Identification of potential therapeutic targets using breast cancer stroma expression profiling
Introduction
Breast cancer is a major cause of morbidity and mortality in women worldwide (1). Breast cancer is recognized as a heterogeneous disease. Even when morphological characteristics and ER phenotypes are similar, patients have varying prognosis or chemotherapy response (2). Therefore, individual treatment for breast cancer patients requires modification to a more practical clinical signature.
The view of cancer as a systemic disease indicates that the development of malignant tumors is involved in a complex biological process that be directed by the body’s systemic responses to malignancy (3). Paraneoplastic syndromes are the manifestation of systemic complications caused by organ or tissue damage at locations remote from the site of the primary tumor or metastases (4), such as leukocytosis and thrombocytosis, commonly associated multiple type of malignancies including breast cancer (5). Primary tumors communicate with distant tissues by varied means, such as releasing of soluble cytokines into the circulation. For instance, increased hepatic thrombopoietin synthesis in response to tumor-derived interleukin-6 was an underlying mechanism of paraneoplastic thrombocytosis (6). Excessive IL-6 has been demonstrated in primary breast tumors and breast cancer patient sera and is associated with poor clinical outcomes in breast cancer (7,8).
Carcinomas are composed of malignant epithelial cells and complex tissue environment of diverse stromal cells, which they depend upon for sustained growth, invasion and metastasis. In addition to tumor-driven systemic perturbations, the stroma cells of tumor microenvironment (TME) be educated and sculpted by tumor cells via paracrine and juxtacrine, may also have capacity of promoting systemic effects (9).
Cytokines and chemokines are important constituents of the TME. Increasing number of studies indicate that cytokines and chemokines function as critical regulators involving immune cell responses, angiogenesis, lymphangiogenesis, extracellular matrix (ECM) dynamics (10-12). These studies implied that cytokines and chemokines may be commonly used in para-tumor context. Herein, we hypothesized that the cytokine expression pattern is potentially a manifestation of paraneoplastic responses, which seems to be consistent in breast cancer patients. Further specification of this cytokine pattern could offer novel therapeutic approaches.
Methods
Data sources
Microarray data, accession number GSE9014, deposited by Finak et al. (13), were downloaded from gene expression omnibus (GEO) (14), samples included 53 cases (50 invasive ductal carcinoma IDC and 3 invasive lobular carcinoma cases) of tumor stroma, and 31 cases of individual-matched normal adjacent stroma. Patient’s surgical excision were frozen in liquid nitrogen within 30 min of surgical resection. A clinical pathologist identified distinct regions of tumor stroma and stroma surrounding morphologically normal ducts prior to laser-capture microdissection (LCM) (13). The clinical characteristics of the patients are showed in Table 1 (14). All of the patients had not underwent neoadjuvant therapy before surgical (13). Genes whose expression varied most between tumor tissue and normal stroma for the 31 tissue-matched pairs of this data had been identified to be independent of ER, HER2 and lymph node status, as well as age, grade and tumor size (13). In Finak’s study, all patients provided written, informed consent and the experiments were approved by the McGill University Health Centre (MUHC) Research Ethics Board (Protocols SUR-99-780 and SUR-00-966) (13).
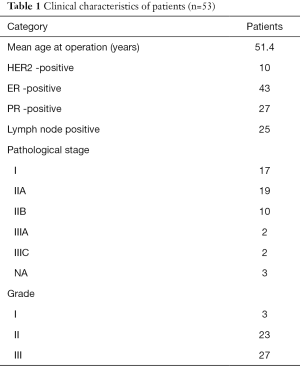
Full table
Data preprocessing and identification of differentially-expressed genes (DEGs)
The raw data were analyzed using the integrated software package biometric research branch (BRB) ArrayTools version 4.0 (15). BRB-Array tools performed a series of preprocessing steps including filtering and computing hybridization data of each probe in the probe set. Probes with the following conditions were removed: (I) greater than 20% of expression data values had more than 1.5-fold change in either direction from the median value; (II) more than 50% of the gene expression data were “absent”. The Robust Multiarray Average (RMA) method (16) was used for normalization. The statistical analysis of microarray (SAM) method (17) was used to analyze the transcription profiles and screen for DEGs [false discovery rate (FDR) ≤1%, |log (fold change, FC)|≥1].
Functional enrichment analysis of DEGs
Gene ontology (GO) (18) enrichment analysis was carried out to uncover the biological processes of the DEGs. Additionally, the biological pathway enrichment analysis of DEGs was performed based on the Kyoto Encyclopedia of Genes and Genomes (KEGG) database (19). The online based tool of the database for annotation, visualization and integrated discovery (DAVID) (20) was used to perform these analyses.
Screening of DEGs for cytokines and cytokine receptors
The cytokines, cytokine receptors, and chemokines were screened based on KEGG ko04052: Cytokines (21) and their functions are listed in NCBI Mesh (22). KEGG ko04052 collected 230 cytokines in 8 families, including class I cytokines (hematopoietic family), class II cytokines (interferon/IL-10 family), PDGF family, TNF family, IL-1 family, IL-17 family, TGF-beta family, and chemokines.
Protein-protein interaction (PPI) network construction and subnetwork analysis
PPI network of the DE cytokine and chemokine genes was established based on the Search Tool for the Retrieval of Interacting Genes (STRING) database (23), and only interactions with combined score >0.4 were selected to construct the PPI network and visualized. The property of the network was evaluated using the network analyzer plugin of Cytoscape (24). The proteins in the network served as nodes and the degree of a node corresponded to the number of interactions with other proteins. The proteins with high degrees were considered as the hub nodes. In addition, we further performed subnetwork mining in the PPI network based on KMEANS clustering algorithm to cluster the proteins displaying in the network. The only input to the clustering algorithm was the distance matrix obtained from the String global scores (therefore interacting proteins with a higher global score had more chances to end up in the same cluster).
Results
DEGs between breast cancer stroma samples and normal samples
A total of 3,420 transcripts, corresponding to 2,737 DEGs, including 1,122 upregulated DEGs and 1,615 down regulated DEGs were identified between the breast cancer stroma samples and the controls. Among these DEGs, 55 were up-regulated and 48 were down-regulated cytokines and cytokine–related genes (for instance cytokine receptors).
Functional enrichment analysis of up- and down-regulated cytokines
GO biological processes enrichment analysis revealed that 185 functional terms for DE cytokine genes (both down- and up-regulated cytokine genes) were enriched; the top five significantly enriched processes were mainly related to immune response and leukocyte activation; beyond that, the DE cytokine genes were also involved in a variety of biological processes, such as fibroblast growth factor receptor signaling pathway and angiogenesis. The GO functional terms ranked by statistical significance were listed in Table 2.
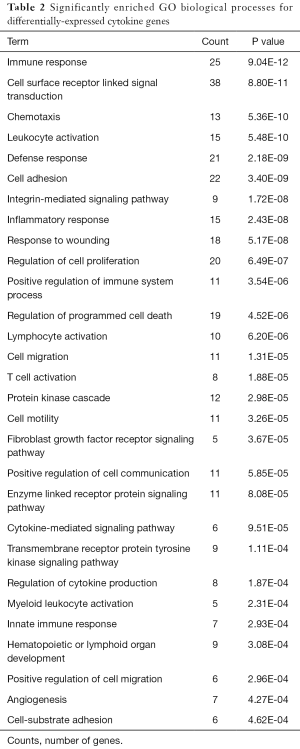
Full table
The up-regulated cytokines were enriched in seven pathways, which were mainly involved in pathways related to cancer such as cytokine-cytokine receptor interaction pathways and hematopoietic cell lineage. Meanwhile, the down-regulated cytokines were enriched in four pathways, which were cytokine-cytokine receptor interaction, toll-like receptor (TLR) signaling pathway, chemokine signaling pathway, cell adhesion molecules (CAMs). The significantly enriched pathways of up- and down-regulated cytokines are listed in Table 3.
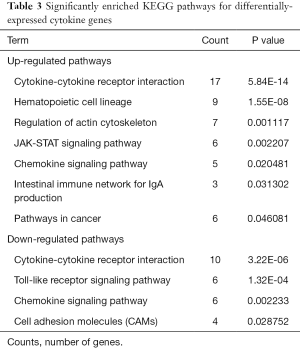
Full table
PPI network construction and subnetwork analysis
The PPI network of DE cytokine genes (both down- and up-regulated) was constructed, and included 85 nodes and 236 interactions, as shown in Figure 1. Nodes with large degree and high BC (Betweenness Centrality, a metric that captures the importance of each individual node in the overall network structure) are represented as the hub genes displayed in Table 4. Furthermore, three subnetworks were selected from DE PPI pairs, Figure 2. The hub proteins CXCL12, CXCR4, KIT, TIMP1, and CD34 were demonstrated to be involved in subnetwork 1, while TLR2, ITGAM, and CD36 were in subnetwork 2, and SOCS3 in subnetwork 3.
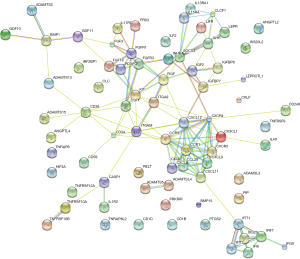
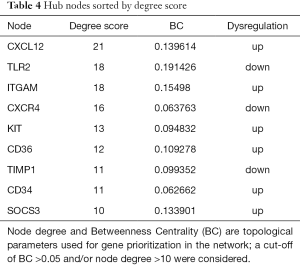
Full table
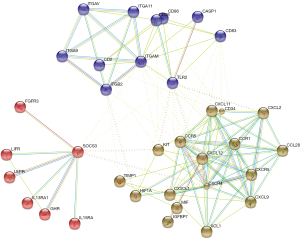
Discussion
During tumor progression, a variety of factors released by tumor tissue not only regulate the local microenvironment, but also regulate distal tissues. Alterations within the tumor macro-environment, leads to the promotion of tumor growth, invasion, and eventual metastasis (25). Though tumor cell is master regulator of the tumor macro-environment, other types of cells in tumor microenvironment may also coordinate the development of cancer-associated systemic syndromes.
In this study, using mRNA expression profile, we focused on breast cancer stroma, and identified 56 up-regulated and 48 down-regulated genes of cytokines or chemokines. GO functional annotation and KEGG pathway analyses revealed that the DE cytokines were enriched in a variety of biological progresses (BP). These multiple progresses were in relation to inducing inflammation, blood vessel growth, leuko-monocyte differentiation, ECM turnover and remodeling, thus reflecting the continual changes and evolution of the tumor macro- and micro-environment as the tumor grows.
PPIs analysis can visualize functional links between DEGs at the molecular level, and is useful in helping understand the molecular mechanism of diseases and their potentially essential genes. We therefore constructed the PPI network between the DE cytokines, and observed three subnetworks with highly connected nodes, using K-MEANS clustering algorithm.
Subnetwork 1 was mainly enriched in two KEGG pathways, cytokine-cytokine receptor interaction and chemokine signaling pathway. CXCL12 and its receptor CXCR4, were the hub proteins of subnetwork 1. CXCL12, also known as stromal-derived factor 1α (SDF-1), is a secreted protein expressed widely in immune cells, endothelial cells, stromal fibroblasts (26). Through CXCL12/CXCR4 axis, interplays exist not only between the local neighboring cells, but also between the tumor microenvironment and the patient’s organs and systems. Megakaryocytes (MKs) are specialized precursor cells that produce platelets. MKs express the receptor CXCR4 in response to SDF1 and to increase their platelet production (27). A recent study shows that SDF-1 increases megakaryocyte-vascular association and resulting in thrombopoiesis (28). Paraneoplastic thrombocytosis is associated with many solid tumors and can promote tumor growth and metastasis (29). The present study revealed that the CXCL12 gene was up-regulated in the stroma of breast cancer and it was a hub protein with the highest degree score in the established PPI network. Therefore, CXCL12 may be a key regulator in stroma-host interactions as in breast cancer development. Potentially, therapeutic strategies targeting CXCL12 may lead to pleiotropic treatments for breast cancer by interrupting stroma-driven systemic perturbations.
ITGAM and TLR2 were the hub proteins of subnetwork 2. ITGAM (Integrin Alpha M), encoding CD11b, is the common marker of myeloid-derived suppressor cells (MDSCs) (30). CD11b binds to ligands to regulate leukocyte adhesion and migration across the endothelium to inflammatory sites (31). The expression of ITGAM was significantly up-regulated in breast tumor stroma indicating the accumulation of MDSCs in the tumor microenvironment, which is a paraneoplastic phenomenon of cancer-associated myeloproliferation (32). The immature myeloid cells lead to the suppression of host immunity and promotion of tumor angiogenesis, both of which play a role in tumorigenesis and metastasis, representing a novel therapeutic target in cancer (33). Research in colorectal cancer (CRC) shows that CD11b deficiency contributes to the inhibition of myeloid cell trafficking to the tumor microenvironment and thereby suppresses the angiogenesis and tumor growth (34). Since CD11b collectively expressed on the surface of a variety of myeloid cells across different tumor types, it would be a potential therapeutic target in breast cancer.
TLRs are key factors in the innate immune system, which recognize antigens that are not normally expressed within the host, and initiate the inflammatory response by promoting the release of cytokines, chemokines, and other agents required in antitumor immunity (35). TLR2 is found in a large diversity of cells of the immune system. It has been implicated in the response to tumor-derived factors, and that genetic ablation of TLR2 in primary bone marrow-derived macrophages abolished the activation effect in breast cancer (36). In the present study,TLR2 was down-regulated in breast cancer stroma cells and was hub node in the same PPI subnetwork with CD11b. We supposed that both of the two molecules might play a key role in the development of MDSCs in breast cancer. Given the emerging importance of MDSCs in breast cancer, modulating MDSCs actions is an attractive avenue of further therapy research for those incurable breast cancers (37).
Suppressor of cytokine signaling protein 3 (SOCS3), hub protein of the subnetwork 3, is negative regulator of cytokine signaling mediated by the JAK-STAT signaling pathway. Many studies suggest that macrophage SOCS3 is associated with M1 macrophages and pro-inflammatory responses. However the exact consequences of SOCS3 signaling, either positive or negative, are still controversial (38-40). Heys et al. (41), determined that the number of SOCS3-expressing pro-inflammatory and cytotoxic macrophages correlated with early response to therapy, but not with worse survival. SOCS3 expression may identify macrophages with enhanced tumor-killing capacity. Moreover, in addition to macrophages, the function of endothelial cells and dendritic cells are affected by SOCS3 (42,43). Targeting SOCS3 may therefore be important an efficient means of tailoring the inflammatory response in tumor biology.
In conclusion, we found that tumor stroma gene expression profiles were markedly altered during the development and progression of breast cancer. Specifically, cytokines, as well as their related genes, may be important in the paraneoplastic response. We identified CXCL12, TLR2, ITGAM, and SOCS3 as the key genes of cytokine regulatory network, thus may be potential targets in the development of treatments for breast cancer. However, because the results are based on microarray data with a small sample size, more experimental validations are warranted. Further studies are necessary to evaluate their potential applications as therapeutic targets.
Acknowledgments
We thank LetPub for its linguistic assistance during the preparation of this manuscript.
Funding: This work was supported by the National Natural Science Foundation of China (grant no.81201702). Health and Family Planning Commission of Sichuan Province of China (grant no.120491). Scientific research project of Sichuan Provincial Health Department (grant no.13Z068); Science and technology support program of Sichuan Science and Technology Department (grant no.2016GZ0364).
Footnote
Conflicts of Interest: All authors have completed the ICMJE uniform disclosure form (available at http://dx.doi.org/10.21037/tcr.2016.05.07). The authors have no conflicts of interest to declare.
Ethical Statement: The authors are accountable for all aspects of the work in ensuring that questions related to the accuracy or integrity of any part of the work are appropriately investigated and resolved. The study was conducted in accordance with the Declaration of Helsinki (as revised in 2013). In Finak’s study, all patients provided written, informed consent and the experiments were approved by the McGill University Health Centre (MUHC) Research Ethics Board (Protocols SUR-99-780 and SUR-00-966).
Open Access Statement: This is an Open Access article distributed in accordance with the Creative Commons Attribution-NonCommercial-NoDerivs 4.0 International License (CC BY-NC-ND 4.0), which permits the non-commercial replication and distribution of the article with the strict proviso that no changes or edits are made and the original work is properly cited (including links to both the formal publication through the relevant DOI and the license). See: https://creativecommons.org/licenses/by-nc-nd/4.0/.
References
- WHO|Breast cancer: prevention and control. In. 2015. Available online: http://www.who.int/cancer/detection/breastcancer/en/
- Breast Cancer Treatment (PDQ®) - National Library of Medicine - PubMed Health. In. 2015. Available online: http://www.ncbi.nlm.nih.gov/pubmedhealth/PMH0032825/
- McAllister SS, Weinberg RA. The tumour-induced systemic environment as a critical regulator of cancer progression and metastasis. Nat Cell Biol 2014;16:717-27. [Crossref] [PubMed]
- Kanaji N, Watanabe N, Kita N, et al. Paraneoplastic syndromes associated with lung cancer. World J Clin Oncol 2014;5:197-223. [Crossref] [PubMed]
- Pelosof LC, Gerber DE. Paraneoplastic syndromes: an approach to diagnosis and treatment. Mayo Clin Proc 2010;85:838-54. [Crossref] [PubMed]
- Stone RL, Nick AM, McNeish IA, et al. Paraneoplastic thrombocytosis in ovarian cancer. N Engl J Med 2012;366:610-8. [Crossref] [PubMed]
- Heo TH, Wahler J, Suh N. Potential therapeutic implications of IL-6/IL-6R/gp130-targeting agents in breast cancer. Oncotarget 2016; [Epub ahead of print]. [Crossref] [PubMed]
- Stravodimou A, Voutsadakis IA. Pretreatment thrombocytosis as a prognostic factor in metastatic breast cancer. Int J Breast Cancer 2013;2013:289563.
- Quail DF, Joyce JA. Microenvironmental regulation of tumor progression and metastasis. Nat Med 2013;19:1423-37. [Crossref] [PubMed]
- Rivas-Fuentes S, Salgado-Aguayo A, Pertuz Belloso S, et al. Role of Chemokines in Non-Small Cell Lung Cancer: Angiogenesis and Inflammation. J Cancer 2015;6:938-52. [Crossref] [PubMed]
- Doldi V, Callari M, Giannoni E, et al. Integrated gene and miRNA expression analysis of prostate cancer associated fibroblasts supports a prominent role for interleukin-6 in fibroblast activation. Oncotarget 2015;6:31441-60. [PubMed]
- Friedl P, Alexander S. Cancer invasion and the microenvironment: plasticity and reciprocity. Cell 2011;147:992-1009. [Crossref] [PubMed]
- Finak G, Bertos N, Pepin F, et al. Stromal gene expression predicts clinical outcome in breast cancer. Nat Med 2008;14:518-27. [Crossref] [PubMed]
- GEO Accession viewer. In. 2015.
- Simon R, Lam A, Li MC, et al. Analysis of gene expression data using BRB-ArrayTools. Cancer Inform 2007;3:11-7. [PubMed]
- Irizarry RA, Hobbs B, Collin F, et al. Exploration, normalization, and summaries of high density oligonucleotide array probe level data. Biostatistics 2003;4:249-64. [Crossref] [PubMed]
- Tusher VG, Tibshirani R, Chu G. Significance analysis of microarrays applied to the ionizing radiation response. Proc Natl Acad Sci U S A 2001;98:5116-21. [Crossref] [PubMed]
- Gene Ontology Consortium. going forward. Nucleic Acids Res 2015;43:D1049-56. [Crossref] [PubMed]
- Kanehisa M, Goto S, Furumichi M, et al. KEGG for representation and analysis of molecular networks involving diseases and drugs. Nucleic Acids Res 2010;38:D355-60. [Crossref] [PubMed]
- Huang da W. Sherman BT, Lempicki RA. Systematic and integrative analysis of large gene lists using DAVID bioinformatics resources. Nat Protoc 2009;4:44-57. [PubMed]
- KEGG BRITE: Cytokines. In. 2015. Available online: http://www.kegg.jp/kegg-bin/get_htext?htext=ko04052.keg&query=Cytokines
. In.Cytokines - MeSH - NCBI 2015 . Available online: http://www.ncbi.nlm.nih.gov/mesh/68016207- Jensen LJ, Kuhn M, Stark M, et al. STRING 8--a global view on proteins and their functional interactions in 630 organisms. Nucleic Acids Res 2009;37:D412-6. [Crossref] [PubMed]
- Shannon P, Markiel A, Ozier O, et al. Cytoscape: a software environment for integrated models of biomolecular interaction networks. Genome Res 2003;13:2498-504. [Crossref] [PubMed]
- Rutkowski MR, Svoronos N, Perales-Puchalt A, et al. The Tumor Macroenvironment: Cancer-Promoting Networks Beyond Tumor Beds. Adv Cancer Res 2015;128:235-62. [Crossref] [PubMed]
- Yu Y, Xiao CH, Tan LD, et al. Cancer-associated fibroblasts induce epithelial-mesenchymal transition of breast cancer cells through paracrine TGF-β signalling. Br J Cancer 2014;110:724-32. [Crossref] [PubMed]
- Abraham M, Weiss ID, Wald H, et al. Sequential administration of the high affinity CXCR4 antagonist BKT140 promotes megakaryopoiesis and platelet production. Br J Haematol 2013;163:248-59. [PubMed]
- Niswander LM, Fegan KH, Kingsley PD, et al. SDF-1 dynamically mediates megakaryocyte niche occupancy and thrombopoiesis at steady state and following radiation injury. Blood 2014;124:277-86. [Crossref] [PubMed]
- Lin RJ, Afshar-Kharghan V, Schafer AI. Paraneoplastic thrombocytosis: the secrets of tumor self-promotion. Blood 2014;124:184-7. [Crossref] [PubMed]
- Talmadge JE, Gabrilovich DI. History of myeloid-derived suppressor cells. Nat Rev Cancer 2013;13:739-52. [Crossref] [PubMed]
- Schmid MC, Varner JA. Myeloid cells in tumor inflammation. Vasc Cell 2012;4:14. [Crossref] [PubMed]
- Wilcox RA. Cancer-associated myeloproliferation: old association, new therapeutic target. Mayo Clin Proc 2010;85:656-63. [Crossref] [PubMed]
- Casbon AJ, Reynaud D, Park C, et al. Invasive breast cancer reprograms early myeloid differentiation in the bone marrow to generate immunosuppressive neutrophils. Proc Natl Acad Sci U S A 2015;112:E566-75. [Crossref] [PubMed]
- Zhang QQ, Hu XW, Liu YL, et al. CD11b deficiency suppresses intestinal tumor growth by reducing myeloid cell recruitment. Sci Rep 2015;5:15948. [Crossref] [PubMed]
- Erridge C. Endogenous ligands of TLR2 and TLR4: agonists or assistants? J Leukoc Biol 2010;87:989-99. [Crossref] [PubMed]
- Chow A, Zhou W, Liu L, et al. Macrophage immunomodulation by breast cancer-derived exosomes requires Toll-like receptor 2-mediated activation of NF-κB. Sci Rep 2014;4:5750. [Crossref] [PubMed]
- Markowitz J, Wesolowski R, Papenfuss T, et al. Myeloid-derived suppressor cells in breast cancer. Breast Cancer Res Treat 2013;140:13-21. [Crossref] [PubMed]
- Arnold CE, Whyte CS, Gordon P, et al. A critical role for suppressor of cytokine signalling 3 in promoting M1 macrophage activation and function in vitro and in vivo. Immunology 2014;141:96-110. [Crossref] [PubMed]
- Qin H, Holdbrooks AT, Liu Y, et al. SOCS3 deficiency promotes M1 macrophage polarization and inflammation. J Immunol 2012;189:3439-48. [Crossref] [PubMed]
- Yu H, Liu Y, McFarland BC, et al. SOCS3 Deficiency in Myeloid Cells Promotes Tumor Development: Involvement of STAT3 Activation and Myeloid-Derived Suppressor Cells. Cancer Immunol Res 2015;3:727-40. [Crossref] [PubMed]
- Heys SD, Stewart KN, McKenzie EJ, et al. Characterisation of tumour-infiltrating macrophages: impact on response and survival in patients receiving primary chemotherapy for breast cancer. Breast Cancer Res Treat 2012;135:539-48. [Crossref] [PubMed]
- Stahl A, Joyal JS, Chen J, et al. SOCS3 is an endogenous inhibitor of pathologic angiogenesis. Blood 2012;120:2925-9. [Crossref] [PubMed]
- Zeng B, Li H, Liu Y, et al. Tumor-induced suppressor of cytokine signaling 3 inhibits toll-like receptor 3 signaling in dendritic cells via binding to tyrosine kinase 2. Cancer Res 2008;68:5397-404. [Crossref] [PubMed]