Identification of KNOP1 as a prognostic marker in hepatocellular carcinoma
Highlight box
Key findings
• KNOP1 overexpression was associated with poor HCC prognosis and increased proportions of immune cell infiltration and checkpoints.
What is known and what is new?
• KNOP1 is a nucleolar protein that interacts with zinc finger 106 protein, which was first reported in 1999. It is predominantly expressed in proliferating somatic cells and in male germ cells, and may therefore be involved in development of the testes.
• In this study, the results show that KNOP1 is significantly overexpressed in a variety of tumors, including HCC. KNOP1 is also associated with pathological stage, histologic grade, tumor status, lower methylation levels, and immune infiltration. High KNOP1 expression is associated with less favorable overall survival, progression-free interval, and disease-specific survival, which indicates that high KNOP1 expression may be a significant indicator of poor HCC prognosis.
What is the implication, and what should change now?
• KNOP1 is a potential biomarker for evaluating HCC prognosis.
Introduction
Hepatocellular carcinoma (HCC) is the sixth most common type of primary malignancy worldwide, and the fourth leading cause of cancer-related death (1). Current treatment options for liver cancer include surgical resection, interventional therapy, liver transplantation, chemotherapy, and targeted therapy. However, the prognosis of HCC remains poor due to the aggressive, metastatic, and immune escape characteristics (2). Therefore, there is an urgent need to explore the mechanisms involved in HCC progression and to identify new therapeutic targets that can improve early diagnosis, prognostic assessment, and treatment of HCC.
Lysine-rich nucleolar protein 1 (KNOP1) is a nucleolar protein that interacts with zinc finger 106 protein. Also known as Tsg118, it was first reported in 1999 (3). KNOP1 is predominantly expressed in proliferating somatic cells and in male germ cells, and may therefore be involved in testicular development (4). However, there have been few investigations of KNOP1 in HCC. This study analyzed the relationship between KNOP1 expression and clinical prognosis of HCC with an objective to identify new HCC biomarkers and therapeutic target genes and to generate new ideas for HCC diagnosis and treatment. We present this article in accordance with the Transparent Reporting of a multivariable prediction model for Individual Prognosis or Diagnosis (TRIPOD) reporting checklist (available at https://tcr.amegroups.com/article/view/10.21037/tcr-23-4/rc).
Methods
RNA sequencing (RNA-Seq) data and bioinformatics analysis
RNA-Seq data in transcripts per million (TPM) format from The Cancer Genome Atlas (TCGA) and genotype-tissue expression (GTEx) databases that were uniformly processed using the Toil process (5) were downloaded from UCSC Xena (https://xenabrowser.net/datapages/). RNA-Seq data from the GTEx database (6) and TCGA were integrated to improve the reliability of the results as the sample size of normal tissues in TCGA was small. To further investigate the expression of KNOP1 in HCC, the expression of KNOP1 at the messenger RNA (mRNA) and protein levels was verified using data from 2 Gene Expression Omnibus (GEO; https://www.ncbi.nlm.nih.gov/geo/) datasets, namely, GSE55092 (7) and GSE69715 (8), and the Human Protein Atlas (HPA). This study complied with the guidelines issued by TCGA, GEO, and GTEx. Unavailable or unknown clinical information for individual patients included in the study were characterized as missing. The study was conducted in accordance with the Declaration of Helsinki (as revised in 2013).
Analysis of differentially expressed genes (DEGs)
The samples were divided into high and low KNOP1 expression groups based on the median KNOP1 expression level. DEGs were identified using the R package DESeq2. The threshold for the DEGs was set at |log2 fold change (FC)| >1.5 and adjusted P<0.05 (9).
Gene Ontology (GO) and Kyoto Encyclopedia of Genes and Genomes (KEGG) analysis
DEGs were used for functional enrichment analysis. GO was used to annotate cell composition, including cellular component (CC), molecular function (MF), and biological process (BP). The clusterProfiler package (10) was used for GO and KEGG enrichment analysis of DEGs.
Gene set enrichment analysis (GSEA) and analysis of co-expression of cell cycle proteins
KNOP1 expression levels were compared using the clusterProfiler package with GSEA to clarify functional and pathway differences. The C2.all.v7.0.symbols.gmt collection in the Molecular Signatures Database (MSigDB) (11) was used as the reference gene collection. Significant enrichment was indicated when P<0.05, false discovery rate (FDR) <0.25, and |normalized enrichment score (NES)| >1. Spearman’s analysis was used to analyze the correlation between KNOP1 expression and cell cycle proteins.
Establishment of protein-protein and gene-gene interaction (GGI) networks
The Search Tool for the Retrieval of Interacting Genes (STRING) online software (version 11.5) (http://string-db.org) was used to predict the protein-protein interaction (PPI) network. Interaction scores were determined by a combined threshold of 0.4 (12). The GGI network was generated using GeneMANIA (http://genemania.org/).
Genetic alterations analysis of HCC patients
Based on 3 datasets (namely, HCC patients from TCGA, Firehose Legacy; AMC Hepatology 2014 and RIKEN, Nat genet 2012), cBioPortal was used to analyze the genomic profiles of KNOP1. The differences between the altered and unaltered KNOP1 groups were assessed using Kaplan-Meier (KM) plots, and a log-rank test. All P values less than 0.05 were considered statistically significant.
Analysis of KNOP1 gene methylation
The DNA Methylation Interactive Visualization Database (DNMIVD) (13) (http://119.3.41.228/dnmivd/index/) was used to analyze DNA methylation levels of KNOP1 in HCC and normal tissues from TCGA database. An analysis of promoter methylation levels and KNOP1 expression was performed using Spearman’s correlation. For assessing the relevance of low and high KNOP1 methylation levels, KM plots were generated and log-rank tests were conducted.
Assessment of immune cell infiltration and immune checkpoints
Immune infiltration analysis was carried out using the single-sample gene set enrichment analysis (ssGSEA) algorithm implemented in the GSVA package (14). A total of 24 infiltrating immune cell types (15) were obtained. A relative enrichment score was calculated for each tumor sample based on its gene expression profile. RNA-Seq expression (level 3) profiles and corresponding clinical information for HCC were downloaded from TCGA database. A total of eight immune checkpoint genes were selected for analysis, including SIGLEC15, TIGIT, CD274, HAVCR2, PDCD1, CTLA4, LAG3, and PDCD1LG2. Each of these transcripts was analyzed to assess its expression value (16-18). Analysis of KNOP1’s correlation with the 24 types of immune cells and checkpoints was performed using Spearman’s correlation. A Wilcoxon rank sum test was used to analyze the differences in immune cell infiltration between groups with high and low levels of KNOP1 expression. The R package ggplot2 (version 3.3.3) was used for data visualization.
Clinical analysis of prognostic state
The patients were divided into high and low expression groups based on the median KNOP1 expression. To explore the prognostic value of KNOP1, the relationship between KNOP1 expression and clinical pathologic factors was analyzed using univariate and multivariate Cox regression analyses implemented in the R package survival (version 3.2.10). KM plots were generated and log-rank test was performed to identify differences in overall survival (OS), disease-specific survival (DSS), and progression-free interval (PFI) between the low and high KNOP1 expression groups. The independent prognostic factors from the multivariate Cox regression analysis were used to construct nomograms and evaluated the 1-, 3-, and 5-year prognostic outcomes. Nomograms were created in R using the rms package, which included calibration plots and significant clinical factors. In order to analyze the calibration curves, the nomogram evaluating probability against actual occurrence was compared to the 45-degree line, which indicates the most accurate prediction. A concordance index (C-index) was analyzed using 1,000 bootstrap resampling steps and the results applied to estimate the discrimination ability of the model. The C-index was used to assess prognostic factors and predictive accuracy of the nomogram. There was a two-tailed statistical test with a significance level of P<0.05. The rsm R package (version 6.2.0) was used to generate nomograms and the survminer package (version 0.4.9) was used for visualization.
Statistical analysis
The data were visualized and analyzed using R statistical software (version 3.6.3) (19). Wilcoxon rank sum test was used to evaluate the relationship between KNOP1 expression and clinical characteristics. KM survival analysis and Cox regression were used to evaluate prognostic factors. Receiver operating characteristic (ROC) curves were generated using the pROC package and used to evaluate the effectiveness of KNOP1 in distinguishing HCC from healthy samples. The significance of all statistical tests was determined when the two-tailed P<0.05.
Results
KNOP1 gene is highly expressed in HCC
Most tumor types in the databases of TCGA and GTEx were found to have overexpressed KNOP1 mRNA compared with normal tissue (Figure 1A). Among unpaired specimens, KNOP1 expression was higher in HCC tumor tissues compared with normal tissues from TCGA database (Figure 1B) and the GEO database (GSE55029 dataset) (Figure 1C). Among paired specimens, the expression of KNOP1 mRNA in HCC tissues was significantly higher than in adjacent normal tissues (Figure 1D) and the GEO database (GSE69715 dataset) (Figure 1E).
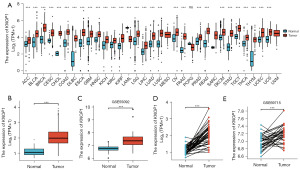
Correlation between KNOP1 expression and clinical pathological features
Wilcoxon rank sum test was used to compare the difference between KNOP1 expression in patients with different clinicopathological features. The results showed that KNOP1 was associated with tumor-node-metastasis (TNM) stage (Figure 2A-2C), pathological stage (Figure 2D), histologic grade (Figure 2E), tumor status (Figure 2F), Child-Pugh grade (Figure 2G), and vascular invasion (Figure 2H). The OS of patients with HCC was also significantly lower than that of healthy controls (Figure 2I). The HPA database was used to compare the levels of KNOP1 protein. KNOP1 protein levels in HCC tissues were higher than in normal tissues (Figure 2J,2K). The ability of KNOP1 to distinguish HCC patients from healthy individuals was analyzed using ROC curves. The area under the curve (AUC) was 0.949, indicating that KNOP1 is a potential biomarker (Figure 2L).
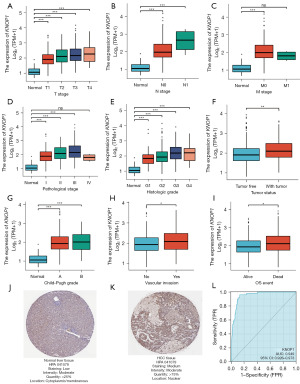
DEGs in HCC specimens with low and high KNOP1 gene expression
The R package DESeq2 was used to analyze HTSeq-count data from TCGA. This study identified 1,102 DEGs (943 upregulated and 159 downregulated) based on the set threshold (|logFC| >1.5 and adjusted P<0.05). DEGs were visualized using a volcano plot (Figure 3A) and the top 10 DEGs were represented on a heatmap (Figure 3B).
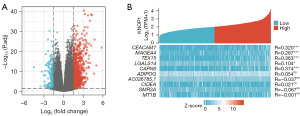
GO and KEGG pathway enrichment analysis of DEGs
GO and KEGG pathway functional enrichment analyses were performed using the clusterProfiler package to better understand the functional significance of the 1,102 genes that were differentially expressed between HCC samples with high and low KNOP1 expression (Figure 4).
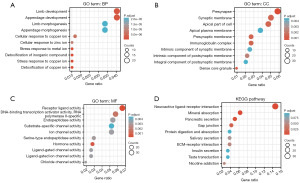
GSEA of KNOP1 gene
GSEA between low and high KNOP1 expression datasets was conducted to identify key signaling pathways associated with KNOP1. High KNOP1 expression was associated with DNA replication, cell cycle, and nuclear factor κB (NF-κB) and T cell receptor signaling pathways (Figure 5). Since the GSEA revealed that KNOP1 expression was mainly enriched in cell cycle-related pathways, this study analyzed the correlation between KNOP1 and cell cycle genes. A Venn diagram of the genes in the KEGG_CELL_CYCLE pathway and the KEGG_DNA_REPLICATION pathway was created and analyzed the correlation of the 7 genes (MCM2, MCM3, MCM4, MCM5, MCM6, MCM7, and PCNA) in the intersection with KNOP1 (Figure 6A). According to the results, there was a significant and positive correlation between KNOP1 and all 7 genes (Figure 6B).
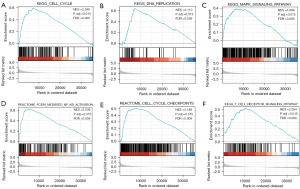
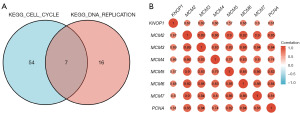
PPI and GGI network analyses
A PPI network of the top 25 proteins that were correlated with KNOP1 was generated using the STRING database. The network had 26 nodes (including KNOP1) and 97 edges (Figure 7A). A GGI network was generated using GeneMANIA, and the resulting network had 20 genes and 73 links (Figure 7B).
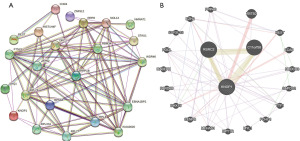
Genetic alterations in patients with HCC
The genetic alterations were analyzed using the cBioPortal database. The results showed that the percentage of genetic alterations in KNOP1 in patients with HCC was 0.8% (Figure 8A), and the difference in disease-free survival between altered and unaltered KNOP1 groups was statistically significant (P=0.0116) (Figure 8B).
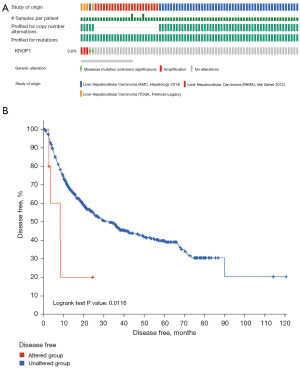
Methylation of KNOP1 gene in HCC patients
KNOP1 DNA methylation levels and the correlation between the prognostic value and promoter methylation levels were investigated using the DNMIVD database. The results showed that KNOP1 promoter methylation levels in the tumor group were lower than those in the normal group (P<0.001) (Figure 9A). The methylation level of the KNOP1 promoter was negatively correlated with KNOP1 expression (Figure 9B). However, the differences in OS and PFI between patients with low and high KNOP1 methylation levels were not statistically significant (Figure 9C,9D).
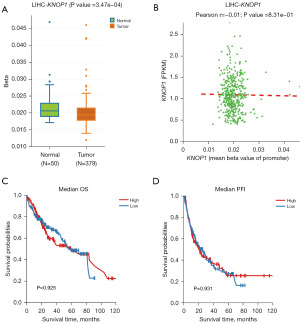
Infiltration of immune cells in HCC
Based on Spearman’s correlation analysis, KNOP1 expression levels in the HCC microenvironment were associated with immune cell infiltration as measured by ssGSEA. Forest plots showed that KNOP1 was positively correlated with 11 immune cell subsets, negatively correlated with 12 immune cell subsets, and not correlated with 1 immune cell subset. KNOP1 was significantly positively correlated with T helper 2 (Th2) cells (Figure 10A). The infiltration levels of Th2 cells were higher in samples in the high KNOP1 expression group compared with samples in the low KNOP1 expression group (P<0.001) (Figure 10B). Explicit KNOP1 expression was positively related to Th2 cell infiltration (r=0.431, P<0.001) (Figure 10C).
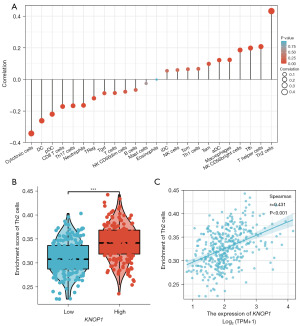
Analysis of immune checkpoints
This study further analyzed the expression level of 8 immune checkpoints: cluster of differentiation 274 (CD274), cytotoxic T-lymphocyte protein 4 (CTLA4), hepatitis A virus cellular receptor 2 (HAVCR2), lymphocyte-activation gene 3 (LAG3), programmed cell death protein 1 (PDCD1), prognostic relevance of programmed cell death 1 ligand 2 (PDCD1LG2), T cell immunoreceptor with immunoglobulin and ITIM domain (TIGIT), and sialic acid-binding immunoglobulin-like lectin 15 (SIGLEC15) in HCC and determined the correlation between them. The results showed that the expression levels of CTLA4, HAVCR2, PDCD1, PDCD1LG2, TIGIT, and SIGLEC15 were higher in HCC tissues than in normal tissues, whereas the expression levels of CD274 and LAG3 were higher in normal tissues than in HCC tissues (Figure 11A). Figure 11B showed the correlation between KNOP1 and the 8 immune checkpoints in HCC.
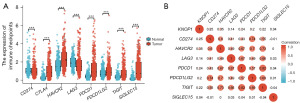
High KNOP1 gene expression is associated with adverse outcomes in HCC
Data from 250 male and 121 female patients (median age 59 years old) were included in this study. HCC samples were classified into low or high KNOP1 mRNA expression groups based on the median expression value (2.608 for TPM). A detailed description of the clinicopathological features can be found in Table 1.
Table 1
Characteristics | Low expression of KNOP1 (n=185) | High expression of KNOP1 (n=186) | P value |
---|---|---|---|
Gender, n (%) | 0.395 | ||
Female | 56 (15.1) | 65 (17.5) | |
Male | 129 (34.8) | 121 (32.6) | |
Age (years), n (%) | 0.677 | ||
≤60 | 86 (23.2) | 91 (24.6) | |
>60 | 99 (26.8) | 94 (25.4) | |
AFP (ng/mL), n (%) | 0.067 | ||
≤400 | 115 (41.4) | 98 (35.3) | |
>400 | 26 (9.4) | 39 (14.0) | |
T stage, n (%) | 0.011 | ||
T1 | 105 (28.5) | 76 (20.7) | |
T2 | 40 (10.9) | 54 (14.7) | |
T3 | 33 (9.0) | 47 (12.8) | |
T4 | 4 (1.1) | 9 (2.4) | |
N stage, n (%) | 0.622 | ||
N0 | 127 (49.6) | 125 (48.8) | |
N1 | 1 (0.4) | 3 (1.2) | |
M stage, n (%) | 1.000 | ||
M0 | 135 (50.0) | 131 (48.5) | |
M1 | 2 (0.7) | 2 (0.7) | |
Pathologic stage, n (%) | 0.011 | ||
Stage I | 100 (28.8) | 71 (20.5) | |
Stage II | 38 (11.0) | 48 (13.8) | |
Stage III | 33 (9.5) | 52 (15.0) | |
Stage IV | 3 (0.9) | 2 (0.6) | |
Histologic grade, n (%) | 0.001 | ||
G1 | 35 (9.6) | 20 (5.5) | |
G2 | 98 (26.8) | 79 (21.6) | |
G3 | 45 (12.3) | 77 (21.0) | |
G4 | 4 (1.1) | 8 (2.2) | |
Child-Pugh grade, n (%) | 0.813 | ||
A | 117 (49.0) | 100 (41.8) | |
B | 10 (4.2) | 11 (4.6) | |
C | 1 (0.4) | 0 (0) | |
Fibrosis Ishak score, n (%) | 0.994 | ||
0 | 39 (18.4) | 35 (16.5) | |
1/2 | 17 (8.0) | 14 (6.6) | |
3/4 | 15 (7.1) | 13 (6.1) | |
5/6 | 41 (19.3) | 38 (17.9) | |
Vascular invasion, n (%) | 0.088 | ||
No | 113 (35.9) | 93 (29.5) | |
Yes | 48 (15.2) | 61 (19.4) | |
Residual tumor, n (%) | <0.001 | ||
R0 | 172 (50.3) | 152 (44.4) | |
R1 | 2 (0.6) | 15 (4.4) | |
R2 | 1 (0.3) | 0 (0) |
HCC, hepatocellular carcinoma; KNOP1, lysine-rich nucleolar protein 1; TCGA, The Cancer Genome Atlas; AFP, alpha fetoprotein.
KM survival analysis showed that patients with high KNOP1 expression in TCGA dataset had less favorable OS [hazard ratio (HR) =1.86, 95% confidence interval (CI): 1.31–2.64, P=0.001; Figure 12A), DSS (HR =2.63, 95% CI: 1.65–4.20, P<0.001; Figure 12B], and PFI (HR =1.69, 95% CI: 1.26–2.27, P<0.001; Figure 12C) compared with patients with low KNOP1 expression. Univariate and multifactorial Cox regression analyses were used to assess the prognostic factors affecting HCC patients. The results of the univariate Cox regression analysis showed that T3 stage (P<0.001), T4 stage (P<0.001), pathological M1 stage (P=0.018), and high KNOP1 expression (P<0.001) were predictive of poor HCC prognosis. Inclusion of T stage, M stage, and KNOP1 in a multivariate Cox regression showed that T3 stage (P<0.001), T4 stage (P=0.006), and high KNOP1 expression (P=0.005) were independent predictors of poor HCC prognosis (Table 2).
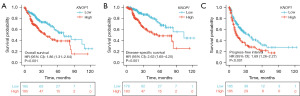
Table 2
Characteristics | Total (n) | Univariate analysis | Multivariate analysis | |||
---|---|---|---|---|---|---|
Hazard ratio (95% CI) | P value | Hazard ratio (95% CI) | P value | |||
Gender | 370 | |||||
Female | 121 | Reference | – | – | – | |
Male | 249 | 0.816 (0.573–1.163) | 0.260 | – | – | |
Age (years) | 370 | |||||
≤60 | 177 | Reference | – | – | – | |
>60 | 193 | 1.248 (0.880–1.768) | 0.214 | – | – | |
T stage | 367 | |||||
T1 | 181 | Reference | – | Reference | – | |
T2 | 93 | 1.436 (0.906–2.276) | 0.124 | 1.305 (0.718–2.372) | 0.382 | |
T3 | 80 | 2.615 (1.723–3.970) | <0.001 | 2.737 (1.635–4.584) | <0.001 | |
T4 | 13 | 5.294 (2.644–10.599) | <0.001 | 4.285 (1.522–12.064) | 0.006 | |
N stage | 256 | |||||
N0 | 252 | Reference | – | – | – | |
N1 | 4 | 2.004 (0.491–8.181) | 0.333 | – | – | |
M stage | 270 | |||||
M0 | 266 | Reference | – | Reference | – | |
M1 | 4 | 4.032 (1.267–12.831) | 0.018 | 1.442 (0.341–6.100) | 0.619 | |
Histologic grade | 365 | |||||
G1 | 55 | Reference | – | – | – | |
G2 | 177 | 1.179 (0.696–1.997) | 0.541 | – | – | |
G3 | 121 | 1.232 (0.710–2.139) | 0.457 | – | – | |
G4 | 12 | 1.692 (0.625–4.580) | 0.301 | – | – | |
AFP (ng/mL) | 277 | |||||
≤400 | 213 | Reference | – | – | – | |
>400 | 64 | 1.056 (0.646–1.727) | 0.827 | – | – | |
Child-Pugh grade | 238 | |||||
A & B | 237 | Reference | – | – | – | |
C | 1 | 2.012 (0.277–14.606) | 0.489 | – | – | |
Fibrosis Ishak score | 211 | |||||
0 | 74 | Reference | – | – | – | |
1/2 | 31 | 0.916 (0.428–1.961) | 0.822 | – | – | |
3/4 | 28 | 0.681 (0.281–1.654) | 0.396 | – | – | |
5/6 | 78 | 0.761 (0.424–1.368) | 0.361 | – | – | |
Vascular invasion | 314 | |||||
No | 206 | Reference | – | – | – | |
Yes | 108 | 1.348 (0.890–2.042) | 0.159 | – | – | |
Residual tumor | 341 | |||||
R0 | 323 | Reference | – | – | – | |
R1 & R2 | 18 | 1.571 (0.795–3.104) | 0.194 | – | – | |
KNOP1 | 370 | |||||
Low | 185 | Reference | – | Reference | – | |
High | 185 | 1.856 (1.306–2.637) | <0.001 | 1.917 (1.222–3.010) | 0.005 |
HCC, hepatocellular carcinoma; TCGA, The Cancer Genome Atlas; CI, confidence interval; AFP, alpha fetoprotein; KNOP1, lysine-rich nucleolar protein 1.
To better predict HCC prognosis, the R package rms was used to construct a nomogram to predict the 1-, 3-, and 5-year OS rates of patients with HCC. The model included 5 prognostic variables: T stage, N stage, M stage, age, and KNOP1 expression. To determine the number of points on these variables, a straight line was drawn upward using a point scale, and a scale of 0 to 100 was applied to each variable’s sum of points. Finally, the score of each variable was recorded and the accumulated score taken as the total score. The 1-, 3-, and 5-year OS rates of patients with HCC were determined by drawing a line from the total point axis straight down to the outcome axis. The results showed that the 1-year OS rate was <30%, and the 3- and 5-year OS rates were <20% for advanced HCC patients. Meanwhile, the 1-, 3-, and 5-year OS for HCC patients in early stage reached up to around 60% (Figure 13A). According to the nomogram calibration curve, the patient observations matched results predicted by the nomogram (Figure 13B).
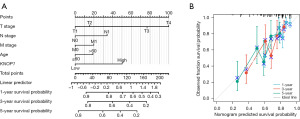
Discussion
HCC is one of the most common gastrointestinal tumors and is associated with poor prognosis. It is therefore crucial to identify new targets for HCC diagnosis and treatment. Utilizing the TCGA database, this study investigated KNOP1 overexpression in HCC tissues compared to normal liver tissues and showed that high KNOP1 expression was associated with high TNM stage, high pathologic and histologic stages, high Child-Pugh grade, and vascular invasion. In addition, univariate and multifactorial Cox regression analyses showed that high KNOP1 expression was a significant indicator of poor prognosis. The ability of KNOP1 expression to predict prognosis was further confirmed using a nomogram prediction model, and the calibration plots showed agreement between column line graph predictions associated with KNOP1 and actual observations of 1-, 3-, and 5-year OS probabilities, with individualized scores provided for individual patients. In conclusion, KNOP1 is a potential novel prognostic marker for HCC.
Instability in genomic structure and DNA methylation can lead to cancer development (20). In this study, the percentage of KNOP1 gene alterations in HCC was approximately 0.8%, but the DSS was higher in the unaltered group compared with the altered group. Analysis of methylation showed that KNOP1 methylation levels were lower in the tumor group compared with the normal group, but the differences in OS and PFI between patients in the hypermethylated and hypomethylated groups were not statistically significant.
We performed functional enrichment analysis using GO, KEGG, and GSEA to better explore KNOP1 function. GO analysis showed that high KNOP1 expression was associated with detoxification of copper ion, stress response to copper ion, detoxification of inorganic compounds, stress response to metal ion, and cellular response to copper and zinc ion. Several recent studies have shown that alterations in metals such as zinc and copper can modulate molecular targets such as cyclic adenosine-dependent protein kinase (PKA), protein kinase C (PKC), and transcription factors, including NF-κB, that play important roles in the progression and development of various cancers (21,22). Using GSEA, we showed that KNOP1 was associated with cell cycle, MAPK, and NF-κB related pathways. Several studies have shown that significant enhancement of cell cycle pathways in HCC cells can inhibit the progression of HCC by suppressing the cell cycle (23,24). Abnormal mitotic processes are a key cause of susceptibility and progression of cancers such as HCC. Mitotic errors in HCC progression activate Chk2, resulting in lagging chromosome and DNA damage (25). In addition, mitotic spindles enhance chromosomal instability and HCC progression (25). Microchromosome maintenance families (MCMs) play a central role in replication as replicative DNA helicases, and at least 10 homologs have been identified in humans. Among them, the MCM2–7 complex is involved in the formation of pre-replication complexes and exhibits helicase activity (26). MCM is a candidate marker of cell proliferation, and elevated levels of MCM indicate proliferation of malignant cells. Previous research has shown that mRNA levels of MCM2, MCM6, and MCM7 are correlated with certain tumor characteristics and with HCC prognosis (27). In this study, KNOP1 was significantly and positively correlated with MCM2, MCM3, MCM4, MCM5, MCM6, MCM7, and PCNA, indicating that high KNOP1 expression may be associated with the proliferation of HCC cells, hence providing a new diagnostic tool for HCC. The MAPK pathway is a ubiquitous signaling pathway involved in many life processes and is frequently altered in many diseases (28). The pathway regulates cellular activities during cancer development, including cell proliferation, apoptosis, and immune escape. Inhibition of the upstream kinases involved in the MAPK pathway is a therapeutic strategy for some cancers (29).
Tumor-infiltrating immune cells (TIICs) in the immune microenvironment have been associated with immune surveillance and immunotherapy in HCC (30). Th2 cells play an important proto-oncogenic role in tumorigenesis (31), and Lorvik et al. (32) suggested that pericyte therapy using tumor-specific Th2 cells may be a highly effective immunotherapeutic option for cancer. In addition, T follicular helper cells (Tfh) cells can be differentiated into Th1 and Th2 cells and regulate humoral immune responses (33). Th2 cells can in turn induce the polarization of M1 macrophages into immunosuppressive M2 macrophages (34), leading to the suppression of the host immune system and thus promoting tumorigenesis. In this study, tumor infiltration by Th2 cells, Tfh cells, and macrophages was positively correlated with KNOP1 expression, suggesting that KNOP1 may plays an important role in the regulation of immune infiltrating cells in HCC.
Immune checkpoint inhibitors (ICIs) have revolutionized cancer treatment and led to long term remission in patients with different cancer types (35). Immune checkpoints are involved in the suppression of T-cell or natural killer (NK) cell activation and in the initiation and maintenance of tumor immune tolerance. The best-known ICIs are CTLA4 and PDCD1, which inhibit T cell proliferation and the release of cytotoxic mediators (36,37). We found a positive correlation between KNOP1 expression and CTLA4, PDCD1, HAVCR2, PDCD1LG2, TIGIT, SIGLEC15, CD274, and LAG3, suggesting that targeting KNOP1 may improve the efficacy of ICIs in HCC.
Conclusions
KNOP1 was found to play a crucial role in regulating the cell cycle and immune response in HCC. However, this study was limited to in vitro investigations, and additional in vitro as well as in vivo studies are needed to verify the findings herein and to elucidate the specific mechanism of action of KNOP1 in HCC growth and metastasis in order to determine the clinical applications for patients with HCC.
Acknowledgments
We would like to thank https://www.xiantao.love/ for their help in data analysis.
Funding: This work was supported by
Footnote
Reporting Checklist: The authors have completed the TRIPOD reporting checklist. Available at https://tcr.amegroups.com/article/view/10.21037/tcr-23-4/rc
Peer Review File: Available at https://tcr.amegroups.com/article/view/10.21037/tcr-23-4/prf
Conflicts of Interest: All authors have completed the ICMJE uniform disclosure form (available at https://tcr.amegroups.com/article/view/10.21037/tcr-23-4/coif). The authors have no conflicts of interest to declare.
Ethical Statement: The authors are accountable for all aspects of the work in ensuring that questions related to the accuracy or integrity of any part of the work are appropriately investigated and resolved. The study was conducted in accordance with the Declaration of Helsinki (as revised in 2013).
Open Access Statement: This is an Open Access article distributed in accordance with the Creative Commons Attribution-NonCommercial-NoDerivs 4.0 International License (CC BY-NC-ND 4.0), which permits the non-commercial replication and distribution of the article with the strict proviso that no changes or edits are made and the original work is properly cited (including links to both the formal publication through the relevant DOI and the license). See: https://creativecommons.org/licenses/by-nc-nd/4.0/.
References
- Villanueva A. Hepatocellular Carcinoma. N Engl J Med 2019;380:1450-62. [Crossref] [PubMed]
- D'Avola D, Granito A, Torre-Aláez M, et al. The importance of liver functional reserve in the non-surgical treatment of hepatocellular carcinoma. J Hepatol 2022;76:1185-98. [Crossref] [PubMed]
- Larsson M, Brundell E, Jörgensen PM, et al. Characterization of a novel nucleolar protein that transiently associates with the condensed chromosomes in mitotic cells. Eur J Cell Biol 1999;78:382-90. [Crossref] [PubMed]
- Available online: https://www.genecards.org/cgi-bin/carddisp.pl?gene=KNOP1#pathways_interactions
- Vivian J, Rao AA, Nothaft FA, et al. Toil enables reproducible, open source, big biomedical data analyses. Nat Biotechnol 2017;35:314-6. [Crossref] [PubMed]
- Carithers LJ, Moore HM. The Genotype-Tissue Expression (GTEx) Project. Biopreserv Biobank 2015;13:307-8. [Crossref] [PubMed]
- Melis M, Diaz G, Kleiner DE, et al. Viral expression and molecular profiling in liver tissue versus microdissected hepatocytes in hepatitis B virus-associated hepatocellular carcinoma. J Transl Med 2014;12:230. [Crossref] [PubMed]
- Sekhar V, Pollicino T, Diaz G, et al. Infection with hepatitis C virus depends on TACSTD2, a regulator of claudin-1 and occludin highly downregulated in hepatocellular carcinoma. PLoS Pathog 2018;14:e1006916. [Crossref] [PubMed]
- Love MI, Huber W, Anders S. Moderated estimation of fold change and dispersion for RNA-seq data with DESeq2. Genome Biol 2014;15:550. [Crossref] [PubMed]
- Yu G, Wang LG, Han Y, et al. clusterProfiler: an R package for comparing biological themes among gene clusters. OMICS 2012;16:284-7. [Crossref] [PubMed]
- Liberzon A, Birger C, Thorvaldsdóttir H, et al. The Molecular Signatures Database (MSigDB) hallmark gene set collection. Cell Syst 2015;1:417-25. [Crossref] [PubMed]
- Szklarczyk D, Gable AL, Lyon D, et al. STRING v11: protein-protein association networks with increased coverage, supporting functional discovery in genome-wide experimental datasets. Nucleic Acids Res 2019;47:D607-13. [Crossref] [PubMed]
- Ding W, Chen J, Feng G, et al. DNMIVD: DNA methylation interactive visualization database. Nucleic Acids Res 2020;48:D856-62. [Crossref] [PubMed]
- Hänzelmann S, Castelo R, Guinney J. GSVA: gene set variation analysis for microarray and RNA-seq data. BMC Bioinformatics 2013;14:7. [Crossref] [PubMed]
- Bindea G, Mlecnik B, Tosolini M, et al. Spatiotemporal dynamics of intratumoral immune cells reveal the immune landscape in human cancer. Immunity 2013;39:782-95. [Crossref] [PubMed]
- Ravi R, Noonan KA, Pham V, et al. Bifunctional immune checkpoint-targeted antibody-ligand traps that simultaneously disable TGFβ enhance the efficacy of cancer immunotherapy. Nat Commun 2018;9:741. [Crossref] [PubMed]
- Wang J, Sun J, Liu LN, et al. Siglec-15 as an immune suppressor and potential target for normalization cancer immunotherapy. Nat Med 2019;25:656-66. [Crossref] [PubMed]
- Yi L, Wu G, Guo L, et al. Comprehensive Analysis of the PD-L1 and Immune Infiltrates of m(6)A RNA Methylation Regulators in Head and Neck Squamous Cell Carcinoma. Mol Ther Nucleic Acids 2020;21:299-314. [Crossref] [PubMed]
- Isidro-Sánchez J, Akdemir D, Montilla-Bascón G. Genome-Wide Association Analysis Using R. Methods Mol Biol 2017;1536:189-207. [Crossref] [PubMed]
- Mattei AL, Bailly N, Meissner A. DNA methylation: a historical perspective. Trends Genet 2022;38:676-707. [Crossref] [PubMed]
- Michalczyk K, Cymbaluk-Płoska A. The Role of Zinc and Copper in Gynecological Malignancies. Nutrients 2020;12:3732. [Crossref] [PubMed]
- Feng Y, Zeng JW, Ma Q, et al. Serum copper and zinc levels in breast cancer: A meta-analysis. J Trace Elem Med Biol 2020;62:126629. [Crossref] [PubMed]
- Rebouissou S, Nault JC. Advances in molecular classification and precision oncology in hepatocellular carcinoma. J Hepatol 2020;72:215-29. [Crossref] [PubMed]
- Lee D, Jang MK, Seo JH, et al. ARD1/NAA10 in hepatocellular carcinoma: pathways and clinical implications. Exp Mol Med 2018;50:1-12. [Crossref] [PubMed]
- Carloni V, Lulli M, Madiai S, et al. CHK2 overexpression and mislocalisation within mitotic structures enhances chromosomal instability and hepatocellular carcinoma progression. Gut 2018;67:348-61. [Crossref] [PubMed]
- Vijayraghavan S, Schwacha A. The eukaryotic Mcm2-7 replicative helicase. Subcell Biochem 2012;62:113-34. [Crossref] [PubMed]
- Liu Z, Li J, Chen J, et al. MCM family in HCC: MCM6 indicates adverse tumor features and poor outcomes and promotes S/G2 cell cycle progression. BMC Cancer 2018;18:200. [Crossref] [PubMed]
- Lee S, Rauch J, Kolch W. Targeting MAPK Signaling in Cancer: Mechanisms of Drug Resistance and Sensitivity. Int J Mol Sci 2020;21:1102. [Crossref] [PubMed]
- Peluso I, Yarla NS, Ambra R, et al. MAPK signalling pathway in cancers: Olive products as cancer preventive and therapeutic agents. Semin Cancer Biol 2019;56:185-95. [Crossref] [PubMed]
- Dong LQ, Peng LH, Ma LJ, et al. Heterogeneous immunogenomic features and distinct escape mechanisms in multifocal hepatocellular carcinoma. J Hepatol 2020;72:896-908. [Crossref] [PubMed]
- Kogame M, Nagai H, Shinohara M, et al. Th2 Dominance Might Induce Carcinogenesis in Patients with HCV-related Liver Cirrhosis. Anticancer Res 2016;36:4529-36. [Crossref] [PubMed]
- Lorvik KB, Hammarström C, Fauskanger M, et al. Adoptive Transfer of Tumor-Specific Th2 Cells Eradicates Tumors by Triggering an In Situ Inflammatory Immune Response. Cancer Res 2016;76:6864-76. [Crossref] [PubMed]
- Rezende RM, Lanser AJ, Rubino S, et al. γδ T cells control humoral immune response by inducing T follicular helper cell differentiation. Nat Commun 2018;9:3151. [Crossref] [PubMed]
- DeNardo DG, Barreto JB, Andreu P, et al. CD4(+) T cells regulate pulmonary metastasis of mammary carcinomas by enhancing protumor properties of macrophages. Cancer Cell 2009;16:91-102. [Crossref] [PubMed]
- Hiam-Galvez KJ, Allen BM, Spitzer MH. Systemic immunity in cancer. Nat Rev Cancer 2021;21:345-59. [Crossref] [PubMed]
- Cariani E, Missale G. Immune landscape of hepatocellular carcinoma microenvironment: Implications for prognosis and therapeutic applications. Liver Int 2019;39:1608-21. [Crossref] [PubMed]
- Lee HW, Cho KJ, Park JY. Current Status and Future Direction of Immunotherapy in Hepatocellular Carcinoma: What Do the Data Suggest? Immune Netw 2020;20:e11. [Crossref] [PubMed]