A signature of cuproptosis-related lncRNAs predicts prognosis and provides basis for future anti-tumor drug development in breast cancer
Highlight box
Key findings
• We selected 11 cuproptosis-related lncRNAs to construct a risk score system to predict the prognosis and guide treatment of breast cancer patients.
What is known and what is new?
• Cuproptosis is a novel and promising modality of tumor cell death, and lncRNAs play an important role in cancer development.
• We proposed a new risk score system using cuproptosis-related lncRNAs.
What is the implication, and what should change now?
• The risk score system provides a basis for the clinical treatment of breast cancer patients, and more experiments will be done on these cuproptosis-related lncRNAs in future.
Introduction
Breast cancer has surpassed lung cancer as the most prevalent malignancy worldwide (1). In addition, breast cancer is the leading culprit for death in women (1). Although the prognosis for early-stage breast cancer patients has dramatically improved thanks to advances in imaging technologies, significant regional disparities still exist. Breast cancer patients in less developed areas are still facing a difficult situation due to delayed diagnosis (2,3). Meanwhile, patients with advanced breast cancer are still in despair, with a 5-year survival rate of 20% and a median overall survival time of 2–3 years (4). Therefore, it is of great importance to explore biomarkers for prognosis prediction and novel anti-tumor drug development.
Cuproptosis is a novel pattern of cell death differing from traditional cell death processes like apoptosis, autophagy, pyroptosis, and ferroptosis. The copper ion is an indispensable trace element in living organisms and is maintained at an extremely low level in the normal physiological setting. When the intracellular concentration of copper ions exceeds the threshold for maintaining homeostasis, excess coppers bind directly to the lipid-acylated components of the tricarboxylic acid cycle (TCA), resulting in lipid acylated protein aggregation and iron-sulfur cluster protein loss, leading to proteotoxic stress and cell death. Researchers knocked out the essential apoptosis factors BAX and BAK1 and treated them with well-known cell death modality inhibitors (ferrostatin-1 against ferroptosis, necrostatin-1 against necroptosis, and N-acetyl cysteine against oxidative stress) to examine the crosstalk between cuproptosis and existing cell death patterns. As a result, they discovered that cuproptosis was solely dependent on the accumulation of intracellular copper ions and could not be interfered with by any of the known cell death patterns (5). This novel and manageable cell death pattern promises to usher in a new era in the treatment of breast cancer.
Long non-coding RNAs (lncRNAs) are a category of transcript RNAs with a length of more than 200 nucleotides with no ability to code proteins (6). Instead, it can achieve post-transcriptional modification of mRNAs and regulation of cellular functions by competing endogenous RNAs (ceRNA) (7). This epistemic regulatory pattern of lncRNAs has been proven to be closely related to the development, progression, and cell death of breast cancer (8,9). Both Zhao et al. and Dong et al. demonstrated that lncRNAs could affect the apoptosis of breast cancer cells by interacting with miRNAs in their studies (10,11). Wang et al. revealed that lncRNA H19 could promote drug resistance of breast cancer cells by regulating autophagy (12). Yan et al. demonstrated in their study that lncRNA MEG3 was related to Cisplatin-induced pyroptosis (13). In the study conducted by Mao et al., they proposed that lncRNA P53RRA could interact with G3BP1 to regulate breast cancer ferroptosis through P53 (14). Meanwhile, lncRNAs also serve as the biomarker for cancer diagnosis and prognosis (15). However, it is still not clear what the relationship between lncRNAs and cuproptosis is and what role cuproptosis-related lncRNAs play in breast cancer.
In this study, a prognostic risk score system comprising cuproptosis-related lncRNAs based on the TCGA data was first developed. Then, the role of cuproptosis-related lncRNA, immune microenvironment, tumor mutational burden (TMB), and N6-adenylate methylation (m6a) in breast cancer prognosis was explored. We present this article in accordance with the TRIPOD reporting checklist (available at https://tcr.amegroups.com/article/view/10.21037/tcr-22-2702/rc).
Methods
Acquisition and processing of patient data
The RNA-seq data comprised 1,113 breast cancer samples and 113 normal breast tissues were downloaded from the BRCA (breast invasive carcinoma) project of TCGA (The Cancer Genome Atlas) (https://portal.gdc.cancer.gov/). Sequencing data was processed into the STAR format for further analysis. Clinical information and mutation data were also obtained from TCGA. Patients lacking clinical information were excluded. Cuproptosis-related genes were obtained from a piece of published literature (5). This study was conducted in accordance with the Declaration of Helsinki (as revised in 2013).
Identification of cuproptosis-related lncRNAs
The “limma” package in R was used to identify the expression of cuproptosis-related genes in tumor samples and to identify cuproptosis-related lncRNAs by co-expression analysis. The existence of a co-expression relationship was implied by meeting the criteria of |Cor| >0.4 and P<0.001.
Construction and validation of a cuproptosis-related lncRNAs risk score system
All patients were allocated at random into the training cohort and the validation cohort in a 1:1 ratio. In the training cohort, the univariate Cox regression analysis was used to select lncRNAs associated with prognosis. The least absolute shrinkage and selection operator (LASSO) regression was used to further specify hallmark genes comprising the risk score. On this basis, every patient got a risk score. Risk score = (−0.623693964126545*ExpressionNIFK-AS1) + (−0.441658254819315*Expression AL023882.1) + (1.40269668671941*Expression AC091588.1) + (−2.4380379946419*Expression AC138028.2) + (0.918403164700327*Expression AC027514.1) + (0.879444991789677*Expression MECOM-AS1) +( -2.36124060410255*Expression LRRC8C-DT) + (0.403143544676337*Expression OTUD6B-AS1) + (−1.03019000430915*ExpressionRNF32-AS1) + (2.42969891942799*Expression MFF-DT) + (0.795910837876363*ExpressionAL592301.1). Based on the mean value of risk scores, they were divided into the high-risk group and the low-risk group. In both the training cohort and validation cohort, the Kaplan-Meier analysis was used to investigate the relationship between risk score and patient prognosis, including overall survival (OS) and progression-free survival (PFS). The receiver operating characteristic (ROC) curve was used to assess the prognostic prediction power of the risk scoring system. Packages in R including “survival”, “caret”, “glmnet”, “survminer” and “timeROC” were used.
Construction and assessment of a nomogram
The univariate and multivariate Cox regressions were used to examine the independent prognostic role of the risk score system and to clarify remaining prognostic factors. On this basis, a nomogram was developed to predict the 1-, 3- and 5-year survival rates of patients. Its accuracy was assessed by a calibration plot. Packages in R including “survival”, “regplot” and “rms” were used.
Functional enrichment analysis between high- and low-risk groups
Differentially expressed genes (DEGs) were identified between high- and low-risk groups in the tumor samples. The criteria for differential expression were |logFC|>1 and P<0.05. The Gene Ontology (GO) and Kyoto Encyclopedia of Genes and Genomes (KEGG) were used to identify functions and key signaling pathways associated with DEGs. Packages in R including “limma”, “colorspace”, “stringi”, “ggplot2”, “circlize”, “RcolorBrewer”, “ggpubr”, “colorspace”, “stringi”, “ggplot2” “circlize” and “RColorBrewer” were used.
Analysis and quantification of the mutation burden
The mutation data were downloaded from TCGA. TMB refers to the number of mutated bases per million bases. After obtaining the TMB for each patient, the variations in patient TMB between the high- and low-risk groups were first investigated. Waterfall plots were used to demonstrate differences in mutation frequencies between high- and low-risk groups. Then, all patients were divided into high- and low-mutation groups based on the TMB cut-off value to examine the relationship between TMB and prognosis. Packages in R including “ggpubr”, “limma”, “survival” and “survminer” were used.
Analysis of the immune infiltration
Differences in immune infiltration and immune-related processes between the high- and low-risk groups with the single-sample gene set enrichment analysis (ssGSEA) algorithm were examined. In addition, differences in the immune checkpoint inhibitors expression between the two groups with a view were examined to offer advice for potential immunotherapy. Packages in R including “limma”, “GCVA”, “GSEABase”, “Pheatmap” and “reshape2” were used.
Analysis of the drug sensitivity
IC50 refers to the drug concentration required to inhibit 50% of a target, which is used to evaluate the sensitivity of a drug. To explore the relationship between cuproptosis-Related lncRNAs and the anti-tumor drug sensitivity, the IC50 of 251 kinds of anti-tumor drugs was calculated and their differences between the high- and low-risk groups were compared. Packages in R including “pRRophetic”, “ggpubr”, “limma” and “ggplot2” were used.
Statistical analysis
The Chi-square test was used to analyze differences in patients’ general characteristics between the two groups. Pearson correlation analysis was used to investigate the co-expression relationship between lncRNAs and cuproptosis-related genes. The Kaplan-Meier analysis with a log-rank test was used to investigate the OS and PFS of patients in different groups. The Cox regression was used to identify independent prognostic factors. Factors with P<0.1 in univariate regression were included in multivariate regression. Statistics were judged significant at P<0.05. All statistical analyses were carried out in R (v.3.6.2).
Results
Acquisition of cuproptosis-related lncRNAs
Initially, 19 cuproptosis-related genes from the original literature were extracted. Then, using co-expression analysis, 677 cuproptosis-related lncRNAs were found in the TCGA database. The co-expression relationships are displayed in a Sankey diagram shown in Figure 1, above the picture are cuproptosis-related genes and below the picture are cuproptosis-related lncRNAs, the connections between them represent the co-expression relationships (Figure 2). The process of data collection and analysis is shown in Figure 2.

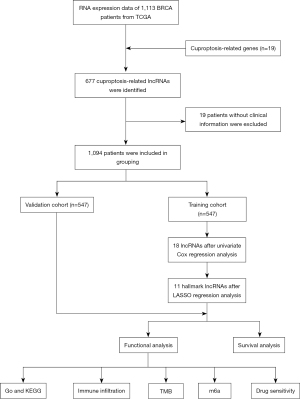
Construction of a risk score system
All 1,094 eligible patients were divided randomly into the training and validation cohorts in a 1:1 ratio after those lacking clinical information were excluded. The training cohort was used to construct the risk score system and the validation group was used to verify its prognostic value. After statistical analysis, there were no statistically significant differences between the two patient groups in terms of demographics like age, gender, or stage (Table 1). The construction of the risk score system is shown in Figure 3. In the training cohort, 18 prognostic-related lncRNAs were identified using univariate cox regression analysis (Figure 3A). Then, by LASSO regression analysis, we obtained the minimum error from the figure that we should select 11 genes that were used to construct the risk score system (Figure 3C). And Coefficient distribution diagram showed the risk score of each lncRNA (Figure 3B). In the meantime, a heat map was plotted to display the co-expression relationship between these lncRNAs and the original 19 cuproptosis-related genes (Figure 3D). According to the risk score system, each patient was assigned a risk score. Based on the average of the risk scores, they were further divided into high- and low-risk groups.
Table 1
Variables | Total cohort (n=1,094), n (%) | Training cohort (n=547), n (%) | Validation cohort (n=547), n (%) | P value |
---|---|---|---|---|
Age (year) | 1.000 | |||
≤65 | 774 (70.75) | 387 (70.75) | 387 (70.75) | |
>65 | 320 (29.25) | 160 (29.25) | 160 (29.25) | |
Gender | 0.1467 | |||
Female | 1,082 (98.9) | 544 (99.45) | 538 (98.35) | |
Male | 12 (1.1) | 3 (0.55) | 9 (1.65) | |
Stage | 0.1729 | |||
Stage I | 182 (16.64) | 102 (18.65) | 80 (14.63) | |
Stage II | 619 (56.58) | 309 (56.49) | 310 (56.67) | |
Stage III | 249 (22.76) | 113 (20.66) | 136 (24.86) | |
Stage IV | 20 (1.83) | 11 (2.01) | 9 (1.65) | |
Unknown | 24 (2.19) | 12 (2.19) | 12 (2.19) | |
T stage | 0.3438 | |||
T1 | 280 (25.59) | 150 (27.42) | 130 (23.77) | |
T2 | 633 (57.86) | 314 (57.4) | 319 (58.32) | |
T3 | 138 (12.61) | 66 (12.07) | 72 (13.16) | |
T4 | 40 (3.66) | 16 (2.93) | 24 (4.39) | |
Unknown | 3 (0.27) | 1 (0.18) | 2 (0.37) | |
N stage | 0.2541 | |||
N0 | 516 (47.17) | 271 (49.54) | 245 (44.79) | |
N1 | 361 (33.00) | 181 (33.09) | 180 (32.91) | |
N2 | 120 (10.97) | 54 (9.87) | 66 (12.07) | |
N3 | 77 (7.04) | 33 (6.03) | 44 (8.04) | |
Unknown | 20 (1.83) | 8 (1.46) | 12 (2.19) | |
M stage | 0.5441 | |||
M0 | 910 (83.18) | 457 (83.55) | 453 (82.82) | |
M1 | 22 (2.01) | 13 (2.38) | 9 (1.65) | |
Unknown | 162 (14.81) | 77 (14.08) | 85 (15.54) |
T, tumor; N, node; M, metastasis.
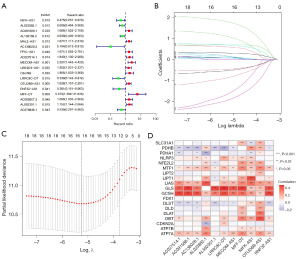
Validation of the risk score system
As shown in Figure 4, we discovered that patients in the high-risk group had a worse prognosis than those in the low-risk group. On this basis, Figure 5 shows the validation of the risk score system, results of the Kaplan-Meier analysis proved that patients in the high-risk group had worse 10-year OS, compared to those in the low-risk group (Figure 5A). This discovery was confirmed in the validation cohort, and the total cohort (Figure 5B,5C). By principal component analysis (PCA), four graphs are used to show the differentiation efficacy of various gene sets (Figure 5D-5G). The results demonstrated that the signature lncRNAs had the strongest differentiation efficacy among all four gene sets (signature lncRNAs, cuproptosis-related lncRNAs, cuproptosis-related genes, and total genes).
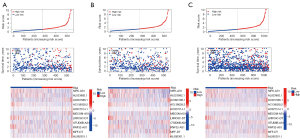
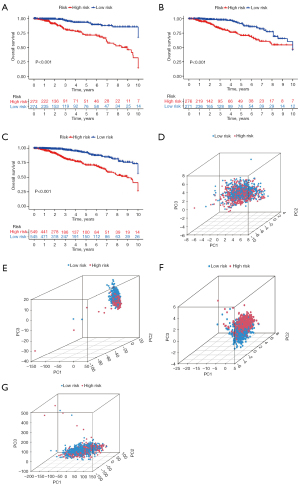
The risk score system has a strong prognostic value for both early and late-stage patients
The prognostic value of the risk score system in different patient subgroups was examined in Figure 6. The result demonstrated that patients in the high-risk group had a worse prognosis compared with those in the low-risk group, in both early and late-stage subgroups (Figure 6A,6B).
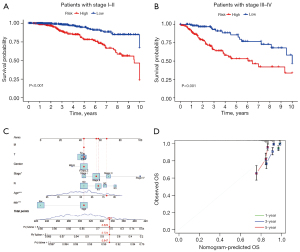
Construction and validation of a nomogram
As shown in Figure 7, the univariate and multivariate cox regressions were used to examine the prognostic significance of variables. The P value of our risk score system was less than 0.05 in both univariate and multivariate regressions, confirming the independence of its prognostic significance (HR =1.025, 95% CI: 1.014–1.036, P<0.001). The remaining independent prognostic factors were age (HR =1.035, 95% CI: 1.022–1.049, P<0.001) and stage (HR =2.168, 95% CI: 1.728–2.719, P<0.001) (Figure 7A,7B). The Kaplan-Meier analysis confirmed the prognostic value of our risk score for PFS (Figure 7C). The receptor operating characteristic curve (ROC) was used to examine the predictive power of independent prognostic factors. With AUC values of 0.753, 0.760, and 0.702, respectively, the results demonstrated that our risk score system had a decent ability to predict patients’ prognosis at 1-, 3-, and 5-year (Figure 7D). Further, the C-index indicated that our risk score system exhibited higher predictive accuracy than other factors (Figure 7E,7F). Based on this, a nomogram was developed to achieve a quantitative forecast of the patient’s prognosis (Figure 6C). Its prediction accuracy was verified in the subsequent Calibration (Figure 6D).
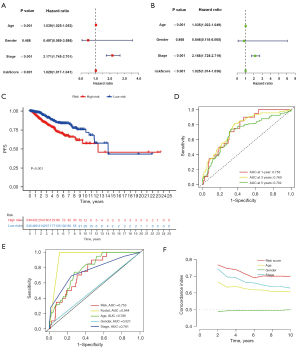
GO and KEGG enrichment analysis
The functional enrichment analysis was performed to investigate which cellular functions and signaling pathways were related to patients’ risk scores in Figure 8. First, 148 differentially expressed genes (DEGs) between high- and low-risk groups were distinctly found. They are shown in Table S1. On this basis, the GO analysis described their significantly enriched molecular function (MF), cellular component (CC), and biological process (BP). In the MF category, they were mainly enriched in “signaling receptor activator activity”, “receptor ligand activity”, and “immunoglobulin receptor binding”. In the CC category, they were mainly enriched in the “external side of plasma membrane”, “apical plasma membrane”, and “immunoglobulin complex”. In the BP category, they were mainly enriched in “activation of immune response”, “B cell activation”, and “immune-response-activating signal transduction” (Figure 8A,8B). Besides, the KEGG analysis revealed that they were significantly enriched in signaling pathways including “neuroactive ligand-receptor interaction”, “primary immune deficiency”, “cytokine-cytokine receptor interaction”, and “B cell receptor signaling pathway” (Figure 8C,8D). Taken together, the two groups showed significantly different levels of immune-related functions and signaling pathways activation.
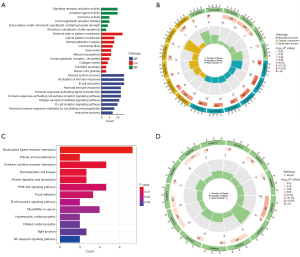
Analysis of immune infiltration and immune-related functions
Using the ssGSEA algorithm, differences in immune functions between the high- and low-risk groups are depicted in Figure 9. The results revealed that “type II IFN response”, “cytolytic activity”, “inflammation-promoting”, “T-cell co-stimulation” and “CCR” were significantly different between the two groups (P<0.05). And they were all inhibited in the high-risk group (Figure 9A,9B). The relationship between risk score and immune checkpoint genes was also examined. The findings showed that 34 immune checkpoint genes substantially differed in expression between high- and low-risk groups (Figure 9C).
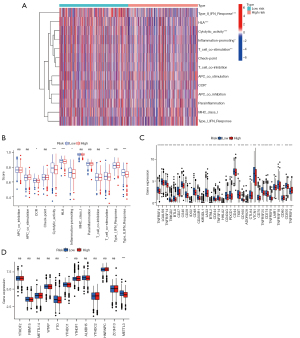
Patients in the high-risk group faced a heavier tumor mutational load
Alteration in cancer-related genes is one of the most important causes of carcinogenesis which is shown in Figure 10. According to different mutation patterns of oncogenes and tumor suppressor genes, such as missense mutations and code-shifting mutations, anti-tumor drugs have been developed. In this study, differences in tumor-related gene mutation patterns between high- and low-risk groups were examined. The results indicated that patients in the high-risk group faced a heavier tumor mutation load (Figure 10A). And the missense mutation made up the highest percentage of all mutation types (Figure 10B,10C). Based on the TMB of patients, they were divided into high TMB and low TMB groups. Kaplan-Meier analysis revealed that patients in the high TMB group had a worse prognosis compared with those in the low TMB group (Figure 10D) (P<0.05).
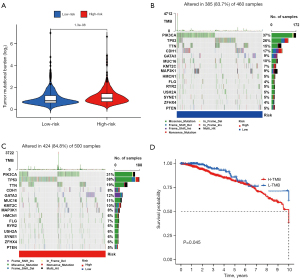
Identification of the relationship between the risk score system and N6-adenylate methylation
The N6-adenylate methylation (m6a) is a form of internal modification of RNAs. Aberrant expression of genes regulating the m6a modifying enzyme has been demonstrated to be closely related to tumorigenesis and progression. In this study, differences in m6a modifier gene expression between the low- and high-risk groups were analyzed. The results indicated that “METTL3” and “YTHDC1” expressed differentially between the two groups (Figure 9D).
Identification of the relationship between the risk score system and drug sensitivity
As shown in Figure 11, the relationship between the risk score system and anticancer drugs was examined, to further validate its clinical use. A total of 251 drugs were included in the analysis, and the results revealed that the sensitivity of 26 of them differed significantly between high- and low-risk groups (P<0.05) (Figure 11).
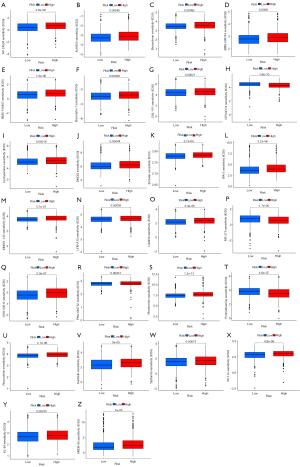
Discussion
The prognosis for breast cancer patients has improved dramatically over the past few decades, but the existence of insurmountable obstacles cannot be denied (16,17). The formidable heterogeneity of breast cancer forbids us from covering all patients with a single treatment strategy (18). Additionally, over the lengthy course of treatment, tumor cells frequently undergo mutation, resulting in resistance to the existing treatment regimens (19). This condition spurs us to delve more deeply into breast tumor cells’ physiological processes and discover the mechanisms underlying their development and death. On the one hand, it aids in the development of novel targeted drugs. On the other hand, oncologists might use prognostic biomarkers or decision support tools to rationally “escalate” or “de-escalate” therapy plans while facing complicated treatment options.
“Resisting cell death” is one of the most important hallmarks of cancer and researches on tumor cell death has always been a hot topic in both basic and clinical settings (20). Cuproptosis is a novel modality of cell death that may shed new light on tumor management (5). In addition, lncRNAs participate in the regulation of cell functions by the means of post-transcriptional modification (21). Cell death-related lncRNAs can act as prognostic biomarkers for cancers and have been used for drug development. However, the relationship between lncRNAs and cuproptosis and the role cuproptosis-related lncRNAs play in breast cancer remain shrouded in the veil.
In this study, we obtained 677 cuproptosis-related lncRNAs in breast cancer and identified 18 prognostic ones by univariate cox regression analysis. After the LASSO regression, 11 of them, including AL023882.1, AC091588.1, AC138028.2, AC027514.1, AL592301.1, LRRC8C-DT, MFF-DT, NIFK-AS1, MECOM-AS1, OTUD6B-AS1, and RNF32-AS1 were selected to develop a risk score system, which was subsequently proven to have an independent prognostic value.
OTUD6B-AS1 (ovarian tumor domain containing 6B antisense RNA1) was reported to be related to the cell death of cancers including bladder cancer, clear renal cell cancer, and breast cancer (22). Xu et al. found that OTUD6B-AS1 was related to ferroptosis and able to influence the immune microenvironment in breast cancer (23). Li et al. reported in their study that OTUD6B-AS1 could promote paclitaxel resistance of triple-negative breast cancers by mediating autophagy and genomic instability (24). Wang et al. reported in their study that OTUD6B-AS1 could promote apoptosis of clear renal cell carcinoma through the Wnt/β-catenin pathway (25). NIFK-AS1 was a lncRNA well-known for its ability to promote polarization of macrophages (26). Chen et al. found that upregulation of NIFK-AS1 could promote the progression of hepatocellular carcinoma and sorafenib resistance (27). LRRC8C-DT was reported to be connected with a prognosis of melanoma patients in the study by Li et al. (28). Unfortunately, there are no relevant studies for the remaining lncRNAs to clarify their functions.
GO and KEGG analysis revealed that cancer signaling pathways and immune-related functions were differentially activated between the high- and low-risk groups. Further analysis revealed that immune processes related to tumor-suppressing including type II IFN response, T-cell co-stimulation, and cytolytic activity were suppressed in varying degrees in the high-risk group (29). In addition, genes of immune checkpoint inhibitors were also found related to the cuproptosis-related lncRNAs. It is well-known that lncRNAs participate in epigenetic modulation through complex mechanisms, which consequently have an impact on the biological behavior of cancer cells. The findings in this study indicated that cuproptosis related lncRNAs were able to modulate a series of signaling pathways, which may further influence cellular functions like immunol response and drug sensitivity. For example, the Akt signaling pathway, which was found to be enriched in this study, has been proven to be able to influence cancer cells’ drug sensitivity in previous studies (30,31). On this basis, we speculate that there must be some unrevealed links between cuproptosis and these cellular functions. In this context, lncRNAs must play an integral role, but the exact mechanisms by which they are involved are still a misery. We believe that a deeper exploration of this issue is certain to novel unprecedented discoveries, and lncRNAs may be a suitable portal for a breakthrough.
Besides, we discovered that patients in the high-risk group faced a high tumor mutational burden, with PIK3CA and P53 as the most frequently mutated genes in breast cancer. Our findings suggested the potential connection between TMB and cuproptosis. Then, we grouped patients according to the TMB and proved its relationship with prognosis. Both cuproptosis and TMB serve as important prognostic factors for breast cancer patients, but we are not able to explore the detailed mechanisms in this article. More preclinical mechanical studies on this basis are wanted. Abnormalities of enzymes that participated in m6a are closely associated with tumorigenesis and progression (32). In this study, we noticed that genes regulating m6a modifying enzymes were closely related to cuproptosis related-lncRNAs, which may provide new targets for future tumor treatment. In addition, the sensitivity of anti-tumor drugs was significantly different between the two groups, indicating the potential clinical value of the risk score system.
Cuproptosis is a novel and promising modality of tumor cell death. However, there is still a long way to go before it can be used in clinical settings (33). In this study, we obtained cuproptosis-related lncRNAs and constructed a prognostic risk score system on this basis, which may be useful for the clinical treatment of breast cancer patients. In addition, we analyzed cellular functions, signaling pathways, immune microenvironment, tumor mutational load, m6a, and drug sensitivity associated with cuproptosis-related lncRNAs, aiming to provide possible targets for the development of novel anti-tumor drugs. However, some limitations of this study cannot be ignored. This is only a study relying on the public database. More preclinical studies are required to explore specific molecular mechanisms, and large clinical trials are needed to further validate the prognostic value of cuproptosis-related lncRNAs.
Conclusions
In this study, we developed a prognostic risk score system for breast cancer patients based on cuproptosis-related lncRNAs and demonstrated that it had satisfactory predictive accuracy. In addition, we discovered that cuproptosis-related lncRNAs were associated with the immune microenvironment, TMB, m6a, and drug sensitivity in breast cancer, which may provide the basis for future anti-tumor drug development.
Acknowledgments
Funding: This study was supported by
Footnote
Reporting Checklist: The authors have completed the TRIPOD reporting checklist. Available at https://tcr.amegroups.com/article/view/10.21037/tcr-22-2702/rc
Peer Review File: Available at https://tcr.amegroups.com/article/view/10.21037/tcr-22-2702/prf
Conflicts of Interest: All authors have completed the ICMJE uniform disclosure form (available at https://tcr.amegroups.com/article/view/10.21037/tcr-22-2702/coif). The authors have no conflicts of interest to declare.
Ethical Statement: The authors are accountable for all aspects of the work in ensuring that questions related to the accuracy or integrity of any part of the work are appropriately investigated and resolved. The study was conducted in accordance with the Declaration of Helsinki (as revised in 2013).
Open Access Statement: This is an Open Access article distributed in accordance with the Creative Commons Attribution-NonCommercial-NoDerivs 4.0 International License (CC BY-NC-ND 4.0), which permits the non-commercial replication and distribution of the article with the strict proviso that no changes or edits are made and the original work is properly cited (including links to both the formal publication through the relevant DOI and the license). See: https://creativecommons.org/licenses/by-nc-nd/4.0/.
References
- Loibl S, Poortmans P, Morrow M, et al. Breast cancer. Lancet 2021;397:1750-69. [Crossref] [PubMed]
- Dave A, Charytonowicz D, Francoeur NJ, et al. The Breast Cancer Single-Cell Atlas: Defining cellular heterogeneity within model cell lines and primary tumors to inform disease subtype, stemness, and treatment options. Cell Oncol (Dordr) 2023; Epub ahead of print. [Crossref] [PubMed]
- Fahad Ullah M. Breast Cancer: Current Perspectives on the Disease Status. Adv Exp Med Biol 2019;1152:51-64. [Crossref] [PubMed]
- Verret B, Cortes J, Bachelot T, et al. Efficacy of PI3K inhibitors in advanced breast cancer. Ann Oncol 2019;30:x12-20. [Crossref]
- Tsvetkov P, Coy S, Petrova B, et al. Copper induces cell death by targeting lipoylated TCA cycle proteins. Science 2022;375:1254-61. [Crossref] [PubMed]
- Przanowska RK, Weidmann CA, Saha S, et al. Distinct MUNC lncRNA structural domains regulate transcription of different promyogenic factors. Cell Rep 2022;38:110361. [Crossref] [PubMed]
- Aich M, Chakraborty D. Role of lncRNAs in stem cell maintenance and differentiation. Curr Top Dev Biol 2020;138:73-112. [Crossref] [PubMed]
- Dong F, Ruan S, Wang J, et al. M2 macrophage-induced lncRNA PCAT6 facilitates tumorigenesis and angiogenesis of triple-negative breast cancer through modulation of VEGFR2. Cell Death Dis 2020;11:728. [Crossref] [PubMed]
- Liu S, Sun Y, Hou Y, et al. A novel lncRNA ROPM-mediated lipid metabolism governs breast cancer stem cell properties. J Hematol Oncol 2021;14:178. [Crossref] [PubMed]
- Zhao W, Geng D, Li S, et al. LncRNA HOTAIR influences cell growth, migration, invasion, and apoptosis via the miR-20a-5p/HMGA2 axis in breast cancer. Cancer Med 2018;7:842-55. [Crossref] [PubMed]
- Dong S, Ma M, Li M, et al. LncRNA MEG3 regulates breast cancer proliferation and apoptosis through miR-141-3p/RBMS3 axis. Genomics 2021;113:1689-704. [Crossref] [PubMed]
- Wang J, Xie S, Yang J, et al. The long noncoding RNA H19 promotes tamoxifen resistance in breast cancer via autophagy. J Hematol Oncol 2019;12:81. [Crossref] [PubMed]
- Yan H, Luo B, Wu X, et al. Cisplatin Induces Pyroptosis via Activation of MEG3/NLRP3/caspase-1/GSDMD Pathway in Triple-Negative Breast Cancer. Int J Biol Sci 2021;17:2606-21. [Crossref] [PubMed]
- Mao C, Wang X, Liu Y, et al. A G3BP1-Interacting lncRNA Promotes Ferroptosis and Apoptosis in Cancer via Nuclear Sequestration of p53. Cancer Res 2018;78:3484-96. [Crossref] [PubMed]
- Shi Q, Li Y, Li S, et al. LncRNA DILA1 inhibits Cyclin D1 degradation and contributes to tamoxifen resistance in breast cancer. Nat Commun 2020;11:5513. [Crossref] [PubMed]
- Sung H, Ferlay J, Siegel RL, et al. Global Cancer Statistics 2020: GLOBOCAN Estimates of Incidence and Mortality Worldwide for 36 Cancers in 185 Countries. CA Cancer J Clin 2021;71:209-49. [Crossref] [PubMed]
- Waks AG, Winer EP. Breast Cancer Treatment: A Review. JAMA 2019;321:288-300. [Crossref] [PubMed]
- Liang Y, Zhang H, Song X, et al. Metastatic heterogeneity of breast cancer: Molecular mechanism and potential therapeutic targets. Semin Cancer Biol 2020;60:14-27. [Crossref] [PubMed]
- Kaur P, Campo D, Porras TB, et al. A Pilot Study for the Feasibility of Exome-Sequencing in Circulating Tumor Cells Versus Single Metastatic Biopsies in Breast Cancer. Int J Mol Sci 2020;21:4826. [Crossref] [PubMed]
- Strasser A, Vaux DL. Cell Death in the Origin and Treatment of Cancer. Mol Cell 2020;78:1045-54. [Crossref] [PubMed]
- Statello L, Guo CJ, Chen LL, et al. Gene regulation by long non-coding RNAs and its biological functions. Nat Rev Mol Cell Biol 2021;22:96-118. [Crossref] [PubMed]
- Wang Y, Yang T, Han Y, et al. lncRNA OTUD6B-AS1 Exacerbates As(2)O(3)-Induced Oxidative Damage in Bladder Cancer via miR-6734-5p-Mediated Functional Inhibition of IDH2. Oxid Med Cell Longev 2020;2020:3035624. [Crossref] [PubMed]
- Xu Z, Jiang S, Ma J, et al. Comprehensive Analysis of Ferroptosis-Related LncRNAs in Breast Cancer Patients Reveals Prognostic Value and Relationship With Tumor Immune Microenvironment. Front Surg 2021;8:742360. [Crossref] [PubMed]
- Li PP, Li RG, Huang YQ, et al. LncRNA OTUD6B-AS1 promotes paclitaxel resistance in triple negative breast cancer by regulation of miR-26a-5p/MTDH pathway-mediated autophagy and genomic instability. Aging (Albany NY) 2021;13:24171-91. [Crossref] [PubMed]
- Wang G, Zhang ZJ, Jian WG, et al. Novel long noncoding RNA OTUD6B-AS1 indicates poor prognosis and inhibits clear cell renal cell carcinoma proliferation via the Wnt/β-catenin signaling pathway. Mol Cancer 2019;18:15. [Crossref] [PubMed]
- Zhou YX, Zhao W, Mao LW, et al. Long non-coding RNA NIFK-AS1 inhibits M2 polarization of macrophages in endometrial cancer through targeting miR-146a. Int J Biochem Cell Biol 2018;104:25-33. [Crossref] [PubMed]
- Chen YT, Xiang D, Zhao XY, et al. Upregulation of lncRNA NIFK-AS1 in hepatocellular carcinoma by m(6)A methylation promotes disease progression and sorafenib resistance. Hum Cell 2021;34:1800-11. [Crossref] [PubMed]
- Li FW, Luo SK. Identification and Construction of a Predictive Immune-Related lncRNA Signature Model for Melanoma. Int J Gen Med 2021;14:9227-35. [Crossref] [PubMed]
- Castro F, Cardoso AP, Gonçalves RM, et al. Interferon-Gamma at the Crossroads of Tumor Immune Surveillance or Evasion. Front Immunol 2018;9:847. [Crossref] [PubMed]
- Vitale SR, Martorana F, Stella S, et al. PI3K inhibition in breast cancer: Identifying and overcoming different flavors of resistance. Crit Rev Oncol Hematol 2021;162:103334. [Crossref] [PubMed]
- Zhou G, Zhang F, Guo Y, et al. miR-200c enhances sensitivity of drug-resistant non-small cell lung cancer to gefitinib by suppression of PI3K/Akt signaling pathway and inhibites cell migration via targeting ZEB1. Biomed Pharmacother 2017;85:113-9. [Crossref] [PubMed]
- Lan Q, Liu PY, Bell JL, et al. The Emerging Roles of RNA m(6)A Methylation and Demethylation as Critical Regulators of Tumorigenesis, Drug Sensitivity, and Resistance. Cancer Res 2021;81:3431-40. [Crossref] [PubMed]
- Tang D, Chen X, Kroemer G. Cuproptosis: a copper-triggered modality of mitochondrial cell death. Cell Res 2022;32:417-8. [Crossref] [PubMed]