Cytokine-induced apoptosis inhibitor 1: a comprehensive analysis of potential diagnostic, prognosis, and immune biomarkers in invasive breast cancer
Highlight box
Key findings
• Cytokine-induced apoptosis inhibitor 1 (CIAPIN1) is a promising diagnostic and prognostic marker for invasive breast cancer (IBC).
What is known and what is new?
• Some studies have shown that CIAPIN1 was highly expressed in cholangiocarcinoma and has prognostic significance.
• CIAPIN1 is a promising diagnostic and prognostic marker for IBC.
What is the implication, and what should change now?
• CIAPIN1 levels may have a significant prognostic value in invasive breast cancer and it may be a target for invasive breast cancer immunotherapy.
Introduction
At present, breast cancer is a high incidence of malignant tumors that endanger the lives and health of females globally. According to the 2022 International Agency for Research on Cancer statistics, breast cancer was the first among women’s malignancies in the United States, with more than 339,000 new cases (1). Despite the continuous development and improvement of diagnosis and treatment technology, breast cancer, with more than 43,000 new deaths in the United States in 2022, remains the primary reason of death among women (2). Invasive breast cancer (IBC) is the commonest type of breast cancer. Different molecular subtypes of IBC have different biological and clinical characteristics. IBC is a group of heterogeneous diseases. The heterogeneity of tumors leads to great individual differences in the medical therapy response and prognosis of subjects, and there is still no complete cure method. The recurrence and distant metastasis of tumor cells are the main reasons for treatment failure and death in individuals at advanced stage. Now, the early detection of IBC relies primarily on the CA153 and CEA serum biomarkers, but they still show low sensitivity and specificity (3,4). Therefore, we need to further explore molecular biomarkers or targets for more effective diagnosis, treatment and prevention of IBC.
Cytokine-induced apoptosis inhibitor 1 (CIAPIN1), also called anamorsin, is encoded by the CIAPIN1 gene situated on chromosome 16q21 and has a molecular weight of 33 kDa. It accumulates in the nucleus and localizes to the cytoplasm, nucleus and mitochondria (5,6). As a regulator and important effector of non-homologous RAS signaling pathway, CIAPIN1 is different from the caspase family and BCL-2 family (7). CIAPIN1 is widely distributed in fetal and adult normal tissues, especially in differentiated tissues and activated metabolic tissues (8). The current research revealed that CIAPIN1 can suppress the overgrowth of tumor cells, like non-small cell lung malignancy and pancreatic tumor (9,10). Per contra, studies have suggested that CIAPIN1 is connected with worse prognosis of a variation of tumors, such as stomach adenocarcinoma (11), cholangiocarcinoma (12) and ovarian serous carcinoma (13). In diffuse large B-cell lymphoma and leukemia cells, the up-regulation of CIAPIN1 gene can significantly promote the development of cancer cells, and the degree of expression is inversely proportional to the prognostic index (14). At present, there are few reports about the CIAPIN1 expression in IBC, and the effect of CIAPIN1 expression in IBC is still unclear. CIAPIN1 might act as a potential molecular target for IBC. We aimed to investigate CIAPIN1 expression in IBC and its possible diagnostic and prognostic importance.
Online databases such as TIMER and TCGA were used to examine the relation between CIAPIN1 expression and clinicopathological features, diagnostic and prognosis importance of IBC. Furthermore, the co-expression genes of CIAPIN1 and the possible cellular mechanisms were studied. Last, the correlation between CIAPIN1 expression and immune checkpoint genes and cancer immune cell infiltration was investigated, and the potential mechanism of CIAPIN1 involvement in the development and advancement of IBC was discussed. We present this article in accordance with the TRIPOD reporting checklist (available at https://tcr.amegroups.com/article/view/10.21037/tcr-23-34/rc).
Methods
Data acquisition
TIMER database (http://timer.cistrome.org/) (15) and TCGA database (https://portal.gdc.cancer.gov/) were utilized to examine CIAPIN1 expression levels in 33 kinds of human tumors. The TCGA-Breast Invasive Carcinoma (BRCA) dataset was obtained from the TCGA database, including RNAseq data and corresponding clinicopathological data of 113 normal samples and 1,109 IBC tumor samples. Data were converted to log2 Transcripts Per Million to analyze CIAPIN1 expression differences between IBC samples and healthy tissues. GSE45827 (16) and GSE65194 (17) datasets were obtained from the GEO database (https://www.ncbi.nlm.nih.gov/geo/) for confirmation. Expression level of CIAPIN1 protein in IBC and the immunohistochemical results of CIAPIN1 expression in human IBC tissues were obtained from Clinical Proteomic Tumor Analysis Consortium (PCTAC) database and the Human Protein Atlas (HPA) database (http://www.proteinatlas.org), respectively. The study was conducted in accordance with the Declaration of Helsinki (as revised in 2013).
Clinicopathological characteristics
CIAPIN1 was classified into low expression and elevated expression groups depending on the median expression values obtained in the TCGA-BRCA dataset, and the variations in CIAPIN1 expression between various clinical groups were tested.
Survival analysis
CIAPIN1 was separated into groups of low or elevated expression depending on the median CIAPIN1 mRNA expression. Overall survival (OS), relapse free survival (RFS), distant metastasis free survival (DMFS), disease specific survival (DSS), and progress free interval (PFI) curves were all accomplished utilizing Kaplan-Meier assessment (18) and the log-rank test. Then, we obtained GSE1456-GPL96 (19), GSE4922-GPL96 (20), GSE7390 (21) and GSE12276 (22) datasets from GEO database for confirmation. Furthermore, Cox regression analysis was performed on clinicopathological properties and CIAPIN1 expression level of patients in TCGA-BRCA dataset to screen out risk factors related to DSS and progression free survival (PFS).
Diagnostic value analysis
To investigate the diagnostic significance of CIAPIN1 in connection to various clinical parameters of IBC, a receiver operating characteristic (ROC) curve was created to illustrate the link between the sensitivity and specificity of CIAPIN1 in the diagnosis of IBC. The nomogram model prediction scale was constructed depending on the outcomes of multivariate Cox regression examination to predict the survival probability of IBC patients. The concordance index (C-index) and calibration plots were employed to assess how well the nomogram functioned.
Differentially expressed gene (DEG) analysis
Based on the median score of CIAPIN1 expression, individuals in the TCGA-BRCA dataset were divided into elevated or reduced CIAPIN1 expression categories. The DEG assessment between these two clusters was conducted employing the R package DESeq2, and the thresholds for DEGs were adjusted as P value <0.05 and |log2-fold-change (FC)|>1. Employing Spearman’s correlation analysis, the relationship between the expression of the top 20 DEGs and CIAPIN1 was assessed.
Protein-protein interaction (PPI) network construction and functional enrichment examination
CIAPIN1 interaction network of related functional proteins was constructed and visualized using STRING database (https://cn.string-db.org/), and the reaction between CIAPIN1 and related proteins was analyzed. The main biological functions, predicted pathways and related functions of CIAPIN1 and its related proteins were analyzed by utilizing Gene Ontology (GO) and Kyoto Encyclopedia of Genes and Genomes (KEGG). The enrichment of DEGs in earlier mechanisms was then confirmed by employing Gene Set Enrichment Analysis (GSEA). The gene sets were obtained from the MSigBD Collections database (http://www.gsea-msigdb.org/gsea/msigdb/index.jsp) (23).
DNA methylation examination
We used the UALCAN database (http://ualcan.path.uab.edu/index.html) (24) to study the methylation status of the CIAPIN1 promoter. The DNA methylation status of CIAPIN1 gene CpG sites in TCGA-BRCA dataset and its prognostic value were analyzed by MethSurv database (https://biit.cs.ut.ee/methsurv/) (25). Additionally, we evaluated the association between CpG methylation status of CIAPIN1 and OS in IBC patients.
Correlation assessment between CIAPIN1 expression levels, TP53, and immune checkpoints in IBC
Utilizing R program and Spearman’s correlation approach, the relation between the expression levels of CIAPIN1, TP53, and immune checkpoint genes (like PDCD-1 and TIGIT) was examined in the TCGA-BRCA dataset.
Correlation analysis of immune cell infiltration
The connection between CIAPIN1 and cancer purity, in addition to other immunocytes like CD8+ T cells, NK cells, and neutrophils, was examined using the TIMER database. The association between the expression of CIAPIN1 and the infiltration of 24 different types of immune cells was assessed using the Spearman’s correlation assessment. In the TCGA-BRCA dataset, immune scores were calculated and compared across groups of low and high CIAPIN1 expression level by utilizing the Estimation of Stromal and Immune cells in Malignant Tumor tissues Using Expression (ESTIMATE) program and the Wilcoxon rank-sum test.
Statistical analysis
All statistical examination were conducted by utilizing R (version 3.6.3), and R package ggplot2 (version 3.3.3) was employed for displaying. The connection between CIAPIN1 expression and clinicopathological variables was analyzed by χ2 test or Fisher examination. The log-rank approach was employed to assess if one survival curve differed from another after the Kaplan-Meier method was employed to create the curves. Cox regression analysis determined independent prognostic factors and estimated hazard ratios (HRs) and 95% confidence intervals (CIs) for various clinical features. For all assays, the significance threshold was considered to be two-sided and P values of less than 0.05.
Results
Compared with the normal tissue, CIAPIN1 was of high expression in IBC
First, the expression level of CIAPIN1 mRNA was assessed for 33 human cancers included in the TCGA database. Compared with healthy tissues, CIAPIN1 expression level was substantially raised in IBC (P<0.001). CIAPIN1 was also greatly expressed in the following cancers: uterine corpus endometrial carcinoma, bladder urothelial carcinoma, colon adenocarcinoma, esophageal carcinoma, head and neck squamous cell carcinoma, renal papillary cell carcinoma, liver hepatocellular carcinoma, lung squamous cell carcinoma, rectal adenocarcinoma, stomach adenocarcinoma, and colon adenocarcinom (P<0.001). CIAPIN1 expression levels were lower in chromophobe, clear cell and thyroid carcinomas than in healthy tissues (P<0.001) (Figure 1A). CIAPIN1 was substantially overexpressed in IBC in both paired and unpaired samples (P<0.001) (Figure 1B,1C). Meanwhile, GSE45827 and GSE65194 datasets in GEO database were used for verification, which were consistent with the above results (Figure 1D,1E). Furthermore, CIAPIN1 protein levels in the CPATC dataset were significantly expressed in IBC samples (P<0.001) (Figure 1F). Immunohistochemical outcomes from the HPA database also illustrated that CIAPIN1 protein level was significantly expressed in the cytoplasm and membrane of IBC cells, but not expressed or weakly expressed in healthy tissues (Figure 2).
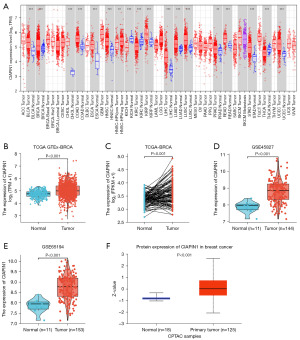
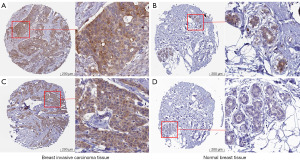
CIAPIN1 expression level was connected with various clinicopathological features in individuals with IBC
The clinicopathological characteristics of 1,065 samples with retained clinical information in the TCGA-BRCA dataset were analyzed. Correlation analysis indicted that CIAPIN1 was statistically various from T stage (P<0.001), race (P=0.001), age (P<0.001), histological type (P<0.001), ER status (P<0.001), PR status (P<0.001), PAM50 (P<0.001), DSS event (P=0.021) and PFI event (P=0.049). There were no significant differences between CIAPIN1 and N stage, M stage, pathologic phase, menopause status, radiation_treatment, and OS event (P>0.05) (Table 1).
Table 1
Characteristic | Low-CIAPIN1 expression | High-CIAPIN1 expression | P |
---|---|---|---|
Total number of patients | 532 | 533 | |
T stage, n (%) | |||
T1 | 155 (14.6) | 120 (11.3) | <0.001 |
T2 | 278 (26.2) | 337 (31.7) | |
T3 | 83 (7.8) | 54 (5.1) | |
T4 | 15 (1.4) | 20 (1.9) | |
N stage, n (%) | |||
N0 | 266 (25.4) | 241 (22.6) | 0.075 |
N1 | 170 (16.3) | 179 (17.1) | |
N2 | 46 (4.4) | 70 (6.7) | |
N3 | 40 (3.8) | 34 (3.3) | |
M stage, n (%) | |||
M0 | 437 (48.1) | 452 (49.7) | 0.142 |
M1 | 6 (0.7) | 14 (1.5) | |
Pathologic stage, n (%) | |||
Stage I | 102 (9.8) | 78 (7.5) | 0.126 |
Stage II | 299 (28.7) | 307 (29.5) | |
Stage III | 114 (10.9) | 124 (11.9) | |
Stage IV | 6 (0.6) | 12 (1.2) | |
Race, n (%) | |||
Asian | 21 (2.2) | 39 (3.7) | 0.001 |
Black or African American | 74 (7.6) | 105 (10.8) | |
White | 391 (40.1) | 346 (35.5) | |
Age (years), n (%) | |||
≤60 | 265 (24.9) | 323 (30.3) | <0.001 |
>60 | 267 (25.1) | 210 (19.7) | |
Histological type, n (%) | |||
Infiltrating ductal carcinoma | 314 (32.7) | 443 (46.2) | <0.001 |
Infiltrating lobular carcinoma | 169 (17.6) | 33 (3.4) | |
ER status, n (%) | |||
Negative | 68 (6.7) | 169 (16.6) | <0.001 |
Indeterminate | 0 (0) | 2 (0.2) | |
Positive | 444 (43.7) | 334 (32.8) | |
PR status, n (%) | |||
Negative | 120 (11.8) | 218 (21.5) | <0.001 |
Indeterminate | 1 (0.1) | 3 (0.3) | |
Positive | 391 (38.5) | 283 (27.9) | |
HER2 status, n (%) | |||
Negative | 280 (39.1) | 268 (37.4) | 0.056 |
Indeterminate | 3 (0.4) | 9 (1.3) | |
Positive | 68 (9.5) | 89 (12.4) | |
PAM50, n (%) | |||
Normal | 19 (1.8) | 21 (2.0) | <0.001 |
LumA | 357 (33.5) | 194 (18.2) | |
LumB | 83 (7.8) | 119 (11.2) | |
Her2 | 28 (2.6) | 54 (5.1) | |
Basal | 45 (4.2) | 145 (13.6) | |
Menopause status, n (%) | |||
Pre | 98 (10.3) | 126 (13.2) | 0.129 |
Peri | 19 (1.8) | 20 (2.1) | |
Post | 357 (37.3) | 336 (35.1) | |
Radiation_therapy, n (%) | |||
No | 222 (22.8) | 210 (21.6) | 0.715 |
Yes | 270 (27.8) | 270 (27.8) | |
OS event, n (%) | |||
Alive | 466 (43.8) | 452 (42.4) | 0.218 |
Dead | 66 (6.2) | 81 (7.6) | |
DSS event, n (%) | |||
Alive | 493 (47.1) | 472 (45.1) | 0.021 |
Dead | 30 (2.9) | 51 (4.9) | |
PFI event, n (%) | |||
Alive | 472 (44.3) | 450 (42.3) | 0.049 |
Dead | 60 (5.6) | 83 (7.8) | |
Age, median [IQR] | 61 [51, 69] | 56 [47, 66] | <0.001 |
IBC, invasive breast cancer; TCGA, The Cancer Genome Atlas; CIAPIN1, Cytokine-induced apoptosis inhibitor 1; T, tumor; N, lymph node; M, metastasis; ER, estrogen receptor; PR, progesterone receptor; HER2, human epidermal growth factor receptor 2; PAM50, prediction analysis of microarray 50; OS, overall survival; DSS, disease specific survival; PFI, progress free interval; IQR, interquartile range.
CIAPIN1 was related to poor prognosis in IBC subjects
The survival curve was visualized using Kaplan-Meier assay and the logrank assessment to determine the relationship between CIAPIN1 and survival in patients with IBC. As shown in Figure 3, higher CIAPIN1 mRNA levels were significantly connected with poorer OS (HR: 1.63, P<0.001), RFS (HR: 1.35, P<0.001), and DMFS (HR: 1.61, P<0.001) (Figure 3A-3C). Meanwhile, GSE1456-GPL96, GSE4922-GPL96, GSE7390 and GSE12276 datasets in GEO database were used for verification, which were consistent with the above results (Figure 3D-3J). Further, the connection between CIAPIN1 expression and prognosis in various IBC subgroups was evaluated. The results found that regardless of DSS or PFI, individuals with high CIAPIN1 expression had significantly poorer prognosis in N1&N2&N3 stage, white, age >60 years, post menopause status, and anatomic neoplasm subdivision-left subgroups (P<0.05) (Figure 4).
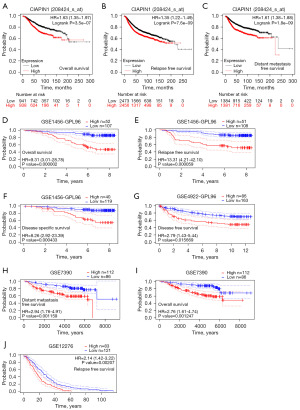
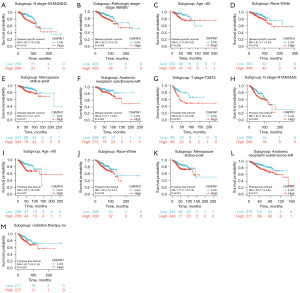
Cox regression assessment assessed the risk factors related to OS, DSS, and PFS in patients with CIAPIN1 in IBC. In the univariate Cox model, clinicopathological characteristics with P<0.1 were included for multivariate Cox assay. Univariate analysis indicated that T phase, N phase, M phase, pathologic stage and PAM50 were significantly associated with OS, DSS and PFS in subjects with IBC. CIAPIN1 expression level, ER status and PR status were considerably related to DSS and PFS. Menopause status and radiation therapy were associated with OS. In multivariate Cox model, T stage (HR: 2.470, P<0.05), age (HR: 2.645, P<0.01), menopause status (HR: 0.332, P<0.05) and radiation_therapy (HR: 0.470, P<0.05) were independent risk factors for OS in subjects with BRCA. In IBC individuals, ER condition was a distinct risk factor for OS and DSS (P<0.05). In subjects with IBC, M stage and pathologic phase were independent risk factors for DSS and PFS (P<0.001) (Table 2).
Table 2
Characteristics | HR for overall survival (95% CI) | HR for disease-specific survival (95% CI) | HR for progression-free survival (95% CI) | |||||
---|---|---|---|---|---|---|---|---|
Univariate | Multivariate | Univariate | Multivariate | Univariate | Multivariate | |||
T stage (T3&T4 vs. T1&T2) | 1.673** | 2.470* | 2.037** | 0.847 | 2.117*** | 1.026 | ||
N stage (N1&N2&N3 vs. N0) | 2.145 *** | 1.004 | 3.584*** | 1.877 | 2.250*** | 1.363 | ||
M stage (M1 vs. M0) | 4.327*** | 1.965 | 7.697*** | 5.114*** | 8.288*** | 4.167*** | ||
Pathologic stage (Stage III&IV vs. I&II) | 2.519*** | 2.259 | 3.870*** | 2.559* | 2.962*** | 2.030* | ||
Age (>60 vs. ≤60) | 2.036*** | 2.645** | 1.418 | – | 1.232 | – | ||
ER status (positive vs. negative) | 0.704 | 0.369* | 0.523** | 0.420* | 0.599** | 0.784 | ||
PR status (positive vs. negative) | 0.762 | – | 0.529** | 0.881 | 0.567*** | 0.638 | ||
PAM50 (LumA&LumB&Basal vs. Her2) | 0.509** | 0.830 | 0.447* | 0.842 | 0.529* | 0.806 | ||
Menopause status (Pre&Peri vs. Post) | 0.416*** | 0.332* | 0.628 | – | 0.889 | – | ||
Radiation_therapy (yes vs. no) | 0.558** | 0.470* | 0.755 | – | 0.885 | – | ||
CIAPIN1 (high vs. low) | 1.287 | – | 1.772 * | 1.513 | 1.482* | 1.192 |
*, P<0.05, **, P<0.01, ***, P<0.001. IBC, invasive breast cancer; HR, hazard ratio; CI, confidential interval; T, tumor; N, lymph node; M, metastasis; ER, estrogen receptor; PR, progesterone receptor; PAM50, prediction analysis of microarray 50; CIAPIN1, Cytokine-induced apoptosis inhibitor 1.
CIAPIN1 had potential diagnostic value in IBC
The findings herein confirmed that CIAPIN1 expression was associated with clinical features such as ER status, PR status, M stage and pathologic stage in IBC. Therefore, the ROC curve was used to analyze the effectiveness of CIAPIN1 mRNA expression level in differentiating breast malignancy tissues from healthy tissues. Compared with the normal tissue group, the zone below curve (AUC) of CIAPIN1 in the ER(−) group was 0.880 (95% CI: 0.845–0.915), with 78.1% sensitivity and 89.2% specificity (Figure 5A). The AUC of the PR(−) group was 0.815 (95% CI: 0.777–0.853), with 68.9% sensitivity and 89.2% specificity (Figure 5B). The AUC of the M1 stage group was 0.875 (95% CI: 0.790–0.960), with 85.0% sensitivity and 77.5% specificity (Figure 5C). In the pathologic stage IV group, AUC was 0.895 (95% CI: 0.820–0.970), with 88.9% sensitivity and 77.5% specificity (Figure 5D). The AUC of the Asian group was 0.833 (95% CI: 0.762–0.903), with 81.7% sensitivity and 76.6% specificity (Figure 5E). The nomogram we constructed, including CIAPIN1 expression levels and highly significant clinical prognostic variables, and calibration curves were employed to assess the nomogram predictive effectiveness (Figure 5F-5I). The C-index of this model was 0.717 (95% CI: 0.692–0.743), which revealed moderate accuracy in anticipating the OS of subjects with IBC at 1, 3 and 5 years.
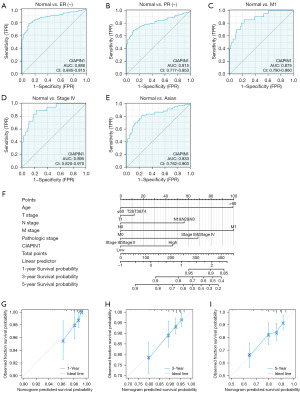
DEGs between elevated- and low-CIAPIN1 expressing IBC individuals
In the TCGA-BRCA dataset, compared with the CIAPIN1 low expression cohort, there were 884 DEGs in the CIAPIN1 high expression group, among which 756 DEGs were raised and 128 DEGs were reduced (Padj<0.05, |Log2-FC|>1.5) (Figure 6A). The single gene co-expression heat map showed the top 20 DEGs most significant with CIAPIN1 (Figure 6B).
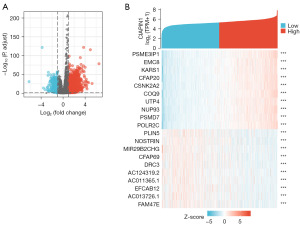
PPI network and enrichment examination of CIAPIN1 co-expressing genes in IBC
Using the STRING tool to determine the adjacent nodes of the CIAPIN1 gene network. The PPI network contained 21 nodes and 160 edges, with an enrichment P value <0.001 (Figure 7A). The top 10 genes related to CIAPIN1 included GLRX3, NDOR1, NUBP1, NUBP2, BOLA1, NARFL, GLRX5, CHCHD4, BOLA3 and BOLA2B, and the interaction scores with CIAPIN1 were all higher than 0.90 (Table 3). According to the outcomes of the GO analysis, the DEGs connected with CIAPIN1 were particularly abundant in biological pathways (BP), including metallo-sulfur cluster construction, iron-sulfur cluster assembly, and protein maturation through iron-sulfur collection transfer. Molecular function (MF) mechanisms including metal cluster binding, iron-sulfur group binding, and 4-iron, 4-sulfur group binding were particularly concentrated in this region. DNA replication, base removal repair, and mismatch repair were substantially enriched in the DEGs linked with CIAPIN1 according to KEGG mechanism enrichment analysis (Figure 7B,7C). GSEA showed that neutrophil degranulation and signaling by interleukins, signaling by RHO GTPases, m phase, and metabolism of amino acids and derivatives were significant enrichment pathways in the CIAPIN1 high expression group (Figure 7D,7E).
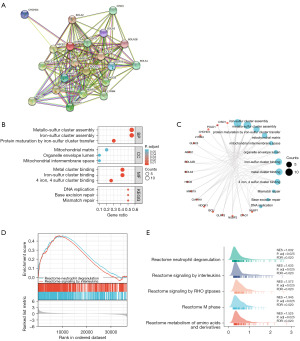
Table 3
Gene symbol | Annotation | Score |
---|---|---|
GLRX3 | Glutaredoxin-3 | 0.999 |
NDOR1 | NADPH-dependent diflavin oxidoreductase 1 | 0.999 |
NUBP1 | Cytosolic Fe-S cluster assembly factor NUBP1 | 0.997 |
NUBP2 | Cytosolic Fe-S cluster assembly factor NUBP2 | 0.980 |
BOLA1 | BolA family member 1 | 0.975 |
NARFL | Cytosolic Fe-S cluster assembly factor NARFL | 0.936 |
GLRX5 | Glutaredoxin-related protein 5, mitochondrial | 0.931 |
CHCHD4 | Mitochondrial intermembrane space import and assembly protein 40 | 0.921 |
BOLA3 | BolA-like protein 3 | 0.920 |
BOLA2B | BolA family member 2B | 0.918 |
BOLA2 | BolA-like protein 2 | 0.905 |
MMS19 | MMS19 nucleotide excision repair protein homolog | 0.904 |
CIAO1 | Probable cytosolic iron-sulfur protein assembly protein CIAO1 | 0.898 |
ABCB7 | ATP-binding cassette sub-family B member 7, mitochondrial | 0.828 |
POLD1 | DNA polymerase delta catalytic subunit | 0.826 |
ABCE1 | ATP-binding cassette sub-family E member 1 | 0.823 |
HSCB | Iron-sulfur cluster co-chaperone protein HscB, mitochondrial | 0.822 |
LYRM4 | LYR motif-containing protein 4 | 0.812 |
ISCU | Iron-sulfur cluster assembly enzyme ISCU, mitochondrial | 0.809 |
CISD1 | CDGSH iron-sulfur domain-containing protein 1 | 0.802 |
Methylation status of the CIAPIN1 gene was connected with the prognosis of individuals with IBC
According to the UALCAN database, promoter methylation level was higher in IBC malignancy tissues than in healthy breast tissues (P<0.001) (Figure 8A). The heat map of CIAPIN1 methylation analyzed by MetSurv tool. cg09373350, cg09675895, cg06919205 and cg27589921 methylated CpG islands were shown, and CIAPIN1 methylation level was elevated in these four CpG islands (Figure 8B). In addition, increased CIAPIN1 methylation in cg09675895 compared with patients with lower CpG methylation in CIAPIN1 was related to poorer OS in IBC patients (HR: 1.707; CI: 1.11–2.627, P=0.012) (Figure 8C).
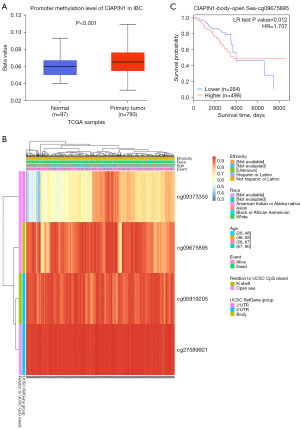
The expression level of CIAPIN1 was related with TP53 expression and immune checkpoint genes in IBC
As a tumor suppressor gene, TP53 was significantly up-regulated in malignant tumors. In addition, CTLA-4, PDCD-1, TIGIT, CD276, LAG3 and PVR (CD155) were momentous immune checkpoint protein, which was closely related to immune escape of tumor cells. The expression level of CIAPIN1 indicated positive relation with the expression levels of TP53, CTLA-4, PDCD-1, TIGIT, IDO1, CD276, LAG3, CD155, ICOS and SIGLEC7 in the TCGA-BRCA dataset (P<0.001) (Figure 9).
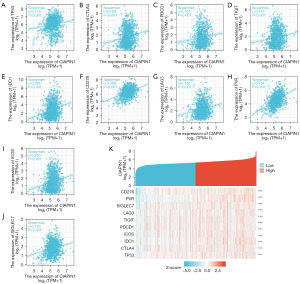
CIAPIN1 expression levels correlated with the infiltration of several immune cell categories in IBC
CIAPIN1 and B cells, CD8+ T cells, CD4+ T cells, macrophages, neutrophils, and dendritic cells in IBC revealed statistically significant relationships (P<0.001) in TIMER data (Figure 10A). By using ssGSEA, the infiltration status of 24 various types of immune cells in the IBC tissues was assessed. By employing Spearman’s correlation assessment, it was estimated that CIAPIN1 expression and immune cell infiltration were associated. T helper (Th) cells 2, Th1 cells, activated dendritic cells (aDC), regulatory T cells (TReg), macrophages, natural killer (NK) CD56dim cells, Tgd cells, dendritic cells (DC), and B cells were among the 9 immune cell categories that were linked with CIAPIN1 expression (P<0.001). Seven different immune cell categories, such as plasmacytoid dendritic cells (pDC), NK cells, NK CD56bright cells, Th17 cells, mast cells, eosinophils, and CD8+ T cells, were negatively related with CIAPIN1 expression (P<0.001) (Figure 10B-10D). According to the ESTIMATE methodology, the elevated-CIAPIN1 cohort scored higher on immunity than the low-CIAPIN1 group (P=0.021) (Figure 10E).
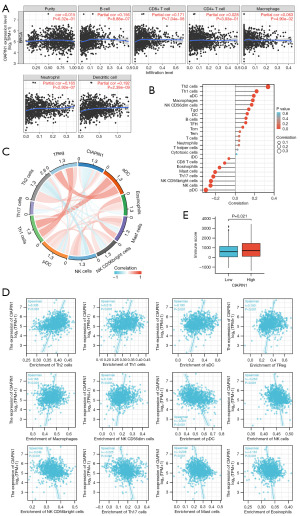
Discussion
In our study, CIAPIN1 mRNA expression level was substantially expressed in 14 malignant cancers, including IBC, cholangiocarcinoma, liver hepatocellular carcinoma and non-small cell lung cancer, which is consistent with earlier outcomes (12,26,27). Concurrently, CIAPIN1 expression level protein in IBC was also considerably more than that in healthy tissues in PCTAC database and HPA database. Kaplan-Meier examination discovered that overexpression of CIAPIN1 was strictly linked to worse OS, RFS and DMFS in individuals with IBC. We used GEO database to download four datasets for survival analysis, and the results are consistent with those mentioned above. Similarly, some preceding investigations have illustrated that CIAPIN1 expression might be a probable prognostic marker of decreased survival in individuals with certain solid cancers, like stomach adenocarcinoma (11), cholangiocarcinoma (12), ovarian serous carcinoma (13), and colon adenocarcinoma (28). We evaluated the prognostic profile of CIAPIN1 in different IBC subgroups and discovered that raised CIAPIN1 expression was considerably connected to adverse clinicopathological factors in IBC patients, such as high TNM stage, high pathological stage, advanced age, and post menopause status. Cox analysis showed that CIAPIN1 was a hazard factor for bad prognosis in IBC patients and was significantly negatively correlated with DSS and PFS in IBC patients. Study have shown that overexpressed CIAPIN1 can promote angiointima formation and cell proliferation and migration by regulating p53 and JAK2-STAT3 of vascular smooth muscle cells, which may play a part in tumor proliferation (29). CIAPIN1 has an important function in preventing apoptosis and supporting cell growth of lung cancer. miR-195-5p can directly target CIAPIN1 to suppress cancer cell growth and cause G0/G1 stage stop and apoptosis (27). Our above results indicate that CIAPIN1 might act as a tumor promoting gene of IBC, which promotes tumor proliferation and metastasis.
At present, early detection is the key to tumor treatment. ROC analysis and nomogram were used to evaluate the diagnostic and prognostic significance of CIAPIN1 in IBC. Our data analysis shows that CIAPIN1 has a certain diagnostic value in distinguishing ER-negative, M1 stage, pathological stage IV breast cancer from normal tissues. Recently, a novel 7-AAb (cancer associated autoantibodies) panel including CIAPIN1 was reported to be not only better than AFP in overall detection of liver hepatocellular carcinoma, but also showed good diagnostic potential in AFP(−) and early LIHC (30). Our nomogram C-index prediction model was 0.717, which showed a moderate accuracy of prediction. It can accurately estimate the OS of IBC individuals at 1, 3 and 5 years. These data indicate that CIAPIN1 can be employed as a probable marker for diagnosis and prognosis of IBC.
In order to discover the probable mechanism and role of CIAPIN1 in IBC, we conducted GO and KEGG enrichment examination on DEGs of CIAPIN1. Our data show that CIAPIN1 associated DEGs were enriched in metallo-sulfur cluster assembly, iron-sulfur cluster binding, DNA replication, and base excision repair. As an Fe-S cluster binding protein, CIAPIN1 is involved in many important life processes, including regulation of gene expression, DNA repair and metabolic reactions (31,32). CIAPIN1 may affect the transcription level of genes by directly or indirectly regulating the expression of cell cyclin-related proteins, and play an critical role in multi-drug resistance of IBC and promoting the proliferation of LIHC cells (33,34). Since IBC patients with high CIAPIN1 expression showed poor OS, RFS and DMFS, we investigated the possible cellular mechanism by GSEA. Our data revealed that signaling by interleukins was significantly correlated enrichment pathway in the CIAPIN1 high expression group. IL-8 is involved in inflammation and immune defense response in vivo. Most tumors can secrete IL-8 to promote their own growth and participate in the formation of tumor microenvironment (35). These data suggest that high expression of CIAPIN1 promotes IBC by regulating cell cycle and DNA replication. However, further experiments are needed to verify the results.
DNA methylation is an usual epigenetic mechanism, and variations in the methylation status of some genes are connected to the origin, proliferation and metastasis deterioration of tumors (36). Our data show that high CIAPIN1 expression in IBC was related with promoter methylation, and IBC patients with high CIAPIN1 methylation showed poor OS. DNA methylation may affect chromatin construction, DNA conformation, DNA stability, and the manner DNA interacts with proteins, all of which can modify the way genes are expressed. Our KEGG results found that CIAPIN1 related DEGs were significantly enriched in DNA replication and repair, suggesting that the hypermethylation level of CIAPIN1 may promote the progression of IBC by affecting the repair of tumor DNA damage, which needs further investigation.
Nowadays, not many publications are available on the relationship between CIAPIN1 and immune cells in IBC. Immune cells that infiltrate tumors have been shown to have predictive significance in solid tumors and may be applied to anticipate how a patient will respond to immune checkpoint inhibitor (ICI) treatment (37). Our investigation supports the hypothesis that tumor immune cell infiltration and CIAPIN1 expression may be correlated. In IBC tissues, CIAPIN1 expression was positively linked with Th2 cells, Th1 cells, TReg, and macrophages. T cells mediate tumor antigen-specific immune responses through the specificity and diversity of their clonotypic T cell receptors. In kidney cell carcinoma and melanoma, adjacent tumors and immune cells show different gene expressions, and T cell status and invasion degree are different within and between clones (38). CIAPIN1 expression was negatively related with pDC, NK cells, NK CD56bright cells and CD8+ T cells in IBC tissues. NK cells are a special type of immunity cell that could eliminate nearby cells with surface markers associated with oncogenic transformation. The research on tumor immunotherapy based on NK cells has increased exponentially in recent years (39). The negative correlation between CIAPIN1 expression and NK cell infiltration in IBC suggests that CIAPIN1 may inhibit NK cell activation. Impaired NK cell function can increase the incidence of breast cancer and tumors in animal models (40). Thus, our data suggest that highly expressed CIAPIN1 has an irreplaceable function in the immunity escape mechanism of IBC tumor cells. CIAPIN1 affects T cells and other immune cells, thereby manipulating anti-tumor immune responses and influencing the progression and prognosis of IBC patients.
Although ICI treatment has demonstrated great results in the management of IBC with the development of immunotherapy, only a limited number of patients benefit (41). TIGIT, CD276, PD-1, CD155, LAG3 and CTLA-4 are key target molecules associated with tumor immune escape (42,43). According to our findings, immune checkpoint genes and TP53 expression levels in IBC are significantly positively correlated with CIAPIN1 expression levels. During tumor proliferation, the highly expressed CD155 receptor on the surface of effector lymphocytes assists the immune escape of tumor cells by suppressing the cytotoxic killing ability of lymphocytes (44). Combined use of anti-TGIT and anti-PD-1 ICIs can promote rejection in tumor models and increase the proportion of cytotoxic T cells to regulatory T cells in cancers. Simultaneously, the anti-tumor impact of CD8+ T cells is enhanced, which significantly suppressed cancer development and lengthening patient survival (45,46). In addition, TP53 is overexpressed in malignant tumors, and TP53 mutation can inhibit anti-tumor immunity and reduce the effect of tumor immunotherapy, which is correlative with poor prognosis of a variety of malignancies (47). Our findings found that CIAPIN1 may have a negative regulatory function in tumor immunity.
Our findings give novel insights into the connection between CIAPIN1 expression and diagnostic and prognostic significance in individuals with IBC, several limitations remain. Our results were based on IBC samples from online databases and were not validated in vivo or in vitro. Therefore, we will elucidate the potential mechanism of CIAPIN1 in IBC by using cell and animal model experiments.
Conclusions
All in all, our study confirms that CIAPIN1 is overexpressed in IBC and is a vital factor for the adverse prognosis of patients with IBC. CIAPIN1 expression levels correlate with the infiltration of multiple tumor immune cells and might have a function in the immunotherapy of IBC patients. Thus, CIAPIN1 is a promising diagnostic biomarker and immunotherapeutic target in IBC. However, further investigation is required to confirm the results.
Acknowledgments
Funding: This study was funded by
Footnote
Reporting Checklist: The authors have completed the TRIPOD reporting checklist. Available at https://tcr.amegroups.com/article/view/10.21037/tcr-23-34/rc
Peer Review File: Available at https://tcr.amegroups.com/article/view/10.21037/tcr-23-34/prf
Conflicts of Interest: All authors have completed the ICMJE uniform disclosure form (available at https://tcr.amegroups.com/article/view/10.21037/tcr-23-34/coif). The authors have no conflicts of interest to declare.
Ethical Statement: The authors are accountable for all aspects of the work in ensuring that questions related to the accuracy or integrity of any part of the work are appropriately investigated and resolved. The study was conducted in accordance with the Declaration of Helsinki (as revised in 2013).
Open Access Statement: This is an Open Access article distributed in accordance with the Creative Commons Attribution-NonCommercial-NoDerivs 4.0 International License (CC BY-NC-ND 4.0), which permits the non-commercial replication and distribution of the article with the strict proviso that no changes or edits are made and the original work is properly cited (including links to both the formal publication through the relevant DOI and the license). See: https://creativecommons.org/licenses/by-nc-nd/4.0/.
References
- Siegel RL, Miller KD, Fuchs HE, et al. Cancer statistics, 2022. CA Cancer J Clin 2022;72:7-33. [Crossref] [PubMed]
- Giaquinto AN, Sung H, Miller KD, et al. Breast Cancer Statistics, 2022. CA Cancer J Clin 2022;72:524-41. [Crossref] [PubMed]
- Li X, Xu Y, Zhang L. Serum CA153 as biomarker for cancer and noncancer diseases. Prog Mol Biol Transl Sci 2019;162:265-76. [Crossref] [PubMed]
- Tang S, Zhou F, Sun Y, et al. CEA in breast ductal secretions as a promising biomarker for the diagnosis of breast cancer: a systematic review and meta-analysis. Breast Cancer 2016;23:813-9. [Crossref] [PubMed]
- Chua-On D, Proungvitaya T, Techasen A, et al. High expression of apoptosis-inducing factor, mitochondrion-associated 3 (AIFM3) in human cholangiocarcinoma. Tumour Biol 2016;37:13659-67. [Crossref] [PubMed]
- Banci L, Bertini I, Calderone V, et al. Molecular view of an electron transfer process essential for iron-sulfur protein biogenesis. Proc Natl Acad Sci U S A 2013;110:7136-41. [Crossref] [PubMed]
- Shibayama H, Takai E, Matsumura I, et al. Identification of a cytokine-induced antiapoptotic molecule anamorsin essential for definitive hematopoiesis. J Exp Med 2004;199:581-92. [Crossref] [PubMed]
- Hao Z, Li X, Qiao T, et al. Subcellular localization of CIAPIN1. J Histochem Cytochem 2006;54:1437-44. [Crossref] [PubMed]
- Wang J, Zhou Y, Ma L, et al. CIAPIN1 Targeted NHE1 and ERK1/2 to Suppress NSCLC Cells' Metastasis and Predicted Good Prognosis in NSCLC Patients Receiving Pulmonectomy. Oxid Med Cell Longev 2019;2019:1970818. [Crossref] [PubMed]
- Chen X, Li X, Chen J, et al. Overexpression of CIAPIN1 inhibited pancreatic cancer cell proliferation and was associated with good prognosis in pancreatic cancer. Cancer Gene Ther 2012;19:538-44. [Crossref] [PubMed]
- Lopes LO, Maués JH, Ferreira-Fernandes H, et al. New prognostic markers revealed by RNA-Seq transcriptome analysis after MYC silencing in a metastatic gastric cancer cell line. Oncotarget 2019;10:5768-79. [Crossref] [PubMed]
- Truong SDA, Wongwattanakul M, Proungvitaya T, et al. Prediction of CIAPIN1 (Cytokine-Induced Apoptosis Inhibitor 1) Signaling Pathway and Its Role in Cholangiocarcinoma Metastasis. J Clin Med 2022;11:3826. [Crossref] [PubMed]
- Nymoen DA, Holth A, Hetland Falkenthal TE, et al. CIAPIN1 and ABCA13 are markers of poor survival in metastatic ovarian serous carcinoma. Mol Cancer 2015;14:44. [Crossref] [PubMed]
- Wang J, Li Q, Wang C, et al. Knock-down of CIAPIN1 sensitizes K562 chronic myeloid leukemia cells to Imatinib by regulation of cell cycle and apoptosis-associated members via NF-κB and ERK5 signaling pathway. Biochem Pharmacol 2016;99:132-45. [Crossref] [PubMed]
- Li T, Fu J, Zeng Z, et al. TIMER2.0 for analysis of tumor-infiltrating immune cells. Nucleic Acids Res 2020;48:W509-14. [Crossref] [PubMed]
- Gruosso T, Mieulet V, Cardon M, et al. Chronic oxidative stress promotes H2AX protein degradation and enhances chemosensitivity in breast cancer patients. EMBO Mol Med 2016;8:527-49. [Crossref] [PubMed]
- Maire V, Némati F, Richardson M, et al. Polo-like kinase 1: a potential therapeutic option in combination with conventional chemotherapy for the management of patients with triple-negative breast cancer. Cancer Res 2013;73:813-23. [Crossref] [PubMed]
- Lánczky A, Győrffy B. Web-Based Survival Analysis Tool Tailored for Medical Research (KMplot): Development and Implementation. J Med Internet Res 2021;23:e27633. [Crossref] [PubMed]
- Hall P, Ploner A, Bjöhle J, et al. Hormone-replacement therapy influences gene expression profiles and is associated with breast-cancer prognosis: a cohort study. BMC Med 2006;4:16. [Crossref] [PubMed]
- Ivshina AV, George J, Senko O, et al. Genetic reclassification of histologic grade delineates new clinical subtypes of breast cancer. Cancer Res 2006;66:10292-301. [Crossref] [PubMed]
- Desmedt C, Piette F, Loi S, et al. Strong time dependence of the 76-gene prognostic signature for node-negative breast cancer patients in the TRANSBIG multicenter independent validation series. Clin Cancer Res 2007;13:3207-14. [Crossref] [PubMed]
- Bos PD, Zhang XH, Nadal C, et al. Genes that mediate breast cancer metastasis to the brain. Nature 2009;459:1005-9. [Crossref] [PubMed]
- Liberzon A, Birger C, Thorvaldsdóttir H, et al. The Molecular Signatures Database (MSigDB) hallmark gene set collection. Cell Syst 2015;1:417-25. [Crossref] [PubMed]
- Chandrashekar DS, Karthikeyan SK, Korla PK, et al. UALCAN: An update to the integrated cancer data analysis platform. Neoplasia 2022;25:18-27. [Crossref] [PubMed]
- Modhukur V, Iljasenko T, Metsalu T, et al. MethSurv: a web tool to perform multivariable survival analysis using DNA methylation data. Epigenomics 2018;10:277-88. [Crossref] [PubMed]
- Li X, Jiang P, Li R, et al. Analysis of cuproptosis in hepatocellular carcinoma using multi-omics reveals a comprehensive HCC landscape and the immune patterns of cuproptosis. Front Oncol 2022;12:1009036. [Crossref] [PubMed]
- Zheng J, Xu T, Chen F, et al. MiRNA-195-5p Functions as a Tumor Suppressor and a Predictive of Poor Prognosis in Non-small Cell Lung Cancer by Directly Targeting CIAPIN1. Pathol Oncol Res 2019;25:1181-90. [Crossref] [PubMed]
- Shi H, Zhou Y, Liu H, et al. Expression of CIAPIN1 in human colorectal cancer and its correlation with prognosis. BMC Cancer 2010;10:477. [Crossref] [PubMed]
- Han JH, Heo KS, Myung CS. Cytokine-induced apoptosis inhibitor 1 (CIAPIN1) accelerates vascular remodelling via p53 and JAK2-STAT3 regulation in vascular smooth muscle cells. Br J Pharmacol 2021;178:4533-51. [Crossref] [PubMed]
- Zhang S, Liu Y, Chen J, et al. Autoantibody signature in hepatocellular carcinoma using seromics. J Hematol Oncol 2020;13:85. [Crossref] [PubMed]
- Ciofi-Baffoni S, Nasta V, Banci L. Protein networks in the maturation of human iron-sulfur proteins. Metallomics 2018;10:49-72. [Crossref] [PubMed]
- Lill R, Dutkiewicz R, Freibert SA, et al. The role of mitochondria and the CIA machinery in the maturation of cytosolic and nuclear iron-sulfur proteins. Eur J Cell Biol 2015;94:280-91. [Crossref] [PubMed]
- Lu D, Xiao Z, Wang W, et al. Down regulation of CIAPIN1 reverses multidrug resistance in human breast cancer cells by inhibiting MDR1. Molecules 2012;17:7595-611. [Crossref] [PubMed]
- Huang Z, Su GF, Hu WJ, et al. The study on expression of CIAPIN1 interfering hepatocellular carcinoma cell proliferation and its mechanisms. Eur Rev Med Pharmacol Sci 2017;21:3054-60. [PubMed]
- Henke E, Nandigama R, Ergün S. Extracellular Matrix in the Tumor Microenvironment and Its Impact on Cancer Therapy. Front Mol Biosci 2020;6:160. [Crossref] [PubMed]
- Yang W, Zhang K, Li L, et al. Downregulation of lncRNA ZNF582-AS1 due to DNA hypermethylation promotes clear cell renal cell carcinoma growth and metastasis by regulating the N(6)-methyladenosine modification of MT-RNR1. J Exp Clin Cancer Res 2021;40:92. [Crossref] [PubMed]
- Havel JJ, Chowell D, Chan TA. The evolving landscape of biomarkers for checkpoint inhibitor immunotherapy. Nat Rev Cancer 2019;19:133-50. [Crossref] [PubMed]
- Liu S, Iorgulescu JB, Li S, et al. Spatial maps of T cell receptors and transcriptomes reveal distinct immune niches and interactions in the adaptive immune response. Immunity 2022;55:1940-1952.e5. [Crossref] [PubMed]
- Myers JA, Miller JS. Exploring the NK cell platform for cancer immunotherapy. Nat Rev Clin Oncol 2021;18:85-100. [Crossref] [PubMed]
- Dewan MZ, Terunuma H, Takada M, et al. Role of natural killer cells in hormone-independent rapid tumor formation and spontaneous metastasis of breast cancer cells in vivo. Breast Cancer Res Treat 2007;104:267-75. [Crossref] [PubMed]
- Adams S, Loi S, Toppmeyer D, et al. Pembrolizumab monotherapy for previously untreated, PD-L1-positive, metastatic triple-negative breast cancer: cohort B of the phase II KEYNOTE-086 study. Ann Oncol 2019;30:405-11. [Crossref] [PubMed]
- Guo C, Luo Z, Ismtula D, et al. TIGIT as a Novel Prognostic Marker for Immune Infiltration in Invasive Breast Cancer. Comb Chem High Throughput Screen 2023;26:639-51. [Crossref] [PubMed]
- Zitvogel L, Kroemer G. Targeting PD-1/PD-L1 interactions for cancer immunotherapy. Oncoimmunology 2012;1:1223-5. [Crossref] [PubMed]
- Molfetta R, Zitti B, Lecce M, et al. CD155: A Multi-Functional Molecule in Tumor Progression. Int J Mol Sci 2020;21:922. [Crossref] [PubMed]
- Zhang Q, Bi J, Zheng X, et al. Blockade of the checkpoint receptor TIGIT prevents NK cell exhaustion and elicits potent anti-tumor immunity. Nat Immunol 2018;19:723-32. [Crossref] [PubMed]
- Chauvin JM, Zarour HM. TIGIT in cancer immunotherapy. J Immunother Cancer 2020;8:e000957. [Crossref] [PubMed]
- Hassin O, Oren M. Drugging p53 in cancer: one protein, many targets. Nat Rev Drug Discov 2023;22:127-44. [Crossref] [PubMed]