Screening of novel biomarkers for breast cancer based on WGCNA and multiple machine learning algorithms
Highlight box
Key findings
• This study identified DIXDC1, DUSP6, PDK4, CXCL12, IRF7, ITGA7, NEK2, and NR3C1 as potential diagnostic biomarkers for breast cancer, with NEK2 having the highest potential to aid in diagnosis and prognosis in clinical settings.
What is known and what is new?
• WGCNA, LASSO analysis, SVM-REF analysis, and random forest model are commonly used in bioinformatics to identify prospective biomarkers in tumor and nontumor diseases to support doctors to predict prognosis and response to therapy.
• This study for the first time combined WGCNA and three machine learning algorithms to identify NEK2, a potential biomarker for breast cancer, and predicted drugs targeting NEK2 including etoposide and luciferone.
What is the implication, and what should change now?
• NEK2 may be considered as a new biomarker for the diagnosis and prognosis of breast cancer, helping clinicians to focus on altered metabolic pathways in breast cancer as well as providing new ideas for immunotherapy of breast cancer.
Introduction
Breast cancer (BC) is the most prevalent malignancy in women, accounting for approximately 11.7% of all cancers, and poses a serious threat to patients’ lives and makes prevention and control critical situation. Although improvements in timely recognition and treatment have recently reduced BC mortality, BC metastasis and related complications remain the leading cause of death. The mechanism of BC metastasis has not been fully elucidated in clinical practice to date. Study has shown that once distant metastases occur in BC patients, the 5-year survival rate is dramatically reduced from over 90% to 25% (1). Therefore, early detection and prognostic assessment is the best way to prevent and treat BC dissemination. With the advancement of bioinformatics, it is now possible to investigate the underlying mechanisms of BC incidence and progression and identify novel targets and therapeutic approaches to optimize BC patients’ survival rates.
With the remarkable development of sequencing technologies, bioinformatics has gained significant applications in several fields, especially in medicine. Bioinformatics is most commonly used in medical research to identify prospective biomarkers in tumor and nontumor diseases to support doctors to predict prognosis and response to therapy (2,3). For example, Weighted Gene Coexpression Network Analysis (WGCNA) combined with Support Vector Machine-Recursive Elimination Feature (SVM-REF), Least Absolute Shrinkage and Selection Operator (LASSO) regression models, and Gaussian mixture model (GMM) algorithms identified early diagnostic genes for tendinopathy (4). Moreover, WGCNA combined with the SVM-REF model with Systemic Lupus Erythematosus Disease Activity Index (SLEDAI) correlation, random forest models, and differential gene analysis identified MX2 as an original immunological biomarker for Systemic Lupus Erythematosus (5). However, WGCNA and machine learning have not been addressed in the majority of bioinformatics studies for breast malignancy.
In order to evaluate prospective biomarkers in BC, the study combined WGCNA with three machine learning algorithms, including the LASSO analysis, SVM-REF analysis, and random forest model. For the differentially expressed gene (DEG) and WGCNA hub module gene analyses, transcriptional data were first obtained from The Cancer Genome Atlas (TCGA) database. Crossover genes between the two were identified as key objectives associated with BC and were functionally analyzed. Subsequently, the three machine learning algorithms mentioned above were used to identify prospective biomarkers in order to investigate the function and regulatory mechanisms of these genes. In conclusion, the results of this study could assist in the identification of key genes regarding BC diagnosis and prognosis and to discover new therapeutic options. Figure 1 shows the overall study’s flowchart. We present this article in accordance with the STREGA reporting checklist (available at https://tcr.amegroups.com/article/view/10.21037/tcr-23-3/rc).
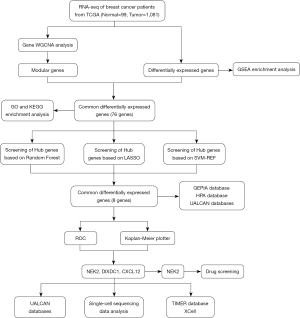
Methods
Data collection
Transcriptomic RNA data and survival data were obtained from TCGA for 1,180 samples (including 1,081 breast tumor samples and 99 breast normal samples). The GSE15852 dataset (including 43 breast tumor samples and 43 breast normal samples) was obtained from the Gene Expression Omnibus database (GEO). The databases were as follows: The University of ALabama at Birmingham CANcer (UALCAN) database (http://ualcan.path.uab.edu/), Tumor Immune Estimation Resource (TIMER) database (https://cistrome.shinyapps.io/timer/), Human Protein Atlas (HPA) database (https://www.proteinatlas.org/), STRING2 database (https://string-db.org/), KM Plotter (https://kmplot.com/analysis/), Gene Expression Profiling Interactive Analysis (GEPIA) dataset (http://gepia.cancer-pku.cn/), CancerSEA (http://biocc.hrbmu.edu.cn/CancerSEA/home.jsp) and Enrichr (https://maayanlab.cloud/Enrichr/). The study was conducted in accordance with the Declaration of Helsinki (as revised in 2013).
Identification and functional enrichment analysis of DEGs in BC patients
The raw data from the TCGA dataset were corrected and normalized in R using log2, and duplicated genes were removed. Bioconductor platform gene annotation files (http://www.bioconductor.org/) were used to probe the matrix (6). Identification of DEGs and gene set enrichment analysis (GSEA) functional analysis was performed using the R packages “limma” and “GSEABase”. |log2 FC| >1 and P value <0.05 were screened as DEGs, and the data were visualized by using the R package ggplot2 to plot volcanoes (7).
Screening of target genes by WGCNA
The WGCNA package is a widely used open-source technology for creating unsigned co-expression networks to precisely locate co-expression modules, which mainly includes four steps: calculation of correlation coefficients between genes, determination of gene modules, co-expression network, and association between modules and traits. The correlation matrix is constructed using Pearson correlation coefficients, and then it is transformed into a weighted adjacency matrix using a soft threshold function. A soft connectivity algorithm was used to calculate scale independence and mean connectivity for different powers in order to achieve a balanced coexpression network between them. The adjacency matrix is transformed into a topological overlap matrix (TOM). The gene clusters were classified into coexpression modules with a depth partition value of 2 using 1-tom as a distance measure. The gene’s weighted correlation coefficient is used to classify genes based on their expression patterns, and genes with similar patterns are grouped into the same module. To determine the association between coexpression modules and clinical features, the scale-free topology criterion was used to estimate the “soft” threshold power (β), and the tree was divided into modules using a dynamic shearing method (the minimum number of genes is 50) and the height was reduced to 0.3, with 0.25 set as the merging threshold (cut height) at which modules were merged (modules with correlations higher than 0.75 were merged). The feature gene network was then shown after we computed gene significance (GS), module affiliation (MM), and correlation modules with clinical characteristics. Finally, to identify prospective gene targets for BC, an intersection between WGCNA-derived module genes associated with BC development and DEGs was performed.
Gene Ontology (GO), Disease Ontology (DO), and Kyoto Encyclopedia of Genes and Genomes (KEGG) analysis
The R package ClusterProfiler was used to calculate GO analysis, DO, and KEGG pathway enrichment analysis. Enrichment analysis of prospective targets was performed by using a P value of 0.05 as a filtering criterion. Subsequently, GO analyses were performed to determine biological processes (BPs), molecular functions (MFs), and cellular components (CCs) associated with hub genes. Moreover, KEGG enrichment analysis was performed to identify prospective target-enriched signaling pathways.
Protein-protein interaction network construction
The STRING2 database is a protein interaction database for the analysis of interactions between known and predicted proteins. The STRING2 database was utilized to create interaction networks of prospective targets. Finally, the network’s genes were selected for biomarker screening after being determined to be crucial in the pathological development of BC.
Screening biomarkers for BC by machine learning algorithms
In this work, three machine learning methods were employed. First, Lasso logistic regression is a machine learning method that identifies variables by finding the value of λ that minimizes the classification error (8). Support Vector Machine-Recursive Feature Elimination (SVM-RFE) is a support vector machine-based machine learning method that finds the best variables by subtracting the feature vectors generated by SVM. LASSO regression analysis was performed using the R package “glmnet”. The “svmRFE” function was utilized to compute the data and the R package “e1071” to remove the recursive features of the resulting. Then, the Lapply function was used to do feature ranking overall training sets. In order to minimize error rates and provide the best gene signature, the “top.features” function was used to acquire all compressed top features and the loop function to estimate the generalization error for the various numbers of top features using the 10× coefficient of variation (CV) criterion. Finally, a random forest analysis was performed using the R package “randomForest”, and 38 genes with importance greater than 1 were retained. The intersection genes discovered by the three analyses were regarded as prospective biomarkers for BC patients.
Gene expression analysis and protein expression analysis
Using the GEPIA dataset, the expression of eight biomarker mRNAs was compared in BC and breast tissues. These features were confirmed in the UALCAN databases. Protein expression of the eight genes in BC was obtained through the HPA database.
Correlation of prospective biomarkers and analysis of their prognostic ability
The R package “corrplot” was used to conduct a correlation analysis of prospective biomarker expression. R package “pROC” was used to perform receiver operating characteristic (ROC) analysis on the TCGA dataset and the area under curve (AUC) values were calculated to see if prospective biomarkers could well distinguish between cancerous and normal tissues. Additionally, the online Kaplan-Meier mapper tool was used to assess the prognostic capabilities of biomarkers for BC patients in the database.
Correlation of transcript levels of NEK2, DIXDC1 and CXCL12 with molecular subtypes and tumor stage of BC and gene set enrichment analysis
Using the UALCAN databases to analyze the correlation of transcript levels of NEK2, DIXDC1 and CXCL12 with molecular subtypes and tumor stage of BC. Furthermore, gene enrichment analysis was performed.
Single cell sequencing data analysis
CancerSEA is a database dedicated to deciphering the functional state of cancer cells at the single-cell level. The application of CancerSEA aids in the understanding of the biological pathways causing tumor cell functional heterogeneity. Based on the single cell sequencing data from CancerSEA, the correlation data of NEK2, DIXDC1, and CXCL12 expression with various tumor functional states were downloaded and the heat map was plotted. All individual cell t-Distributed Stochastic Neighbor Embedding (t-SNE) maps were downloaded straight from the CancerSEA website.
Correlation of NEK2 with immune cell infiltration in BC
Correlation analysis of NEK2 with different molecular types of BC immune cells was performed by the TIMER2.0 database. Furthermore, the relationship between NEK2 and immune infiltration in BC was explored using the R package “xCell”.
Prospective drug screening
Novel therapeutic agents are indispensable since metastatic BC (MBC) still lacks significantly effective treatments. Based on the research results herein, we identified biomarkers and searched the Drug Signature Database (DSigDB) on the enrihr5 website for prospective biomarker-related drug predictions. In addition, the PubChem6 database was used to screen and visualize the structures of the related drugs.
Statistical analysis
All statistical analyses were performed by using R software (version 4.2.1, https://www.r-project.org/). Group comparisons were conducted using Student’s t-test, Wilcoxon test, or one-way analysis of variance (ANOVA). Statistical significance was set at P<0.05, and significance levels were denoted as *P<0.05, **P<0.01, ***P<0.001, and ****P<0.0001. Non-significant differences were denoted as “ns”.
Results
Identification of DEGs in patients with BC
Samples were first normalized to obtain 1,673 DEGs in tissues from BC patients; 1,069 of these DEGs were downregulated, and 604 were upregulated (Figure 2A). The expression of the top 30 genes that differed most between cancerous and normal tissues is visualized in Figure 2B. Finally, 1,673 DEGs of GSEA produced DNA replication, cytochrome P450, fatty acid degradation, homologous recombination, peroxisome proliferator-activated receptor (PPAR) signaling path-way, proteasome, Janus kinase-signal transducer and activator of transcription (JAK-STAT) signaling pathway and so on (Figure 2C).
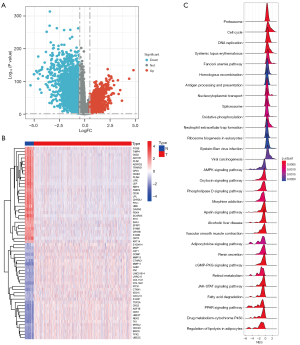
WGCNA-based hub gene screening
During the clustering process, no outliers were found. The lowest soft threshold for creating the scale-free network was then obtained by setting the scale-free fit index to 0.9 (Figure 3A). Subsequently, we integrated the modules with feature factors more than 0.75 in the clustering tree results and found 16 modules in the gene expression tree graph and the correlation heat map between module characteristics (Figure 3B). MM denotes the relationship between module gene expression levels and module signature genes (MEs). GS denotes the relationship between samples and module genes. Modules were correlated with clinical characteristics by calculating MM and GS values. The midnight blue and purple modules were most associated with BC, with ModuleTraitCor =0.65 and ModuleTraitPvalue =2E-05 in midnight blue and ModuleTraitCor =0.58 and ModuleTraitPvalue =2E-04 in purple (Figure 3C). Finally, the midnight blue and purple module genes intersected with DEGs to generate 76 prospective targets associated with breast malignancy (Figure 3D).
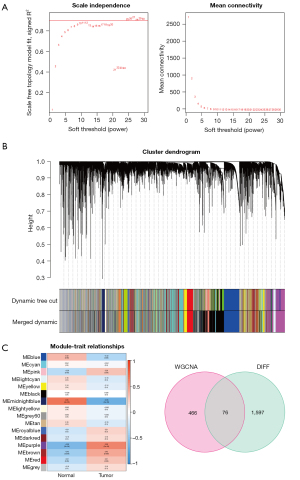
GO, DO, and KEGG analysis
Enrichment analysis was used to investigate the biological functions of 76 prospective genes generated by DEGs mapped to WGCNA module genes. GO analysis showed that these genes had numerous functions, such as RNA polymerase II specific, DNA-binding transcription activator activity, and chemokine receptor binding. Additionally, they were linked to many CCs, including the transcription regulator complex, midbody, and phosphatase complex, and were involved in various BPs, such as cytokine-mediated signaling pathways, response to peptide hormones, and skeletal muscle development (Figure 4A). DO analysis showed that the targets were strongly linked to female reproductive organ cancer, endocrine system disease, and brain disease (Figure 4B). According to KEGG enrichment analysis, these genes were mainly enriched through several signaling pathways including the Kaposi sarcoma-associated herpesvirus infection, tumor necrosis factor (TNF) signaling pathway, IL-17 signaling pathway, and coronavirus disease (Figure 4C). Figure 4D showed the specific role of TNF signaling pathway, including cell survival, death, and differentiation.
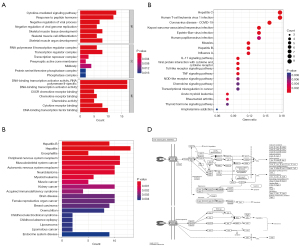
Protein-protein interaction network construction
A potential target interaction network was built with 76 nodes and 220 lines (Figure 5). Several genes in the network’s center influencing other genes included CXCL11, CXCL10, IRF7, EGR1, SGK1, FOXO1, IRS2, MYC, JUN, FOS, ATF3, and IER3. In addition, this network’s 76 nodal genes were chosen for further biomarker screening.
Biomarker screening in patients with BC based on machine learning algorithms
In this study, three machine-learning algorithms were applied to investigate prospective BC biomarkers in 76 critical genes. In the random forest results, 38 genes with a significance greater than 1 were chosen as potential biomarkers (Figure 6A). LASSO regression models based on cancerous and normal tissues were created. The λ analysis revealed that the model was the most accurate to predict BC when λ =24. As a result of the LASSO analysis, 24 potential genes were identified (Figure 6B). Furthermore, SVM-REF analysis showed that the most accurate model was the one with 23 genes (Figure 6C). Ultimately, the outputs of above algorithms were combined, yielding DIXDC1, DUSP6, PDK4, CXCL12, IRF7, ITGA7, NEK2, and NR3C1 as prospective biomarkers associated with BC (Figure 6D).
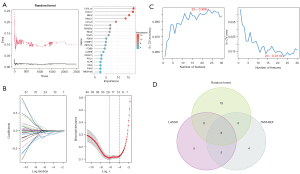
Gene expression analysis and protein expression analysis
Using the GEPIA dataset, we compared the expression of eight biomarker mRNAs in BC and breast tissues. The results showed that IRF7 and NEK2 expression levels were higher in BC tissues than in normal tissues, whereas DIXDC1, DUSP6, PDK4, CXCL12, ITGA7, and NR3C1 expression levels were lower in BC tissues than in normal tissues (Figure 7A). The above findings were verified in the UALCAN databases (Figure S1). Using the HPA database to investigate the protein level of biomarkers in BC tissues, we found no significant difference in NEK2, NR3C1, ITGA7, and IRF7 protein expressions between BC and normal breast tissues. The expressions of PDK4, DIXDC1, CXCL12 and DUSP6 proteins were higher in BC tissues than in normal breast tissues (Figure 7B).
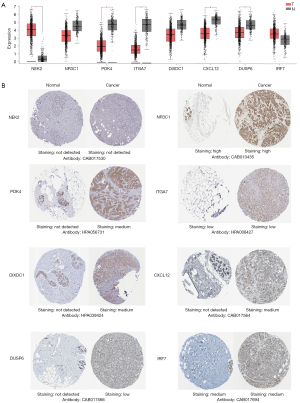
Correlation of prospective biomarkers and analysis of their prognostic ability
Initially, correlation analysis revealed that NEK2 expression levels in BC patients had a significantly negative correlation with CXCL12 and DIXDC1 levels. Furthermore, ITGA7 revealed a significantly positive correlation with PDK4 expression levels, while IRF7 showed a positive correlation with NR3C1 expression levels (Figure 8A). Following that, ROC analyses were run on eight potential biomarkers, indicating that DIXDC1, DUSP6, PDK4, CXCL12, IRF7, ITGA7, NEK2, and NR3C1 were prospective as diagnostic biomarkers for BC. Among them, NEK2 showed outstanding clinical diagnostic ability (Figure S2). To better understand the relationship between the expression levels of these eight biomarkers and recurrence-free survival (RFS), overall survival (OS), distant metastasis-free survival (DMFS), and post-progression survival (PPS) in BC patients, the online Kaplan-Meier mapper tool was used to analyze the clinical data and expression profile of the markers in the database. As demonstrated in Figure 8B, elevated expression of NR3C1, PDK4, DIXDC1, CXCL12, and DUSP6 coupled with diminished expression of NEK2 was positively associated with RFS of BC patients (P<0.05). Elevated expression of NR3C1, DIXDC1, and CXCL12 coupled with diminished expression of NEK2 was positively associated with OS of BC patients (P<0.05). Elevated expression of DIXDC1, CXCL12, and DUSP6 coupled with diminished expression of NEK2 was positively associated with DMFS of BC patients (P<0.05). In conclusion, elevated expression of DIXDC1 and CXCL12 coupled with diminished expression of NEK2 was positively associated with RFS, OS, and DMFS of BC patients (P<0.05).
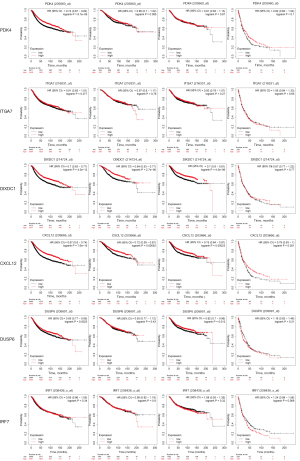
Correlation of transcript levels of NEK2, DIXDC1 and CXCL12 genes with molecular subtypes and tumor stage of BC and gene set enrichment analysis
After exploring the expression pattern and prognostic ability of these eight genes in BC, we selected NEK2, DIXDC1 and CXCL12, which have a better prognostic ability, to explore whether their transcript levels are associated with the molecular subtype and stage of BC. To find the answer, we analyzed the data from the UALCAN database based on the TCGA database. DIXDC1 and CXCL12 are lowly expressed in triple-negative BCs and NEK2 is highly expressed in triple-negative BCs compared to ductal BC and Her2+ BCs (Figure 9A). The expressions of NEK2, DIXDC1, and CXCL12 were significantly associated with the stage of BC tumor (Figure 9B). Furthermore, we performed GSEA of NEK2, DIXDC1 and CXCL12 and detected multiple pathways, such as extracellular matrix (ECM)-receptor interaction and proteasome (Figure 9C).
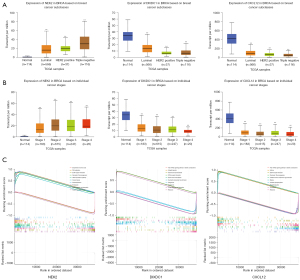
Expression pattern of NEK2, DIXDC1 and CXCL12 in single cell and their relationship with cancer functional status
The CANCERSEA website was used to investigate the expression of NEK2, DIXDC1, and CXCL12 in tumors and their association with tumor functional status. We found that NEK2 expression in lung adenocarcinoma (LUAD) and colorectal cancer (CRC) was significantly positively associated with cell cycle, proliferation, and DNA damage, NEK2 expression in BC was positively correlated with cell cycle, proliferation, and DNA damage. DIXDC1 expression in prostate cancer (PC) was significantly positively associated with angiogenesis and invasion. DIXDC1 expression in uveal melanoma (UM) was significantly positively associated with angiogenesis and differentiation. CXCL12 expression in UM was significantly negatively associated with DNArepair, DNAdamage, and metastasis. DIXDC1 and CXCL1 expression in BC were not significantly associated with tumor functional status (Figure 10A). Figure 10B showed the relationship of NEK2 expression with CellCycle, Proliferation, and DNAdamage in BC. The expression profiles of NEK2, DIXDC1 and CXCL12 were shown in single cells of BC by t-SNE diagram (Figure 10C). t-SNE diagram depicts the distribution of cells, each dot represents a cell and the color of the dot represents the expression level of that gene (gene list) in the cell. NEK2 expression levels in BC were generally higher than those of DIXDC1 and CXCL12 through t-SNE diagram, suggest that NEK2 may play an important role in BC tumor progression.
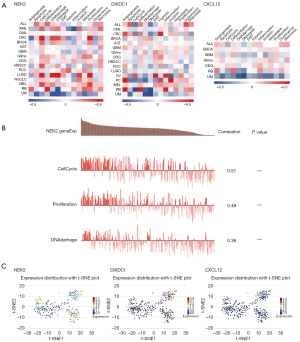
Correlation of NEK2 with immune cell infiltration in breast malignancy
The tumor microenvironment includes immune cell infiltration, which also serves as an indispensable signal of genetics and prognostic frontiers of lymph node metastasis. As illustrated in Figure 11A, the TIMER2.0 database was used to explore the correlation of common immune cells with NEK2 in different molecular types of breast cancer (BRCA). The level of NEK2 expression was positively related to the number of neutrophils in BRCA-luminal and not related to the number of neutrophils in BRCA-Basal and BRCA-Her2. The level of NEK2 expression was positively related to the number of CD4+ cells in BRCA-luminal and negatively related to the number of CD4+ cells in BRCA-Her2. The level of NEK2 expression was positively related to the number of CD8+ cells in BRCA-luminal and BRCA-Her2 (P<0.05). Significant differences were shown by R package xCell between immune cells with high and low expression of NEK2, including CD4 cells, M macrophages, and fibroblasts (Figure 11B). These findings suggest that the NEK2 gene is significant for immune cell regulation in BC.
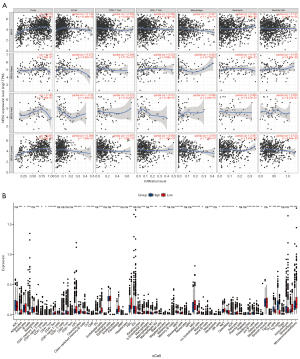
Prediction of therapeutic drugs
Using the DSigDB database, relevant drugs were predicted based on NEK2 in BC tissues. We considered the top 10 drugs with P values in the prediction outcomes to be potential NEK2 inhibitors, including etoposide, LUCANTHONE, testosterone, etc. (Figure 12). Additionally, we chose the biomarker (ARHGAP11A) that is strongly linked to the drug (etoposide) as a representative and used the Dock-Thor online platform to carry out molecular docking to confirm the level of binding between the biomarker and the medication. The outcomes were then displayed using ChimeraX software. The findings revealed that biomarkers and pharmaceuticals had good binding capacities and that the docking energy was −7.632 kJ/moL (Figure 13).
Discussion
In recent years, BC has overtaken lung cancer as the major cause of cancer mortality globally. Among women, BC accounts for one-sixth of deadly cancers and one-tenth of cancer cases, posing a great challenge to women’s health (9). Although many research results have been accumulated by previous authors and a growing number of treatment plans for BC have emerged, the molecular mechanisms underlying its development are still unclear. With the advancement of bioinformatics, key tumor therapeutic targets can be rapidly identified based on numerous sample sequencing data. Previous studies have identified BC-related biomarkers by WGCNA or differential analysis (10) and have provided references for endocrine therapy and immunotherapy for BC (11,12). There are also studies that have identified novel genetic algorithms to predict the efficacy of BC treatment by machine learning algorithms such as random forest algorithms (13). Fewer studies have combined WGCNA with machine learning algorithms to identify BC biomarkers. In this study, we combined differential analysis and WGCNA screening of pivotal genes, integrated three machine learning algorithms to screen for biomarkers, and then performed functional and prognostic analysis on the resulting targets. Finding these biomarkers will assist in disease diagnosis, therapy selection, and treatment response prediction.
In the present study, 1,673 DEGs were first identified in BC tissues. These DEGs were then subjected to a GSEA functional analysis, and it was revealed that these genes are involved in the pathways of DNA replication, fatty acid degradation, homologous recombination, mismatch repair, PPAR signaling pathway, regulation of lipolysis in adipocytes and taurine metabolic pathway. Previous study has shown that free fatty acids (FFAs) regulate carcinogenesis through fatty acid metabolism and lipid peroxidation and that fatty acid receptors may be a promising target for BC therapy (14). In BC, the PPAR signaling pathway has been identified as a biological process of great relevance, and attempts have been made to investigate the genes connected to the PPAR signaling system (15,16). The taurine metabolic pathway has also been associated with BC growth and lung metastasis (17). WGCNA produced 542 genes, while crossover analysis produced 76 crossover genes. Functional analysis of these targets showed that these genes are mainly related to BPs like cytokine-mediated signaling pathway, response to peptide hormone, and skeletal muscle development, and enriched in KEGG pathways, including IL-17 signaling pathway and chemokine signaling pathway. Additionally, DO analysis identified critical targets mainly associated with female reproductive organ cancer and endocrine system disease. There is no doubt that the female endocrine system plays a key role in the occurrence and development of BC, such as estrogen, progesterone, and prolactin (18-20). In both humans and experimental animals, the association between hormone exposure and BC has a strong body of evidence. Hormone replacement therapy increases risk while ovariectomy decreases it in humans. Hormone supplementation increases mammary tumors in both rats and mice (21). Chemicals that promote E2 and P4 steroidogenesis tend to raise the risk of BC (22). Moreover, the pro-carcinogenic role of the IL-17 family in BC is well established in the IL-17 signaling pathway. This corresponds to the findings of functional analysis (23). Inflammation is an immunovascular defensive reaction, and chronic inflammation can set off a chain of molecular processes that result in differentiated cells becoming malignant and the inhibition of antitumor immunity, which in turn promotes the growth and metastasis of BC (24).
In addition, we researched the relationships between 76 genes and created a Protein-Protein Interaction (PPI) network. Random forest, SVM-REF, and LASSO algorithms were then used to identify DIXDC1, DUSP6, PDK4, CXCL12, IRF7, ITGA7, NEK2, and NR3C1 as potential diagnostic biomarkers for BC. NEK2 is the most promising diagnostic biomarker among them. Functional analysis of NEK2 also suggests that it may be involved in several metabolic pathways associated with BC, such as amino acid biosynthesis and glycolysis/glycogenesis. As a member of the NEK protein family, NEK2 is a mitogenic kinase present in many human cancers, including myeloma (25), PC (26), and CRC (27). It commonly exhibits upregulation in tumors, resulting in malignancy and drug resistance. The primary pro-tumorigenic effects of NEK2 are mainly due to its critical role in promoting cell cycle progression, which is consistent with the results of the aforementioned single-cell analysis. NEK2 achieves this by regulating multiple aspects of the cell cycle, such as microtubule stabilization, chromatin condensation, centrosome replication and segregation, kinetochore attachment, spindle pole body age markers, and spindle assembly checkpoints (28,29). The HPA database indicated no significant difference in the expression of NEK2 protein between BC tissues and normal breast tissues. This finding may be attributed to several factors, such as ubiquitination modification, post-transcriptional modification of the protein, and regulation of protein translation efficiency, which warrant further investigation. According to the UALCAN and GEPIA2 databases, NEK2 is highly expressed in BC, especially triple-negative breast cancer (TNBC). It has been discovered that the oncogenic kinase NEK2 is highly overexpressed in TNBC and helps to create its distinctive splicing profile. Dysregulation of selective splicing has been shown to be a feature of TNBC (30). NEK2 is elevated in paclitaxel-resistant cells, and NEK2 upregulation phosphorylates Catenin β1 (CTNNB 1), which activates the WNT signaling pathway and leads to paclitaxel resistance in TNBC. High NEK2 expression predicts lower survival in TNBC patients with residual disease after treatment with paclitaxel plus anthracyclines (31). Additionally, NEK2 phosphorylates TRF1, inducing abnormal mitosis and chromosomal instability in BC cells, and activates extracellular signal-regulated kinase/mitogen-activated protein kinases (ERK/MAPK) signaling to promote their growth (32). The critical oncogenic role of NEK2 determines that NEK2-targeted methods could be a prospective therapeutic tool for TNBC treatment. In addition, according to Anuraga et al., NEK2 plays a role in immune infiltration and can be employed as a predictive biomarker for the advancement of BC (33). Based on the results of ROC analysis, single cell sequencing data analysis, and the Kaplan-Meier Plotter database in the current study, low NEK2 expression is frequently associated with a favorable prognosis, indicating that it may have prospective as a biomarker for BC.
A large amount of evidence points to immune cell infiltration as a key factor in determining the success of immunotherapies and the ability of carcinogenesis and recurrence. Therefore, more scholars are recognizing the significance of the immune system in tumorigenesis. The tumor immune microenvironment is the main battlefield of the competitive game between the tumor and the host immune system. Due to heterogeneity, immune cells turn from host “soldiers” to “enemies”, such as the polarization of M1-like to M2-like tam (34). Although BC has not been classified as immunogenic, there are many immunotherapeutic agents that can modulate its interaction with the immune system, especially in triple-negative BCs that lack multiple therapeutic options (35). The TIMER2.0 database and the R package “X cell” analysis revealed that NEK2 gene expression correlated with the level of immune infiltration of CD8+ T cells, CD4+ T cells, neutrophils, and macrophages. Tumor-reactive CD4 and CD8 T cells predict the response to immune checkpoint blockade (ICB) (36). Moreover, the interaction between CD8+ T cells and a group of FOLR2-expressing tissue-resident macrophages in BC tissue has also been demonstrated, providing a direction for macrophage-based cancer immunotherapy (37). All of the above findings suggest that NEK2 may be involved in BC cell immunity and may provide a basis for BC immunotherapy. In addition, we predicted prospective drugs that interact with NEK2, such as etoposide and LUCANTHONE. Etoposide is an inhibitor of topoisomerase-ii (encoded by TOP2A) and is used to combat different types of cancer, including BC. Numerous studies have demonstrated the clinical activity of etoposide in MBC as an option for the treatment of TNBC or metastatic HER2-positive BC that has received multiple lines of therapy (38-40), with excellent efficacy and controlled safety. Lukasunone is a blood-brain barrier-crossing anti-schistosomal drug and modulates tumor survival/death in an autophagy-dependent manner. Lukasunone has recently been shown to inhibit autophagy in BC cells (41).
This study also has some limitations. In this study, we used only the TCGA database and GSE15852 database. Furthermore, the targets we identified lacked confirmation of their expression profile in clinical or animal trials. Our study only investigated NEK2, which has the largest AUC value, and the remaining 7 genes were not further explored.
Conclusions
In summary, we screened 76 hub genes in the tissues of BC patients. Using three machine learning algorithms, DIXDC1, DUSP6, PDK4, CXCL12, IRF7, ITGA7, NEK2, and NR3C1 were discovered in BC patients’ tissues as potential diagnostic biomarkers. Among them, NEK2 was the most valuable diagnostic biomarker. Both the critical targets in BC and the NEK2 functional enrichment indicate differences in metabolic pathways in BC development, and an increasing number of studies are beginning to focus on changes in metabolic pathways in BC patients. Immune infiltration analysis has also identified immune cells that are closely associated with NEK2. BC is complex and heterogeneous, which makes the treatment extremely difficult. The results of this study provide new ideas for immunotherapy of BC. In addition, drugs such as etoposide and lukasunone that may act on BC are predicted. Overall, the findings herein contribute to improving BC diagnosis and treatment. However, more research is required to determine the mechanism underlying the function of these eight genes, particularly NEK2, in the onset and spread of BC.
Acknowledgments
Funding: None.
Footnote
Reporting Checklist: The authors have completed the STREGA reporting checklist. Available at https://tcr.amegroups.com/article/view/10.21037/tcr-23-3/rc
Peer Review File: Available at https://tcr.amegroups.com/article/view/10.21037/tcr-23-3/prf
Conflicts of Interest: All authors have completed the ICMJE uniform disclosure form (available at https://tcr.amegroups.com/article/view/10.21037/tcr-23-3/coif). The authors have no conflicts of interest to declare.
Ethical Statement: The authors are accountable for all aspects of the work in ensuring that questions related to the accuracy or integrity of any part of the work are appropriately investigated and resolved. The study was conducted in accordance with the Declaration of Helsinki (as revised in 2013).
Open Access Statement: This is an Open Access article distributed in accordance with the Creative Commons Attribution-NonCommercial-NoDerivs 4.0 International License (CC BY-NC-ND 4.0), which permits the non-commercial replication and distribution of the article with the strict proviso that no changes or edits are made and the original work is properly cited (including links to both the formal publication through the relevant DOI and the license). See: https://creativecommons.org/licenses/by-nc-nd/4.0/.
References
- Siegel RL, Miller KD, Fuchs HE, et al. Cancer Statistics, 2021. CA Cancer J Clin 2021;71:7-33. [Crossref] [PubMed]
- Zhu C, Xu J, Sun J, et al. Circulating Tumor Cells and Breast Cancer Metastasis: From Enumeration to Somatic Mutational Profile. J Clin Med 2022;11:6067. [Crossref] [PubMed]
- Xiao Q, Cheng Z, Kuang W, et al. Clinical Value of PPM1G Gene in Survival Prognosis and Immune Infiltration of Hepatocellular Carcinoma. Appl Bionics Biomech 2022;2022:8926221. [Crossref] [PubMed]
- Zhu YX, Huang JQ, Ming YY, et al. Screening of key biomarkers of tendinopathy based on bioinformatics and machine learning algorithms. PLoS One 2021;16:e0259475. [Crossref] [PubMed]
- Meng XW, Cheng ZL, Lu ZY, et al. MX2: Identification and systematic mechanistic analysis of a novel immune-related biomarker for systemic lupus erythematosus. Front Immunol 2022;13:978851. [Crossref] [PubMed]
- Gautier L, Cope L, Bolstad BM, et al. affy--analysis of Affymetrix GeneChip data at the probe level. Bioinformatics 2004;20:307-15. [Crossref] [PubMed]
- Ritchie ME, Phipson B, Wu D, et al. limma powers differential expression analyses for RNA-sequencing and microarray studies. Nucleic Acids Res 2015;43:e47. [Crossref] [PubMed]
- Antonacci Y, Toppi J, Mattia D, et al. Single-trial Connectivity Estimation through the Least Absolute Shrinkage and Selection Operator. Annu Int Conf IEEE Eng Med Biol Soc 2019;2019:6422-5. [Crossref] [PubMed]
- Sung H, Ferlay J, Siegel RL, et al. Global Cancer Statistics 2020: GLOBOCAN Estimates of Incidence and Mortality Worldwide for 36 Cancers in 185 Countries. CA Cancer J Clin 2021;71:209-49. [Crossref] [PubMed]
- Bao Z, Cheng J, Zhu J, et al. Using Weighted Gene Co-Expression Network Analysis to Identify Increased MND1 Expression as a Predictor of Poor Breast Cancer Survival. Int J Gen Med 2022;15:4959-74. [Crossref] [PubMed]
- Ye Z. Identification of T cell-related biomarkers for breast cancer based on weighted gene co-expression network analysis. J Chemother 2022; Epub ahead of print. [Crossref] [PubMed]
- Lu C, Yang Y, Lingmei L, et al. Identification of hub genes in AR-induced tamoxifen resistance in breast cancer based on weighted gene co-expression network analysis. Breast Cancer Res Treat 2023;197:71-82. [Crossref] [PubMed]
- Johnson H, Ali A, Zhang X, et al. K-RAS Associated Gene-Mutation-Based Algorithm for Prediction of Treatment Response of Patients with Subtypes of Breast Cancer and Especially Triple-Negative Cancer. Cancers (Basel) 2022;14:5322. [Crossref] [PubMed]
- Karmokar PF, Moniri NH. Oncogenic signaling of the free-fatty acid receptors FFA1 and FFA4 in human breast carcinoma cells. Biochem Pharmacol 2022;206:115328. [Crossref] [PubMed]
- Wang X, Yao Z, Fang L. miR-22-3p/PGC1β Suppresses Breast Cancer Cell Tumorigenesis via PPARγ. PPAR Res 2021;2021:6661828. [Crossref] [PubMed]
- Xu Y, Shu D, Shen M, et al. Development and Validation of a Novel PPAR Signaling Pathway-Related Predictive Model to Predict Prognosis in Breast Cancer. J Immunol Res 2022;2022:9412119. [Crossref] [PubMed]
- Chen W, Li Q, Hou R, et al. An integrated metabonomics study to reveal the inhibitory effect and metabolism regulation of taurine on breast cancer. J Pharm Biomed Anal 2022;214:114711. [Crossref] [PubMed]
- Brisken C, Scabia V. 90 YEARS OF PROGESTERONE: Progesterone receptor signaling in the normal breast and its implications for cancer. J Mol Endocrinol 2020;65:T81-94. [Crossref] [PubMed]
- Fernandez SV, Russo J. Estrogen and xenoestrogens in breast cancer. Toxicol Pathol 2010;38:110-22. [Crossref] [PubMed]
- Ali S, Hamam D, Liu X, et al. Terminal differentiation and anti-tumorigenic effects of prolactin in breast cancer. Front Endocrinol (Lausanne) 2022;13:993570. [Crossref] [PubMed]
- Rudel RA, Ackerman JM, Attfield KR, et al. New exposure biomarkers as tools for breast cancer epidemiology, biomonitoring, and prevention: a systematic approach based on animal evidence. Environ Health Perspect 2014;122:881-95. [Crossref] [PubMed]
- Cardona B, Rudel RA. Application of an in Vitro Assay to Identify Chemicals That Increase Estradiol and Progesterone Synthesis and Are Potential Breast Cancer Risk Factors. Environ Health Perspect 2021;129:77003. [Crossref] [PubMed]
- Song X, Wei C, Li X. The potential role and status of IL-17 family cytokines in breast cancer. Int Immunopharmacol 2021;95:107544. [Crossref] [PubMed]
- Amara S, Majors C, Roy B, et al. Critical role of SIK3 in mediating high salt and IL-17 synergy leading to breast cancer cell proliferation. PLoS One 2017;12:e0180097. [Crossref] [PubMed]
- Zhou W, Yang Y, Xia J, et al. NEK2 induces drug resistance mainly through activation of efflux drug pumps and is associated with poor prognosis in myeloma and other cancers. Cancer Cell 2013;23:48-62. [Crossref] [PubMed]
- Zeng YR, Han ZD, Wang C, et al. Overexpression of NIMA-related kinase 2 is associated with progression and poor prognosis of prostate cancer. BMC Urol 2015;15:90. [Crossref] [PubMed]
- Neal CP, Fry AM, Moreman C, et al. Overexpression of the Nek2 kinase in colorectal cancer correlates with beta-catenin relocalization and shortened cancer-specific survival. J Surg Oncol 2014;110:828-38. [Crossref] [PubMed]
- Sonn S, Jeong Y, Rhee K. Nip2/centrobin may be a substrate of Nek2 that is required for proper spindle assembly during mitosis in early mouse embryos. Mol Reprod Dev 2009;76:587-92. [Crossref] [PubMed]
- Wei R, Ngo B, Wu G, et al. Phosphorylation of the Ndc80 complex protein, HEC1, by Nek2 kinase modulates chromosome alignment and signaling of the spindle assembly checkpoint. Mol Biol Cell 2011;22:3584-94. [Crossref] [PubMed]
- Naro C, Barbagallo F, Caggiano C, et al. Functional Interaction Between the Oncogenic Kinase NEK2 and Sam68 Promotes a Splicing Program Involved in Migration and Invasion in Triple-Negative Breast Cancer. Front Oncol 2022;12:880654. [Crossref] [PubMed]
- Roberts MS, Sahni JM, Schrock MS, et al. LIN9 and NEK2 Are Core Regulators of Mitotic Fidelity That Can Be Therapeutically Targeted to Overcome Taxane Resistance. Cancer Res 2020;80:1693-706. [Crossref] [PubMed]
- Xing Z, Zhang M, Wang X, et al. Silencing of Nek2 suppresses the proliferation, migration and invasion and induces apoptosis of breast cancer cells by regulating ERK/MAPK signaling. J Mol Histol 2021;52:809-21. [Crossref] [PubMed]
- Anuraga G, Wang WJ, Phan NN, et al. Potential Prognostic Biomarkers of NIMA (Never in Mitosis, Gene A)-Related Kinase (NEK) Family Members in Breast Cancer. J Pers Med 2021;11:1089. [Crossref] [PubMed]
- He L, Jhong JH, Chen Q, et al. Global characterization of macrophage polarization mechanisms and identification of M2-type polarization inhibitors. Cell Rep 2021;37:109955. [Crossref] [PubMed]
- Yang F, Xiao Y, Ding JH, et al. Ferroptosis heterogeneity in triple-negative breast cancer reveals an innovative immunotherapy combination strategy. Cell Metab 2023;35:84-100.e8. [Crossref] [PubMed]
- Liu B, Zhang Y, Wang D, et al. Single-cell meta-analyses reveal responses of tumor-reactive CXCL13(+) T cells to immune-checkpoint blockade. Nat Cancer 2022;3:1123-36. [Crossref] [PubMed]
- Nalio Ramos R, Missolo-Koussou Y, Gerber-Ferder Y, et al. Tissue-resident FOLR2(+) macrophages associate with CD8(+) T cell infiltration in human breast cancer. Cell 2022;185:1189-207.e25. [Crossref] [PubMed]
- Hu N, Zhu A, Si Y, et al. A Phase II, Single-Arm Study of Apatinib and Oral Etoposide in Heavily Pre-Treated Metastatic Breast Cancer. Front Oncol 2021;10:565384. [Crossref] [PubMed]
- Chen TW, Jan IS, Chang DY, et al. Systemic treatment of breast cancer with leptomeningeal metastases using bevacizumab, etoposide and cisplatin (BEEP regimen) significantly improves overall survival. J Neurooncol 2020;148:165-72. [Crossref] [PubMed]
- Chalumeau C, Carton M, Eeckhoutte A, et al. Oral Etoposide and Trastuzumab Use for HER2-Positive Metastatic Breast Cancer: A Retrospective Study from the Institut Curie Hospitals. Cancers (Basel) 2022;14:2114. [Crossref] [PubMed]
- Esteve JM, Esteve-Esteve M. Molecular pathways of autophagy regulation by BRCA1: Implications in cancer. Rev Esp Patol 2020;53:246-53. [Crossref] [PubMed]