Predictive accuracy of machine learning for radiation-induced temporal lobe injury in nasopharyngeal carcinoma patients: a systematic review and meta-analysis
Highlight box
Key findings
• This study presented evidence-based data for the first time on machine learning (ML) models predicting radiation-induced temporal lobe injury (RTLI) in nasopharyngeal carcinoma (NPC) patients. The best discrimination was achieved by models that utilized both radiomics and clinical features.
What is known and what is new?
• Previous studies attempted to create predictive models of RTLI such as NTCP models. However, most variables in these models only contained dosimetric predictors, and no study provided predictive accuracy.
• Recently, ML models have been employed for early RTLI prediction, utilizing radiomics and clinical data as predictors, but their predictive accuracy remained unclear.
What is the implication, and what should change now?
• ML models have been shown to predict RTLI effectively and can prevent serious RTLI at an early stage. Clinicians can use these models to adjust personalized radiotherapy treatment plans and improve patient outcomes.
Introduction
Nasopharyngeal carcinoma (NPC) is a type of cancer that originates from the epithelium of the nasopharynx. The age-standardized incidence of NPC was 0.4 per 100,000 to 3.0 per 100,000 (1). In 2012, there were over 129,000 new cases, with 71% of them occurring in the east and southeast parts of Asia (1). Radiotherapy is the mainstay treatment of NPC (2). For patients with locoregionally advanced NPC, concurrent chemoradiotherapy was also applied (3). However, 2.3–16% of patients who undergo this treatment may experience radiation-induced temporal lobe injury (RTLI) (4), which can cause irreversible damage to emotional and cognitive functions (5,6). To mitigate this risk, intensity modulation has been used during radiotherapy, and temporal lobe dose tolerance has been established since several dosimetric predictors are significantly associated with RTLI (7). However, dose tolerance varies among individuals, and it is more practical to adjust the dose according to the individual’s risk of RTLI, which highlights the importance of predictive models of RTLI. Although previous studies have attempted to create predictive models of RTLI, including normal tissue complication probability (NTCP) models (8-10), most of these models have only included dosimetric predictors and have not provided sufficient predictive accuracy. Recently, additional variables, such as radiomics and clinical data, have been incorporated into predictive models. Radiomics, which is high-dimensional mineable data (11), can contain many details of RTLI and thus improve prediction accuracy. However, previous models were constructed without radiomics due to the limitations of manual data processing in handling large volumes of complex data. An increasing number of promising studies have attempted to employ machine learning (ML) approaches to develop prediction models relying on radiomics (12,13). The biggest strength of this kind of model is the personalized prediction at the early stage of RTLI whereas the biggest weakness is the dependence on imaging data, which may lead to possible additional medical expenses. ML was used to develop predictive models of NPC prognosis and was proven to have great predictive performance (14). Nevertheless, the predictive accuracy of ML models of RTLI is still debated since it varies from around 0.31 to 0.97, which may be caused by different variables and ML approaches (15,16). Also, there is a lack of relevant evidence-based information. Therefore, it is essential to conduct a meta-analysis to evaluate the predictive accuracy of ML for RTLI after radiotherapy. We present this article in accordance with the PRISMA reporting checklist (17) (available at https://tcr.amegroups.com/article/view/10.21037/tcr-23-859/rc).
Methods
The quality of this meta-analysis was assessed using AMSTAR2 (18). The systematic review was registered on PROSPERO (No. CRD42023380907).
Inclusion and exclusion criteria
Inclusion criteria
A study was included when it was nested case-control studies, cohort studies, case-control studies, or case-cohort studies that investigate the predictive value of ML for RTLI in NPC patients; it fully developed one or more ML risk models of RTLI after radiotherapy for NPC; it was with or without external validation; it used at least one outcome parameter, such as receiver operating characteristic (ROC) curve, C-statistic, concordance index (C-index), accuracy, sensitivity (Sen), specificity (Spe), diagnostic 4 grid table, confusion matrix, F1 score, and calibration curve; it developed different models even if they are based on the same cohort; and it was written in English.
Exclusion criteria
A study was excluded when it was randomized controlled trials (RCTs), review articles, meta-analyses, guidelines, or expert opinions; the sample size of RTLI patients was less than 10 (19); it included only risk factor analysis and an incomplete ML model; any of the outcome parameters listed above were unavailable; it included only validation of a mature predictive model; or it was research on the accuracy of univariate factor prediction.
Search strategy
We searched the Web of Science, PubMed, Embase, and Cochrane Library databases from their inception to November 2022. We used Medical Subject Heading (MeSH) terms and text word terms related to NPC, ML, and radiotherapy were used for the literature search, with no language or region restrictions. The full search strategy is provided in Table S1.
Study selection
We imported the searched articles into Endnote and removed duplicates. After screening the titles and abstracts, we discarded studies that did not meet the criteria and considered the remaining studies as potentially eligible. We downloaded the full texts of these studies and evaluated them. Based on the inclusion/exclusion criteria, we either included or excluded the remaining studies. Two independent reviewers, Li Y and Guo Y carried out the selection procedure. Any disagreements between the reviewers were resolved by consensus or by a third reviewer, if required.
Data extraction
We generated a standardized table to record information from the literature, including the title, author, year of publication, country, type of study, duration of follow-up, treatment, number of total patients, number of RTLI patients, number of test/train patients, type of model, type of predictors, method of predictor selection, statistical outcomes, and outcome parameter. We extracted the data from the included studies according to this table. The process of data extraction was carried out independently by two reviewers, Li Y and Gong F, and any disagreements were resolved through consensus or with the involvement of a third reviewer if necessary.
Quality assessment
We used the Prediction model Risk Of Bias Assessment Tool (PROBAST) to assess the quality of the included records. PROBAST has four domains: participants, predictors, outcome, and analysis. The risk of bias in the study was evaluated through these domains, while the applicability was evaluated in the first three domains. Each domain contains 2, 3, 6, and 9 signaling questions, respectively. There were three possible answers to each question: Yes/Probably Yes, No/Probably No, and No information. A domain was judged to have a minimal risk of bias only if all responses to the questions were Yes/Probably Yes. The entire study was considered to have a low risk of bias only when all domains were rated as low risk of bias. If a domain had at least one question with the response No/Probably No, it was considered high risk. The entire study was considered to have a high risk of bias if at least one domain was considered high risk. The quality assessment process was carried out independently by two reviewers, Li Y and Gong F, with any disagreements resolved through consensus or with the involvement of a third reviewer if necessary.
Data synthesis and statistical analysis
The primary outcome of this review was the C-index. We also considered Sen, and Spe as the main outcomes, as the C-index alone may not be sufficient to express the predictive accuracy of ML when there is a difference in the number of individuals between the RTLI group and the non-RTLI group. We performed a meta-analysis based on the outcome parameter above. If both the 95% confidence interval (CI) and the standard error of the C-index were unavailable, we estimated the standard error using the formula (20). We used a random-effects model to conduct the meta-analysis based on the C-index given the differences in predictors used by each ML model. Furthermore, for the Sen and Spe meta-analysis, we utilized a bivariate mixed-effects model. Sensitivity analyses were conducted to evaluate the stability of the meta-analysis. Publication bias was evaluated by Egger’s test. We conducted these analyses using Stata 15.1 (StataCorp., College Station, TX, USA).
Results
Study selection
Figure 1 depicts the study selection process. Initially, we identified 699 unique records, and after assessing the titles and abstracts, we excluded 675 articles, leaving 24 for full-text review. Following a review of the full text of the papers, we excluded 10 studies (Table S2), primarily because they did not provide a complete ML model. Finally, our review finally included 14 studies (4,15,16,21-31).
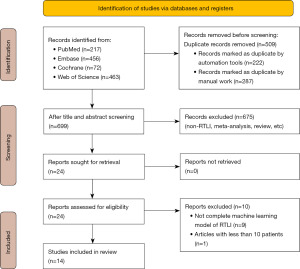
Characteristics of included studies
Our review included 8 cohort studies, 5 case-control studies, and 1 nested case-control study. All were single-center studies conducted in China. The included studies involved 15,573 NPC patients, with 2,267 (14.56%) of them identified as having RTLI. The authors, last author, publication year, center location, data source, patient inclusion and exclusion criteria, determined criteria of the outcome, time of predictor assessment, and time of outcome assessment of included studies are provided in available online: https://cdn.amegroups.cn/static/public/tcr-23-859-1.xlsx.
Characteristics of included prediction models
The included studies reported a total of 72 prediction models. These models were developed using 10 ML methods, including random forest (RF), Naïve Bayes (NB), k-nearest neighbors (KNN), Adaboost (AB), support vector machines (SVM), generalized linear regression (GLR), logistic regression (LR), Gradient Boosting Trees (GBT), Decision Tree (DT), and Cox proportional hazards (Cox). Radiomics was employed as one of the variables in 57 models, dosimetric predictors in 28 models, and clinical data in 27 models. Age, T/N/overall stage, and gender were the first three major clinical factors in the studies with clinical data models, accounting for 83.33%, 66.67%, and 33.33%, respectively.
Risk of bias assessment
Table S3 presents the results of the risk of bias assessment for each model in the included articles using the PROBAST tool. Figure 2 shows the proportion of models with different risks of bias for each domain. Overall, 94.44% of models were found to have a high risk of bias, primarily due to the high risk of bias in the analysis domain (81.94%). This was mainly because of the unreasonable number of participants (70.83%) or not accounting for model overfitting and optimism (9.72%) in model performance. Sensitivity analyses showed that the impact of models with a high risk of bias on this meta-analysis is acceptable (Figure S1). Egger’s test showed that there was a publication bias in included models (P<0.01).
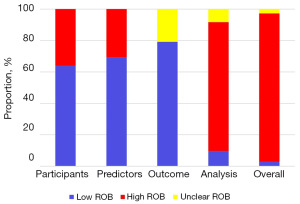
Meta-analysis
The included studies reported 48 models and 37 independent validation sets, with most models demonstrating good discrimination.
C-index
As shown in Table 1, the pooled C-index of the models was 0.769 (95% CI: 0.749–0.790) in the training set and 0.781 (95% CI: 0.754–0.808) in the validation set. The most common ML methods used to develop models were LR, SVM, and RF, all of which exhibited acceptable discrimination. For example, RF models performed well in predicting RTLI, with pooled C-index of 0.815 (95% CI: 0.781–0.850) in the training set, and 0.764 (95% CI: 0.710–0.818) in the validation set. We also investigated how models with different variables may perform. There were 31 models developed using radiomics data which was the most common variable in the included studies. The pooled C-index of these models was 0.745 (95% CI: 0.716–0.774) in the training set and 0.812 (95% CI: 0.766–0.859) in the validation set. The best-performing models were those established using both radiomics data and clinical data with a pooled C-index of 0.895 (95% CI: 0.860–0.930) in the training set and 0.880 (95% CI: 0.810–0.950) in the validation set (Table 1). Figure S2 showed the forest plots of meta-analysis. Sensitivity analyses excluding any one model included in this meta-analysis yielded results that were consistent with the primary analysis (Figure S1).
Table 1
Subgroup | Training set | Validation set | |||
---|---|---|---|---|---|
Number | C-index | Number | C-index | ||
ML method type | |||||
RF | 7 | 0.815 (0.781–0.850) | 7 | 0.764 (0.710–0.818) | |
SVM | 8 | 0.756 (0.668–0.844) | 7 | 0.898 (0.843–0.953) | |
Cox | 6 | 0.793 (0.763–0.823) | 2 | 0.753 (0.730–0.775) | |
LR | 14 | 0.810 (0.773–0.847) | 17 | 0.780 (0.725–0.835) | |
AB | 3 | 0.687 (0.580–0.794) | |||
KNN | 4 | 0.649 (0.509–0.789) | 4 | 0.608 (0.411–0.805) | |
GLR | 3 | 0.562 (0.510–0.613) | |||
NB | 1 | 0.83 | |||
DT | 1 | 0.72 | |||
GBT | 1 | 0.88 | |||
Variable type | |||||
R | 31 | 0.745 (0.716–0.774) | 7 | 0.812 (0.766–0.859) | |
D | 5 | 0.781 (0.741–0.821) | 3 | 0.728 (0.707–0.748) | |
C+D | 5 | 0.791 (0.759–0.822) | 4 | 0.776 (0.749–0.802) | |
C | 1 | 0.74 | 2 | 0.705 (0.640–0.770) | |
R+C | 2 | 0.895 (0.860–0.930) | 2 | 0.880 (0.810–0.950) | |
R+C+D | 4 | 0.827 (0.805–0.849) | 19 | 0.768 (0.721–0.815) | |
Overall | 48 | 0.769 (0.749–0.790) | 37 | 0.781 (0.754–0.808) |
C-index, concordance index; ML, machine learning; RF, random forest; SVM, support vector machines; Cox, Cox proportional hazards; LR, logistic regression; AB, AdaBoost; KNN, k-nearest neighbors; GLR, generalized linear regression; NB, Naïve Bayes; DT, Decision Tree; GBT, Gradient Boosting Trees; R, radiomics; D, dosimetric predictors; C, clinical data.
Sen and Spe
As shown in Table 2, the summary of Sen was 0.75 (95% CI: 0.69–0.80) in the training set and 0.70 (95% CI: 0.66–0.73) in the validation set. Additionally, the summary of Spe was 0.78 (95% CI: 0.73–0.82) in the training set and 0.79 (95% CI: 0.75–0.82) in the validation set. Among models developed using different ML methods, those developed using SVM showed the highest Sen (pooled Sen in the training set 0.80, 95% CI: 0.70–0.87; pooled Sen in the validation set 0.72, 95% CI: 0.57–0.83), while those developed using RF showed the highest Spe (pooled Spe in the training set 0.83, 95% CI: 0.63–0.94; pooled Spe in the validation set 0.76, 95% CI: 0.68–0.82). Among models developed using different variables, those established using radiomics data, clinical data, and dosimetric predictors showed the highest Sen (pooled Sen in the training set 0.78, 95% CI: 0.71–0.83; pooled Sen in the validation set 0.71, 95% CI: 0.62–0.79), while those established using both radiomics data and clinical data showed the highest Spe (pooled Spe in the training set 0.88, 95% CI: 0.78–0.98; pooled Spe in the validation set 0.90, 95% CI: 0.84–0.96).
Table 2
Subgroup | Training set | Validation set | |||||
---|---|---|---|---|---|---|---|
Number | Sen | Spe | Number | Sen | Spe | ||
ML method type | |||||||
RF | 4 | 0.63 (0.30–0.87) | 0.83 (0.63–0.94) | 7 | 0.67 (0.58–0.74) | 0.76 (0.68–0.82) | |
SVM | 5 | 0.80 (0.70–0.87) | 0.80 (0.65–0.89) | 7 | 0.72 (0.57–0.83) | 0.81 (0.72–0.87) | |
LR | 14 | 0.73 (0.67–0.78) | 0.78 (0.72–0.83) | 16 | 0.70 (0.65–0.75) | 0.78 (0.73–0.83) | |
KNN | 1 | 0.950 | 0.690 | 3 | 0.59 (0.42–0.75) | 0.84 (0.75–0.90) | |
NB | 1 | 0.800 | 0.690 | ||||
DT | 1 | 0.770 | 0.650 | ||||
GBT | 1 | 0.840 | 0.780 | ||||
Variable type | |||||||
R | 15 | 0.75 (0.64–0.83) | 0.79 (0.72–0.85) | 7 | 0.70 (0.63–0.76) | 0.78 (0.65–0.87) | |
D | 3 | 0.78 (0.67–0.86) | 0.66 (0.62–0.71) | 2 | 0.57 (0.46–0.66) | 0.74 (0.72–0.77) | |
C+D | 3 | 0.72 (0.62–0.80) | 0.72 (0.69–0.75) | 3 | 0.68 (0.59–0.76) | 0.76 (0.70–0.80) | |
C | 1 | 0.590 | 0.870 | 1 | 0.59 | 0.71 | |
R+C | 2 | 0.75 (0.72–0.79) | 0.88 (0.78–0.98) | 2 | 0.76 (0.71–0.81) | 0.90 (0.84–0.96) | |
R+C+D | 3 | 0.78 (0.71–0.83) | 0.75 (0.69–0.80) | 18 | 0.71 (0.62–0.79) | 0.79 (0.73–0.83) | |
Overall | 27 | 0.75 (0.69–0.80) | 0.78 (0.73–0.82) | 33 | 0.70 (0.66–0.73) | 0.79 (0.75–0.82) |
Sen, sensitivity; Spe, specificity; ML, machine learning; RF, random forest; SVM, support vector machines; LR, logistic regression; KNN, k-nearest neighbors; NB, Naïve Bayes; DT, Decision Tree; GBT, Gradient Boosting Trees; R, radiomics; D, dosimetric predictors; C, clinical data.
Discussion
The meta-analysis results indicate that ML is a robust technique for predicting RTLI, with radiomics playing a crucial role as a predictor. LR, SVM, and RF were the most common ML methods used to develop models and all performed well in predicting RTLI, which provided a solution for NPC patients that the radiation dose could be reduced individually at the early stage in the patients with high predicted risk of RTLI. Models developed with both radiomics and clinical features demonstrated the best discrimination, with age and T/N/overall stage being the most commonly used clinical features. These findings can serve as a reference for future research in this area.
The radical treatment for NPC always involves the delivery of radiotherapy but RTLI is a severe late complication that can cause irreversible emotional and cognitive impairment. Medications such as bevacizumab and corticosteroids can be administered for RTLI to alleviate symptoms and prevent further deterioration (32,33). More importantly, researchers have focused on adjusting radiation doses to find the most appropriate dose to prevent RTLI, using the “as low as reasonably practicable” principle (34). However, inconsistent results have been reported, with some identifying D1cc as the most important predictor and a tolerance dose of 62.8 Gy (8,9), while others found D2cc (10) and D0.5cc (35) to be the best predictor. Given the inconsistent findings and differentiated actual tolerance dose of each person, it is challenging to recommend a tolerance dose. Additionally, the proximity of targets to organs at risk involves a delicate balancing act that involves tumor coverage and normal tissue. Therefore, more studies are focusing on predicting RTLI, enabling clinicians to estimate the risk before radiotherapy and make individualized radiation plans or adjust the dose in a timely manner.
Radiomics was viewed as a valuable feature that should be explored for use in developing RTLI prediction models. It has been frequently employed in radiation-induced toxicity prediction models and has outperformed clinical and dosimetric parameters in predicting toxicities such as xerostomia in NPC patients, cardiac toxicity in breast cancer patients, and lung damage in esophageal cancer patients after radiotherapy (36). As expected, radiomics performed well in predicting RTLI as it can detect microstructural changes in the temporal lobe early (11), enhancing prediction accuracy, as seen in the meta-analysis for each subgroup above. ML methods are required to process high-dimensional mineable data like radiomics, and these methods have been shown to play a crucial part in predictive models based on radiomics and standard clinical characteristics, such as predicting rapidly deteriorating mild cognitive impairment in Alzheimer’s disease (13) and the prognosis of acute ischemic stroke (12). LR is the most often used ML approach for building models due to its ease of use and consistently good performance. However, to determine the best-performing model, comparisons of various ML methods based on the same dataset should be made (15,23,24). As indicated in the meta-analysis above, SVM appears to have the highest performance, particularly in the validation set, although further comparison studies are needed to confirm this finding.
In addition, age and T/N/overall stage were shown to be major clinical predictors in several studies (4,24), emphasizing the need for paying attention to the RTLI risk of elderly or have advanced-stage NPC patients and developing specific radiation strategies for them. Elderly patients may have a lower tolerance dose due to their susceptibility to cerebrovascular injury, which significantly affects RTLI (37). Moreover, patients with advanced-stage NPC are treated with higher doses in a wider area to control tumors, resulting in more damage to normal brain tissue (5,34).
The greatest strength of our study is its originality. This is the first study to present evidence-based data for ML models predicting RTLI in NPC patients after radiotherapy. Besides, the quality of this meta-analysis was high based on AMSTAR2 (Appendix 1). Nevertheless, this research has several limitations. First, although we conducted a comprehensive search, the number of included studies was relatively limited and all included studies were conducted in China, due to the geographical distribution of the disease. Second, since only a few models were included, further research is required to design and identify the most optimal ML model for predicting RTLI. Third, there was a publication bias in included models, which may cause the overestimation of prediction accuracy of them. Fourth, it would be better to verify if the SVM is the ML method with highest predictive performance in more clinical experimental research.
Conclusions
Our study demonstrated that ML methods can effectively predict RTLI in NPC patients after radiotherapy. While the primary goal of radiotherapy is to maximize survival with the appropriate radiation dose, stratifying the risk of RTLI through ML can help adjust personalized radiation doses for NPC patients. Therefore, more multicenter and multi-ethnic studies are necessary to develop tools for predicting RTLI, particularly ML models with radiomics, to prevent or mitigate it by timely adjusting the treatment.
Acknowledgments
Funding: This study was supported by
Footnote
Reporting Checklist: The authors have completed the PRISMA reporting checklist. Available at https://tcr.amegroups.com/article/view/10.21037/tcr-23-859/rc
Peer Review File: Available at https://tcr.amegroups.com/article/view/10.21037/tcr-23-859/prf
Conflicts of Interest: All authors have completed the ICMJE uniform disclosure form (available at https://tcr.amegroups.com/article/view/10.21037/tcr-23-859/coif). WTN reports funding support from the Shenzhen Key Laboratory for cancer metastasis and personalized therapy (No. ZDSYS20210623091811035) and the Shenzhen Fundamental Research Program, China (No. CYJ20210324114404013). The other authors have no conflicts of interest to declare.
Ethical Statement: The authors are accountable for all aspects of the work in ensuring that questions related to the accuracy or integrity of any part of the work are appropriately investigated and resolved.
Open Access Statement: This is an Open Access article distributed in accordance with the Creative Commons Attribution-NonCommercial-NoDerivs 4.0 International License (CC BY-NC-ND 4.0), which permits the non-commercial replication and distribution of the article with the strict proviso that no changes or edits are made and the original work is properly cited (including links to both the formal publication through the relevant DOI and the license). See: https://creativecommons.org/licenses/by-nc-nd/4.0/.
References
- Bray F, Ferlay J, Soerjomataram I, et al. Global cancer statistics 2018: GLOBOCAN estimates of incidence and mortality worldwide for 36 cancers in 185 countries. CA Cancer J Clin 2018;68:394-424. [Crossref] [PubMed]
- Chen YP, Chan ATC, Le QT, et al. Nasopharyngeal carcinoma. Lancet 2019;394:64-80. [Crossref] [PubMed]
- Ou D, Blanchard P, El Khoury C, et al. Induction chemotherapy with docetaxel, cisplatin and fluorouracil followed by concurrent chemoradiotherapy or chemoradiotherapy alone in locally advanced non-endemic nasopharyngeal carcinoma. Oral Oncol 2016;62:114-21. [Crossref] [PubMed]
- Wen DW, Lin L, Mao YP, et al. Normal tissue complication probability (NTCP) models for predicting temporal lobe injury after intensity-modulated radiotherapy in nasopharyngeal carcinoma: A large registry-based retrospective study from China. Radiother Oncol 2021;157:99-105. [Crossref] [PubMed]
- Su SF, Huang Y, Xiao WW, et al. Clinical and dosimetric characteristics of temporal lobe injury following intensity modulated radiotherapy of nasopharyngeal carcinoma. Radiother Oncol 2012;104:312-6. [Crossref] [PubMed]
- Chen W, Qiu S, Li J, et al. Diffusion tensor imaging study on radiation-induced brain injury in nasopharyngeal carcinoma during and after radiotherapy. Tumori 2015;101:487-90. [Crossref] [PubMed]
- Su SF, Huang SM, Han F, et al. Analysis of dosimetric factors associated with temporal lobe necrosis (TLN) in patients with nasopharyngeal carcinoma (NPC) after intensity modulated radiotherapy. Radiat Oncol 2013;8:17. [Crossref] [PubMed]
- Kong C, Zhu XZ, Lee TF, et al. LASSO-based NTCP model for radiation-induced temporal lobe injury developing after intensity-modulated radiotherapy of nasopharyngeal carcinoma. Sci Rep 2016;6:26378. [Crossref] [PubMed]
- Zeng L, Huang SM, Tian YM, et al. Normal Tissue Complication Probability Model for Radiation-induced Temporal Lobe Injury after Intensity-modulated Radiation Therapy for Nasopharyngeal Carcinoma. Radiology 2015;276:243-9. [Crossref] [PubMed]
- Feng M, Huang Y, Fan X, et al. Prognostic variables for temporal lobe injury after intensity modulated-radiotherapy of nasopharyngeal carcinoma. Cancer Med 2018;7:557-64. [Crossref] [PubMed]
- Gillies RJ, Kinahan PE, Hricak H. Radiomics: Images Are More than Pictures, They Are Data. Radiology 2016;278:563-77. [Crossref] [PubMed]
- Wang X, Lyu J, Meng Z, et al. Small vessel disease burden predicts functional outcomes in patients with acute ischemic stroke using machine learning. CNS Neurosci Ther 2023;29:1024-33. [Crossref] [PubMed]
- Zhao X, Sui H, Yan C, et al. Machine-Based Learning Shifting to Prediction Model of Deteriorative MCI Due to Alzheimer's Disease - A Two-Year Follow-Up Investigation. Curr Alzheimer Res 2022;19:708-15. [Crossref] [PubMed]
- Wang Y, He Y, Duan X, et al. Construction of diagnostic and prognostic models based on gene signatures of nasopharyngeal carcinoma by machine learning methods. Transl Cancer Res 2023;12:1254-69. [Crossref] [PubMed]
- Lin X, Li Z, Chen S, et al. Divergent white matter changes in patients with nasopharyngeal carcinoma post-radiotherapy with different outcomes: a potential biomarker for prediction of radiation necrosis. Eur Radiol 2022;32:7036-47. [Crossref] [PubMed]
- Zhao LM, Kang YF, Gao JM, et al. Functional Connectivity Density for Radiation Encephalopathy Prediction in Nasopharyngeal Carcinoma. Front Oncol 2021;11:687127. [Crossref] [PubMed]
- Page MJ, McKenzie JE, Bossuyt PM, et al. The PRISMA 2020 statement: An updated guideline for reporting systematic reviews. PLoS Med 2021;18:e1003583. [Crossref] [PubMed]
- Shea BJ, Reeves BC, Wells G, et al. AMSTAR 2: a critical appraisal tool for systematic reviews that include randomised or non-randomised studies of healthcare interventions, or both. BMJ 2017;358:j4008. [Crossref] [PubMed]
- Riley RD, Ensor J, Snell KIE, et al. Calculating the sample size required for developing a clinical prediction model. BMJ 2020;368:m441. [Crossref] [PubMed]
- Debray TP, Damen JA, Riley RD, et al. A framework for meta-analysis of prediction model studies with binary and time-to-event outcomes. Stat Methods Med Res 2019;28:2768-86. [Crossref] [PubMed]
- Zhang B, Lian Z, Zhong L, et al. Machine-learning based MRI radiomics models for early detection of radiation-induced brain injury in nasopharyngeal carcinoma. BMC Cancer 2020;20:502. [Crossref] [PubMed]
- Bao D, Zhao Y, Li L, et al. A MRI-based radiomics model predicting radiation-induced temporal lobe injury in nasopharyngeal carcinoma. Eur Radiol 2022;32:6910-21. [Crossref] [PubMed]
- Zhong L, Zhang X, Xi Y, et al. Deep Longitudinal Feature Representations for Detection of Postradiotherapy Brain Injury at Presymptomatic Stage. IEEE Access 2020;8:184710-21. [Crossref]
- Bin X, Zhu C, Tang Y, et al. Nomogram Based on Clinical and Radiomics Data for Predicting Radiation-induced Temporal Lobe Injury in Patients with Non-metastatic Stage T4 Nasopharyngeal Carcinoma. Clin Oncol (R Coll Radiol) 2022;34:e482-92. [Crossref] [PubMed]
- Guan W, Xie K, Fan Y, et al. Development and Validation of a Nomogram for Predicting Radiation-Induced Temporal Lobe Injury in Nasopharyngeal Carcinoma. Front Oncol 2020;10:594494. [Crossref] [PubMed]
- Hou J, Li H, Zeng B, et al. MRI-based radiomics nomogram for predicting temporal lobe injury after radiotherapy in nasopharyngeal carcinoma. Eur Radiol 2022;32:1106-14. [Crossref] [PubMed]
- Fang J, Li A, Ouyang PY, et al. Weighted Concordance Index Loss-Based Multimodal Survival Modeling for Radiation Encephalopathy Assessment in Nasopharyngeal Carcinoma Radiotherapy. Springer, Cham; 2022.
- Wang J, Miao Y, Ou X, et al. Development and validation of a model for temporal lobe necrosis for nasopharyngeal carcinoma patients with intensity modulated radiation therapy. Radiat Oncol 2019;14:42. [Crossref] [PubMed]
- Zhang YM, Kang YF, Zeng JJ, et al. Surface-Based Falff: A Potential Novel Biomarker for Prediction of Radiation Encephalopathy in Patients With Nasopharyngeal Carcinoma. Front Neurosci 2021;15:692575. [Crossref] [PubMed]
- Kang YF, Chen RT, Ding H, et al. Structure-Function Decoupling: A Novel Perspective for Understanding the Radiation-Induced Brain Injury in Patients With Nasopharyngeal Carcinoma. Front Neurosci 2022;16:915164. [Crossref] [PubMed]
- Du QH, Gan YX, Wang RS, et al. Half-Brain Delineation for Prediction of Radiation-Induced Temporal Lobe Injury in Nasopharyngeal Carcinoma Receiving Intensity-Modulated Radiotherapy. Front Oncol 2021;11:599942. [Crossref] [PubMed]
- Leavitt RJ, Limoli CL, Baulch JE. miRNA-based therapeutic potential of stem cell-derived extracellular vesicles: a safe cell-free treatment to ameliorate radiation-induced brain injury. Int J Radiat Biol 2019;95:427-35. [Crossref] [PubMed]
- Xu Y, Rong X, Hu W, et al. Bevacizumab Monotherapy Reduces Radiation-induced Brain Necrosis in Nasopharyngeal Carcinoma Patients: A Randomized Controlled Trial. Int J Radiat Oncol Biol Phys 2018;101:1087-95. [Crossref] [PubMed]
- Lee AW, Ng WT, Pan JJ, et al. International Guideline on Dose Prioritization and Acceptance Criteria in Radiation Therapy Planning for Nasopharyngeal Carcinoma. Int J Radiat Oncol Biol Phys 2019;105:567-80. [Crossref] [PubMed]
- Sun Y, Zhou GQ, Qi ZY, et al. Radiation-induced temporal lobe injury after intensity modulated radiotherapy in nasopharyngeal carcinoma patients: a dose-volume-outcome analysis. BMC Cancer 2013;13:397. [Crossref] [PubMed]
- Desideri I, Loi M, Francolini G, et al. Application of Radiomics for the Prediction of Radiation-Induced Toxicity in the IMRT Era: Current State-of-the-Art. Front Oncol 2020;10:1708. [Crossref] [PubMed]
- Smart D. Radiation Toxicity in the Central Nervous System: Mechanisms and Strategies for Injury Reduction. Semin Radiat Oncol 2017;27:332-9. [Crossref] [PubMed]
(English Language Editor: J. Jones)