A novel eight-gene Immune Cell-Associated Predictive Gene model to predict recurrence in triple-negative breast cancer
Highlight box
Key findings
• This study used single cell sequencing to develop and validate a new eight-gene prediction model based on immune cell marker genes to predict relapse in TNBC patients.
What is known and what is new?
• Tumour immune microenvironment has an impact on prognosis.
• This study proposes a novel eight-gene ICAPG model for predicting the recurrence of triple-negative breast cancer.
What is the implication, and what should change now?
• The results of this study can be applied to clinical patients to predict tumour recurrence and guide treatment.
Introduction
Currently, breast carcinoma has become one of the most common cancers among Chinese women and seriously endangers women’s health (1). Triple-negative breast cancer (TNBC) is defined as the deficiency of estrogen or progesterone receptors (ER or PR), and human epidermal growth factor receptor 2 (HER2) expression (2). TNBC breast cancer (BC) accounts for 10–20% of all BC types (3). It occurs mostly in young women and has the most aggressive behaviour of all BC types. In addition, TNBC has a very high rate of recurrence, especially in a few years after implementation of adjuvant chemotherapy (4-6). Because of these characteristics, the prognosis of TNBC is also worse than that of other types (7). Therefore, predicting TNBC recurrence may act as a more important part in guiding treatment and improving patient care.
It is well known that tumour tissue includes more than just tumour cells; the environment in which tumour cells reside is known as the tumour immune microenvironment (TME) (8-10). The components of the TME are diverse and include diverse stromal cells, immune cells, extracellular matrix molecules, and other various cytokines (11). The new-found evidence suggests that the cellular and cell-free elements in the TME can alter tumorigenesis, growth, invasions, metastases, and answer to therapy (12). In the adaptive immune system, CD8+ T cells become the most fatal effectors in the antitumour immunoreaction and are deemed to be the main reasons of antitumour immunity (13). CD8+ tumour-infiltrating lymphocytes (TILs) intercede rejection of tumour by recognizing cancer antigens and immediately eliminating transformed cells. Effector CD8+ T cells in the cancer microenvironment generate IL-2, IL-12, and IFNγ, that improve the cytotoxic ability of CD8+ T cells and guide to specified tumour cell elimination. Rising levels of cytotoxic CD8+ T cells in the cancer microenvironment are related with enhanced antitumour abilities and prognosis in all kinds of tumours. Macrophages infiltrate heavily into malignant tissues and become tumour-associated macrophages (TAMs), which many studies have shown to be closely associated with tumour progression (14). TAM promotes immunosuppression, tumour growth, invasion, and metastasis by interacting with tumour cells. TAMs have two polarized forms, M1 and M2. Numerous studies have shown that M1 TAMs have some antitumour effects, while a higher density of M2 TAMs is closely related with poorer clinical prognoses in various of cancers. B cells have a unique role in antitumour immunity (15). Tumour-infiltrating B lymphocytes (TIBs) can be observed at all phases of tumour progress. TIBs are involved in both humorall and cellular immunity, and B cells have both tumour-promoting and tumour-suppressive roles. Some studies have shown that TIBs restrain tumour growth by producing immunoglobulins, improving T-cell reactions, and directly eliminating tumour cells (15). Other studies have noticed that B cells may make suppressive functions work due to the various immune-suppressive subtypes.
The therapeutic regimen for TNBC is very restricted due to the lack of targets. Homologous recombination deficiency (HRD) can be used as a predictor of BRCA mutations to guide treatment of TNBC. The HRD score is an unweighted numerical sum of deletion of heterozygosity value, telomere allele imbalance (TAI) value, and large segment shift (LST) score. Clinical trials have confirmed that TNBC patients with high HRD scores do better with platinum-containing chemotherapeutic agents and poly (ADP-ribose) polymerase inhibitors (16,17).
For the past few years, the progress of single-cell RNA-seq technology has advanced rapidly, which provides an opportunity to reveal different cell clusters in the TME (18). In this study, scRNA-seq of TNBC was performed to identify three cell clusters associated with immunity and to identify marker genes differentially expressed in immune cells. Next, immune cell-associated predictive genes (ICAPG) were identified in the Fudan University Shanghai Cancer Center (FUSCC) Triple Negative Breast Cancer training group by Cox and least absolute shrinkage and selection operator (LASSO) regression analysis for the prediction of TNBC recurrence. In addition, the accuracy of the prediction model in the validation group was also be confirmed. Finally, immune cell infiltration was investigated in the high-risk and low-risk groups. We present this article in accordance with the TRIPOD reporting checklist (available at https://tcr.amegroups.com/article/view/10.21037/tcr-22-2608/rc).
Methods
Data extraction
Six samples from the Gene Expression Omnibus (GEO) database, 7 clinical samples from the The Cancer Genome Atlas (TCGA) database downloaded from UCSC Xena, and 360 TNBC samples from the FUSCC-TNBC cohort were included in this study. All patients were clearly diagnosed with TNBC. GEO data were used to identify differential genes in immune cells. A portion of the FUSCC-TNBC cohort was used for model development, and another portion of the FUSCC-TNBC as well as TCGA data were used for validation. Single-cell RNA sequencing data of TNBC examples from GSE118389 were obtained from the GEO database and applied to identify differentially expressed genes (DEGs) in TNBC immune cells. RNA-seq and clinical data of TNBC were acquired from the cohort of the TNBC study at the Shanghai Cancer Center of Fudan University (19). This retrospective study used datasets from GEO and TCGA which are publicly available, and data from FUSCC-TNBC. The study was conducted with the approval of the Ethics Committee of Fudan University Cancer Hospital (No. 2019171) and operated in compliance with the Helsinki Declaration (as revised in 2013) (20). Informed consent was obtained from the participants.
Definition of immune cell marker genes by scRNA sequencing
The software packages “Seurat” and “SingleR” were used to analyze the scRNA sequencing data. First, the raw data were filtered for genes expressed in at least 3 single cells, and only cells expressing >50 genes were retained, and only cells expressing less than 5% of mitochondrial genes were retained. Next, the data were normalized by setting the normalization method to “LogNormalize” and using the “FindVariableFeatures” function to determine the top 1,500 highly variable genes. After that, the “RunPCA” function was run to perform principal component (PC) analysis on these 1,500 genes. JackStraw analysis was applied to select 20 PCs with P values <0.05 for cell clustering analysis. Using the “FindNeighbors”, “FindClusters” and “RunTSNE” functions, cell proximity distances were first calculated, and then cell clustering analysis was performed. The “TSNEplot” command was used to output the visual map. Finally, the “FindAllMarkers” command was used to find the differentially expressed genes (DEGs) in each cluster. The modified P value was set to <0.05 and |log2 (fold change)| to >1.
Pathway and functional enrichment analyses
The R package “clusterProfiler” was applied to execute Gene Ontology (GO) and Kyoto Encyclopedia of Genes and Genomes (KEGG) analyses. Annotated P values <0.05 were viewed remarkable enrichment.
Construction and verification of the eight-gene ICAPG model based on immune cell marker genes
First, The FUSCC-TNBC cohort data consisting of 360 samples were randomly divided into two groups in a 1:1 ratio, including the training group and the validation group. A blinded assessment method was used to construct the model. Univariate Cox regression analysis was performed to select genes with P<0.05. Next, LASSO regression was performed for the selected genes to reduce overfitting. Cross-validation was then performed using the “cv.glmnet” function to select the best model. Finally, model construction was performed based on the genes screened using the training set data. Stepwise multivariate Cox regression analysis was applied to identify the genes that best predicted recurrence. In addition, patients were divided into two groups based on median, including a low-risk group and a high-risk group. To validate the predictive power of ICAPG, the Kaplan-Meier method was applied to both the training and validation groups. In addition, the area under the curve (AUC) was planned using the “survROC” package. Finally, patients’ risk values were ranked using the “pHeatmap” software package to plot recurrence risk curves and heat maps.
Construction and verification of the nomogram model
Based on data availability and clinical evidence, a nomogram was constructed to integrate risk scores for eight genes, mRNA isoforms, Ki67, T, N, and surgical modality. The predictive power of the nomogram was assessed by calibration curve and decision curve analysis.
Differential analysis of the infiltration in immune cell
The CIBERSORT and ssGSEA algorithms were used to assess immune cell infiltration. Immune cell infiltration was then compared between patients in the high-risk and low-risk groups and box plots were drawn.
HRD score
DNA was analyzed by applying a recently published next-generation sequencing-based assay to produce genome-wide single nucleotide polymorphism (SNP) profiles from which three elements of HRD values were calculated: TAI, loss of heterozygosity (LOH) and LST. The HRD value was explained as the unweighted sum of the TAI, LOH and LST values: HRD = TAI + LOH + LST.
Statistical analysis
All statistical analyses were done by using R. The chi-squared test and Fisher’s exact test were applied to determine the clinical features of FUSCC-TNBC subjects. The predictive value of ICAPG was investigated using univariate, multivariate Cox regression, and LASSO regression analyses. P<0.05 was deemed statistically significant.
Results
Baseline patient features
Table 1 describes the pathological and clinical features of 360 patients with FUSCC-TNBC. Overall, the mean age at surgery was 53.27 (±11.36) years; the mean tumour size at the time of surgery was 2.5 (interquartile range, 2–3) cm; the follow-up time was 45.6 (interquartile range, 34.82–58.56) months. Most patients (74%) underwent modified radical mastectomy (MRM); 49 of the 360 patients (14%) were diagnosed with recurrence; and the median time of recurrence-free survival (RFS) was 1,359 (interquartile range, 1,031.75–1,768.25) days.
Table 1
Characteristic | Values |
---|---|
Sex | |
Female, n [%] | 360 [100] |
Age at surgery (years), mean ± SD | 53.27±11.36 |
Intrinsic subtype, n [%] | |
Basal | 277 [77] |
Other | 83 [23] |
mRNA subtype, n [%] | |
BLIS | 132 [37] |
IM | 80 [22] |
LAR | 79 [22] |
MES | 69 [19] |
Size (cm), median [interquartile range] | 2.5 [2–3] |
Ki67, median [interquartile range] | 60 [30–70] |
T, n [%] | |
1 | 131 [36] |
2 | 220 [61] |
3 | 9 [3] |
N, n [%] | |
0 | 210 [58] |
1 | 98 [26] |
1mi | 3 [1] |
2 | 32 [9] |
3 | 17 [5] |
Follow-up (months), median [interquartile range] | 45.6 [34.82–58.56] |
RFS status, n [%] | |
0 | 311 [86] |
1 | 49 [14] |
RFS time (days), median [interquartile range] | 1,359 [1,031.75–1,768.25] |
Surgery, n [%] | |
BCS | 5 [1] |
MRM | 265 [74] |
MTX | 88 [24] |
MTX + SLN | 2 [1] |
SD, standard deviation; BLIS, basal-like immune-suppressive; IM, immunomodulatory; LAR, luminal androgen receptor; MES, mesenchymal-like; T, tumour of the Tumour, Node, Metastasis staging system; N, node of the Tumour, Node, Metastasis staging system; RFS, recurrence-free survival; BCS, breast-conserving surgery; MRM, modified radical mastectomy; MTX, mastectomy; SLN, sentinel lymph node.
Identification of immune cell marker genes
An overview of the research design is shown in Figure 1. Based on GSE118389, gene expression profiles of different cells from six major TNBC samples were obtained. First, the top 1,500 genes that were significant were screened (Figure 2A), and the dimensionality using PCA was reduced to identify 20 PCs (Figure 2B). Subsequently, clustering analysis was performed and annotated the genomes, with CD8+ T cells in Group 5, macrophages in Group 7, and B cells in Group 10 (Figure 2C,2D, Table 2). In addition, heatmaps of the distribution of genes in different clusters (Figure 2E) that were identified as marker genes for immune cells in TNBC were drawn (available online: https://cdn.amegroups.cn/static/public/tcr-22-2608-1.xlsx). Enrichment of functionality, such as GO and KEGG analyses, proved that immune cell marker genes are almost entirely associated with immunologic function for example positive regulation of cytokine production, immune response regulation, and signalling pathway mononuclear cells (Figure 3A,3B).
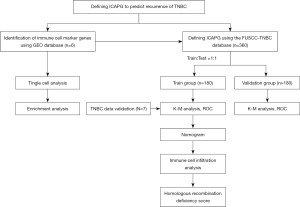
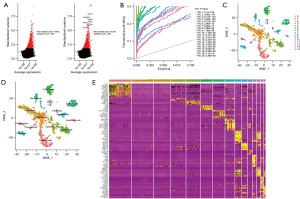
Table 2
Cluster | Cell type |
---|---|
0 | Epithelial cells |
1 | Epithelial cells |
2 | Adipocytes |
3 | Epithelial cells |
4 | Epithelial cells |
5 | CD8+ T-cells |
6 | Epithelial cells |
7 | Macrophages |
8 | Fibroblasts |
9 | Chondrocytes |
10 | B-cells |
11 | Chondrocytes |
12 | Chondrocytes |
13 | Endothelial cells |
14 | Fibroblasts |
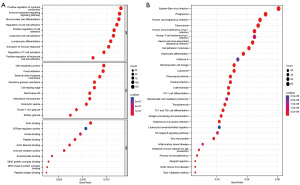
Construction of a novel eight-gene ICAPG model
The RNA sequencing results of immune cell-related marker genes from single cell analysis and FUSCC-TNBC patients were first compared, and 910 differentially-expressed genes were screened. Next, the subjects were divided into two groups by randomization, a training group (n=180) and a validation group (n=1,800). In the training group, we eight genes were selected using Cox and LOSSO regression models, including ASTN2, INSR, MOG, NR4A2, TMEM212, HLA-DRB6, PFKFB3, and RNASE1 (Table 3). The outcome event was patient recurrence. The formula for the genomic risk value was as shown below: genomic risk value =−0.666378645 × ASTN2 + 0.584943064 × INSR + 0.442594812 × MOG + 0.444171212 × NR4A2 − 0.842061141 × TMEM212 − 0.704936712 × HLA-DRB6 + 0.755100196 × PFKFB + 0.360498366 × RNASE1. Figure 4A shows the results of the eight-gene multifactorial Cox analysis. Next, the patients were divided into two groups, high-risk group or low-risk group, in terms of the median. Kaplan-Meier as well as receiver operating characteristic (ROC) curves were used to assess the ICAPG predictive effect (Figure 4B,4C). The AUC at 1-, 3-, and 5-year were 0.777, 0.816, and 0.844 in the training set, respectively. The risk curves and gene expression heatmap also validated the findings (Figure 4D,4E). A list of predictive models is in Table 3.
Table 3
ID | Coef | HR | HR.95L | HR.95H | P value |
---|---|---|---|---|---|
ASTN2 | −0.666378645 | 0.513565016 | 0.193519995 | 1.362903226 | 0.180831058 |
INSR | 0.584943064 | 1.794888789 | 1.106607434 | 2.911263440 | 0.017765136 |
MOG | 0.442594812 | 1.556741434 | 1.002410566 | 2.417616068 | 0.048759999 |
NR4A2 | 0.444171212 | 1.559197416 | 0.936874133 | 2.594902021 | 0.087438823 |
TMEM212 | −0.842061141 | 0.430821623 | 0.191538041 | 0.969036074 | 0.041748840 |
HLA-DRB6 | −0.704936712 | 0.494139846 | 0.220339577 | 1.108172172 | 0.087135142 |
PFKFB3 | 0.755100196 | 2.127824712 | 1.194436889 | 3.790604631 | 0.010375754 |
RNASE1 | 0.360498366 | 1.434043916 | 1.003771347 | 2.048755385 | 0.047631043 |
Coef, coefficient; HR, hazard ratio.
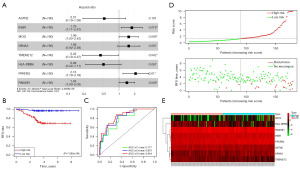
Validation of ICAPG
Patients were also divided from validation group into two subgroups, high-risk and low-risk subgroups, to validate the correctness of the eight-gene model. Figure 5A,5B reveal the Kaplan-Meier curves (P=2.536×10−2 and ROC curves (1-year AUC =0.870, 3-year AUC =0.622, and 5-year AUC =0.609) for the validation group. The risk curves and gene expression heatmaps of the validation group also validated the predictive ability of ICAPG (Figure 5C,5D).
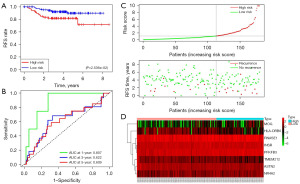
In addition, The TCGA database was used to further verify the model accuracy. Due to the lack of RFS data in the database, only 7 samples were found with relevant records, 2 of which were recurrences. By calculation, we detected that patients with recurrence had higher values and were assigned to the high-risk group (Table S1). Due to the small number of included samples, the Kaplan-Meier analysis, although not statistically significant (P=0.1161), also clearly showed that RFS times were shorter in high-risk patients (Figure S1).
Construction and verification of the nomogram
The eight-gene score was combined with mRNA subtypes, Ki67, T, N, and surgical modalities to construct a line plot of RFS predictions using data from the training group (Figure 6A). Then, the nomogram was validated, and the calibration analysis of the 5-year RFS prediction showed a close fit of the red solid line to the grey dashed line, which indicated that the nomogram had a high prediction accuracy and prediction precision (Figure 6B).
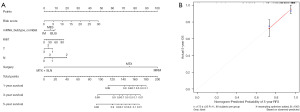
Expression of eight genes in single cells
The GEO single-cell genome sequencing data was used to observe the cellular locations of the eight genes (Figure 7A-7C). The results proved that in addition to their high expression in immune cells, some of the genes were also highly expressed in other cell types. ASTN2, MOG, and TMEM212 were also highly expressed in adipocytes; INSR, HLA-DRB6, and PFKFB3 were also highly expressed in epithelial cells, endothelial cells, and chondrocytes, respectively.
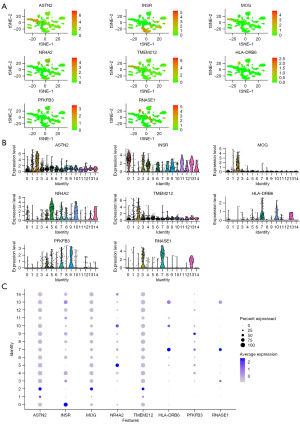
Eight genes are related with infiltration of immune cell in the TME
The relationship between these eight genes and immune cell infiltration in TNBC patients was investigated. The CIBERSORT algorithm was used to assess the degree of infiltration of various types of immune cells in the TME of TNBC patients. The results showed that low-risk subjects had higher levels of CD8+ T-cell infiltration (Figure 8A,8B). CD8+ T cells may exert antitumour effects through specific immunity, which explains why the high-risk group may have a higher risk of recurrence to some extent. Furthermore, the values of the ssGSEA algorithm also proved higher levels of CD8+ T cells and TILs in the low-risk group (Figure 8C).
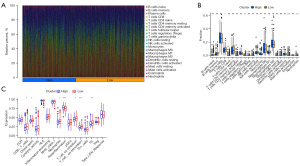
Relationship between HRD score and ICAPG
The HRD scores were also explored in both high-risk and low-risk groups, including LOH, TAI, and LST scores (Figure 9A-9D). The results revealed that the high-risk group showed higher HRD, LOH, and LST scores (P<0.05). Previous studies have confirmed that subjects with high HRD scores have better results with platinum-containing chemotherapy drugs or poly(ADP-ribose) polymerase (PARP) inhibitors. This finding may also guide future drug selection.
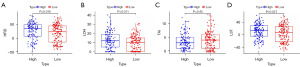
Discussion
We first screened the immune-related marker genes of TNBC using single-cell genome sequencing data from the database of GEO. Subsequently, we defined ICAPG using a training data set of 180 samples from FUSCC-TNBC, which included eight genes, namely, ASTN2, INSR, MOG, NR4A2, TMEM212, HLA-DRB6, PFKFB3, and RNASE1. We then validated the constructed model with data from the validation set. In addition, we tried to combine the ICAPG score with other clinical and pathological characteristics of the patients (including mRNA subtypes, Ki67, T, N, and surgical modality) to estimate the likelihood of recurrence in TNBC patients and drew a nomogram. In addition, we divided the subjects into two groups, low-risk and high-risk groups, according to the median and performed a test to measure the infiltration of immune cell. The results proved that the levels of CD8+ T cells and TILs from high-risk group were lower. Finally, we also explored the relationship between the collected HRD scores and ICAPG and detected that the HDR values from high-risk group were higher, which also predicts that the high-risk group might have more sensitivities to platinum-containing drugs or PARP inhibitors.
Through further investigation, we found that four of the eight genes, ASTN2, INSR, NR4A2, and PFKFB3, were related with tumorigenesis and progression. Wang et al. reported that ASTN2 (astrotactin 2) can be linked to PAPPA antisense to constitute chimeric RNA (21). ASTN2-PAPPA antisense can aggravate human oesophageal cancer tumour progression and metastasis by regulating OCT4. In addition, the chimaera also enhances the stemness of tumour cells. Hu et al. found that polymorphisms of INSR (insulin receptor) are of great importance for sensitivity to chemotherapy in ovarian cancer patients (22). They determined that INSR rs2252673 and rs3745546 polymorphisms were linked with sensitive level to platinum-based chemotherapy in patients with epithelial ovarian cancer through a clinical study. NR4A2 is an element of nuclear receptor family 4 subgroup A. Establishing a coculture model of intrahepatic cholangiocarcinoma cells and hepatic stellate cells, Jing et al. found that HSCs stimulated the expression of NR4A2 (23). NR4A2 acts as a transcription factor to promote tumour proliferation and metastasis and can serve as an independent prognostic index of all the survival in patients with intrahepatic cholangiocarcinoma. Mechanistically, NR4A2 enhances Wnt/β-linked protein signalling activity by upregulating bone bridge protein expression through transcriptional activation. Furthermore, Karki et al. demonstrated that NR4A2 can promote the proliferation, invasion, and migration of glioblastoma tumours (24). Bisindole-derived NR4A2 antagonists could be effective agents for the treatment of glioblastoma. PFKFB3 (6-phosphofructo-2-kinase/fructose-2,6-biphosphatase 3) has been shown to play a role in carcinogenetic effect, cancer cell spread, vascular invasiveness, resistance to drugs, and the tumour microenvironment in breast, pancreatic, gastric, and colon cancers (25).
However, this study has several limitations. Firstly, additional clinical data need to be included to validate the reliability of the prediction model in this research. Secondly, only make predictions at the mRNA level and did not discuss them at the protein level. Future studies could search after the expression as well as prognostic effects of the eight genes on the level of protein. In conclusion, this study is descriptive in nature. The underlying mechanism between ICAPG gene expression and TNBC recurrence can be explored in future studies.
Conclusions
In summary, this study identified TNBC immune cell marker genes using single-cell data. A new eight-gene signature model was defined and validated using the FUSCC-TNBC database and TCGA database, followed by the construction of a nomogram combining mRNA subtypes, Ki67, T, N and surgical approaches to predict RFS. Finally, immune cell infiltration and HRD were assessed. This may have implications for postoperative follow-up time and drug selection for TNBC patients.
Acknowledgments
Funding: The study was sponsored by
Footnote
Reporting Checklist: The authors have completed the TRIPOD reporting checklist. Available at https://tcr.amegroups.com/article/view/10.21037/tcr-22-2608/rc
Data Sharing Statement: Available at https://tcr.amegroups.com/article/view/10.21037/tcr-22-2608/dss
Peer Review File: Available at https://tcr.amegroups.com/article/view/10.21037/tcr-22-2608/prf
Conflicts of Interest: All authors have completed the ICMJE uniform disclosure form (available at https://tcr.amegroups.com/article/view/10.21037/tcr-22-2608/coif). The authors have no conflicts of interest to declare.
Ethical Statement: The authors are accountable for all aspects of the work in ensuring that questions related to the accuracy or integrity of any part of the work are appropriately investigated and resolved. This retrospective study used datasets from GEO and TCGA which are publicly available, and data from FUSCC-TNBC. The study was conducted with the approval of the Ethics Committee of Fudan University Cancer Hospital (No. 2019171) and operated in compliance with the Helsinki Declaration (as revised in 2013). Informed consent was obtained from the participants.
Open Access Statement: This is an Open Access article distributed in accordance with the Creative Commons Attribution-NonCommercial-NoDerivs 4.0 International License (CC BY-NC-ND 4.0), which permits the non-commercial replication and distribution of the article with the strict proviso that no changes or edits are made and the original work is properly cited (including links to both the formal publication through the relevant DOI and the license). See: https://creativecommons.org/licenses/by-nc-nd/4.0/.
References
- Fan L, Strasser-Weippl K, Li JJ, et al. Breast cancer in China. Lancet Oncol 2014;15:e279-89. [Crossref] [PubMed]
- Zaharia M, Gómez H. Triple negative breast cancer: a difficult disease to diagnose and treat. Rev Peru Med Exp Salud Publica 2013;30:649-56. [PubMed]
- Dent R, Trudeau M, Pritchard KI, et al. Triple-negative breast cancer: clinical features and patterns of recurrence. Clin Cancer Res 2007;13:4429-34. [Crossref] [PubMed]
- Malorni L, Shetty PB, De Angelis C, et al. Clinical and biologic features of triple-negative breast cancers in a large cohort of patients with long-term follow-up. Breast Cancer Res Treat 2012;136:795-804. [Crossref] [PubMed]
- Guarneri V, Dieci MV, Conte P. Relapsed triple-negative breast cancer: challenges and treatment strategies. Drugs 2013;73:1257-65. [Crossref] [PubMed]
- Billar JA, Dueck AC, Stucky CC, et al. Triple-negative breast cancers: unique clinical presentations and outcomes. Ann Surg Oncol 2010;17:384-90. [Crossref] [PubMed]
- Carey L, Winer E, Viale G, et al. Triple-negative breast cancer: disease entity or title of convenience? Nat Rev Clin Oncol 2010;7:683-92. [Crossref] [PubMed]
- Balkwill F, Mantovani A. Inflammation and cancer: back to Virchow? Lancet 2001;357:539-45. [Crossref] [PubMed]
- Quail DF, Joyce JA. Microenvironmental regulation of tumor progression and metastasis. Nat Med 2013;19:1423-37. [Crossref] [PubMed]
- Risom T, Glass DR, Averbukh I, et al. Transition to invasive breast cancer is associated with progressive changes in the structure and composition of tumor stroma. Cell 2022;185:299-310.e18. [Crossref] [PubMed]
- Hinshaw DC, Shevde LA. The Tumor Microenvironment Innately Modulates Cancer Progression. Cancer Res 2019;79:4557-66. [Crossref] [PubMed]
- Jin MZ, Jin WL. The updated landscape of tumor microenvironment and drug repurposing. Signal Transduct Target Ther 2020;5:166. [Crossref] [PubMed]
- van der Leun AM, Thommen DS, Schumacher TN. CD8(+) T cell states in human cancer: insights from single-cell analysis. Nat Rev Cancer 2020;20:218-32. [Crossref] [PubMed]
- Komohara Y, Fujiwara Y, Ohnishi K, et al. Tumor-associated macrophages: Potential therapeutic targets for anti-cancer therapy. Adv Drug Deliv Rev 2016;99:180-5. [Crossref] [PubMed]
- Wang SS, Liu W, Ly D, et al. Tumor-infiltrating B cells: their role and application in anti-tumor immunity in lung cancer. Cell Mol Immunol 2019;16:6-18. [Crossref] [PubMed]
- Telli ML, Timms KM, Reid J, et al. Homologous Recombination Deficiency (HRD) Score Predicts Response to Platinum-Containing Neoadjuvant Chemotherapy in Patients with Triple-Negative Breast Cancer. Clin Cancer Res 2016;22:3764-73. [Crossref] [PubMed]
- Cerrato A, Morra F, Celetti A. Use of poly ADP-ribose polymerase [PARP] inhibitors in cancer cells bearing DDR defects: the rationale for their inclusion in the clinic. J Exp Clin Cancer Res 2016;35:179. [Crossref] [PubMed]
- Chen H, Ye F, Guo G. Revolutionizing immunology with single-cell RNA sequencing. Cell Mol Immunol 2019;16:242-9. [Crossref] [PubMed]
- Jiang YZ, Ma D, Suo C, et al. Genomic and Transcriptomic Landscape of Triple-Negative Breast Cancers: Subtypes and Treatment Strategies. Cancer Cell 2019;35:428-40.e5. [Crossref] [PubMed]
- World Medical Association Declaration of Helsinki. ethical principles for medical research involving human subjects. JAMA 2013;310:2191-4. [Crossref] [PubMed]
- Wang L, Xiong X, Yao Z, et al. Chimeric RNA ASTN2-PAPPA(as) aggravates tumor progression and metastasis in human esophageal cancer. Cancer Lett 2021;501:1-11. [Crossref] [PubMed]
- Hu JL, Hu XL, Han Q, et al. INSR gene polymorphisms correlate with sensitivity to platinum-based chemotherapy and prognosis in patients with epithelial ovarian cancer. Gene Ther 2017;24:392-8. [Crossref] [PubMed]
- Jing CY, Fu YP, Zhou C, et al. Hepatic stellate cells promote intrahepatic cholangiocarcinoma progression via NR4A2/osteopontin/Wnt signaling axis. Oncogene 2021;40:2910-22. [Crossref] [PubMed]
- Karki K, Li X, Jin UH, et al. Nuclear receptor 4A2 (NR4A2) is a druggable target for glioblastomas. J Neurooncol 2020;146:25-39. [Crossref] [PubMed]
- Shi L, Pan H, Liu Z, et al. Roles of PFKFB3 in cancer. Signal Transduct Target Ther 2017;2:17044. [Crossref] [PubMed]