Value of a combined magnetic resonance imaging-based radiomics-clinical model for predicting extracapsular extension in prostate cancer: a preliminary study
Highlight box
Key findings
• We found that a combined model considering MRI-based radiomics and clinical variables had a satisfactory predictive value for ECE in PCa patients.
What is known and what is new?
• MRI and clinical variables are commonly used for clinical evaluation in the ECE of PCa, but there is a problem of misdiagnosis or missed diagnosis. There is a need for a more effective diagnostic method to predict ECE.
• In this study, we constructed a predictive model combining radiomics and clinical parameters and found its diagnostic efficacy to be superior to the clinical model, Mehralivand grade, and radiomics model.
What is the implication, and what should change now?
• The combined model we have constructed may help in the diagnosis of ECE in PCa patients, but still needs to be validated with large data.
Introduction
Prostate cancer (PCa) is a common genitourinary malignancy in men and the leading cause of cancer incidence and mortality (1). Radical prostatectomy (RP) is the preferred treatment for patients with localized PCa (2). While achieving the goal of tumor resection, the preservation of erectile function and urinary continence is also paramount. However, the choice of preserving the neurovascular bundle around the prostate depends on the presence of extracapsular extension (ECE) of PCa (3). ECE is closely associated with adverse pathologies such as positive surgical margins, biochemical recurrence and progression, and an increased risk of PCa-related mortality (4). Therefore, accurate preoperative prediction of ECE is a key step to optimize treatment for patients with PCa, and has an important impact on clinical decision making and prognosis of PCa patients.
From an image perspective, magnetic resonance imaging (MRI) can aid in diagnosing ECE by clearly displaying the anatomical morphology of the prostate and lesions with high soft tissue resolution (5). Due to the different signs of ECE on MRI images and the lack of standardized description of ECE, the diagnostic accuracy and sensitivity of different experienced radiologists vary greatly (6). Some scholars proposed to use structured reports to describe ECE, which mainly include Likert score, the European Society of Urogenital Radiology (ESUR) score, and the Mehralivand grade. Among them, Mehralivand grade had the highest correlation with pathological ECE and the best diagnostic performance with its simplicity and little imaging features (7). Even so, Mehralivand grade is only dependent on the subjective assessment of radiologists by the relatively limited imaging features, and the diagnostic performance of ECE remains limited in practice (8).
Radiomics has been increasingly used in medical imaging as an emerging research technology in recent years. It enables the extraction of many diagnostic features (kurtosis, skewness, wavelet, etc.) from specific areas of the medical image through data characterization algorithms to make an objective and quantitative analysis of the images. Medical images can be transformed into minable data in the form of features by radiomics, which can provide valuable information for clinical decision-making (9,10). Relevant research results have shown that radiomics has broad application prospects in the detection, grading, and invasiveness prediction of PCa (11,12). Qi et al. (13) established a radiomics model in patients with prostate-specific antigen (PSA) level of 4–10 ng/mL, which could provide potential preoperative prediction for PCa patients and help to reduce unnecessary biopsies. A study conducted comparative analysis based on radiomics model and the Prostate Imaging Report and Data System (PI-RADS) score in PCa diagnosis and pathological grading, which verified the excellent diagnostic efficacy of radiomics model in distinguishing cancerous and non-cancerous prostate lesions and high or low grade PCa lesions (14). Previous fundings also showed that radiomics features hold promise for the prediction of the therapy response in the radioligand therapy of PCa patients by positron emission tomography/computed tomography (PET/CT) (15,16). In summary, the potential application value of radiomics in ECE is promising. However, there are few studies on the applications of radiomics in the preoperative prediction of ECE in PCa patients.
This study aimed to evaluate the predictive value of a combined model of MRI-based radiomics and clinical variables for predicting ECE in PCa, and further compare it with the radiologists’ assessments using Mehralivand grade. We present this article in accordance with the TRIPOD reporting checklist (available at https://tcr.amegroups.com/article/view/10.21037/tcr-22-2750/rc).
Methods
Patients
This study was conducted in accordance with the Declaration of Helsinki (as revised in 2013). This study was approved by the Ethics Committee of The First Affiliated Hospital of Soochow University (No. 262; 2021) and individual consent for this retrospective analysis was waived.
A total of 557 patients with pathologically confirmed PCa underwent preoperative 3.0-T MRI followed by RP between January 2018 to March 2022 at The First Affiliated Hospital of Soochow University, out of which 392 patients were retrospectively enrolled in this study. The inclusion criteria were as follows: (I) all patients received RP and had confirmed PCa; (II) patients with detailed and complete clinical data, imaging information, and pathological reports; and (III) prostate MRI was performed within one month before RP. The exclusion criteria were as follows: (I) patients with an incomplete pathological report and no precise diagnosis of ECE (n=78); (II) the patients who received prior therapies (such as biopsy, surgery, radiation therapy, or hormonal therapy) before MRI (n=51); (III) poor image quality or severe motion artifacts, which affect observation (n=21); and (IV) the lesion volume is too small (diameter <3 mm) to define the boundary of the region of interest (ROI) (n=15). Figure 1 shows a flowchart depicting the patient recruitment in this study.
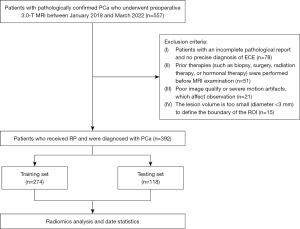
The clinical variables, including age, PSA at admission, lesion location distribution, and biopsy Gleason group (GG) for each patient, were obtained from the medical records. Further, the PI-RADS v2.1 scores were assessed by radiologists (with more than 10 years of prostate MRI experience). The lesion location distribution included the peripheral zone (PZ), transitional zone (TZ), or PZ + TZ. The biopsy pathology GG was divided into five grades according to the 2014 International Society of Urological Pathology (ISUP) guidelines (17).
Standard of reference
The final histopathologic assessment after the RP procedure was defined as the standard reference. One dedicated pathologist (with more than 10 years of experience) blinded to the MRI reports reviewed all the specimens according to a standard protocol and filled out a structured pathological report per the clinical routine. The pathologist then recorded the ECE status for each lesion. Another senior urologist (with more than 10 years of experience) coordinated the workflow to ensure the tumor depicted by the pathologist matched the lesion analyzed by the radiologist. Only lesions that were visible on MRI images were finally enrolled in subsequent analysis. According to the measure of ISUP (17), ECE is defined as the presence of tumors beyond the borders of the prostate.
Scanning equipment and parameters
A 3.0-T superconducting MR scanner (Skyra; Siemens, Munich, Germany) with an abdominal 32-channel surface phased line coil was used to perform prostate MRI. Patients were taken in a supine position with the head advanced. The scanning sequence included axial T1-weighted imaging (T1WI), axial T2-weighted imaging (T2WI) (no fast-saturated), sagittal T2WI, coronal T2WI, and diffusion-weighted imaging (DWI) (b=100, 1,000, 1,500 s/mm2). Based on the DWI images of b=1,500 s/mm2, the apparent diffusion coefficient (ADC) icons were calculated by an extended single exponential fitting model. The specific scanning parameters were recommended by PI-RADS v2.1 (18). The detailed scan parameters are shown in Table 1.
Table 1
Sequence | Repetition (ms) | Echo time (ms) | Layer thickness (mm) | Interlayer spacing (mm) | Field of view (mm × mm) |
---|---|---|---|---|---|
Axial T1WI | 680.00 | 13.00 | 5 | 0.50 | 380×380 |
Axial T2WI | 6,980.00 | 104.00 | 3 | 0 | 200×200 |
Sagittal T2WI | 3,900.00 | 89.00 | 3 | 0.45 | 200×200 |
Coronal T2WI | 3,500.00 | 85.00 | 3 | 0.60 | 220×220 |
DWI | 5,000.00 | 72.00 | 3 | 0 | 288×288 |
MRI, magnetic resonance imaging; T1WI, T1-weighted imaging; T2WI, T2-weighted imaging; DWI, diffusion-weighted imaging.
Mehralivand grade
Two radiologists (reader 1 and 2), with 6–8 years of prostate MRI experience, assessed ECE by Mehralivand grade based on T2WI, ADC, and DWI images without obtaining pathological results. The assessment of radiologist is mainly based on T2WI images, assisted by DWI and ADC images to determine the boundary between the lesion and the prostate capsule. If a patient has multiple lesions, each lesion of the patient is evaluated separately, and the highest grade among the multiple lesions is selected as the final assessment of the patient.
A staging assessment of ECE was performed using a criteria-based grading system for the Mehralivand grade introduced by Mehralivand et al. (19). Imaging diagnosis of ECE was based on a four-level grading approach using capsular contact length (CCL) of 15 mm or greater, capsular irregularity or bulge, and frank breach of the capsule: (I) grade 0, no capsular contact or CCL less than 15 mm; (II) grade 1, either CCL of 15 mm or greater, or capsular irregularity or bulge; (III) grade 2, both CCL of 15 mm or greater, and capsular irregularity or bulge; and (IV) grade 3, frank breach of the capsule at MRI with tumor extension into the periprostatic space or invasion of adjacent anatomic structures. Each scoring example is shown in Figure 2.
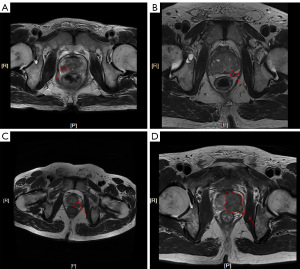
Radiomics analysis
Tumor segmentation
The axial T2WI, high b-value (1,500 s/mm2) DWI, and ADC images were the target for radiomics analysis. Firstly, different target images of the same patient were matched spatially by Elastix software package (v. 4.10, http://elastix.isi.uu.nl/index.php). Based on T2WI images, DWI and ADC images were registered successively, which could ensure that three sequences had the same resolution, field of vision and orientation. Secondly, the processed T2WI, DWI and ADC images (nii format) were imported into ITK-SNAP software (v. 4.7.2; https://itk.org/) for lesion segmentation. A radiologist with 6 years of prostate MRI experience (reader 3) manually outlined the ROI on the T2WI, ADC, and DWI images without obtaining pathological results. Then, the total volume of interest (VOI) of the tumor was obtained. Finally, the results of the segmentation were reviewed by a senior radiologist who had 12 years of prostate MRI experience (reader 4). For some controversial lesions, readers 3 and 4 determined after consultation.
To ensure the stability and repeatability of ROI, reader 4 randomly selected 50 lesions in the enrolled cases to draw ROI, and reader 3 randomly selected 50 lesions to repeat the ROI one month later. The inter-observer and intra-observer correlation coefficients were calculated.
Feature extraction
Before feature extraction, the images of each patient were standardized to improve the texture recognition rate. The T2WI, DWI, and ADC images of each patient were firstly resampled to 1×1×1 cm3 voxel sizes to normalize the voxel spacing. Then, the voxel intensity discretization was accomplished by fixing the bin width at 25 Hounsfield unit (HU) to reduce imaging noise and standardize the intensity. At last, Z-score normalization was carried out for different sequences of each patient to reduce the impact of inconsistent image parameters on the changes of radiomics features, and the voxel intensity was converted into a distribution with a mean of 0 and a standard deviation of 1 (20). After image preprocessing completed, FeAture Explorer (FAE, v.0.4.0), an open-source radiomics software based on pyradiomics software package (21), was used to extract features from VOI of each sequence. Based on T2WI, DWI, and ADC sequences, 851 radiomics features were extracted from the VOI of each sequence of each lesion separately. There were a total of 2,553 radiomics features, including 42 morphology features, 54 first-order statistics features, 15 neighboring gray level dependence matrix (NGLDM) features, 42 gray level dependence matrix (GLDM) features, 48 gray level size zone matrix (GLSZM) features, 48 gray level run length matrix (GLRLM) features, 72 gray level co-occurrence matrix (GLCM) features, and 2,232 wavelet transform features.
Feature selection and radiomics model construction
All samples were randomly divided into a training set and validation set at a ratio of 7:3. To remove the unbalance of sample, up-sampling was performed by repeating random cases to make positive/negative samples balance. The Z-score method was applied to normalize the extracted features again by subtracting the mean value and dividing it by the standard deviation. The dimension reduction was then applied to the normalized features. The Pearson correlation coefficient (PCC) was estimated on each pair of two features, and the ones with a value larger than 0.9 were dropped. Analysis of recursive feature elimination (RFE) was used to select features, and the F-value of each feature was calculated based on the label. Only the top 20 features were reserved. Finally, the different classifiers, including least absolute shrinkage and selection operator (LASSO), decision tree (DT), logistics regression (LR), random forest (RF) and support vector machine (SVM), were trained on the selected features to build the radiomics model. The optimal model parameters were chosen by 10-fold cross-validation and finally the predictive value of the radiomics model was obtained through the linear combination of the coefficients weighted by the selected radiomics features, so as to quantify the diagnostic efficiency. All the experiments above were run in FAE (21).
Construction of the clinical model and combined radiomics-clinical model
The clinical variables (including age, PSA at admission, lesion location, biopsy GG, and PI-RASD v2.1 score for each patient) were first evaluated by the univariate analysis. Then, the multivariate binary LR analysis was used to screen out independent risk factors from the significant clinical variables in the univariate analysis. The clinical model was built by the independent risk factors.
Moreover, a combined radiomics-clinical model was constructed by integrating the independent risk factors in clinical model and the predictive value of ECE in radiomics model. Meanwhile, the nomogram for the prediction of ECE was constructed based on the combined model.
Statistical analysis
SPSS 25.0 and MedCalc 18.0 software were used for statistical analysis. The counting data were compared by the chi-square test. The Shapiro-Wilk test was performed to test whether the measurement data was a normal distribution. Then, an independent sample t-test or Mann-Whitney U test was conducted. Parameters including the receiver operating characteristic (ROC) curve, the area under the curve (AUC), 95% confidence interval (CI), sensitivity, specificity, positive predictive value (PPV), negative predictive value (NPV), and accuracy were all used to evaluate the performance in above models. DeLong test was used to compare the AUCs among these models, and the Hosmer-Lemeshow test was used to verify the agreement between the combined model and the pathological results. Finally, decision curve analysis (DCA) was used to quantify the net clinical benefits of each predictive method. A P<0.05 was considered statistically significant.
Results
Baseline characteristics
A total of 392 PCa patients who received RP were enrolled in this study, with 180 (45.9%) lesions having pathologically confirmed ECE. General data of patients in the training set and validation set are summarized in Table 2. There was no statistical significance in the age, PSA, lesion location distribution, PI-RADS v2.1 score, Mehralivand grade, biopsy GG, and the final pathological result of ECE between the two groups (P>0.05).
Table 2
Variables | Total | Training set | Validation set | P value |
---|---|---|---|---|
N (%) | 392 | 274 (69.9) | 118 (30.1) | – |
Age (years), median [IQR] | 70 [66, 75] | 71 [66, 75] | 72 [67, 75] | 0.290* |
PSA (ng/mL), median [IQR] | 16.55 [9.26, 33.05] | 15.89 [9.25, 29.91] | 17.40 [9.45, 36.69] | 0.378* |
Lesion location distribution, n (%) | 0.747# | |||
PZ | 227 (57.9) | 160 (58.4) | 67 (56.8) | |
TZ | 113 (28.8) | 80 (29.2) | 33 (28.0) | |
PZ + TZ | 52 (13.3) | 34 (12.4) | 18 (15.3) | |
PI-RADS v2.1 score, n (%) | 0.295# | |||
2 | 38 (9.7) | 24 (8.8) | 14 (11.9) | |
3 | 45 (11.5) | 27 (9.9) | 18 (15.3) | |
4 | 85 (21.7) | 62 (22.6) | 23 (19.5) | |
5 | 224 (57.1) | 161 (58.8) | 63 (53.4) | |
Mehralivand grade of reader 1, n (%) | 0.808# | |||
0 | 102 (26.0) | 70 (25.5) | 32 (27.1) | |
1 | 105 (26.8) | 72 (26.3) | 33 (28.0) | |
2 | 130 (33.2) | 95 (34.7) | 35 (29.7) | |
3 | 55 (14.0) | 37 (13.5) | 18 (15.3) | |
Mehralivand grade of reader 2, n (%) | 0.454# | |||
0 | 111 (28.3) | 71 (25.9) | 40 (33.9) | |
1 | 89 (22.7) | 64 (23.4) | 25 (21.2) | |
2 | 118 (30.1) | 85 (31.0) | 33 (28.0) | |
3 | 74 (18.9) | 54 (19.7) | 20 (16.9) | |
Biopsy GG, n (%) | 0.917# | |||
GG ≤3 | 249 (63.5) | 175 (63.9) | 74 (62.7) | |
GG ≥4 | 143 (36.5) | 99 (36.1) | 44 (37.3) | |
Final pathologic result of ECE, n (%) | 1.000# | |||
ECE positive | 180 (45.9) | 126 (46.0) | 54 (45.8) | |
ECE negative | 212 (54.1) | 148 (54.0) | 64 (54.2) |
*, Mann-Whitney U test; #, chi-squared test. IQR, interquartile range; PSA, prostate specific antigen; PZ, peripheral zone; TZ, transition zone; PI-RADS, prostate imaging reporting and data system; GG, Gleason group; ECE, extracapsular extension.
Performance of clinical model and Mehralivand grade
After univariate and multivariate binary LR analyses, PSA, lesion location, biopsy GG, and PI-RADS v2.1 score were all independent risk factors of ECE (P<0.05) (Table 3) and were used to build a clinical model. The AUC, sensitivity, specificity, and accuracy of the clinical model in the validation set were 0.749 (95% CI: 0.658–0.840), 87.0%, 56.3%, and 70.3%, respectively, which were shown in Figure 3 and Table 4.
Table 3
Variables | ECE positive (n=180) | ECE negative (n=212) | Univariate analysis | Multivariate binary logistics regression | |||
---|---|---|---|---|---|---|---|
t/Z/χ2 | P value | Odds ratio (95% CI) | P value | ||||
PSA (ng/mL), median [IQR] | 25.84 [13.4, 50.1] | 12.16 [7.81, 20.6] | −4.567 | <0.001* | 1.017 (1.003–1.031) | 0.015 | |
Age (years), median [IQR] | 72 [66, 76] | 71 [66, 75] | −1.404 | 0.128* | – | – | |
PI-RADS v2.1 score, n (%) | 82.108 | <0.001# | 1.521 (1.069–2.165) | 0.020 | |||
2 | 3 (1.7) | 35 (16.5) | |||||
3 | 8 (4.4) | 37 (17.5) | |||||
4 | 23 (12.8) | 62 (29.2) | |||||
5 | 146 (81.1) | 78 (36.8) | |||||
Biopsy GG, n (%) | 49.270 | <0.001# | 2.038 (1.135–3.661) | 0.017 | |||
GG ≤3 | 81 (45.0) | 168 (79.2) | |||||
GG ≥4 | 99 (55.0) | 44 (20.8) | |||||
Lesion location distribution, n (%) | 41.670 | <0.001# | 1.287 (1.066–1.553) | 0.024 | |||
PZ | 96 (53.3) | 131 (61.8) | |||||
TZ | 39 (21.7) | 74 (34.9) | |||||
PZ + TZ | 45 (25.0) | 7 (3.3) |
*, Mann-Whitney U test; #, chi-squared test. ECE, extracapsular extension; CI, confidence interval; PSA, prostate specific antigen; IQR, interquartile range; PI-RADS, prostate imaging reporting and data system; GG, Gleason group; PZ, peripheral zone; TZ, transition zone.
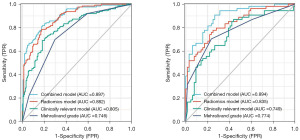
Table 4
Groups | AUC (95% CI) | Sensitivity (%) | Specificity (%) | PPV (%) | NPV (%) | Accuracy (%) | P value (vs. combined model) |
---|---|---|---|---|---|---|---|
Training set | |||||||
Combined model | 0.897 (0.861–0.934) | 81.7 (103/126) | 81.8 (121/148) | 79.2 (103/130) | 84.0 (121/144) | 81.8 (224/274) | – |
Radiomics model | 0.882 (0.844–0.921) | 78.6 (99/126) | 82.4 (122/148) | 79.2 (99/125) | 81.9 (122/149) | 80.7 (221/274) | 0.567 |
Clinical model | 0.805 (0.753–0.857) | 69.0 (87/126) | 81.8 (121/148) | 76.3 (87/114) | 75.6 (121/160) | 75.9 (208/274) | 0.005 |
Mehralivand grade | 0.746 (0.690–0.801) | 69.8 (88/126) | 70.3 (104/148) | 66.7 (88/132) | 73.2 (104/142) | 70.1 (192/274) | <0.001 |
Validation set | |||||||
Combined model | 0.894 (0.837–0.950) | 90.7 (49/54) | 73.4 (47/64) | 74.2 (49/66) | 90.4 (47/52) | 81.4 (96/118) | – |
Radiomics model | 0.835 (0.763–0.908) | 77.8 (42/54) | 75.0 (48/64) | 72.4 (42/58) | 80.0 (48/60) | 76.3 (90/118) | 0.167 |
Clinical model | 0.749 (0.658–0.840) | 87.0 (47/54) | 56.3 (36/64) | 62.7 (47/75) | 83.7 (36/43) | 70.3 (83/118) | 0.005 |
Mehralivand grade | 0.774 (0.691–0.857) | 70.4 (38/54) | 76.6 (49/64) | 71.7 (38/53) | 75.4 (49/65) | 73.7 (87/118) | 0.010 |
AUC, area under the curve; CI, confidence interval; PPV, positive predictive value; NPV, negative predictive value.
The inter-observer consistency for assessment of Mehralivand grade in terms of Kappa value was 0.887, indicating a strong consistency between readers 1 and 2. The Mehralivand grade of reader 1 was selected as the final for the following analysis. The AUC, sensitivity, specificity, and accuracy of radiologists’ Mehralivand grade in the validation set were 0.774 (95% CI: 0.691–0.857), 70.4%, 76.6%, and 73.7%, respectively. The performance of the Mehralivand grade in predicting ECE was shown in Figure 3 and Table 4.
Performance of radiomics model and combined model
The reliability of the radiomics features was quantified by inter-observer (as determined by readers 3 and 4), and intra-observer statistics (as determined by reader 3 twice) tested based on all radiomics features. The mean inter-observer reliability was 0.805 (95% CI: 0.702–0.869), and the mean intra-observer reliability was 0.859 (95% CI: 0.784–0.913), indicating that the repeatability of feature extraction was fine.
After the features normalization of Z-score and the redundant features remotion of RFE, different classifiers (including LASSO, DT, LR, RF, and SVM) were used to select the optimal radiomics model. The performance of the radiomics model using different classifiers in the training set and validation set was shown in Table 5. Based on the validation set, the AUC of the radiomics model constructed by LASSO classifier was 0.835 (95% CI: 0.763–0.908), which was higher than that of other classifiers. And the sensitivity, specificity, and accuracy of radiomics model were 77.8%, 75.0%, and 76.3%, respectively (Figure 3 and Table 4). In the LASSO classifier analysis, 20 radiomics features were selected to build the final radiomics model. The chosen features incorporated nine from ADC, six from DWI, and five from T2WI, which were all shown in Table 6. Meanwhile, based on the validation set, the AUC of the radiomics model was significantly higher than that of the clinical model and Mehralivand grade (both P<0.05).
Table 5
Classifiers | Training set | Validation set | |||||
---|---|---|---|---|---|---|---|
AUC (95% CI) | Sensitivity (%) | Specificity (%) | AUC (95% CI) | Sensitivity (%) | Specificity (%) | ||
LASSO | 0.882 (0.844–0.921) | 78.6 | 82.4 | 0.835 (0.763–0.908) | 77.8 | 75.0 | |
DT | 1.000 (1.000–1.000) | 100.0 | 100.0 | 0.619 (0.531–0.706) | 51.9 | 71.9 | |
LR | 0.881 (0.843–0.921) | 77.0 | 83.1 | 0.828 (0.754–0.902) | 77.8 | 75.0 | |
RF | 1.000 (1.000–1.000) | 100.0 | 100.0 | 0.804 (0.725–0.883) | 70.4 | 76.6 | |
SVM | 0.879 (0.838–0.919) | 78.6 | 84.5 | 0.826 (0.750–0.901) | 79.6 | 75.0 |
AUC, area under the curve; CI, confidence interval; LASSO, least absolute shrinkage and selection operator; DT, decision tree; LR, logistics regression; RF, random forest; SVM, support vector machine.
Table 6
Sequence | Category | Radiomics features | Coefficient |
---|---|---|---|
ADC | GLCM | Correlation | 0.613772031 |
GLRLM | Run entropy | 0.956982134 | |
First-order | Mean | 0.136096474 | |
GLSZM | Small area emphasis | 0 | |
GLSZM | Size zone nonuniformity normalized | −0.328943021 | |
First-order | Skewness | 0.367917783 | |
GLCM | Maximum probability | −0.334694605 | |
First-order | 10th percentile | −0.679633939 | |
First-order | Interquartile range | −0.39148876 | |
DWI | GLDM | Busyness | 0.258039214 |
GLSZM | Size zone nonuniformity | 0.676713449 | |
GLSZM | Low gray level zone emphasis | −0.627712659 | |
GLSZM | Small area high gray level emphasis | −0.059995032 | |
GLSZM | Small area emphasis | 0.229409054 | |
GLSZM | Small area low gray level emphasis | 0.047497771 | |
T2WI | GLSZM | Small area low gray level emphasis | 0.273222621 |
First-order | Kurtosis | −0.128990984 | |
First-order | Skewness | 0.197613557 | |
GLRLM | Long run high gray level emphasis | −0.401781693 | |
GLCM | MCC | 0.303335205 | |
ADC | GLCM | Correlation | 0.613772031 |
GLRLM | Run entropy | 0.956982134 | |
First-order | Mean | 0.136096474 | |
GLSZM | Small area emphasis | 0 | |
GLSZM | Size zone nonuniformity normalized | −0.328943021 | |
First-order | Skewness | 0.367917783 | |
GLCM | Maximum probability | −0.334694605 |
ADC, apparent diffusion coefficient; DWI, diffusion-weighted imaging; T2WI, T2-weighted imaging; GLCM, gray level co-occurrence matrix; GLRLM, gray level run length matrix; GLSZM, gray level size zone matrix; GLDM, gray level dependence matrix; MCC, Matthews correlation coefficient.
Finally, the AUC, sensitivity, specificity, and accuracy of the combined model in the validation set were 0.894 (95% CI: 0.837–0.950), 90.7%, 73.4%, and 81.4%, respectively. The performance of the combined model was listed in Figure 3 and Table 4. Based on the validation set, the predictive performance in the combined model was comparable to the radiomics model (P>0.05, 0.894 vs. 0.835), but the sensitivity (90.7% vs. 77.8%) and accuracy (81.4% vs. 76.3%) were higher in the combined model. Meanwhile, the nomogram for the prediction of ECE was constructed based on the combined model, and a good agreement between the combined model and the pathological results was verified by the Hosmer-Lemeshow test (Figure 4). At last, the DCA implied that the combined model could obtain the maximum net clinical benefits compared with the clinical model, Mehralivand grade, and the radiomics model (Figure 5).
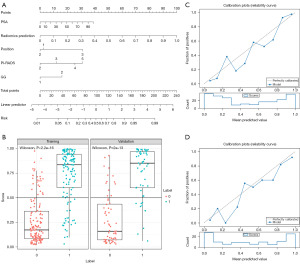
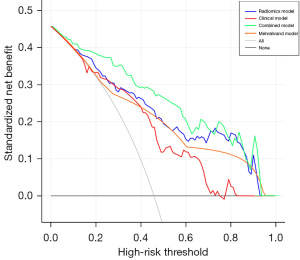
Discussion
In this study, a combined model was constructed based on MRI radiomics and clinical variables for predicting the risk probability of ECE in PCa and developing an individualized and accurate diagnosis or treatment for PCa patients with better prognoses. The study has established the potential value of the combined model in predicting the ECE of PCa. The combined model performed well in the validation set with an AUC of 0.894, and offered the maximum net clinical benefits among other models.
Previous studies have shown that ECE of PCa is closely related to the choice of treatment options and prognosis of patients, especially for disease recurrence and survival following RP (3,4,22). Once ECE is suspected, nerves and blood vessels may be removed to avoid an adverse prognosis (23). The age, PSA, lesion location distribution, percent of biopsy cores positive, biopsy GG, and clinical stage of PCa patients are all closely related to the prognosis of patients (24). Therefore, researchers have built clinical models based on clinical and pathological variables to evaluate preoperative ECE in patients with PCa, such as the Paitin table (25), the Cancer of the Prostate Risk Assessment (CAPRA) score (26), and the Memorial Sloan Kettering Cancer Center (MSKCC) nomogram (27), etc. However, the diagnostic efficiency of the above models varied, with the AUC ranging from 0.609 to 0.805 (28). Krishna et al. (29) used PI-RADS v2 to assess ECE by measuring tumor size and CCL and analyzing the ADC icons of the lesion. The results found a strong correlation between the PI-RADS v2 score and ECE, with an AUC of 0.72. Matsuoka et al. also found that the PI-RADS score could improve the assessment of the clinical stage of the tumor and increase the consistency of ECE assessment among observers (30). Thus, in this study, the PI-RADS v2.1 score was included in clinical variables and it also verified as an independent risk factor of ECE. However, the AUC of the clinical model in the validation set was 0.749, and the accuracy was only 70.3%, roughly similar to the previous studies, indicating the limited value of the clinical model in practice.
Regarding the preoperative diagnosis of ECE in PCa patients, MRI has high diagnostic specificity but low sensitivity. The results also depend a lot on the different experiences of radiologists (31). PI-RADS v2.1 suggests some imaging signs of ECE, including lesion proximity to prostate capsular, irregular or thickened peripheral neurovascular bundles, bulge or disappearance of the capsule and extracapsular lesions, etc. (18). However, there is no definitive quantitative diagnosis of ECE, and PI-RADS v2.1 score alone has limited accuracy for assessing ECE. Recently, some studies have applied staging assessment for ECE (32,33). The Mehralivand grade is an objective and quantitative grading standard approach for the diagnosis of ECE proposed by Mehralivand et al. for the first time (19). In this study, it had verified that there was a fair result in the diagnostic efficacy of the Mehralivand grade in the validation set. The AUC was 0.774, similar to that in Mehralivand et al.’s research (AUC =0.77). However, the sensitivity of the Mehralivand grade was slightly low (70.4%) in this study, which could lead to some missed diagnoses of ECE, resulting in untimely treatment.
The application of radiomics has shown good performance in the diagnosis of ECE. Ma et al. (34) constructed a LASSO regression model based on T2WI to predict ECE in PCa, and 17 radiomics features were finally selected. The AUC of the radiomics model was 0.883 in the validation set, with high sensitivity and specificity, which was better than the performance of the Likert score results in radiologists (AUC =0.600–0.697). In another study by Ma et al. (35), a radiomics model was also built based on T2WI to predict the occurrence of ECE in the unilateral prostate lobe. The results showed that the AUCs in the validation set was 0.821, further verifying the potential predictive value of radiomics in ECE. T2WI is a routine prostate MRI sequence, which can partly reflect the lesion’s histopathological characteristics (36). Meanwhile, DWI and ADC are also commonly used functional sequences for prostate, which can provide more information to quantify tumor heterogeneity for the training of radiomics model, and texture features may be more helpful than grayscale-based statistical or morphological features in the diagnosis of ECE (37). Therefore, this study extracted features from T2WI, DWI, and ADC.
Different classifiers can produce differences in the results of radiomics model. This study showed that the LASSO analysis had the highest AUC among other classifiers based on the validation set, which was similar to the results of Ma et al. (35,36). According to the research, LASSO algorithm can ensure that all important radiomics features can not only be recognized effectively, but also avoid the overfitting problem of classification task (38). So that, it can get better results. In addition, RF, DT and other classifiers have good performance for nonlinear problems. But in the execution of multicollinearity tasks, they may have some problems such as difficulties in processing missing data, over-fitting and ignoring the correlation among each feature attribute, leading to unsatisfactory output results (39). For example, in this study, the AUCs of RF and DT classifiers in the training set were very high, reaching 1.000, but in the validation set, the AUCs were low, only 0.619–0.804, which may be due to the phenomenon of overfitting. Then, the final selected radiomics model incorporated nine features from ADC, six from DWI, and five features from T2WI. Among this selected radiomics features, GLSZM features accounted for the largest proportion, which quantifies gray level zones in an image. A gray level zone is defined as the number of connected voxels that share the same gray level intensity, which reflects the heterogeneity on the medical image (40). The AUC of radiomics model in the validation set was 0.835, which further verified the predictive value of radiomics on ECE by a quantitative and objective method.
Moreover, a combined model was constructed by the PSA, biopsy GG score, lesion location distribution, PI-RADS v2.1 score, and the predictive value in radiomics model in this study. Based on the validation set, the AUC reached 0.894, which showed higher sensitivity and accuracy than the radiomics model, but the AUC value between them was still comparable (P>0.05, 0.894 vs. 0.835). Xu et al. (41) built a combined nomogram based on multiparametric MRI by integrating a radiomics model and a clinical model (considering PSA and biopsy Gleason score) for preoperative prediction of ECE in PCa patients. Their results showed satisfactory diagnostic performance in ECE, with the AUCs of the radiomics model and combined nomogram being 0.865 and 0.857 for the validation set, respectively. The AUC was slightly higher in the radiomics model than in the combined nomogram, but the prediction efficiency was roughly consistent in these two models. The results in this study are slightly different from those of Xu et al. (41) This may be related to the different features extracted from different sequences and the differences between the clinical variables included in the combined model. So far, few studies have reported on the complementarity of clinical variables and radiomics in the prediction of ECE in PCa, so further research is also needed to verify these results.
This study also has some limitations. First, it is a retrospective single-center study without an independent external validation set to evaluate the model’s generalization ability. Second, all ROIs used in this study were outlined manually, and the results largely depended on the experience of radiologists. Semi-automatic or automatic segmentation of ROI should be developed and adopted in the future. Third, this study only focused on the existence of ECE in PCa, and the value of ECE in the prediction of biochemical recurrence or prognosis of PCa was not explored. It is expected that further research will be carried out in a later study.
Conclusions
The proposed combined model based on MRI radiomics and clinical variables has prospected the predictive value for ECE in PCa patients. It can obtain the maximum net clinical benefits compared to the clinical model, Mehralivand grade of radiologists, and radiomics model, which could be conducive to the preoperative individualized and accurate diagnosis and treatment of patients.
Acknowledgments
Funding: This work was supported by
Footnote
Reporting Checklist: The authors have completed the TRIPOD reporting checklist. Available at https://tcr.amegroups.com/article/view/10.21037/tcr-22-2750/rc
Data Sharing Statement: Available at https://tcr.amegroups.com/article/view/10.21037/tcr-22-2750/dss
Peer Review File: Available at https://tcr.amegroups.com/article/view/10.21037/tcr-22-2750/prf
Conflicts of Interest: All authors have completed the ICMJE uniform disclosure form (available at https://tcr.amegroups.com/article/view/10.21037/tcr-22-2750/coif). The authors have no conflicts of interest to declare.
Ethical Statement: The authors are accountable for all aspects of the work in ensuring that questions related to the accuracy or integrity of any part of the work are appropriately investigated and resolved. The study was conducted in accordance with the Declaration of Helsinki (as revised in 2013). The study was approved by the Ethics Committee of The First Affiliated Hospital of Soochow University (No. 262; 2021) and individual consent for this retrospective analysis was waived.
Open Access Statement: This is an Open Access article distributed in accordance with the Creative Commons Attribution-NonCommercial-NoDerivs 4.0 International License (CC BY-NC-ND 4.0), which permits the non-commercial replication and distribution of the article with the strict proviso that no changes or edits are made and the original work is properly cited (including links to both the formal publication through the relevant DOI and the license). See: https://creativecommons.org/licenses/by-nc-nd/4.0/.
References
- Siegel RL, Miller KD, Fuchs HE, et al. Cancer Statistics, 2021. CA Cancer J Clin 2021;71:7-33. [Crossref] [PubMed]
- Mungovan SF, Carlsson SV, Gass GC, et al. Preoperative exercise interventions to optimize continence outcomes following radical prostatectomy. Nat Rev Urol 2021;18:259-81. [Crossref] [PubMed]
- Mottet N, van den Bergh RCN, Briers E, et al. EAU-EANM-ESTRO-ESUR-SIOG Guidelines on Prostate Cancer-2020 Update. Part 1: Screening, Diagnosis, and Local Treatment with Curative Intent. Eur Urol 2021;79:243-62. [Crossref] [PubMed]
- Bill-Axelson A, Holmberg L, Garmo H, et al. Radical Prostatectomy or Watchful Waiting in Prostate Cancer - 29-Year Follow-up. N Engl J Med 2018;379:2319-29. [Crossref] [PubMed]
- Morlacco A, Sharma V, Viers BR, et al. The Incremental Role of Magnetic Resonance Imaging for Prostate Cancer Staging before Radical Prostatectomy. Eur Urol 2017;71:701-4. [Crossref] [PubMed]
- de Rooij M, Hamoen EH, Witjes JA, et al. Accuracy of Magnetic Resonance Imaging for Local Staging of Prostate Cancer: A Diagnostic Meta-analysis. Eur Urol 2016;70:233-45. [Crossref] [PubMed]
- Asfuroğlu U, Asfuroğlu BB, Özer H, et al. Which one is better for predicting extraprostatic extension on multiparametric MRI: ESUR score, Likert scale, tumor contact length, or EPE grade? Eur J Radiol 2022;149:110228. [Crossref] [PubMed]
- Xiang JY, Huang XS, Xu JX, et al. MRI Extraprostatic Extension Grade: Accuracy and Clinical Incremental Value in the Assessment of Extraprostatic Cancer. Biomed Res Int 2022;2022:3203965. [Crossref] [PubMed]
- Cutaia G, La Tona G, Comelli A, et al. Radiomics and Prostate MRI: Current Role and Future Applications. J Imaging 2021;7:34. [Crossref] [PubMed]
- Gillies RJ, Kinahan PE, Hricak H. Radiomics: Images Are More than Pictures, They Are Data. Radiology 2016;278:563-77. [Crossref] [PubMed]
- Chaddad A, Kucharczyk MJ, Cheddad A, et al. Magnetic Resonance Imaging Based Radiomic Models of Prostate Cancer: A Narrative Review. Cancers (Basel) 2021;13:552. [Crossref] [PubMed]
- Damascelli A, Gallivanone F, Cristel G, et al. Advanced Imaging Analysis in Prostate MRI: Building a Radiomic Signature to Predict Tumor Aggressiveness. Diagnostics (Basel) 2021;11:594. [Crossref] [PubMed]
- Qi Y, Zhang S, Wei J, et al. Multiparametric MRI-Based Radiomics for Prostate Cancer Screening With PSA in 4-10 ng/mL to Reduce Unnecessary Biopsies. J Magn Reson Imaging 2020;51:1890-9. [Crossref] [PubMed]
- Chen T, Li M, Gu Y, et al. Prostate Cancer Differentiation and Aggressiveness: Assessment With a Radiomic-Based Model vs. PI-RADS v2. J Magn Reson Imaging 2019;49:875-84. [Crossref] [PubMed]
- Moazemi S, Erle A, Khurshid Z, et al. Decision-support for treatment with (177)Lu-PSMA: machine learning predicts response with high accuracy based on PSMA-PET/CT and clinical parameters. Ann Transl Med 2021;9:818. [Crossref] [PubMed]
- Moazemi S, Essler M, Schultz T, et al. Predicting Treatment Response in Prostate Cancer Patients Based on Multimodal PET/CT for Clinical Decision Support. In: Syeda-Mahmood T, Li X, Madabhushi A, et al. Multimodal Learning for Clinical Decision Support. ML-CDS 2021. Lecture Notes in Computer Science (LNCS, volume 13050). Springer, Cham; 2021:22-35.
- Magi-Galluzzi C, Evans AJ, Delahunt B, et al. International Society of Urological Pathology (ISUP) Consensus Conference on Handling and Staging of Radical Prostatectomy Specimens. Working group 3: extraprostatic extension, lymphovascular invasion and locally advanced disease. Mod Pathol 2011;24:26-38. [Crossref] [PubMed]
- Turkbey B, Rosenkrantz AB, Haider MA, et al. Prostate Imaging Reporting and Data System Version 2.1: 2019 Update of Prostate Imaging Reporting and Data System Version 2. Eur Urol 2019;76:340-51. [Crossref] [PubMed]
- Mehralivand S, Shih JH, Harmon S, et al. A Grading System for the Assessment of Risk of Extraprostatic Extension of Prostate Cancer at Multiparametric MRI. Radiology 2019;290:709-19. [Crossref] [PubMed]
- van Griethuysen JJM, Fedorov A, Parmar C, et al. Computational Radiomics System to Decode the Radiographic Phenotype. Cancer Res 2017;77:e104-7. [Crossref] [PubMed]
- Song Y, Zhang J, Zhang YD, et al. FeAture Explorer (FAE): A tool for developing and comparing radiomics models. PLoS One 2020;15:e0237587. [Crossref] [PubMed]
- Guerra A, Negrão E, Papanikolaou N, et al. Machine learning in predicting extracapsular extension (ECE) of prostate cancer with MRI: a protocol for a systematic literature review. BMJ Open 2022;12:e052342. [Crossref] [PubMed]
- Vis AN, van den Bergh RCN, van der Poel HG, et al. Selection of patients for nerve sparing surgery in robot-assisted radical prostatectomy. BJUI Compass 2021;3:6-18. [Crossref] [PubMed]
- Gaunay GS, Patel V, Shah P, et al. Multi-parametric MRI of the prostate: Factors predicting extracapsular extension at the time of radical prostatectomy. Asian J Urol 2017;4:31-6. [Crossref] [PubMed]
- Eifler JB, Feng Z, Lin BM, et al. An updated prostate cancer staging nomogram (Partin tables) based on cases from 2006 to 2011. BJU Int 2013;111:22-9. [Crossref] [PubMed]
- Cooperberg MR, Pasta DJ, Elkin EP, et al. The University of California, San Francisco Cancer of the Prostate Risk Assessment score: a straightforward and reliable preoperative predictor of disease recurrence after radical prostatectomy. J Urol 2005;173:1938-42. [Crossref] [PubMed]
- Turo R, Forster JA, West RM, et al. Do prostate cancer nomograms give accurate information when applied to European patients? Scand J Urol 2015;49:16-24. [Crossref] [PubMed]
- Zhang JH, Xu LL, Zhang GM, et al. Research Progress of magnetic resonance imaging-based radiomics in prostate cancer. Acta Academiae Medicinae Sinicae 2022;44:123-9. [PubMed]
- Krishna S, Lim CS, McInnes MDF, et al. Evaluation of MRI for diagnosis of extraprostatic extension in prostate cancer. J Magn Reson Imaging 2018;47:176-85. [Crossref] [PubMed]
- Matsuoka Y, Ishioka J, Tanaka H, et al. Impact of the Prostate Imaging Reporting and Data System, Version 2, on MRI Diagnosis for Extracapsular Extension of Prostate Cancer. AJR Am J Roentgenol 2017;209:W76-84. [Crossref] [PubMed]
- Tay KJ, Gupta RT, Brown AF, et al. Defining the Incremental Utility of Prostate Multiparametric Magnetic Resonance Imaging at Standard and Specialized Read in Predicting Extracapsular Extension of Prostate Cancer. Eur Urol 2016;70:211-3. [Crossref] [PubMed]
- Asfuroğlu U, Asfuroğlu BB, Özer H, et al. Which one is better for predicting extraprostatic extension on multiparametric MRI: ESUR score, Likert scale, tumor contact length, or EPE grade? Eur J Radiol 2022;149:110228. [Crossref] [PubMed]
- Li W, Shang W, Lu F, et al. Diagnostic Performance of Extraprostatic Extension Grading System for Detection of Extraprostatic Extension in Prostate Cancer: A Diagnostic Systematic Review and Meta-Analysis. Front Oncol 2022;11:792120. [Crossref] [PubMed]
- Ma S, Xie H, Wang H, et al. MRI-Based Radiomics Signature for the Preoperative Prediction of Extracapsular Extension of Prostate Cancer. J Magn Reson Imaging 2019;50:1914-25. [Crossref] [PubMed]
- Ma S, Xie H, Wang H, et al. Preoperative Prediction of Extracapsular Extension: Radiomics Signature Based on Magnetic Resonance Imaging to Stage Prostate Cancer. Mol Imaging Biol 2020;22:711-21. [Crossref] [PubMed]
- Hectors SJ, Chen C, Chen J, et al. Magnetic Resonance Imaging Radiomics-Based Machine Learning Prediction of Clinically Significant Prostate Cancer in Equivocal PI-RADS 3 Lesions. J Magn Reson Imaging 2021;54:1466-73. [Crossref] [PubMed]
- Zheng H, Miao Q, Liu Y, et al. Multiparametric MRI-based radiomics model to predict pelvic lymph node invasion for patients with prostate cancer. Eur Radiol 2022;32:5688-99. [Crossref] [PubMed]
- Friedman J, Hastie T, Tibshirani R. Regularization Paths for Generalized Linear Models via Coordinate Descent. J Stat Softw 2010;33:1-22. [Crossref] [PubMed]
- Rosenbusch H, Soldner F, Evans AM, et al. Supervised machine learning methods in psychology: A practical introduction with annotated R code. Soc Personal Psychol Compass 2021;15:e12579. [Crossref]
- Thibault G, Fertil B, Navarro C, et al. Texture indexes and gray level size zone matrix application to cell nuclei classification. 10th International Conference on Pattern Recognition and Information Processing (PRIP 2009); Minsk, Belarus; 19-21 May 2009. Springer; 2009:140-5.
- Xu L, Zhang G, Zhao L, et al. Radiomics Based on Multiparametric Magnetic Resonance Imaging to Predict Extraprostatic Extension of Prostate Cancer. Front Oncol 2020;10:940. [Crossref] [PubMed]