4–7 | 12,063 (30.04) | NA | |
>7 | 8,730 (21.74) | NA | |
Pathological types | <0.001 | ||
Papillary | 2,210 (5.50) | 133 (11.20) | |
Chromophobe | 6,093 (15.17) | 105 (8.84) | |
Clear cell | 31,851 (79.32) | 950 (79.97) |
SEER, Surveillance, Epidemiology, and End Results; TCGA, The Cancer Genome Atlas; RCC, renal cell carcinoma; *, Others: American Indian/AK Native, Asian/Pacific Islander; AJCC, American Joint Committee on Cancer; NA, not applicable.
Screening of the independent factors and survival analyses
Univariate and multivariate Cox regression analyses were applied to discover the independent prognostic factors of OS in the development cohort. The HR, 95% CI, and P values of every clinical variable are summarized in Table S1. The results of multivariate analysis revealed that age [45–59 years (HR, 1.875; 95% CI: 1.668–2.107; P<0.001), 60–74 years (HR, 3.074; 95% CI: 2.745–3.443; P<0.001) and ≥75 years (HR, 6.094; 95% CI: 5.421–6.849; P<0.001), refer to <45 years], sex [female (HR, 0.900; 95% CI: 0.862–0.939; P<0.001), refer to male], tumor grade [grades III and IV (HR, 1.459; 95% CI: 1.398–1.523; P<0.001), refer to grades I and II], AJCC stage [stage III (HR, 1.518; 95% CI: 1.426–1.616; P<0.001) and stage IV (HR, 5.133; 95% CI: 4.757–5.540; P<0.001), refer to stage I], tumor size [4–7 cm (HR, 1.372; 95% CI: 1.302–1.446; P<0.001) and >7 cm (HR, 1.899; 95% CI: 1.761–2.048; P<0.001), refer to ≤4 cm], and pathological types [chromophobe (HR, 1.739; 95% CI: 1.549–1.953; P<0.001), and clear cell (HR, 1.468; 95% CI: 1.320–1.633; P<0.001) refer to papillary] were crucial prognostic factors of the OS of RCC patients (Figure 1). Subsequently, Kaplan-Meier analyses and long-rank tests also indicated that the subgroups of these variables were closely associated with the survival of RCC (Figure 2).
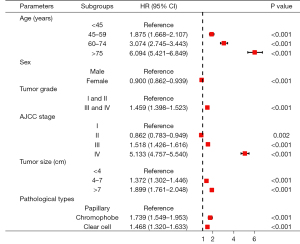
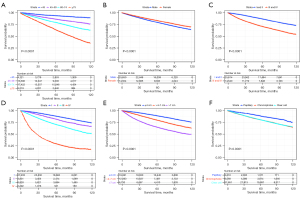
Construction and validation of the prognostic nomogram
On the basis of the results of multivariate Cox regression analysis, a prognostic nomogram composed of six variables was established (Figure 3). In the nomogram, AJCC stage, patients’ age, and tumor size were the top three vital parameters that contributed to the survival outcome of RCC patients, followed by pathological types, tumor grade, and sex. We then evaluated the discrimination and calibration of the nomogram using ROC curves, C-index values, and calibration plots (Figure 4). The area under the ROC curve values of 3- and 5-year survival were 0.785 and 0.769 in the development group (Figure 4A) and 0.786 and 0.763 in the validation group (Figure 4C). Meanwhile, the C-index was 0.746 (95% CI: 0.740–0.752) in the development set and 0.763 (95% CI: 0.738-0.788) in the validation set, indicating that our nomogram had relatively good discrimination. Moreover, 3- and 5-year calibration plots in the development cohort (Figure 4B) and validation cohort (Figure 4D) demonstrated that the predicted survival probabilities were almost consistent with the observed outcome.
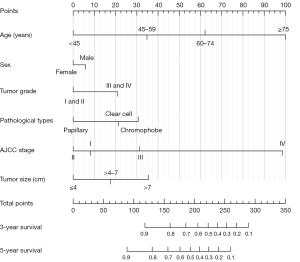
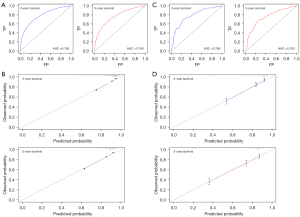
Stratification according to the total risk points
In order to establish a risk group of the RCC patients with the constructed nomogram, each subgroup of the six independent variables was assigned an exact point according to the nomogram and the total points were calculated and adopted for risk-stratification (Figure 5A). Then, patients were classified into a low-risk group (total points ≤5), intermediate-risk group (total points 6–9), or a high-risk group (total points ≥10) (Figure 5B). Kaplan-Meier survival analysis indicated a significant difference in the OS outcomes among the three risk groups in both the development cohort (Figure 5C) and validation cohort (Figure 5D).
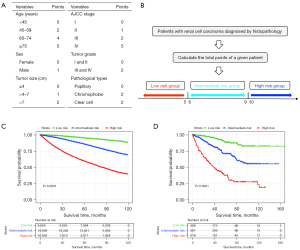
Discussion
RCC is the third-most common cancer among urological malignancies and arises from the renal tubular epithelium (13). The prognosis of RCC patients depends on many factors, but all of these related factors can be classified into three categories according to previous research (5,14). The first category is baseline characteristics, including age, sex, race, clinical symptoms, and blood biochemical values. Numerous studies have revealed that the baseline information is closely associated with the prognosis of RCC patients, but the influence of these values in different populations is inconsistent (15,16). For example, male patients experience poor survival in Western countries, whereas no such discrepancy according to sex is found among Chinese patients (16). The second category is anatomical prognostic factors, such as the AJCC stage and the TNM classification system, which are authoritative and have been strongly recommended by various guidelines (17). T stage also contains information about the tumor size; venous tumor thrombus; and invasion of the adrenal glands, renal capsule, and collecting system. All these features have a significant impact on the prognosis of RCC patients (18). The last category is histological prognostic factors, which include tumor grade and some unfavorable histopathological features like sarcomatoid differentiation, tumor necrosis, microvascular invasion, and extension to perirenal fat (19,20). In terms of tumor grade, the four-tiered Fuhrman grading system, and the World Health Organization/International Society of Urological Pathology grading system [2012] have been widely applied (21,22). Obviously, a poor 5-year survival rate can be seen among patients with grade III or IV RCC compared to those with grade I or II disease (18). In the current study, the information of patients who underwent partial or radical nephrectomy was downloaded from seer database, the baseline characteristics (age, and sex), anatomical factors (AJCC stage and tumor size), and histological factors (tumor grade, pathological types) were analyzed, and univariate and multivariate analyses again proved that all of them were independent predictors of OS of RCC patients.
Other than clinical factors, small molecular biomarkers have also shown enormous potential in the diagnosis, treatment, and prognosis of RCC. Previous studies have demonstrated that the gene-expression profile, germline mutations, epigenetic modifications, proteomics, metabolomics, and immune microenvironment are closely associated with the development and progression of RCC (23-25). For example, the inactivation of the VHL, PTEN, and PI3K/AKT/mTOR pathways has been explored extensively. The gene-expression levels of BAP1 and PBRM1 have also been verified as independent prognostic factors of RCC recurrence (14,26). Recently, blood- or urine-based biomarkers (liquid biopsy), such as circulating tumor DNA, RNA, and extracellular vesicles have emerged as novel biomarkers tested via non-invasive examinations, and the detection of these molecular markers is also helpful for risk-stratification and patient counseling (27). We believe that combining molecular markers and modern prognostic models will definitely improve the prediction accuracy, but future studies are still needed before putting them into clinical practice.
At present, there are number of patients who have advanced or metastatic RCC at the time of diagnosis. Current treatment strategies for these patients include molecular targeted therapy, immunotherapy, surgical resection, and palliative radiotherapy (28). With the use of immune checkpoint inhibitors, immunotherapy has provided a significant survival benefit in patients with advanced or metastatic RCC (29). A study has shown first-line immunotherapy and combination targeted therapy and immunotherapy were associated with improved OS for patients with metastatic RCC (30). Unfortunately, the information on drug therapies is missing due to the limitations of the database and cannot be analyzed in this study.
In the past two decades, several prognostic models for estimating the survival events of patients with RCC had been constructed, including UISS [2001], SSIGN [2002], the Leibovich score [2003], the GRANT score [2017], the Leibovich score [2018], and the VENUSS score [2019] (31-36). All these models present acceptable prediction accuracies in their original populations, but the incorporated factors, method of risk-stratification, and applicable pathological subtypes of these models are not the same (37). A head-to-head study performed by Rosiello et al. showed that there exists a non-negligible difference in the prediction accuracy of these prognostic models, and the use of these prognostic nomograms for developing postoperative follow-up strategies must be histology-specific, with the prognostic risk class stratified by the appropriate prognostic model (38). External validation of UISS, SSIGN, and the Leibovich score was also performed recently in a Chinese cohort, but only the SSIGN model exhibited an intermediate degree of predictive accuracy, which means that regional and national discrepancies are also vital factors that cannot be neglected when using these prognostic models to predict the survival outcomes of RCC patients (39).
This study has several limitations that must be considered. First, the data used in this study were derived from the public database, and an external validation of the nomogram constructed in this study by a cohort of patients from different countries, especially eastern countries is necessary. Second, pathological subtype plays a vital role in patient prognosis (40), but this study did not include all RCC types because of limited patients. Furthermore, the details of some critical histological factors, such as microvascular invasion, tissue necrosis, and perirenal invasion, were also unavailable from the SEER database, yet these factors are also related to the prognosis of RCC patients too. Finally, this was a retrospective study, and prospective consolidation is indispensable.
Conclusions
RCC is a fatal urinary malignancy, and patients with this disease would receive great benefits from accurate prediction schemes. Previous prognostic models have shown good performance, but they are heterogeneous in their scope of applicability. In the present study, a novel nomogram based on a large population from the public database was constructed, which could be a tool for clinicians to counsel patients, develop follow-up regimes, and identify appropriate patients for clinical trials.
Acknowledgments
Funding: This study was supported partly by
Footnote
Reporting Checklist: The authors have completed the TRIPOD reporting checklist. Available at https://tcr.amegroups.com/article/view/10.21037/tcr-22-2692/rc
Peer Review File: Available at https://tcr.amegroups.com/article/view/10.21037/tcr-22-2692/prf
Conflicts of Interest: All authors have completed the ICMJE uniform disclosure form (available at https://tcr.amegroups.com/article/view/10.21037/tcr-22-2692/coif). The authors have no conflicts of interest to declare.
Ethical Statement: The authors are accountable for all aspects of the work in ensuring that questions related to the accuracy or integrity of any part of the work are appropriately investigated and resolved. The study was conducted in accordance with the Declaration of Helsinki (as revised in 2013).
Open Access Statement: This is an Open Access article distributed in accordance with the Creative Commons Attribution-NonCommercial-NoDerivs 4.0 International License (CC BY-NC-ND 4.0), which permits the non-commercial replication and distribution of the article with the strict proviso that no changes or edits are made and the original work is properly cited (including links to both the formal publication through the relevant DOI and the license). See: https://creativecommons.org/licenses/by-nc-nd/4.0/.
References
- Siegel RL, Miller KD, Fuchs HE, et al. Cancer statistics, 2022. CA Cancer J Clin 2022;72:7-33. [Crossref] [PubMed]
- Xia C, Dong X, Li H, et al. Cancer statistics in China and United States, 2022: profiles, trends, and determinants. Chin Med J (Engl) 2022;135:584-90. [Crossref] [PubMed]
- Bahadoram S, Davoodi M, Hassanzadeh S, et al. Renal cell carcinoma: an overview of the epidemiology, diagnosis, and treatment. G Ital Nefrol 2022;39:2022-vol3. [PubMed]
- Hahn AW, Klaassen Z, Agarwal N, et al. First-line Treatment of Metastatic Renal Cell Carcinoma: A Systematic Review and Network Meta-analysis. Eur Urol Oncol 2019;2:708-15. [Crossref] [PubMed]
- Ljungberg B, Albiges L, Abu-Ghanem Y, et al. European Association of Urology Guidelines on Renal Cell Carcinoma: The 2022 Update. Eur Urol 2022;82:399-410. [Crossref] [PubMed]
- Garcia J, Hurwitz HI, Sandler AB, et al. Bevacizumab (Avastin®) in cancer treatment: A review of 15 years of clinical experience and future outlook. Cancer Treat Rev 2020;86:102017. [Crossref] [PubMed]
- Mattila KE, Vainio P, Jaakkola PM. Prognostic Factors for Localized Clear Cell Renal Cell Carcinoma and Their Application in Adjuvant Therapy. Cancers (Basel) 2022;14:239. [Crossref] [PubMed]
- Capitanio U, Montorsi F. Renal cancer. Lancet 2016;387:894-906. [Crossref] [PubMed]
- Powles T, Albiges L, Staehler M, et al. Updated European Association of Urology Guidelines: Recommendations for the Treatment of First-line Metastatic Clear Cell Renal Cancer. Eur Urol 2018;73:311-5. [Crossref] [PubMed]
- Tao T, Wang C, Liu W, et al. Construction and Validation of a Clinical Predictive Nomogram for Improving the Cancer Detection of Prostate Naive Biopsy Based on Chinese Multicenter Clinical Data. Front Oncol 2022;11:811866. [Crossref] [PubMed]
- Zheng X, Guo K, Wasan HS, et al. A population-based study: how to identify high-risk T1 gastric cancer patients? Am J Cancer Res 2021;11:1463-79. [PubMed]
- Patel SH, Singla N, Pierorazio PM. Contemporary Prognostic Model for Renal Cell Carcinoma: Is it Time for Biomarkers? Eur Urol 2021;80:32-3. [Crossref] [PubMed]
- Singh D. Current updates and future perspectives on the management of renal cell carcinoma. Life Sci 2021;264:118632. [Crossref] [PubMed]
- Klatte T, Rossi SH, Stewart GD. Prognostic factors and prognostic models for renal cell carcinoma: a literature review. World J Urol 2018;36:1943-52. [Crossref] [PubMed]
- Yue G, Deyu L, Lianyuan T, et al. Clinical features and prognostic factors of patients with metastatic renal cell carcinoma stratified by age. Aging (Albany NY) 2021;13:8290-305. [Crossref] [PubMed]
- Zhu Y, Zhao YR, Yang XF, et al. Postoperative prognostic model for patients with clear cell renal cell carcinoma in a Chinese population. Int J Urol 2019;26:624-9. [Crossref] [PubMed]
- Swami U, Nussenzveig RH, Haaland B, et al. Revisiting AJCC TNM staging for renal cell carcinoma: quest for improvement. Ann Transl Med 2019;7:S18. [Crossref] [PubMed]
- Cheaib JG, Patel HD, Johnson MH, et al. Stage-specific conditional survival in renal cell carcinoma after nephrectomy. Urol Oncol 2020;38:6.e1-7. [Crossref] [PubMed]
- Delahunt B, Eble JN, Samaratunga H, et al. Staging of renal cell carcinoma: current progress and potential advances. Pathology 2021;53:120-8. [Crossref] [PubMed]
- Bedke J, Heide J, Ribback S, et al. Microvascular and lymphovascular tumour invasion are associated with poor prognosis and metastatic spread in renal cell carcinoma: a validation study in clinical practice. BJU Int 2018;121:84-92. [Crossref] [PubMed]
- Delahunt B, Eble JN, Egevad L, et al. Grading of renal cell carcinoma. Histopathology 2019;74:4-17. [Crossref] [PubMed]
- Delahunt B, Cheville JC, Martignoni G, et al. The International Society of Urological Pathology (ISUP) grading system for renal cell carcinoma and other prognostic parameters. Am J Surg Pathol 2013;37:1490-504. [Crossref] [PubMed]
- Rodrigues D, Monteiro M, Jerónimo C, et al. Renal cell carcinoma: a critical analysis of metabolomic biomarkers emerging from current model systems. Transl Res 2017;180:1-11. [Crossref] [PubMed]
- Clark DJ, Dhanasekaran SM, Petralia F, et al. Integrated Proteogenomic Characterization of Clear Cell Renal Cell Carcinoma. Cell 2019;179:964-983.e31. [Crossref] [PubMed]
- Marchioni M, Rivas JG, Autran A, et al. Biomarkers for Renal Cell Carcinoma Recurrence: State of the Art. Curr Urol Rep 2021;22:31. [Crossref] [PubMed]
- Joseph RW, Kapur P, Serie DJ, et al. Clear Cell Renal Cell Carcinoma Subtypes Identified by BAP1 and PBRM1 Expression. J Urol 2016;195:180-7. [Crossref] [PubMed]
- Bergerot PG, Hahn AW, Bergerot CD, et al. The Role of Circulating Tumor DNA in Renal Cell Carcinoma. Curr Treat Options Oncol 2018;19:10. [Crossref] [PubMed]
- Dutcher JP, Flippot R, Fallah J, et al. On the Shoulders of Giants: The Evolution of Renal Cell Carcinoma Treatment-Cytokines, Targeted Therapy, and Immunotherapy. Am Soc Clin Oncol Educ Book 2020;40:1-18. [Crossref] [PubMed]
- Motzer RJ, Escudier B, McDermott DF, et al. Nivolumab versus Everolimus in Advanced Renal-Cell Carcinoma. N Engl J Med 2015;373:1803-13. [Crossref] [PubMed]
- Chakiryan NH, Jiang DD, Gillis KA, et al. Real-World Survival Outcomes Associated With First-Line Immunotherapy, Targeted Therapy, and Combination Therapy for Metastatic Clear Cell Renal Cell Carcinoma. JAMA Netw Open 2021;4:e2111329. [Crossref] [PubMed]
- Zisman A, Pantuck AJ, Dorey F, et al. Improved prognostication of renal cell carcinoma using an integrated staging system. J Clin Oncol 2001;19:1649-57. [Crossref] [PubMed]
- Frank I, Blute ML, Cheville JC, et al. An outcome prediction model for patients with clear cell renal cell carcinoma treated with radical nephrectomy based on tumor stage, size, grade and necrosis: the SSIGN score. J Urol 2002;168:2395-400. [Crossref] [PubMed]
- Leibovich BC, Blute ML, Cheville JC, et al. Prediction of progression after radical nephrectomy for patients with clear cell renal cell carcinoma: a stratification tool for prospective clinical trials. Cancer 2003;97:1663-71. [Crossref] [PubMed]
- Buti S, Puligandla M, Bersanelli M, et al. Validation of a new prognostic model to easily predict outcome in renal cell carcinoma: the GRANT score applied to the ASSURE trial population. Ann Oncol 2017;28:2747-53. [Crossref] [PubMed]
- Leibovich BC, Lohse CM, Cheville JC, et al. Predicting Oncologic Outcomes in Renal Cell Carcinoma After Surgery. Eur Urol 2018;73:772-80. [Crossref] [PubMed]
- Klatte T, Gallagher KM, Afferi L, et al. The VENUSS prognostic model to predict disease recurrence following surgery for non-metastatic papillary renal cell carcinoma: development and evaluation using the ASSURE prospective clinical trial cohort. BMC Med 2019;17:182. [Crossref] [PubMed]
- Gerald T, Margulis V. Variability in prognostic models for localized renal cell carcinoma. Nat Rev Urol 2022;19:385-6. [Crossref] [PubMed]
- Rosiello G, Larcher A, Fallara G, et al. Head-to-head comparison of all the prognostic models recommended by the European Association of Urology Guidelines to predict oncologic outcomes in patients with renal cell carcinoma. Urol Oncol 2022;40:271.e19-27. [Crossref] [PubMed]
- Shao Y, Xiong S, Sun G, et al. Prognostic analysis of postoperative clinically nonmetastatic renal cell carcinoma. Cancer Med 2020;9:959-70. [Crossref] [PubMed]
- Nguyen DP, Vertosick EA, Corradi RB, et al. Histological subtype of renal cell carcinoma significantly affects survival in the era of partial nephrectomy. Urol Oncol 2016;34:259.e1-8. [Crossref] [PubMed]