Identification and validation of a novel prognostic model for gastric cancer based on m7G-related genes
Highlight box
Key findings
• The established prognostic model consisting of 11 genes has superior efficacy for predicting the prognosis of GC patients.
What is known and what is new?
• The m7G-related genes are known to play important roles in the occurrence and development of GC.
• This study revealed the prognostic value of m7G-related genes in GC, established a prognostic model, and found that the risk model correlated with prognosis and immunotherapy in GC patients.
What is the implication, and what should change now?
• This study highlights the importance of m7G-related genes in clinical practice and implies that m7G-related genes may be a therapeutic priority for GC patients.
Introduction
Gastric cancer (GC) is one of the most common malignant tumors of the digestive system. According to the latest data, GC ranks fifth in incidence and fourth in mortality worldwide (1). Various risk factors exist for the development of GC, including Helicobacter pylori infection, smoking, obesity, high salt intake, and low consumption of fruits and vegetables (2,3). Currently, the treatment of GC comprises a comprehensive treatment model based on surgical resection combined with chemotherapy, immunotherapy, and targeted therapy (4,5). However, the 5-year survival rate for progressive GC remains below 30% after treatment (6). Thus, there is an urgent need to identify novel and precise biomarkers to improve the prognosis and treatment of patients with GC.
RNA methylation refers to post-transcriptional modification on the substrate or the replacement of the original alkylation form of atoms or groups (7). Various forms of RNA methylation exist, depending on the methylation site, including N7-methyladenosine (m7G), N6-methyladenosine (m6A), N1-methyladenosine (m1A), 5-methylcytosine (m5C), and 2-O-dimethyladenosine (m6Am) (8). The dysregulation of RNA methylation is tied to the occurrence and progression of human cancer (9). However, relatively little research has been conducted on m7G in GC. In m7G RNA methylation, a methyl group is added to the 7th N of RNA guanine (G) in the presence of methyltransferase (10). Various studies have identified m7G-related genes in nasopharyngeal carcinoma (11), squamous cell carcinoma (12), and lung adenocarcinoma (13). However, no studies have reported the role of m7G-related genes in GC. Thus, elucidating the role of m7G-related genes in GC and their prognostic value may provide a new strategy for the therapy of GC.
In this study, the gene expression data and related clinical information of GC patients were analyzed through online databases including The Cancer Genome Atlas (TCGA) and Gene Expression Omnibus (GEO). The m7G-related genes were obtained from the differentially expressed genes (DEGs) between 2 clusters which were classified according to the expression level of 29 m7G regulators in GC samples. A prognostic model based on 11 m7G-related genes was constructed and verified. Finally, we analyzed the differences between subgroups based on the risk model in terms of immune cell infiltration, immune function, and immune checkpoints. This study provides great insight for predicting the prognosis and treatment of GC patients. We present this article in accordance with the Transparent Reporting of a multivariable prediction model for Individual Prognosis Or Diagnosis (TRIPOD) reporting checklist (available at https://tcr.amegroups.com/article/view/10.21037/tcr-22-2614/rc).
Methods
Data collection and collation
Transcriptome profiling of gene expression (with 375 tumor samples and 32 normal samples) and clinical data (N=439) for GC specimens were downloaded from TCGA, of which the histological type was stomach adenocarcinoma (STAD). Samples without complete survival information were excluded, and 371 tumor samples with complete survival information were included for subsequent analysis. Moreover, the RNA-sequencing profile data and parallel clinical information of 357 GC samples were extracted from GEO (GSE84433) database to act as an external test cohort. The detailed clinicopathological information of the GC patients in each cohort is displayed in Table 1. A total of 29 m7G regulators were collected from previously published studies (Table S1) (14,15). The study was conducted in accordance with the Declaration of Helsinki (as revised in 2013).
Table 1
Variables | Training group (n=371), n (%) | Testing group A (n=185), n (%) | Testing group B (n=186), n (%) | P value | GEO group (n=357) |
---|---|---|---|---|---|
Age (years) | 0.973 | ||||
≤65 | 163 (43.94) | 81 (43.78) | 82 (44.09) | 241 | |
>65 | 205 (55.26) | 103 (55.68) | 102 (54.84) | 116 | |
Unknown | 3 (0.81) | 1 (0.54) | 2 (1.08) | 0 | |
Gender | 0.969 | ||||
Female | 133 (35.85) | 67 (36.22) | 66 (35.48) | 114 | |
Male | 238 (64.15) | 118 (63.78) | 120 (64.52) | 243 | |
Grade | 0.097 | ||||
G1 | 10 (2.7) | 6 (3.24) | 4 (2.15) | ||
G2 | 134 (36.12) | 76 (41.08) | 58 (31.18) | ||
G3 | 218 (58.76) | 99 (53.51) | 119 (63.98) | ||
Unknown | 9 (2.43) | 4 (2.16) | 5 (2.69) | 357 | |
Tumor invasion (T) | 0.865 | ||||
T1 | 18 (4.85) | 10 (5.41) | 8 (4.3) | 11 | |
T2 | 78 (21.02) | 41 (22.16) | 37 (19.89) | 35 | |
T3 | 167 (45.01) | 80 (43.24) | 87 (46.77) | 67 | |
T4 | 100 (26.95) | 49 (26.49) | 51 (27.42) | 244 | |
Unknown | 8 (2.16) | 5 (2.7) | 3 (1.61) | 0 | |
Lymph node (N) | 0.759 | ||||
N0 | 108 (29.11) | 54 (29.19) | 54 (29.03) | 72 | |
N1 | 97 (26.15) | 46 (24.86) | 51 (27.42) | 154 | |
N2 | 74 (19.95) | 41 (22.16) | 33 (17.74) | 99 | |
N3 | 74 (19.95) | 36 (19.46) | 38 (20.43) | 32 | |
Unknown | 18 (4.85) | 8 (4.32) | 10 (5.38) | 0 | |
Metastasis (M) | 0.987 | ||||
M0 | 328 (88.41) | 164 (88.65) | 164 (88.17) | ||
M1 | 25 (6.74) | 13 (7.03) | 12 (6.45) | ||
Unknown | 18 (4.85) | 8 (4.32) | 10 (5.38) | 357 | |
Tumor stage | 0.569 | ||||
Stage I | 50 (13.48) | 23 (12.43) | 27 (14.52) | ||
Stage II | 111 (29.92) | 61 (32.97) | 50 (26.88) | ||
Stage III | 149 (40.16) | 70 (37.84) | 79 (42.47) | ||
Stage IV | 38 (10.24) | 18 (9.73) | 20 (10.75) | ||
Unknown | 23 (6.2) | 13 (7.03) | 10 (5.38) | 357 |
GEO, gene expression omnibus; GC, gastric cancer.
Cluster analysis according to m7G regulators
The “limma” package in R software (R Foundation for Statistical Computing, Vienna, Austria) was used to obtain the expression of m7G regulators in GC specimens (16). Based on the expression of m7G regulators, consensus analysis was performed using the “ConsensusClusterPlus” package in R. Patients with GC were divided into k (k=2–9) clusters, and then these k clusters were subjected to survival analysis to determine the most appropriate K-value. After determining the most reasonable clustering method, the DEGs between the various aforementioned clusters were retained for the next analysis.
Development and internal validation of the prognostic model
Univariate Cox regression analysis was used to obtain prognostic DEGs. Among them, genes with P<0.05 were included in least absolute shrinkage and selection operator (LASSO) regression analysis. Genes selected by LASSO Cox regression analysis were used to construct a prognostic model and a risk score was calculated for each GC patient.
Risk score = Ʃ (βi × EXPi), and in the formula, βi represented the weight of the corresponding genes and EXPi represented their expression level.
Based on the median risk score of the training cohort, GC patients were assigned to 2 groups, a high-risk group and a low-risk group. Patients in the training cohort were randomly divided into 2 internal test cohorts randomly, at a ratio of 1:1. The GC patients were also divided into high- and low-risk groups. To evaluate whether risk score can independently predict the prognosis of GC patients, multivariate Cox analysis of the risk score was performed with multitudinous clinicopathological features. Kaplan-Meier (KM) analysis was also performed in different clinicopathological subgroups grounded on risk score.
Differential expression and prognostic value of m7G-related genes in the risk model
To verify the differential expression and prognosis of genes used to construct the risk model which had already been screened by LASSO Cox regression, these genes were further analyzed using the Gene Expression Profiling Interactive Analysis (GEPIA) database (17).
External validation of the risk model
To assess whether the prognostic model had similar predictive power in different populations, we subjected it to external validation. The risk score for each GC patient in the external testing cohort was calculated using the above formula, which was also used in training cohort. Similarly, the external queue was divided into high- and low-risk groups which were subjected to KM, scatter plot analyses, ROC curve, and independent prognostic analysis (18,19).
Development of a nomogram
To compare the advantage of risk score and other clinicopathological features in evaluating the prognosis of GC, nomogram was constructed. A calibration chart was developed to appraise the difference between the actual survival probability and the predicted value.
Gene set enrichment analysis of DEGs between high- and low-risk groups
To elucidate biological progresses of the DEGs between the high- and low-risk groups, which were named risk group DEGs (RDEGs), GSEA was performed for each group separately using the “enrichplot” package.
Immunological analysis
To explore the correlation between the risk score and tumor-infiltrating immune cells (TIICs) characteristics, various deconvolution algorithms, including xCell (20), Tumor Immune Estimation Resource (TIMER) (21), quanTIseq (22), MCPcounter (23), Estimating the Proportions of Immune and cancer cells (EPIC) (24), Cell-type Identification by Estimating Relative Subsets of RNA Transcripts (CIBERSORT), and CIBERSORT-ABS (25) were used to calculate the abundances of TIICs in each sample in the training group. A bubble diagram was plotted to demonstrate the association between the risk score and the abundance of TIICs.
Statistical analysis
The software R 4.0.1 used to perform all statistical analyses. Survival data were evaluated using Cox regression, the KM curve, and logarithmic rank test. Statistical significance was considered when P<0.05.
Results
The m7G regulator of tumor classification
The overall work process of the whole study is shown in Figure 1. According to the expression parallelism of the 29 m7G regulators, the consensus clustering method was applied to cluster the STAD samples of the TCGA, with k=2 regarded as a suitable selection with clustering firmness increasing from k=2 to k=9 in the TCGA cohorts, named Cluster 1 and Cluster 2 (Figure 2A-2C). Significant differences in overall survival (OS) were observed between these 2 clusters (P=0.004) (Figure 2D). Thus, we inferred that these results may reveal the potential relevance of m7G regulators to GC patients and subjected them to further exploration.
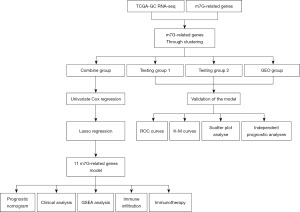
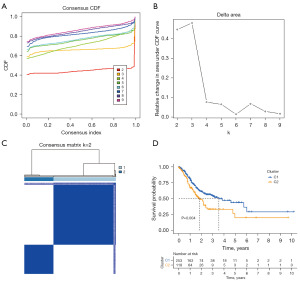
Construction of the prognostic model
Firstly, 928 DEGs (|log2FC| ≥ 0.585 and P<0.05, Table S2) were shown to be linked with the 2 clusters (Figure 3A). Subsequently, 52 genes with significant prognostic value for GC patients were acquired by univariate Cox regression analysis (P<0.01, Figure 3B). Finally, 11 genes (MATN3, GAD1, CYTL1, CD36, SLC7A2, PLCL1, GUCY1A2, GPR173, PCDHB5, GRP, and ST6GALNAC3) were authenticated as independent prognostic elements and selected for the establishment of the prognostic model by LASSO Cox regression (Figure 3C,3D). The formula of the risk score was as follows: risk score = (0.14972*ExpMATN3) + (−0.01235*ExpGAD1) + (0.24836*ExpCYTL1) + (0.00992*ExpCD36) + (0.11964*ExpSLC7A2) + (0.00436*ExpPLCL1) + (0.05246*ExpGUCY1A2) + (0.12356*ExpGPR173) + (0.01976*ExpPCDHB5) + (0.07618*ExpGRP) + (0.00524*ExpST6GALNAC3). The results of TCGA combined with Genotype-Tissue Expression (GTEx) database analysis are shown in the Figure 4. A total of 11 m7G-related genes of the prognostic model were differentially expressed between normal and cancerous tissues, and these genes could predict the prognosis of GC patients independently.
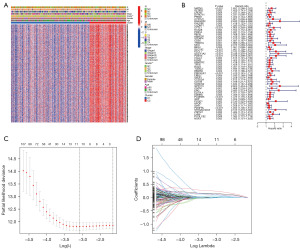
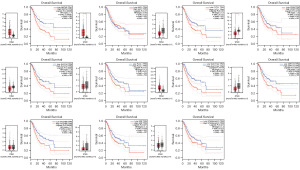
Prognostic assessment and internal validation of the prognostic model
In the training cohort, GC patients were divided into the low- (n=186) and high-risk (n=185) groups based on the mid-value of risk score (mid-value =3.04224). The KM analysis showed that patients in the high-risk group had a worse prognosis than those in the low-risk group (Figure 5A, P<0.001). The risk plot revealed that as the risk score value increased, there was a gradual increase in mortality in GC patients (Figure 5B). The ROC curve indicated that the areas under the curve (AUCs) of 1-, 3-, and 5-year survival were 0.643, 0.680, and 0.733, respectively (Figure 5C). Multivariate Cox analysis revealed that the risk score was an independent parameter of GC patients’ prognosis in the training cohort (Figure 5D, P<0.001). Next, GC patients from the training cohort were randomly allocated to testing cohort A (n=185) and testing cohort B (n=186) to further validate the risk model. GC patients were also divided into high- and low-risk groups in both test groups. In testing cohort A, the prognosis of GC patients in the high-risk group involved poorer OS than that in the low-risk group (Figure 5E, P=0.001). As the risk score value increased, so did the mortality rate of GC patients (Figure 5F). The AUC values of 1-, 3-, and 5-year survival of GC patients were 0.665, 0.691, and 0.780, respectively (Figure 5G). The risk score was an independent predictor of prognosis in GC patients (Figure 5H, P<0.001). The conclusions obtained in testing cohort B were similar to those in testing cohort A and the training cohort. The prognosis of GC patients in the low-risk group involved better OS than that in the high-risk group (Figure 5I, P=0.017). Additionally, the mortality rate of GC patients increased alongside the increase of risk score value (Figure 5J). The AUC values of 1-, 3-, and 5-year survival of GC patients were 0.626, 0.670, and 0.627, respectively (Figure 5K) and the risk score was also an independent predictor of prognosis in GC patients (Figure 5L, P=0.002). All of the above results suggested that the risk score has good efficacy in predicting the prognosis of human GC.
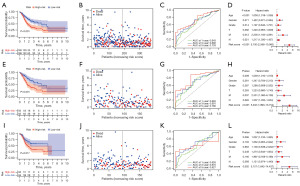
External validation of the prognostic model
Through an independent GEO cohort, we further verified the prognostic ability of the risk model. Similarly, OS analysis in 357 GC patients indicated that patients with high-risk score would have poor survival outcomes (Figure 6A,6B, P=0.003). Correspondingly, the 1-, 3-, and 5-year AUCs were 0.594, 0.603, and 0.617, respectively (Figure 6C). As shown in Figure 6D, the risk score (P=0.024) was an independent predictor of OS for GC patients.
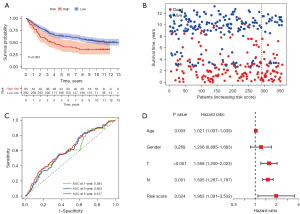
Survival analysis of subgroups of GC patients
To evaluate whether the prognostic model can access the prognosis of GC patients under different clinicopathological variables, GC patients were stratified into 8 subgroups according to different clinicopathological features in the training cohort, including age (>65 and ≤65 years old), gender (female and male), tumor grade (G1–G2 and G3), and tumor-node-metastasis (TNM) stage (stage I–II and III–IV). The results showed that the OS rate of the subdivisions, including age >65 (P=0.036) and age ≤65 years (P<0.001), female (P=0.003) and male (P=0.009), Grade 1–2 (P=0.015), Grade 3 (P=0.003), Stage I–II (P=0.025), and Stage III–IV (P=0.005), were significantly different (Figure 7).
Construction of a nomogram which incorporated clinical features
We integrated age, gender, tumor grade, T stage, N stage, M stage, TNM stage, and risk score to construct a nomogram to predict the OS rate of GC patients at 1-, 3-, and 5-year (Figure 8A). The standard ROC curve revealed that the AUC values corresponding to the nomogram, namely, risk score, age, gender, grade, TNM stage, T stage, M stage, and N stage were 0.733, 0.729, 0.602, 0.579, 0.535, 0.617, 0.538, 0.511, and 0.560, respectively (Figure 8B). The calibration chart demonstrated that the nomogram has good predictive power compared with the ideal model (Figure 8C-8E). The above results revealed that the nomogram has satisfactory accuracy in predicting the outcome of GC patients.
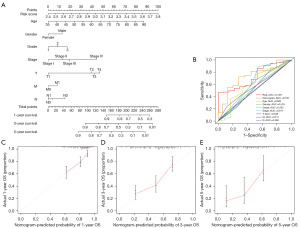
GSEA based on the prognostic model
GSEA of RDEGs (P<0.05 and |log2| ≥1, Table S3) between the high- and low-risk groups was used to determine the difference of biological function. As shown in Figure 9A,9B, these RDEGs in the high-risk group were mainly enriched in “cell adhesion”, “cell junction”, “axon development”, “focal adhesion”, “calcium signaling pathway”, and “muscle contraction”. However, in the low-risk group, these RDEGs were mainly enriched in “cornification”, “keratinization”, “homologous recombination”, and “DNA replication” (Figure 9C,9D).
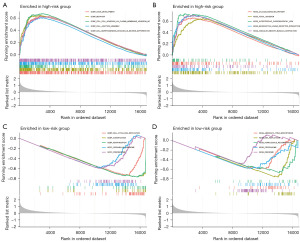
Analysis of immune infiltration and immune checkpoints
To assess the difference in immune cell infiltration between high- and low-risk groups, 7 algorithms were utilized to score the abundance of TIICs. As shown in Figure 10A, Spearman correlation analysis showed that the risk score was positively associated with the infiltration levels of numerous TIICs, including B cell, macrophage, CD8+ T cell, and monocyte. Immune cell subsets and related functions of single sample gene set enrichment analysis (ssGSEA) showed that T cell functions, involving coordination of type I interferon (IFN) response, type II IFN response, MHC class I, and APC-co-inhibition, were distinctively different between low- and high-risk groups (Figure 10B). Furthermore, we explored the expression of immunotherapy markers in low- and high-risk populations. As depicted in Figure 10C-10E, GC patients in the low-risk group expressed higher levels of programmed cell death ligand 1 (PD-L1) compared to the high-risk group. However, the expression levels of programmed cell death ligand 2 (PD-L2) and tumor necrosis factor receptor superfamily member 4 (TNFRSF4) were higher in the high-risk group. Figure 10F shows that the expression of PD-L1 was negatively correlated with risk score, whereas the opposite was true for PD-L2 and TNFRSF4 (Figure 10G,10H). All of the above results indicated that GC is a highly heterogeneous kind of tumor with different responses to different immunosuppressants. As our study showed, the low-risk group was more sensitive to PD-L1 inhibitors, whereas the high-risk group was more sensitive to PD-L2 and TNFRSF4 inhibitors.
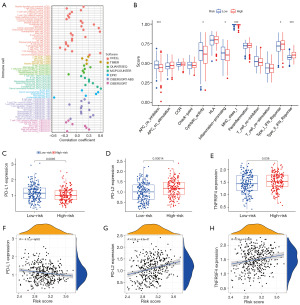
Discussion
GC remains one of the most highly invasive types of malignant tumor with high morbidity and mortality. It is necessary to find reliable biomarkers for novel diagnosis, treatment, and prevention methods of GC. M7G modification is one of the most common base modification forms in post-transcriptional regulation. It is widely distributed in tRNA, rRNA, and the 5 'cap region of eukaryotic mRNA and plays an important role in maintaining RNA processing and metabolism, stability, nucleation, and protein translation (26). Recently, some studies have reported the prognostic value of m7G-related genes in hepatocellular carcinoma (27), prostate cancer (28), and glioma (29), but the roles of m7G-related genes in GC have never been reported.
Our study comprehensively analyzed the clustering effect of m7G-related genes in GC patients according to the expression levels of 29 m7G regulators. The prognosis of GC patients in the 2 groups differed significantly. A risk model consisting of 11 m7G-related genes was established by univariate and LASSO Cox regression analysis based on the differences between the 2 groups and validated in an independent GEO dataset for its accuracy in predicting OS. We utilized the risk model to classify patients in the TCGA and GEO into low- and high-risk groups. The high-risk group had a poorer prognosis in all cohorts. Transient ROC curve analysis confirmed the accuracy and robustness of the prognostic model in TCGA and GEO data sets. Multivariate Cox regression analysis further confirmed that risk score could be used as an independent predictor for the prognosis of GC patients.
It has previously been reported that some of the m7G-related genes contained in this signature play important roles in the carcinogenesis of various cancer types. CD36 is a highly glycosylated 88-kD class B clearance receptor, and it is expressed on the surface of various innate and adaptive immune cells, including monocytes, macrophages, and dendritic cells (DCs). Furthermore, it is also expressed in kinds of tumor cells, such as breast cancer, kidney cancer, glioma, and melanoma and is enriched in the stem cells of these cancers (30). In GC, CD36 can provide a critical source of energy for membrane biosynthesis during rapid proliferation of GC cells by regulating fatty acid uptake (31). GPR173 is a member of the super-conserved receptors expressed in the brain (SREB), which is closely related to schizophrenia and autism (32). A previous study showed that the promoter-associated CpG island of ST6GALNAC3 is significantly hypermethylated in prostate cancer and may act as a relevant biomarker for prostate cancer prognosis (33). MATN3 was shown to be involved in the development of GC by regulating epithelial-mesenchymal transition (EMT), suggesting the important role of MATN3 in GC (34). Wu et al. also demonstrated by immunohistochemistry that compared to normal tissues, MATN3 was overexpressed in GC tissues and that it can accurately predict the prognosis of GC patients (35). A previous study showed that CYTL1 is a cytokine with tumor suppressor properties that inhibits tumor metastasis in multiple tumor types such as lung cancer and breast cancer (36).
On the basis of the prognostic model we constructed, the GC cohort was divided into 2 groups called the high- and low- risk group, and the RDEGs between these groups were identified. GSEA was used to demonstrate the reasons for the significant prognostic differences between the 2 groups. Recent studies have shown that focal adhesion is a complex biological process regulated by focal adhesion kinase (FAK) and that it is involved in the regulation of the tumor microenvironment and immune response affecting tumor progression (37,38). FAK is overexpressed in many cancers and promotes cancer progression by regulating cellular processes such as cell survival, proliferation, apoptosis, and migration (39). The results of the GSEA in our study also showed that RDEGs were predominantly enriched in “cell adhesion”, “cell junction”, and “focal adhesion” in the high-risk group.
Immunotherapy occupies the most important position in the treatment of various cancers, and research on immunotherapy has been ongoing (40,41). However, immunotherapy is only recommended as a second- or third-line treatment option for GC in various clinical guidelines. For example, the 2019 European Society for Medical Oncology (ESMO) guidelines recommend programmed cell death protein 1 (PD-1)/PD-L1 antibody therapy as a third-line treatment option for advanced GC (42). The 2018 Japanese Gastric Cancer Association (JGCA) guidelines recommend PD-1 monotherapy as third-line treatment for advanced GC (43). This may be due to the high heterogeneity of stomach cancer, which complicates its immunotherapy mechanisms (44). In addition, there are numerous factors that can affect the effectiveness of immunotherapy for GC. Published studies have shown that Helicobacter pylori infection reduces the susceptibility of PD-1/PD-L1 blockade therapies for GC (45). In conclusion, the effect of PD-1/PD-L1 blockade therapies for GC is influenced by many factors of the tumor itself and external factors. We analyzed the expression of 3 immune checkpoints in high- and low-risk groups, the findings of which may provide some new methods for immunotherapy of GC.
To date, studies of m7G-related genes in GC are still quite insufficient. The advantage of this study is that 11 m7G-related genes in GC prognosis were identified. Subsequently, a prognostic model was constructed and validated. In addition, together with the TCGA and GTEx databases, we once again verified the differential expression and prognosis of these 11 m7G-related genes in normal tissues and tumor tissues. However, our study still had some limitations. All the data in this study were obtained from online public databases. We did not further examine the underlying mechanism of these genes through basic experiments, which may be conducted in future studies.
Conclusions
A prognostic model involving 11 m7G-related genes was constructed which could accurately predict the prognosis of GC patients. This study also disclosed associations between immune function and m7G-related genes.
Acknowledgments
Funding: None.
Footnote
Reporting Checklist: The authors have completed the TRIPOD reporting checklist. Available at https://tcr.amegroups.com/article/view/10.21037/tcr-22-2614/rc
Peer Review File: Available at https://tcr.amegroups.com/article/view/10.21037/tcr-22-2614/prf
Conflicts of Interest: All authors have completed the ICMJE uniform disclosure form (available at https://tcr.amegroups.com/article/view/10.21037/tcr-22-2614/coif). The authors have no conflicts of interest to declare.
Ethical Statement: The authors are accountable for all aspects of the work in ensuring that questions related to the accuracy or integrity of any part of the work are appropriately investigated and resolved. The study was conducted in accordance with the Declaration of Helsinki (as revised in 2013).
Open Access Statement: This is an Open Access article distributed in accordance with the Creative Commons Attribution-NonCommercial-NoDerivs 4.0 International License (CC BY-NC-ND 4.0), which permits the non-commercial replication and distribution of the article with the strict proviso that no changes or edits are made and the original work is properly cited (including links to both the formal publication through the relevant DOI and the license). See: https://creativecommons.org/licenses/by-nc-nd/4.0/.
References
- Sung H, Ferlay J, Siegel RL, et al. Global Cancer Statistics 2020: GLOBOCAN Estimates of Incidence and Mortality Worldwide for 36 Cancers in 185 Countries. CA Cancer J Clin 2021;71:209-49. [Crossref] [PubMed]
- Yuan C, Adeloye D, Luk TT, et al. The global prevalence of and factors associated with Helicobacter pylori infection in children: a systematic review and meta-analysis. Lancet Child Adolesc Health 2022;6:185-94. [Crossref] [PubMed]
- Wu B, Yang D, Yang S, et al. Dietary Salt Intake and Gastric Cancer Risk: A Systematic Review and Meta-Analysis. Front Nutr 2021;8:801228. [Crossref] [PubMed]
- Tokunaga M, Sato Y, Nakagawa M, et al. Perioperative chemotherapy for locally advanced gastric cancer in Japan: current and future perspectives. Surg Today 2020;50:30-7. [Crossref] [PubMed]
- Sugawara K, Kawaguchi Y, Seto Y, et al. Multidisciplinary treatment strategy for locally advanced gastric cancer: A systematic review. Surg Oncol 2021;38:101599. [Crossref] [PubMed]
- Petrillo A, Smyth EC. Multimodality treatment for localized gastric cancer: state of the art and new insights. Curr Opin Oncol 2020;32:347-55. [Crossref] [PubMed]
- Chi HC, Tsai CY, Tsai MM, et al. Impact of DNA and RNA Methylation on Radiobiology and Cancer Progression. Int J Mol Sci 2018;19:555. [Crossref] [PubMed]
- Xie S, Chen W, Chen K, et al. Emerging roles of RNA methylation in gastrointestinal cancers. Cancer Cell Int 2020;20:585. [Crossref] [PubMed]
- He R, Man C, Huang J, et al. Identification of RNA Methylation-Related lncRNAs Signature for Predicting Hot and Cold Tumors and Prognosis in Colon Cancer. Front Genet 2022;13:870945. [Crossref] [PubMed]
- Enroth C, Poulsen LD, Iversen S, et al. Detection of internal N7-methylguanosine (m7G) RNA modifications by mutational profiling sequencing. Nucleic Acids Res 2019;47:e126. [Crossref] [PubMed]
- Chen B, Jiang W, Huang Y, et al. N(7)-methylguanosine tRNA modification promotes tumorigenesis and chemoresistance through WNT/beta-catenin pathway in nasopharyngeal carcinoma. Oncogene 2022;41:2239-53. [Crossref] [PubMed]
- Chen J, Li K, Chen J, et al. Aberrant translation regulated by METTL1/WDR4-mediated tRNA N7-methylguanosine modification drives head and neck squamous cell carcinoma progression. Cancer Commun (Lond) 2022;42:223-44. [Crossref] [PubMed]
- Ma J, Han H, Huang Y, et al. METTL1/WDR4-mediated m(7)G tRNA modifications and m(7)G codon usage promote mRNA translation and lung cancer progression. Mol Ther 2021;29:3422-35. [Crossref] [PubMed]
- Tomikawa C. 7-Methylguanosine Modifications in Transfer RNA (tRNA). Int J Mol Sci 2018;19:4080. [Crossref] [PubMed]
- Zhang W, Zhang S, Wang Z. Prognostic value of 12 m7G methylation-related miRNA markers and their correlation with immune infiltration in breast cancer. Front Oncol 2022;12:929363. [Crossref] [PubMed]
- Ritchie ME, Phipson B, Wu D, et al. limma powers differential expression analyses for RNA-sequencing and microarray studies. Nucleic Acids Res 2015;43:e47. [Crossref] [PubMed]
- Tang Z, Li C, Kang B, et al. GEPIA: a web server for cancer and normal gene expression profiling and interactive analyses. Nucleic Acids Res 2017;45:W98-W102. [Crossref] [PubMed]
- Mandrekar JN. Receiver operating characteristic curve in diagnostic test assessment. J Thorac Oncol 2010;5:1315-6. [Crossref] [PubMed]
- Rizvi AA, Karaesmen E, Morgan M, et al. gwasurvivr: an R package for genome-wide survival analysis. Bioinformatics 2019;35:1968-70. [Crossref] [PubMed]
- Aran D, Hu Z, Butte AJ. xCell: digitally portraying the tissue cellular heterogeneity landscape. Genome Biol 2017;18:220. [Crossref] [PubMed]
- Li T, Fu J, Zeng Z, et al. TIMER2.0 for analysis of tumor-infiltrating immune cells. Nucleic Acids Res 2020;48:W509-14. [Crossref] [PubMed]
- Finotello F, Mayer C, Plattner C, et al. Molecular and pharmacological modulators of the tumor immune contexture revealed by deconvolution of RNA-seq data. Genome Med 2019;11:34. [Crossref] [PubMed]
- Dienstmann R, Villacampa G, Sveen A, et al. Relative contribution of clinicopathological variables, genomic markers, transcriptomic subtyping and microenvironment features for outcome prediction in stage II/III colorectal cancer. Ann Oncol 2019;30:1622-9. [Crossref] [PubMed]
- Racle J, de Jonge K, Baumgaertner P, et al. Simultaneous enumeration of cancer and immune cell types from bulk tumor gene expression data. Elife 2017;6:e26476. [Crossref] [PubMed]
- Chen B, Khodadoust MS, Liu CL, et al. Profiling Tumor Infiltrating Immune Cells with CIBERSORT. Methods Mol Biol 2018;1711:243-59. [Crossref] [PubMed]
- Wu X, Wei Z, Chen K, et al. m6Acomet: large-scale functional prediction of individual m(6)A RNA methylation sites from an RNA co-methylation network. BMC Bioinformatics 2019;20:223. [Crossref] [PubMed]
- Li XY, Zhao ZJ, Wang JB, et al. m7G Methylation-Related Genes as Biomarkers for Predicting Overall Survival Outcomes for Hepatocellular Carcinoma. Front Bioeng Biotechnol 2022;10:849756. [Crossref] [PubMed]
- Mei W, Jia X, Xin S, et al. A N(7)-Methylguanine-Related Gene Signature Applicable for the Prognosis and Microenvironment of Prostate Cancer. J Oncol 2022;2022:8604216. [Crossref] [PubMed]
- Chen Z, Zhang Z, Ding W, et al. Expression and Potential Biomarkers of Regulators for M7G RNA Modification in Gliomas. Front Neurol 2022;13:886246. [Crossref] [PubMed]
- Chen Y, Zhang J, Cui W, et al. CD36, a signaling receptor and fatty acid transporter that regulates immune cell metabolism and fate. J Exp Med 2022;219:e20211314. [Crossref] [PubMed]
- Li C, Zhang L, Qiu Z, et al. Key Molecules of Fatty Acid Metabolism in Gastric Cancer. Biomolecules 2022;12:706. [Crossref] [PubMed]
- Bayrak A, Hanson J, Laufer S, et al. Super-conserved receptors expressed in the brain: biology and medicinal chemistry efforts. Future Med Chem 2022;14:899-913. [Crossref] [PubMed]
- Haldrup C, Pedersen AL, Øgaard N, et al. Biomarker potential of ST6GALNAC3 and ZNF660 promoter hypermethylation in prostate cancer tissue and liquid biopsies. Mol Oncol 2018;12:545-60. [Crossref] [PubMed]
- Dai W, Xiao Y, Tang W, et al. Identification of an EMT-Related Gene Signature for Predicting Overall Survival in Gastric Cancer. Front Genet 2021;12:661306. [Crossref] [PubMed]
- Wu PL, He YF, Yao HH, et al. Martrilin-3 (MATN3) Overexpression in Gastric Adenocarcinoma and its Prognostic Significance. Med Sci Monit 2018;24:348-55. [Crossref] [PubMed]
- Wang X, Li T, Cheng Y, et al. CYTL1 inhibits tumor metastasis with decreasing STAT3 phosphorylation. Oncoimmunology 2019;8:e1577126. [Crossref] [PubMed]
- Pomella S, Cassandri M, Braghini MR, et al. New Insights on the Nuclear Functions and Targeting of FAK in Cancer. Int J Mol Sci 2022;23:1998. [Crossref] [PubMed]
- Lenzo FL, Cance WG. From tumorigenesis to microenvironment and immunoregulation: the many faces of focal adhesion kinase and challenges associated with targeting this elusive protein. Transl Cancer Res 2017;6:S957-60. [Crossref] [PubMed]
- Chuang HH, Zhen YY, Tsai YC, et al. FAK in Cancer: From Mechanisms to Therapeutic Strategies. Int J Mol Sci 2022;23:1726. [Crossref] [PubMed]
- Lee JB, Kim HR, Ha SJ. Immune Checkpoint Inhibitors in 10 Years: Contribution of Basic Research and Clinical Application in Cancer Immunotherapy. Immune Netw 2022;22:e2. [Crossref] [PubMed]
- Lin EM, Gong J, Klempner SJ, et al. Advances in immuno-oncology biomarkers for gastroesophageal cancer: Programmed death ligand 1, microsatellite instability, and beyond. World J Gastroenterol 2018;24:2686-97. [Crossref] [PubMed]
- Muro K, Van Cutsem E, Narita Y, et al. Pan-Asian adapted ESMO Clinical Practice Guidelines for the management of patients with metastatic gastric cancer: a JSMO-ESMO initiative endorsed by CSCO, KSMO, MOS, SSO and TOS. Ann Oncol 2019;30:19-33. [Crossref] [PubMed]
- Japanese gastric cancer treatment guidelines 2018 (5th edition). Gastric Cancer 2021;24:1-21.
- Alsaab HO, Sau S, Alzhrani R, et al. PD-1 and PD-L1 Checkpoint Signaling Inhibition for Cancer Immunotherapy: Mechanism, Combinations, and Clinical Outcome. Front Pharmacol 2017;8:561. [Crossref] [PubMed]
- Shi Y, Zheng H, Wang M, et al. Influence of Helicobacter pylori infection on PD-1/PD-L1 blockade therapy needs more attention. Helicobacter 2022;27:e12878. [Crossref] [PubMed]