Lipid metabolism-related gene signatures for predicting the prognosis of lung adenocarcinoma
Highlight box
Key findings
• A new prognostic model related to lipid metabolism was constructed.
What is known and what is new?
• In previous studies, it was generally found that LDHA, ACSS3, ESYT3, CFTR, CYP17A1 were differentially expressed in various types of cancer. At present, there is no comprehensive screening and systematic evaluation of LMDG in LUAD.
• This study screened the differentially expressed genes of lipid metabolism (LMDG), revealing its prognostic value and clinical and pathological parameters. In addition, a risk model involving LMDG is constructed using the minimum absolute contraction and selection operator (LASSO).
What is the implication, and what should change now?
• This study provides a risk model for prognostic evaluation and identifies potential therapeutic targets for LUAD.
• Additional clinical data are needed to validate the model. In addition, the interaction mechanism between LMDG and downstream molecules needs to be further explored.
Introduction
Background
Lung cancer is a very common malignancy worldwide. According to World Health Organization (WHO) statistics, there were 4.57 million new cancer cases in China in 2020, including 820,000 lung cancer cases. Out of 3 million cancer deaths, a total of 710,000 lung cancer patients died in 2020 (1), thus, making lung cancer rank first in both new cases and mortality rates in China. Lung cancer is subdivided into two types: small cell lung cancer (SCLC) and non-small cell lung cancer (NSCLC); however, the latter occupies 80–85% of the entire lung cancer cases (2), and has a poor prognosis. Lung adenocarcinoma (LUAD) represents a major NSCLC subtype. LUAD mainly originated from bronchial mucosa epithelium, and a few from mucous glands of large bronchi. It is the most common type of lung cancer among non-smokers, more common in women than in men, and more likely to occur in young people than other types of lung cancer. It takes ≥10 years for lung cancer to progress through different stages. Often, the symptoms of physical discomfort, such as cough and fatigue, are ignored and are considered symptoms of other diseases. Therefore, detection of early stages of lung cancer becomes difficult as undetected I/II stages often develop into full-blown lung cancer cases. If diagnosed early, the initial stages can be surgically treated. Hence, early detection and treatment of cancer are recommended for a good prognosis since it is difficult to treat lung cancer in its advanced stages.
Lipids, along with nucleic acids and proteins, are the essential structural units that form cells and help in biofilm formation. Since lipids are important for cellular metabolism and storage, they exert a critical influence on signal transduction in various cell processes. Regulating lipid metabolism processes, like lipid absorption, biosynthesis, and hydrolysis, are critical for maintaining homeostasis. During tumor progression, nutrient availability in the tumor microenvironment (TME) constantly changes; thus, cancer cells maintain their growth, invasion, migration, metastasis, and survival through lipid metabolism.
Rationale and knowledge gap
An elevated rate of lipid synthesis contributes to fast tumor cell growth as well as fulfilling their increased energy demands. Furthermore, critical genes related to lipid generation like fatty acid synthase (FAS), acetyl-CoA carboxylase (ACC), ATP citrate lyase (ACLY), and a transcription factor (TF) Sterol regulatory element-binding protein 1 (SREBP1), modulate gene expressions and can inhibit carcinogenesis; moreover, cancer cell proliferation can be efficiently suppressed by chemical inhibitors or gene knockouts (3). Another lipid metabolism enzyme related to cancer progression is Stearoyl-CoA Desaturase-1 (SCD1), which converts saturated fatty acids (SFA) into monounsaturated fatty acids (MUFA). SCD1 inhibition in lung cancer cells markedly suppresses the in-vitro tumor cell growth, invasion, survival, and in-vivo tumorigenicity (4). Moreover, SCD1 inhibition occurs by the reduction of MUFA content to prevent the G1/S phase cell cycle progression while inducing apoptosis (4), thus, exerting a critical effect on lung carcinogenesis (5). It has also been demonstrated that upregulated SCD1 expression may promote cancer development by either activating the AKT signaling pathway or inhibiting AMPK and GSK3 pathways, thereby promoting downstream β-catenin activity and activating tumor growth signals in other tumors like prostate cancer.
Objective
Lipid metabolism can be a useful prognostic marker for several malignant tumors (6-8). However, various lipid metabolism changes and their potential to become biomarkers related to the survival and treatment of LUAD require further exploration.
Based on clinical data of The Cancer Genome Atlas (TCGA)-derived LUAD cases, the present study constructed one lipid metabolism-related prognosis prediction model and verified it internally as well as externally. Additionally, functional enrichment and TME analyses were also performed to explore its working mechanism, which can be used later for providing personalized treatment and improving patient survival. We present this manuscript in accordance with the TRIPOD reporting checklist (available at https://tcr.amegroups.com/article/view/10.21037/tcr-23-375/rc).
Methods
Data extraction and differentially expressed genes (DEGs)
The clinical characteristics and RNA-seq data of TCGA-LUAD cases were provided by the University of California Santa Cruz (UCSC) Xena website (https://xena.ucsc.edu) and were log2-transformed as well as normalized to select DEGs. Out of the total 5,395 differential LUAD genes meeting the threshold values: |logFC| >1 and P<0.05, 2022 DEGs with logFC value >1 and 3,373 genes were divided into up- and down-regulated gene expression groups, respectively.
Based on Molecular Signatures Database (MSigDB) and Gene Set Enrichment Analysis (GSEA, https://www.gsea-msigdb.org/gsea) database, we searched for lipid metabolism-related genes (LMRGs) with the keyword “datasets with lipid metabolism”. Simultaneously, LMRGs were extracted from the Gene Card and repetitive GSEA genes were deleted; these genes were later intersected with LUAD DEGs to obtain lipid metabolism-related DEGs (LMDGs). The study was conducted in accordance with the Declaration of Helsinki (as revised in 2013).
DEGs and functional analyses
The identified LMDGs were subjected to a differential analysis with the edgeR Bioconductor package (https://bioconductor.org/packages/release/bioc/) in the TCGA cohort, followed by volcano plot visualization. DEGs were identified at the thresholds of P<0.05 and |logFC| >1. Furthermore, DEGs were intersected with lipid metabolism genes to obtain LMDGs in subsequent analyses. A Venn diagram visualized overlapping datasets.
Gene expression RNA sequence profiles obtained at the UCSC Xena website (https://xena.ucsc.edu) and Gene Expression Omnibus (GEO) datasets (GSE30219 and GSE72094) were designated as training and validation cohorts, respectively.
Gene functional enrichment represents an essential process that translates high-throughput-derived molecular results for obtaining biological significance. Statistical analysis and visualization of LMDG functions were carried out with the R software cluster Profiler package by Gene Ontology (GO) as well as the Kyoto Encyclopedia of Genes and Genomes (KEGG) analysis. Moreover, P<0.05 denoted statistical difference.
Prognosis prediction model establishment
LMDGs underwent univariate Cox proportional hazards regression for selecting significant overall survival (OS)-related genes from the TCGA training cohort. Thereafter, genes obtained were analyzed by LASSO Cox regression. Afterward, LMDGs were incorporated into the multivariate model. A risk score was determined by genes that had nonzero coefficients. Therefore, the patient risk scores were determined as: risk score =gene1 expression* j1 + gene2 expression* j2 + … + genex expression*jx, with j representing the coefficient. Thereafter, TCGA-LUAD cases were classified as high- or low-risk groups based on the median risk score. There are 248 patients at high risk and 249 patients at low risk. For both GEO validation cohorts, the above formula and threshold were used.
We used univariate and multivariate regression analyses together to analyze if the LMDG-based prognosis prediction model independently predicted patient prognosis. Kaplan-Meier (KM) survival analysis was utilized to analyze the patient survival, while the group differences were analyzed by a log-rank test. The ROC curve was later plotted to analyze the sensitivity and specificity of our prognosis model. The model discrimination was determined based on the AUC values.
Nomogram establishment and verification
We built a prognosis prediction nomogram for assessing the 3-and 5-year survival probabilities of LUAD cases using the rms R package. Various independent parameters were also included like age, gender, pathological stage, tumor node metastasis classification (TNM) staging, and risk scores. Nomogram discrimination and calibration were determined by C-index and calibration curves relative to the actual survival, respectively.
Prediction of chemotherapy drug response
Additionally, the chemotherapeutic responses were predicted in LUAD cases according to the Genomics of Drug Sensitivity in Cancer (GDSC) database. We chose nine common drugs, including cisplatin, docetaxel, doxorubicin, erlotinib, etoposide, gemcitabine, paclitaxel, rapamycin, and vinorelbine. Our results were calculated with a pRRophetic R package along with the determination of the drug’s half-maximal inhibitory concentration (IC50) values.
Statistical analysis
Wilcoxon rank-sum test was utilized to select DEGs, while Fisher’s exact test was utilized for comparing variables. Prognosis-associated genes were identified through univariate OS Cox regression analysis.
KM analysis and the log-rank test were conducted to compare patient survival between different groups. Spearman’s correlation analysis was carried out to analyze the relationship between the prediction model’s risk and immune scores, respectively. R software (version 4.0.0, R-project.org), along with associated packages, was used for statistical analysis. Furthermore, P≤0.05 denoted statistical difference.
Results
Identification of LMDGs within LUADs
TCGA-LUAD data were obtained from the UCSC Xena website after performing the Wilcoxon rank-sum test with threshold values of P<0.05 and Log2FC <−1 or >1. We obtained 5,395 differential genes from LUAD and adjacent normal tissues. Additionally, 1,133 LMRG genes were found from the GESA and Gene Card databases, of which 273 were differential genes in TCGA-LUAD DEGs (Table S1). Moreover, 117 and 156 genes showed down- and up-regulation, which were observed from the volcano plot and heatmap, respectively (Figure 1A,1B). Lastly, the intersection of LUAD-DEGs with LMRGs was represented by the Venn diagram (Figure 1C).
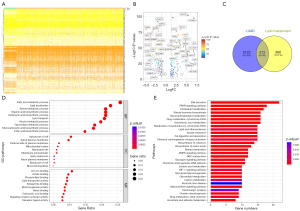
Functional analysis of LMDGs
For exploring LMDG functions, we carried out GO and KEGG enrichment analyses. Based on GO enrichment, LMDGs were correlated with fatty acid metabolism, organic acid biosynthesis, and glycerol lipid metabolism (Figure 1D). A subsequent KEGG enrichment analysis discovered that LMDGs were significantly responsible for the peroxisome proliferator-activated receptor (PPAR) pathway and glycerophospholipid metabolism (Figure 1E).
Prognosis prediction nomogram based on LMDGs
Univariate Cox regression analysis detected 83 LMDGs significantly associated with OS (Figure 2A). Figure 2B,2C displays a differential analysis of LMDGs in LUAD cases compared with the healthy controls (normal people without lung cancer). To avoid model overfitting, we conducted LASSO regression analysis (Figure 2D,2E) which identified 11 genes (LDHA, SEC14L3, LPL, ACSS3, GAPDH, ESYT3, SEC14L4, CYP17A1, CFTR, SLC16A1, and CIDEC) from risk score equation: Risk score = (LDHA * 0.028984915874997 − SEC14L3 × 0.261183701168222 − LPL * 0.0337878152386764 − ACSS3 × 0.178280741516102 + GAPDH * 0.128193589061992 − ESYT3 × 0.0811858233929162 − SEC14L4 × 0.13673431029615 − CYP17A1 × 0.16678096110078 − CFTR * 0.0334880885905273 + SLC16A1 × 0.00307791690707033 + CIDEC * 0.0685768082639508).
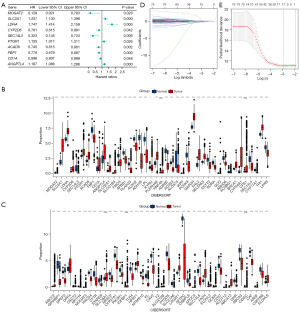
LMDG-based signature’s prognostic value
TCGA-derived cases were divided into high-or low-risk groups based on the median risk score (Figure 3A,3B). Figure 3C displays additional details of 11 genes in both groups. A time-dependent ROC curve analysis was conducted to analyze our signature performance, with AUCs of 0.668, 0.678, and 0.722 for predicting 1-, 3-, and 5-year OS, respectively (Figure 3D). Additionally, all high-risk cases displayed poorer OS (Figure 3E, P<0.05), suggesting that our 11 genes-based risk model was highly accurate in predicting OS for LUADs.
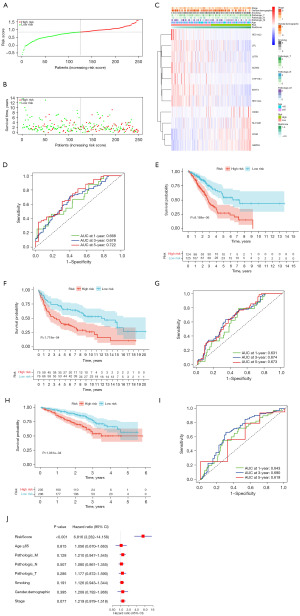
Stability of LMDG-based risk score signature
For checking our LMDG-based signature’s performance, we classified validation cohorts (GSE30219 and GSE72094) as low- or high-risk groups based on the threshold and risk score formula in the TCGA cohort (Figure 3). Based on survival analysis (Figure 3F,3H), all high-risk cases depicted poorer OS compared with the low-risk cases of GSE30219 (P=0.0001718) and GSE72094 (P=0.0001061). For GSE30219, the AUCs for predicting the OS at 1, 3, and 5 years were 0.631, 0.674, and 0.673, respectively (Figure 3G). For the GSE72094 cohort, AUCs were 0.643, 0.690, and 0.619, respectively (Figure 3I). Therefore, these findings confirmed that our constructed signature was robust and universal.
LMDG-based signature independently predicted the patient’s prognosis
Because our constructed LMDG-based signature was extensively verified with independent cohorts, univariate and multivariate Cox regression analyses were conducted to evaluate whether our LMRG-based signature independently predicted early LUAD prognosis. Based on this, the risk score independently predicted poor OS (Figure 3J, HR =6.816, 95% CI: 3.282–14.158, P<0.001) after adjusting additional.
Biological pathway enrichment, along with functional annotation
Since the as-constructed LMDG-based signature performed well in predicting LUAD prognosis, we analyzed its associated mechanisms. Firstly, we completed a differential analysis of both groups (|log2 FC| ≥1, adjusted P<0.05). As a result, for the high-risk group, 97 and 34 genes showed up- and down-regulation, respectively (Figure 4A). Afterward, these DEGs underwent GO and KEGG analyses. Moreover, a biological process analysis showed that hypoxia and humoral immune response enriched DEGs (Figure 4B,4C).
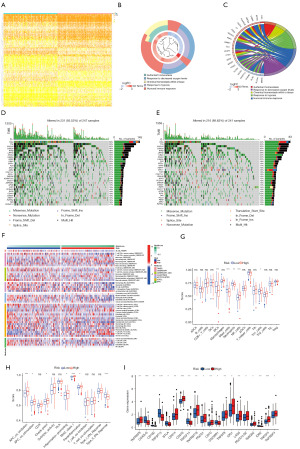
Determination of lipid metabolism gene-related risk score-related mutation landscape
A comparison of both risk groups regarding their mutation landscapes revealed that the high-risk group exhibited a higher mutation frequency; moreover, TTN and TP53 were the most frequent mutations among them (Figure 4D,4E).
Immune status analysis
The relationship between the lipid metabolism DEGs-related risk score and the immune status of TCGA patients was analyzed, which showed significant changes in the immune cells (Figure 4F,4G). There were significant differences in immune-related pathways, such as type II interferon (IFN) response (Figure 4H). A comparison of immune checkpoint-related genes between the two groups revealed that the high-risk patients showed a significant reduction in expressions of CD40LG, CD160, TNFSF15, BTLA, TNFSF14, CD200R1, and IDO2 (Figure 4I). Based on this, high-risk patients displayed enhanced tumor immune activities when compared with the low-risk cases.
Prediction of chemotherapy responses in cases with diverse lipid metabolism-related risk score
Additionally, chemotherapeutic responses were predicted for both risk groups by using the GDSC database to determine possible treatments. Nine common drugs for treating NSCLC were adopted in further analyses. Subsequently, IC50 values of nine agents (cisplatin, docetaxel, doxorubicin, gemcitabine, erlotinib, etoposide, paclitaxel, rapamycin, and vinorelbine) were significantly increased among low-risk cases relative to their high-risk counterparts (Figure 5), suggesting the higher chemoresistance of LUAD cases which displayed lower risk scores when compared with those having increased risk scores.
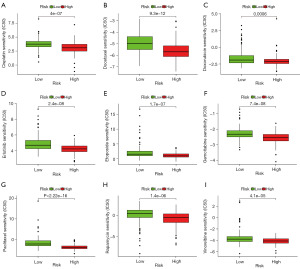
Nomogram establishment and validation for lipid metabolism gene-related risk score
Based on clinical factors like age, TNM staging, gender, smoking status, and LMDG, a nomogram was established (Figure 6). As shown, the risk factors were related to specific estimates for an accurate survival prediction at 1, 3, and 5 years (Figure 7A-7C). Thus, our results exhibited the clinical efficacy of LMDGs-based signature in LUAD.
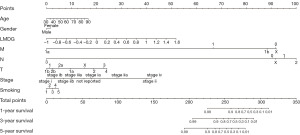
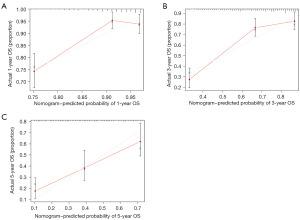
Discussion
Lung cancer is one of the most common malignancies worldwide, accounting for a higher number of new cases and mortality. LUAD is a frequently seen pathological subtype, occupying around 40% of all lung cancer subtypes (9). Due to its low early diagnosis rate and poor prognosis, exploring an efficient and stable prognosis model for its treatment regime is of great importance. Metabolic reprogramming exerts a critical effect on carcinogenesis and cancer progression as cancer cells use metabolic pathways for maintaining rapid proliferation, survival, migration, and invasion (10). Additionally, lipid metabolism is closely associated with biofilm formation, signaling, and energy generation, thus, affecting the interactions between immune response, drug resistance, and TME within a variety of malignant tumors (11,12). Another study (13) reported that lipid metabolism disorders might be associated with cancer occurrence and migration. Our study focused on exploring the relationship of lipid metabolism with LUAD by including lipid metabolism genes for improving the accuracy of prognosis prediction and providing appropriate clinical interventions. Among all prognostic biomarkers, few specific biological processes-based polygenic markers were superior in predicting cancer prognosis (14-16). Therefore, we investigated the role of prognostic gene signatures in early LUAD cases showing lipid metabolism changes.
In this study, 273 LMDGs were identified through intersecting lipid metabolism genes with LUAD DEGs from three databases, GSEA, Gene Card, and UCSC. An enrichment analysis proved that the DEGs were associated with fatty acid metabolism, which is the basic substrate required for energy storage, membrane synthesis, and signal analysis in tumor cells (17). Through a univariate Cox regression analysis, 83 LMDGS were significantly correlated with patient survival. Finally, 11 genes were selected from TCGA and GEO datasets through LASSO analysis as prognostic gene signatures and were included in LUAD prognostic formula, which classified cases as low- or high-risk groups. This signature was verified based on internal subsets and external validation sets. However, OS was significantly different in low-risk when compared with the high-risk groups, showing that our signature model was associated with multiple lipid metabolic pathways. A multivariate Cox regression revealed that risk score independently predicted the patient’s prognosis. Additionally, the signature’s prognosis prediction mechanism in LUAD cases was significantly associated with humoral immune response. The human immune system has a strong antitumor capacity, and immunotherapy is proposed as an effective treatment for tumors. Several studies suggest that immunotherapy can significantly improve the survival of LUAD patients (18-22). Furthermore, we explored the relationship of the LMDG model with immune status and response to chemotherapy drugs. The results exhibited that our model was associated with drug sensitivity and immune checkpoint and validated the finding of whether patients were suitable to receive immunotherapy or chemotherapy.
In conclusion, the prognostic model consisted of 11 genes, namely, LDHA, SEC14L3, LPL, ACSS3, GAPDH, ESYT3, SEC14L4, CYP17A1, CFTR, SLC16A1, and CIDEC, most of which were related to tumor proliferation, migration, and invasion. For example, LDHA lactate dehydrogenase, a key enzyme in the glycolytic pathway, is closely related to the occurrence, metastasis as well as poor tumor survival, like breast cancer (BC), lung cancer, and gastric cancer (GC) (23-25). Additionally, ACSS3 can suppress prostate cancer development (26), as well as enhance GC and bladder cancer cell growth, invasion, and migration, thus, reducing patients’ OS time (27,28). Haiyan’s study (29) indicated that high expression of ESYT3 in BC enhanced cancer cell growth and migration, thereby promoting BC occurrence and development along with poor patient survival. Another study by Zhao (30) showed that a low expression of ESYT3 in LUAD affected cell cycle pathways and the patient’s prognosis. The CFTR is an ion channel. A study (31) reported that CFTR affected cancer cell growth by regulating the cell cycle and influenced cell migration by regulating metabolic pathways. Chuang’s results (32) showed that CYP17A1, a member of the cytochrome P450 family, enhanced glioma cell growth. Based on the characteristics of the 11 genes in our study, a risk score was performed for each LUAD patient to identify high-risk patients.
This feature was validated in LUAD internal cohort outcomes. Moreover, the assessment of clinical parameters, including age, gender, smoking status, and pathological TNM staging, displayed that our model was only associated with the risk score, suggesting that the risk score independently predicted the prognosis. Although these characteristics correlated with prognosis prediction among different cohorts, we focused on its associated mechanisms. A total of 131 DEGs were found in both risk groups. Based on GO and KEGG enrichment analyses, the DEGs showed major enrichment in the humoral immune response pathway, thereby indicating the interplay of different immune activities. In our as-established model, TP53 and TTN displayed more mutations in the high-risk category as compared to low-risk groups. Mutations of these two genes are associated with immunotherapy (33). A study has shown that p53 deletion or mutation may affect T cell activity and thus lead to immune evasion (34). Hence, the correlation between LMDG, immunotherapeutic response, and immune microenvironment was further explored. Our results showed that low-risk patients displayed an increased immune cell [interstitial dendritic cells (iDCs), Mast cells, and neutrophils] enrichment relative to their high-risk counterparts. However, based on immune response results, low-risk patients had reduced expression levels compared with the high-risk group, thus advocating enhanced immune activity of high-risk patients. Besides, the most important pathway in high versus low-risk groups was the humoral immune response, suggesting that immune and inflammatory activities were potential mechanisms affecting LUAD recurrence and survival.
Finally, we also predicted the therapeutic response of commonly used chemotherapy agents by analyzing whether the characteristic model was effective in predicting LUAD prognosis. Consequently, IC50 levels of nine common chemotherapy drugs increased among the low-risk cases relative to their high-risk counterparts, indicating that cases displaying high-risk scores showed high chemosensitivity. Nine conventional chemotherapy drugs used for treating LUAD include cisplatin, docetaxel, etoposide, gemcitabine, paclitaxel, vinorelbine, doxorubicin, erlotinib, and sorafenib (35-39). Our results also indicate that our signature might be utilized for personalizing treatments for LUAD cases. Additionally, the nomogram built for predicting LUAD prognosis can also provide better clinical guidance. The figure also demonstrates the importance of LMDG in predicting LUAD prognosis.
Although our characteristic model provides new hope for predicting LUAD survival, immunotherapy, and chemotherapy response, this study had a few limitations. All data sources were from public databases and needed to be verified experimentally. Collectively, the lipid metabolism prognostic model was built for LUAD patients, which can be used to effectively predict immune checkpoint inhibitors and chemotherapeutic responses, thereby targeting patients for personalized treatments.
Conclusions
The prognosis model of 11 genes related to lipid metabolism was constructed for LUAD, and a new idea was provided for the treatment of high-risk lung adenocarcinoma patients based on immune function mutation.
Acknowledgments
Funding: This work was supported by
Footnote
Reporting Checklist: The authors have completed the TRIPOD reporting checklist. Available at https://tcr.amegroups.com/article/view/10.21037/tcr-23-375/rc
Peer Review File: Available at https://tcr.amegroups.com/article/view/10.21037/tcr-23-375/prf
Conflicts of Interest: All authors have completed the ICMJE uniform disclosure form (available at https://tcr.amegroups.com/article/view/10.21037/tcr-23-375/coif). The authors have no conflicts of interest to declare.
Ethical Statement: The authors are accountable for all aspects of the work in ensuring that questions related to the accuracy or integrity of any part of the work are appropriately investigated and resolved. The study was conducted in accordance with the Declaration of Helsinki (as revised in 2013).
Open Access Statement: This is an Open Access article distributed in accordance with the Creative Commons Attribution-NonCommercial-NoDerivs 4.0 International License (CC BY-NC-ND 4.0), which permits the non-commercial replication and distribution of the article with the strict proviso that no changes or edits are made and the original work is properly cited (including links to both the formal publication through the relevant DOI and the license). See: https://creativecommons.org/licenses/by-nc-nd/4.0/.
References
- Sung H, Ferlay J, Siegel RL, et al. Global Cancer Statistics 2020: GLOBOCAN Estimates of Incidence and Mortality Worldwide for 36 Cancers in 185 Countries. CA Cancer J Clin 2021;71:209-49. [Crossref] [PubMed]
- Pahar B, Lala W, Kuebler D, et al. A significant productive in vivo infection of resting cells with simian immunodeficiency virus in a macaque with AIDS. J Med Primatol 2017;46:59-62. [Crossref] [PubMed]
- Swinnen JV, Brusselmans K, Verhoeven G. Increased lipogenesis in cancer cells: new players, novel targets. Curr Opin Clin Nutr Metab Care 2006;9:358-65. [Crossref] [PubMed]
- Hess D, Chisholm JW, Igal RA. Inhibition of stearoylCoA desaturase activity blocks cell cycle progression and induces programmed cell death in lung cancer cells. PLoS One 2010;5:e11394. [Crossref] [PubMed]
- Noto A, Raffa S, De Vitis C, et al. Stearoyl-CoA desaturase-1 is a key factor for lung cancer-initiating cells. Cell Death Dis 2013;4:e947. [Crossref] [PubMed]
- Guillaumond F, Bidaut G, Ouaissi M, et al. Cholesterol uptake disruption, in association with chemotherapy, is a promising combined metabolic therapy for pancreatic adenocarcinoma. Proc Natl Acad Sci U S A 2015;112:2473-8. [Crossref] [PubMed]
- Delmas D, Rébé C, Micheau O, et al. Redistribution of CD95, DR4 and DR5 in rafts accounts for the synergistic toxicity of resveratrol and death receptor ligands in colon carcinoma cells. Oncogene 2004;23:8979-86. [Crossref] [PubMed]
- Stratton SA, Barton MC. p53-Mediated regulation of hepatic lipid metabolism: forging links between metabolism, atherogenesis, and cancer. J Hepatol 2012;56:518-9. [Crossref] [PubMed]
- Abe Y, Tanaka N. The Hedgehog Signaling Networks in Lung Cancer: The Mechanisms and Roles in Tumor Progression and Implications for Cancer Therapy. Biomed Res Int 2016;2016:7969286. [Crossref] [PubMed]
- Pavlova NN, Thompson CB. The Emerging Hallmarks of Cancer Metabolism. Cell Metab 2016;23:27-47. [Crossref] [PubMed]
- Liu Q, Luo Q, Halim A, et al. Targeting lipid metabolism of cancer cells: A promising therapeutic strategy for cancer. Cancer Lett 2017;401:39-45. [Crossref] [PubMed]
- Long J, Zhang CJ, Zhu N, et al. Lipid metabolism and carcinogenesis, cancer development. Am J Cancer Res 2018;8:778-91. [PubMed]
- Furuta E, Okuda H, Kobayashi A, et al. Metabolic genes in cancer: their roles in tumor progression and clinical implications. Biochim Biophys Acta 2010;1805:141-52. [PubMed]
- Zhang C, Zhang Z, Zhang G, et al. Clinical significance and inflammatory landscapes of a novel recurrence-associated immune signature in early-stage lung adenocarcinoma. Cancer Lett 2020;479:31-41. [Crossref] [PubMed]
- Zhao L, Jiang L, He L, et al. Identification of a novel cell cycle-related gene signature predicting survival in patients with gastric cancer. J Cell Physiol 2019;234:6350-60. [Crossref] [PubMed]
- Liu GM, Xie WX, Zhang CY, et al. Identification of a four-gene metabolic signature predicting overall survival for hepatocellular carcinoma. J Cell Physiol 2020;235:1624-36. [Crossref] [PubMed]
- Currie E, Schulze A, Zechner R, et al. Cellular fatty acid metabolism and cancer. Cell Metab 2013;18:153-61. [Crossref] [PubMed]
- Tennant DA, Durán RV, Gottlieb E. Targeting metabolic transformation for cancer therapy. Nat Rev Cancer 2010;10:267-77. [Crossref] [PubMed]
- Denisenko TV, Budkevich IN, Zhivotovsky B. Cell death-based treatment of lung adenocarcinoma. Cell Death Dis 2018;9:117. [Crossref] [PubMed]
- Spella M, Stathopoulos GT. Immune Resistance in Lung Adenocarcinoma. Cancers (Basel) 2021;13:384. [Crossref] [PubMed]
- Succony L, Rassl DM, Barker AP, et al. Adenocarcinoma spectrum lesions of the lung: Detection, pathology and treatment strategies. Cancer Treat Rev 2021;99:102237. [Crossref] [PubMed]
- Zhang L, Bai L, Liu X, et al. Factors related to rapid progression of non-small cell lung cancer in Chinese patients treated using single-agent immune checkpoint inhibitor treatment. Thorac Cancer 2020;11:1170-9. [Crossref] [PubMed]
- Fan J, Hitosugi T, Chung TW, et al. Tyrosine phosphorylation of lactate dehydrogenase A is important for NADH/NAD(+) redox homeostasis in cancer cells. Mol Cell Biol 2011;31:4938-50. [Crossref] [PubMed]
- Jin L, Chun J, Pan C, et al. Phosphorylation-mediated activation of LDHA promotes cancer cell invasion and tumour metastasis. Oncogene 2017;36:3797-806. [Crossref] [PubMed]
- Li X, Zhang C, Zhao T, et al. Lysine-222 succinylation reduces lysosomal degradation of lactate dehydrogenase a and is increased in gastric cancer. J Exp Clin Cancer Res 2020;39:172. [Crossref] [PubMed]
- Zhou L, Song Z, Hu J, et al. ACSS3 represses prostate cancer progression through downregulating lipid droplet-associated protein PLIN3. Theranostics 2021;11:841-60. [Crossref] [PubMed]
- Chang WC, Cheng WC, Cheng BH, et al. Mitochondrial Acetyl-CoA Synthetase 3 is Biosignature of Gastric Cancer Progression. Cancer Med 2018;7:1240-52. [Crossref] [PubMed]
- Zhang J, Duan H, Feng Z, et al. Acetyl-CoA synthetase 3 promotes bladder cancer cell growth under metabolic stress. Oncogenesis 2020;9:46. [Crossref] [PubMed]
- Haiyan S, Xinna D, Weiping F, et al. Analysis of ESYT3 expression and its clinical significance in breast cancer based on high-throughput multi-omics databases. Chinese Journal of Clinical Laboratory Science 2018;36:579-82.
- Zhao R, Feng SY, Xuan CH, et al. Expression changes, clinical significance, and molecular mechanism of ESYT3 gene in lung adenocarcinoma based on TCGA database. Shandong Medical Journal 2022;62:54-7.
- Shin Y, Kim M, Won J, et al. Epigenetic Modification of CFTR in Head and Neck Cancer. J Clin Med 2020;9:734. [Crossref] [PubMed]
- Chuang JY, Lo WL, Ko CY, et al. Upregulation of CYP17A1 by Sp1-mediated DNA demethylation confers temozolomide resistance through DHEA-mediated protection in glioma. Oncogenesis 2017;6:e339. [Crossref] [PubMed]
- Li Z, Liu Z, Wu Y, et al. Efficacy and safety of apatinib alone or apatinib plus paclitaxel/docetaxel versus paclitaxel/docetaxel in the treatment of advanced non-small cell lung cancer: A meta-analysis. Thorac Cancer 2021;12:2838-48. [Crossref] [PubMed]
- Blagih J, Buck MD, Vousden KH. p53, cancer and the immune response. J Cell Sci 2020;133:jcs237453. [Crossref] [PubMed]
- Kim IK, McCutcheon JN, Rao G, et al. Acquired SETD2 mutation and impaired CREB1 activation confer cisplatin resistance in metastatic non-small cell lung cancer. Oncogene 2019;38:180-93. [Crossref] [PubMed]
- Li Y, Sun R, Li R, et al. Prognostic Nomogram Based on Circular RNA-Associated Competing Endogenous RNA Network for Patients with Lung Adenocarcinoma. Oxid Med Cell Longev 2021;2021:9978206. [Crossref] [PubMed]
- Perdrizet K, Sutradhar R, Li Q, et al. Second and later-line erlotinib use in non-small cell lung cancer: real world outcomes and practice patterns overtime in Canada. J Thorac Dis 2021;13:5419-29. [Crossref] [PubMed]
- Provencio M, Majem M, Guirado M, et al. Phase II clinical trial with metronomic oral vinorelbine and tri-weekly cisplatin as induction therapy, subsequently concomitant with radiotherapy (RT) in patients with locally advanced, unresectable, non-small cell lung cancer (NSCLC). Analysis of survival and value of ctDNA for patient selection. Lung Cancer 2021;153:25-34. [Crossref] [PubMed]
- Yamaguchi M, Tada H, Mitsudomi T, et al. Phase III study of adjuvant gemcitabine compared with adjuvant uracil-tegafur in patients with completely resected pathological stage IB-IIIA non-small cell lung cancer (WJTOG0101). Int J Clin Oncol 2021;26:2216-23. [Crossref] [PubMed]