Development and verification of a newly established cuproptosis-associated lncRNA model for predicting overall survival in uterine corpus endometrial carcinoma
Highlight box
Key findings
• A predictive prognosis model based on cuproptosis-associated lncRNA was established to predict overall survival in uterine corpus endometrial carcinoma.
What is known and what is new?
• Cuproptosis-related lncRNA models are regarded as the novel signature for predicting prognosis and showing therapeutic value for various cancers, but few studies were conducted to explore the cuproptosis-related lncRNA signature for the prognosis of uterine corpus endometrial carcinoma.
• A novel prognosis model based on cuproptosis-related lncRNA of uterine corpus endometrial carcinoma has been successfully developed and verified.
What is the implication, and what should change now?
• This model could be used as a tool for predicting overall survival in uterine corpus endometrial carcinoma, providing new ideas for immune therapy of uterine corpus endometrial carcinoma.
Introduction
Uterine corpus endometrial carcinoma (UCEC) is one of the most prevalent malignant tumors in developed countries, with more than 50,000 deaths each year (1). As the population ages and environmental pollution increases, the morbidity and mortality rates of UCEC rise as well. Factors such as age, race, and history of prior malignancy are strongly correlated with the onset of UCEC (2,3). Although a variety of treatment options are available, such as surgery, radiotherapy, and chemotherapy, UCEC is still prone to metastasis and recrudesce (4). RNA-based therapeutics have recently been found to be an important strategy for combating cancer through neoantigens of RNA vaccines, RNA immune regulation, and RNA interference (5). Long non-coding RNAs (lncRNAs) are composed of more than 200 nucleotides, shown to be increasingly involved in various malignant cancer progression (6). LncRNAs were found to be involved in UCEC cell proliferation, invasion, and metabolic changes by targeting miRNAs axis (7). Experimental study has suggested that LINC00958 regulates the function of IGF2BP3, thus participating in the tumorigenesis and progression of UCEC (8). Given those critical features, lncRNAs have progressively become novel diagnostic and prognostic biomarkers for UCEC.
Copper metabolism, which accounts for mitochondrial respiration, antioxidant effect, and anabolic functions, is one of the most important activities for maintaining healthy cells (9). Recent study has shown that excessive intracellular copper can mediate the regulation of the mitochondrial tricarboxylic acid (TCA) cycle, resulting in cell death, also known as cuproptosis (10). Cuproptosis, distinct from apoptosis and ferroptosis, is a newly discovered form of programmed cell death. A study has shown that cuproptosis plays a vital role in the modulation of UCEC microenvironment, genetic mutation, and response to immune therapy (11). Cuproptosis-related lncRNA models are regarded as the novel signature for predicting prognosis and showing therapeutic value for various cancers, such as lung (12) and cervical cancer (13). Although a study has shown that the lncRNA-related signature was substantially associated with the overall survival (OS) of patients with UCEC (14), few studies were conducted to explore the cuproptosis-related lncRNA signature for the prognosis of UCEC. Therefore, this study was designed to construct cuproptosis-related lncRNAs biomarkers, thus providing new strategies for the diagnosis, targeted immune treatment, and prevention of UCEC.
In this study, we developed a cuproptosis-related lncRNA model for determining the prognosis of UCEC based on The Cancer Genome Atlas (TCGA) database (https://portal.gdc.cancer.gov/). Additionally, the sensitivity, specificity, and accuracy of the proposed model were evaluated. Finally, we delved into the categories of functional enrichment, immune regulation, and drug sensitivity for a deeper understanding of the model. We present this article in accordance with the TRIPOD reporting checklist (available at https://tcr.amegroups.com/article/view/10.21037/tcr-23-61/rc).
Methods
Data acquisition and processing
On July 15, 2022, the transcriptome sequencing data in fragments per kilobase per million (FPKM) format and corresponding clinical information of the UCEC dataset were downloaded from TCGA using ‘TCGAbiolinks’ R package (15). A total of 589 samples (35 normal samples and 554 tumor samples) tumor samples were included in the follow-up analysis after excluding samples without complete lncRNA expression levels. Then, according to the annotation information, messenger RNA (mRNA) and lncRNA expression matrixes were processed using ‘SummarizedExperiment’ R package (16). Finally, the clinical data including age, race, stage, history of prior malignancy, survival status and survival time were extracted from clinical files, and the UCEC prognosis was determined by the survival duration and status of the patients. The study was conducted in accordance with the Declaration of Helsinki (as revised in 2013).
Co-expression analysis
On the basis of a previous study, 12 cuproptosis-related genes (FDX1, LIAS, LIPT1, DLD, DLAT, PDHA1, PDHB, MTF1, GLS, CDKN2A, SLC31A1, and ATP7B) were addressed as cuproptosis-related genes (17). In the study, ‘limma’ R package was utilized to collect cuproptosis-related genes and lncRNA expression profiles. Next, a correlation analysis between genes and lncRNAs was performed to obtain the cuproptosis-related lncRNA with the screening criteria of coefficient (R) >0.2 and P<0.05 (18). Finally, the co-expression results were visualized in Sankey plots by using ‘ggplot2’ R package.
Development of a cuproptosis-related lncRNA signature
In order to obtain unbiased results, clinical data was matched with cuproptosis-related lncRNAs and then randomly and equally divided into two groups (Train and Test) with ‘caret’ R package (19). The clinical characteristic of UCEC patients including age, race, stage, and history of prior malignancy were extracted from clinical information, and chi-square test was applied to make a statistic comparison between All, Train, and Test group. In the study, the Train group was used to construct a prognosis model, and the Test group was used to validate the prediction. In model construction, univariate proportional hazards model (Cox) analysis was performed to screen out the survival-related lncRNAs with ‘survival’ R package (20). Then, least absolute shrinkage and selection operator (LASSO) regression with tenfold cross-validation was employed to minimize the errors for the construction of an ideal risk model (21). The risk score calculation formula was the sum of coefficient × x (x is the FPKM value of each cuproptosis-related lncRNA) (22). At the meantime, a correlation analysis was conducted to explore the relationship between lncRNAs and cuproptosis genes in the model, and the outcome was presented in a plot using ‘ggplot2’ R package. Based on the median risk score, UCEC patients were divided into low-risk and high-risk groups to establish the foundation for further analyses.
Model verification and evaluation
To verify our model performance, lncRNA expression, heatmap, survival analysis curve, risk score, and survival status plots were generated, for different groups (All, Train, and Test). Furthermore, to comprehensively evaluate the specificity of our prognostic model, clinical traits (stage, prior malignancy, race, and age) were extracted from the clinical files. Correspondingly, the race was defined as follows: number 1 represented American Indian or Alaska Native race, number 2 represented Asian race, number 3 represented Black or African American race, number 4 represented Native Hawaiian or other Pacific Islander race, number 5 represented the White race. Next, clinical traits were combined with risk scores to screen out the significant independent factors in univariate and multivariate Cox regression analysis (P<0.05). By using the statistical software R, a receiver operating characteristic (ROC) curve was plotted, and the area under the curve (AUC) was calculated (23). The AUC value over 0.7 indicates a good predictive value (24). To intuitively present the effect sizes of all factors, ‘regplot’ and ‘rms’ R packages were used for the construction of calibration and Nomogram plots with a combination of clinical characteristics and risk scores (25). The C-index value of 0.7 is indicative of a good discrimination ability, according to a previous study (26). For an improved assessment of the model function, data from all genes, cuproptosis-related genes, cuproptosis-related lncRNAs, and risk-related lncRNAs were analyzed using a principal component analysis (PCA) to provide further discrimination between patients with UCEC. Additionally, in the light of the stage status in the tumor classification system, we performed survival analysis in stage I–II and stage III–IV groups, respectively.
Gene Ontology (GO) enrichment and immune-related function analysis
To interpret the potential different biological functions between the low-risk and high-risk groups, risk scores were merged with the mRNAs expression matrix; differential expression analysis was then performed with ‘limma’ R package according to the inclusion criteria [false discovery rate (FDR) <0.05 and |log2 fold change| >1]. Subsequently, GO functional enrichment was plotted using the packages ‘stringi’, ‘ggplot2’, ‘circlize’, ‘RColorBrewer’, and ‘ggpubr’; GO enrichment analyses were visualized in all categories: biological process (BP), molecular function (MF), and cellular compartment (CC) (27). GO terms with FDR <0.05 were considered significantly enriched (28).
To understand and explore the immune function in the cuproptosis-related lncRNA model, a single sample gene set enrichment analysis (ssGSEA) analysis was performed between low-risk and high-risk groups using ‘GSVA’ package in R (29). Subsequently, ssGSEA scores were exported and presented in a heatmap using ‘pheatmap’ R package.
Tumor mutation burden (TMB) analysis of the model
TMB analysis between low-risk and high-risk groups was then conducted to reveal their inner connections. First, the mutation data of TCGA-UCEC was downloaded from TCGA genomic data commons and processed by Perl (version 32) (30). Next, TMB files were classified into low-risk or high-risk groups, as defined by the cutoff of the median risk score. To ease the presentation of mutation data, ‘maftools’ R package was used for the aggregation and visualization of TMB in each group, and the top 15 mutated genes were displayed using waterfall plots (31). By calculating the total number of TMBs in low-risk and high-risk groups, the differences between the two groups were compared. At the meantime, to illustrate the importance of the risk model and TMB in UCEC, we conducted a survival analysis using Kaplan-Meier (KM) with ‘survival’ R package.
Drug sensitivity prediction
For the analysis of drug sensitivity prediction, R packages ‘pRRophetic’, ‘ggpubr’, ‘limma’ were installed (32). Afterwards, the mRNAs matrix was mapped to the risk scores and the expression relationship between risk groups, and drug half maximal inhibitory concentration (IC50) values were acquired based on the sensitivity assessment. Results were considered as statistically significant when P<0.001, and then boxplots were generated using ‘ggplot2’ R package.
Statistical analysis
All statistical analyses and plots were conducted using R software (version 4.1.3). The count data including age, race, stage, and history of prior malignancy were expressed by n (%), and chi-square test was applied to make a statistic comparison between All, Train, and Test group. Univariate and LASSO regression analyses were applied to construct an ideal cuproptosis-related lncRNA prognostic model for UCEC. Univariate and multivariate Cox regression analyses were performed to assess the independent prognostic value of the risk score. ROC curve and KM method were used to evaluate the performance of the cuproptosis-related model. P<0.05 was considered as the threshold for significance.
Results
Cuproptosis-related lncRNAs in UCEC were successfully obtained
To visualize and have a good understanding of this study, a flowchart which depicted the whole process of our analysis was first introduced (Figure 1A). Following successful downloading of UCEC data, cuproptosis gene expressions data were obtained based on the cuproptosis gene dataset. To get the cuproptosis-related lncRNAs, we conducted a correlation analysis between cuproptosis gene expression and lncRNA expression. Finally, 155 lncRNAs that met the criteria were identified as cuproptosis-related lncRNAs (|R| >0.2 and P<0.05) (Table S1), and the correlation results were shown in a Sankey diagram (Figure 1B).
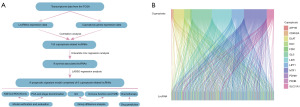
A robust prognostic model based on cuproptosis-related lncRNA was constructed
In this part of study, the dataset was randomly allocated to the Train group and Test group, and clinical characteristics were summarized in a table (Table S2). The result showed that no substantial difference was found in across the Train and Test groups, which reflected a well-balanced baseline characteristic. Subsequently, we used the Train set to develop an ideal model to predict the prognosis of UCEC. First, univariate Cox regression analysis was performed to select prognosis-related lncRNAs; 9 lncRNAs closely associated with the UCEC patient’s outcomes were then included in our study (P<0.05). The results of the 9 lncRNAs Cox regression analyses were represented in a forest plot (Figure 2A). Subsequently, 9 lncRNAs were evaluated by LASSO regression analysis (Figure 2B,2C). As a result, to make a prediction of the prognosis in UCEC patients, we successfully developed a computational model consisting of 6 lncRNAs. The risk score formula was as follows: Risk scores = (AC073046.1 × 0.655) + (AC108479.1 × 1.359) + (ASH1L-AS1 × 0.728) + (LINC01644 × 0.932) − (AL132639.2 × 2.001) − (SBF2-AS1 × 1.446). Simultaneously, the potential associations between the model lncRNA and cuproptosis gene expression were explored (Figure 2D). Based on the correlation analysis results, it could be found that SLC31A1, MTF1, DLAT genes were highly linked with the lncRNA model; meanwhile, AC073046.1 and AC108479.1 lncRNAs were nearly able to maintain a good correlation with all cuproptosis-related genes, reflecting the potential features of the pathogenesis in UCEC.
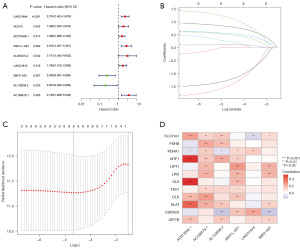
Train set and Test set were validated for model evaluation
In order to test the accuracy of the model, evaluation analysis was performed in groups (All, Train, Test). Based on the median of the risk scores, patients were divided into low-risk and high-risk groups. The heatmap of the All group showed that high-risk group lncRNAs exhibited an upward expression trend, except for SBF2-AS1 and AL132639.2 (Figure 3A). When compared with the low-risk group, the high-risk group occurred with a lower OS (Figure 3B). In contrast to low-risk group, patients in the high-risk group tended to have an even higher risk score (Figure 3C). In addition, the survival status plots showed that patients with high-risk score had a dismal prognosis accompanied with an increased number of deaths (Figure 3D). Additionally, to validate the aforementioned results, the same analysis was performed in the other two subgroups, and the same results were also verified in Train (Figure 3E-3H) and Test groups (Figure 3I-3L).
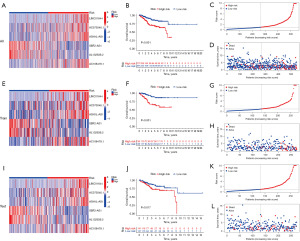
The risk model might be used as a novel independent prognosis biomarker
To investigate the prognostic value of the risk model, we first performed a univariate Cox regression analysis, and the results indicated that stage, age, and risk score could be applied as independent prognosis biomarkers for UCEC (Figure 4A). At the meantime, the hazard ratio of those biomarkers was greater than 1, which implied a positively correlation with poor prognosis (P<0.05). Next, a multivariate Cox regression analysis was conducted to separate the mutual conflicting effects of biomarkers; again, the same results were found (P<0.05) (Figure 4B).
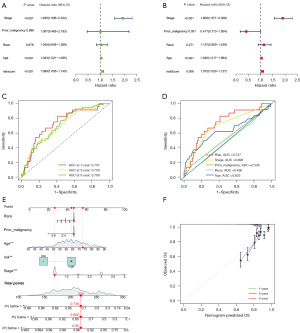
To further test the robustness of the risk model, we then conducted a sensitivity and specificity analysis. The model ROC curve suggested that 1/3/5 years AUC were 0.737/0.700/0.709 (>0.7), which portended a good predictive performance (Figure 4C). Similarly, ROC analysis was applied to overall clinical traits and risk score, and the curve indicated a high significant AUC in risk score (0.737) and stage (0.698), while AUC of age was 0.633 (Figure 4D).
Age and race were established as risk factors for the development of UCEC, with only 4% of patients with UCEC being younger than 40 years old (33). To provide a comprehensive evaluation of clinical traits and risk score function in the prediction of UCEC prognosis, a nomogram (C-index =0.755) was plotted to achieve personalized accurate survival probability prediction (Figure 4E). In the nomogram, for example, a female patient (ID: TCGA-B5-A3FA) aged 73 years, White race, no prior malignancy history, cancer stage I, and high-risk score had a total point of 220 points, corresponding to 1/3/5 years survival rate at 0.95/0.809/0.736. Based on the information obtained from the clinical files, the patient’s 5-year survival status was alive (survival time =2,929 days), which was compatible with the outcome predicted by the nomogram. The calibration plot showed that the curves at 1/3/5 years were close to the diagonal line, which represented an ideal situation when the predicted OS would be identical to the observed OS, indicating a great performance of the nomogram (Figure 4F).
Based on the previous part of the study, the accuracy and specificity were fully addressed. To further explore the function of the model, PCA and stage analyses were employed. In PCA plots, no distinct boundary between low-risk and high-risk patients could be identified in any patterns except risk-related lncRNAs (Figure 5A-5D). Next, compared with the patients in the low-risk group, UCEC patients in the high-risk group had significantly poor OS, either in stage I–II (Figure 5E) or stage III–IV (Figure 5F).
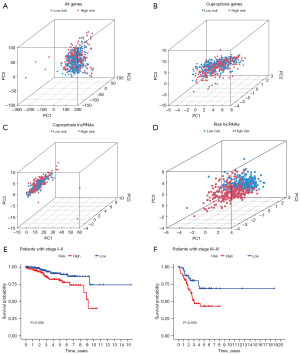
GO enrichment and immune function analyses of the model
By using FDR <0.05 as screening criteria, 7 GO terms were enriched and visualized in a circle (Figure 6A) and bar plot (Figure 6B). From these results, it could be found that MF enrichment took a dominant part in GO activities. In addition, neuropeptide hormone activity (GO:0005184) was the first ranked term according to the percentage. In CC enrichment, only the anchored component of the membrane term (GO:0031225) was filtered. When it came to BP enrichment, extracellular structure organization (GO:0043062) and extracellular matrix organization (GO:0030198) were identified.
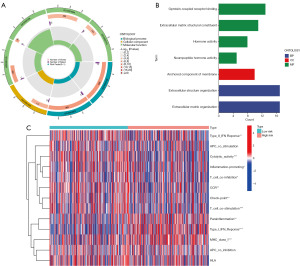
Taking a step further, the GO analysis provided notable findings, which showed GO enrichment results were highly correlated with cellular recognition and hormone modulation. Therefore, for a better interpretation of that difference, we performed an immune function analysis between low-risk and high-risk groups, and the result was presented in a heatmap (Figure 6C). The immune function results exhibited that, when compared to the low-risk group, type II interferon (IFN), cytolytic activity, inflammation promoting, T cell co-inhibition, chemokine receptor (CCR), check-point, and T cell co-stimulation were significantly downregulated in the high-risk group. Conversely, major histocompatibility complex (MHC), parainflammation, and type I IFN were remarkably upregulated. Our study revealed that the activation status of numerous immune functions might play a critical role in regulating anti-tumor response.
The model displayed a distinctive immune signature in TMB
Based on clinical relevance, TMB-related mutations were analyzed in the low-risk and high-risk groups. Visually, the ranks of mutated genes in the two groups were essentially the same. However, in the frequency of mutation, CTNNB1, CTCF, KMT2B genes in the low-risk group had almost more than twice the percentage than that of the high-risk group; conversely, TP53 gene demonstrated the opposite trend in the high-risk group (Figure 7A,7B). As an overall perspective, the TMB in the low-risk group was higher than that in the high-risk group. Furthermore, in the survival analysis, high TMB showed better survival status than low TMB (Figure 7C,7D). Next, we examined the prognosis value of the risk model and TMB by using KM survival analysis. The results indicated that, compared with other groups, the survival rate was the highest in High-TMB + low-risk group and the lowest in Low-TMB + high-risk group (Figure 7E). Taken together, our results suggested that low-risk patient’s positive outcome was likely due to the regulation of TMB.
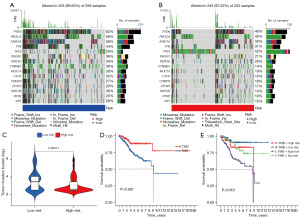
Effective antineoplastic drug prediction obtained by sensitivity analysis
To provide a new strategy for the treatment of UCEC, a drug sensitivity prediction analysis was conducted between low-risk and high-risk groups. The results revealed that the drug sensitivity in high-risk group was higher than that of the low-risk group. Moreover, 18 sensitive chemotherapy drugs were identified, including 15 targeted inhibitors (AZ628, BMS-754807, crizotinib, doxorubicin, FH535, GSK429286A, GW-2580, HG-6-64-1, JQ12, PHA-665752, salubrinal, sorafenib, TAK-715, tubastatin A, and zibotentan) (Figure 8A), 2 non-selective inhibitors (mitomycin C, and vinorelbine) (Figure 8B), and 1 targeted activator (phenformin) (Figure 8C).
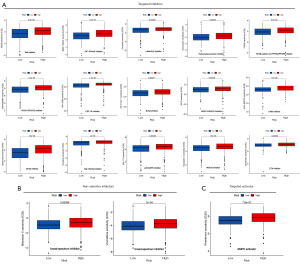
Discussion
In recent years, research on lncRNAs has received more and more attention. lncRNAs have been confirmed to participate extensively in the regulation of numerous processes in humans, such as cell growth, apoptosis, survival, as well as immune response and tissue microenvironment (34). Although lncRNA-targeting therapeutics have not been implemented for clinical application so far, there is evidence to suggest the significant role of lncRNAs in the development of new therapeutic approaches (35). Multiple experimental studies also demonstrated that lncRNAs might affect cell proliferation and metastasis in UCEC by targeting mammalian target of rapamycin (mTOR) and vascular endothelial growth factor A (VEGFA) pathways, holding great promise for clinical application by serving as a therapeutic target (36,37). Compared with the adjacent normal tissues, UCEC tumor tissues expressed a different expression pattern of lncRNAs, which influenced the tumorigenesis, progression, and metastasis (38).
Under normal physiological conditions, copper balance is critical for mediating metabolism and immune regulation. Once this balance is broken, the intracellular copper distribution will be corrupted, which may eventually result in tumorigenesis (39). A clinical study showed that copper levels were closely associated with UCEC development (40). Copper may actively engage in the specific processes of tumor development, such as cell proliferation, angiogenesis, and metastasis; thus, restoring copper equilibrium may be a novel therapeutic strategy for treating cancer (41). Recently, cuproptosis-associated lncRNA signatures were shown to have a high accuracy in predicting the prognosis of tumor OS, which provides novel insights into the clinical application and targeted immunotherapy (42). However, up to now, there have been no relevant study on the cuproptosis-related lncRNAs of UCEC.
In this study, we first scrutinized the correlation between cuproptosis genes and lncRNAs, and then 155 lncRNAs were identified. Subsequently, 9 lncRNAs related to prognosis were obtained through univariate Cox analysis. Furthermore, a 6 cuproptosis-related lncRNAs (AC073046.1, AC108479.1, ASH1L-AS1, LINC01644, AL132639.2, SBF2-AS1) risk model was constructed using LASSO regression analysis. Some studies have shown that LINC01644 and SBF2-AS1 are intimately associated with tumorigenesis and prognosis in cervical cancer and gastric cancer, respectively (43,44). However, currently, there is no detailed information of the 6 lncRNAs reported in UCEC, thus suggesting a further exploration for those connections. Subsequently, the accuracy and reliability of the risk model were verified through univariate Cox regression, multivariate Cox regression, ROC, nomogram, and calibration process. Meanwhile, the risk model was applied to differentiate patients in low-risk and high-risk by PCA and stage analysis. As a result, the reliability and accuracy of the model were verified through a series of analysis, which may provide an entry point for future studies. According to our results, patients in low-risk and high-risk groups could be easily distinguished from each other by the risk score; however, the functional differences between groups were still required to be fully elucidated. Thereupon, GO enrichment analysis was conducted and the results showed that membrane-anchored and extracellular matrix metabolisms were significantly enriched, which reflecting the invasion and metastasis properties of poor OS. The tumor microenvironment is dependent on the function of extracellular matrix remodeling and cell adhesion, and it may eventually have an impact on the final prognosis of the tumor (45).
Currently, immunotherapy represents a great breakthrough for its safety and effectiveness in many cancers; patients who have characteristic immune status such as TMB and immune check-point are prone to benefit from immunosuppressive therapy (46). Functionally, the cuproptosis-associated lncRNAs may contribute to the sensitivity regulation of the immune response through the regulation of immune pattern and TMB. Interestingly, we found that the low-risk group tended to have a positive immune pattern with an elevated activity response (cytolytic activity, inflammation, and T cell regulation), while the high-risk group showed a high trend in immune suppressing pattern with an upregulated activity response (parainflammation, and MHC).
Additionally, the low-risk group had higher check-point and CCR activity than those of the high-risk group, which might indicate better relative outcomes with a high response to inhibitor treatment. In addition, we also investigated the TMB in the combination of risk scores and TMB levels. Our results showed that high TMB was likely to have a good prognosis, which was compatible with the TMB level in the low-risk group. TMB, widely used in predicting response to immunotherapy, is considered to be a vital marker for quantifying mutations in malignant tumors (47). The TMB level is applied to measure the number of somatic mutations in a tumor and is usually viewed as a biomarker in immunotherapy (48). Indeed, there is evidence that UCEC patients with high TMB are likely to be easy recognized by immune cells, thus may benefit from immunotherapy. Our findings showed that the low-risk group tended to have a positive immune pattern and high level of TMB, which may account for the long OS in those patients. Additionally, to provide an adequate treatment for UCEC, 18 sensitive drugs were predicted to be effective agents against UCEC; those include the common chemotherapy drugs to treat UCEC, such as doxorubicin and sorafenib, as confirmed by clinical trials (49,50). Although research about the application of other drugs in UCEC is scare, there were still studies presenting a validation in the treatment of other tumor types using AZ628 (51), BMS-754807 (52), crizotinib (53), FH535 (54), GSK429286A (55), salubrinal (56), zibotentan (57), mitomycin C (58), and vinorelbine (59). Regarding to phenformin drugs, results of experimental study suggested that phenformin can suppress tumor proliferation and invasion through the activation of AMP-activated protein kinase (60). By performing a sensitivity drug analysis between low-risk and high-risk groups, a series of new compounds against UCEC were generated. Based on the prediction of the sensitive drugs, lncRNAs targeted agent therapy may be used as a complementary or new strategy for individualized therapy of UCEC (11). Cuproptosis-associated lncRNAs may be used as targets in immunotherapy to suppress the aggressiveness and progression of UCEC, thereby contributing to the increase in survival time. Currently, the use of lncRNA therapy is limited due to a lack of specificity, delivery safety, and tolerability in the human body, and the inappropriate or incorrect use of lncRNA therapy may result in deleterious effects (35). Thus, further experimental validations and clinical studies are still required in the future.
In summary, a prognostic risk model consisting of 6 cuproptosis-related lncRNAs in UCEC was constructed in our study, and the high accuracy and specificity were verified through a series of validations. The functional enrichment and immune signature were then explored. Additionally, efforts have been made to predict drug responses, which in turn may provide an individualized therapy for patients. However, several limitations in our study should be mentioned. First, as a relatively new concept, the acquisition of precise cuproptosis-related genes was difficult owing to the deficiencies in the professional literature, suggesting that future studies may also need to verify cuproptosis-related genes. Second, cuproptosis-related lncRNAs were obtained based on the existing literature, which may have caused important low correlation lncRNAs to be omitted in the current study. Third, this study was based on the bioinformatic analysis, which may require external validation of experimental studies in vivo and vitro. Four, we found that UCEC clinical data in TCGA did not contain data of tumor grade or TNM stage, which may have caused bias and affected the results. Finally, from the present study, we can only speculate that 6 lncRNAs are highly involved in the regulation of cell adhesion and invasion in UCEC; however, the detailed mechanism is still not fully clear. Therefore, more studies are required in the future to confirm these findings.
Conclusions
This study had successfully constructed a cuproptosis-lncRNA model with high predictive and accuracy performance to predict the outcomes of UCEC. In addition, the risk model could be used as an independent marker for prognosis of UCEC, while providing lncRNA therapy guidance for personalized treatment with immunotherapy and chemotherapy.
Acknowledgments
We thank TCGA for the availability of the data.
Funding: None.
Footnote
Reporting Checklist: The authors have completed the TRIPOD reporting checklist. Available at https://tcr.amegroups.com/article/view/10.21037/tcr-23-61/rc
Data Sharing Statement: Available at https://tcr.amegroups.com/article/view/10.21037/tcr-23-61/dss
Peer Review File: Available at https://tcr.amegroups.com/article/view/10.21037/tcr-23-61/prf
Conflicts of Interest: All authors have completed the ICMJE uniform disclosure form (available at https://tcr.amegroups.com/article/view/10.21037/tcr-23-61/coif). The authors have no conflicts of interest to declare.
Ethical Statement: The authors are accountable for all aspects of the work in ensuring that questions related to the accuracy or integrity of any part of the work are appropriately investigated and resolved. The study was conducted in accordance with the Declaration of Helsinki (as revised in 2013).
Open Access Statement: This is an Open Access article distributed in accordance with the Creative Commons Attribution-NonCommercial-NoDerivs 4.0 International License (CC BY-NC-ND 4.0), which permits the non-commercial replication and distribution of the article with the strict proviso that no changes or edits are made and the original work is properly cited (including links to both the formal publication through the relevant DOI and the license). See: https://creativecommons.org/licenses/by-nc-nd/4.0/.
References
- Holst F, Werner HMJ, Mjøs S, et al. PIK3CA Amplification Associates with Aggressive Phenotype but Not Markers of AKT-MTOR Signaling in Endometrial Carcinoma. Clin Cancer Res 2019;25:334-45. [Crossref] [PubMed]
- Tarney CM, Tian C, Wang G, et al. Impact of age at diagnosis on racial disparities in endometrial cancer patients. Gynecol Oncol 2018;149:12-21. [Crossref] [PubMed]
- Haraga J, Nakamura K, Haruma T, et al. Molecular Characterization of Second Primary Endometrial Cancer. Anticancer Res 2020;40:3811-8. [Crossref] [PubMed]
- Lin Z, Fan W, Sui X, et al. Necroptosis-Related LncRNA Signatures for Prognostic Prediction in Uterine Corpora Endometrial Cancer. Reprod Sci 2023;30:576-89. [Crossref] [PubMed]
- Xue VW, Wong SCC, Song G, et al. Promising RNA-based cancer gene therapy using extracellular vesicles for drug delivery. Expert Opin Biol Ther 2020;20:767-77. [Crossref] [PubMed]
- Chen R, Yang M, Huang W, et al. Cascades between miRNAs, lncRNAs and the NF-Kb signaling pathway in gastric cancer Exp Ther Med 2021;22:769. (Review). [Crossref] [PubMed]
- He WP, Chen YY, Wu LX, et al. A novel necroptosis-related lncRNA signature for predicting prognosis and anti-cancer treatment response in endometrial cancer. Front Immunol 2022;13:1018544. [Crossref] [PubMed]
- Wang C, Kong F, Ma J, et al. IGF2BP3 enhances the Mrna stability of E2F3 by interacting with LINC00958 to promote endometrial carcinoma progression. Cell Death Discov 2022;8:279. [Crossref] [PubMed]
- Wang Y, Zhang L, Zhou F. Cuproptosis: a new form of programmed cell death. Cell Mol Immunol 2022;19:867-8. [Crossref] [PubMed]
- Cobine PA, Brady DC. Cuproptosis: Cellular and molecular mechanisms underlying copper-induced cell death. Mol Cell 2022;82:1786-7. [Crossref] [PubMed]
- Wu Q, Tian R, Tan H, et al. A comprehensive analysis focusing on cuproptosis to investigate its clinical and biological relevance in uterine corpus endometrial carcinoma and its potential in indicating prognosis. Front Mol Biosci 2022;9:1048356. [Crossref] [PubMed]
- Wang X, Jing H, Li H. A novel cuproptosis-related lncRNA signature to predict prognosis and immune landscape of lung adenocarcinoma. Transl Lung Cancer Res 2023;12:230-46. [Crossref] [PubMed]
- Liu L, Zheng J, Xia H, et al. Construction and comprehensive analysis of a curoptosis-related lncRNA signature for predicting prognosis and immune response in cervical cancer. Front Genet 2023;14:1023613. [Crossref] [PubMed]
- Li N, Yu K, Lin Z, et al. Identifying immune subtypes of uterine corpus endometrial carcinoma and a four-paired-lncRNA signature with immune-related lncRNAs. Exp Biol Med (Maywood) 2022;247:221-36. [Crossref] [PubMed]
- Xu Y, Wang X, Chu Y, et al. Analysis of transcript-wide profile regulated by microsatellite instability of colorectal cancer. Ann Transl Med 2022;10:169. [Crossref] [PubMed]
- Joo T, Choi JH, Lee JH, et al. SEQprocess: a modularized and customizable pipeline framework for NGS processing in R package. BMC Bioinformatics 2019;20:90. [Crossref] [PubMed]
- Lv H, Liu X, Zeng X, et al. Comprehensive Analysis of Cuproptosis-Related Genes in Immune Infiltration and Prognosis in Melanoma. Front Pharmacol 2022;13:930041. [Crossref] [PubMed]
- Xu Y, Wang N, Liu R, et al. Epigenetic Study of Esophageal Carcinoma Based on Methylation, Gene Integration and Weighted Correlation Network Analysis. Onco Targets Ther 2021;14:3133-49. [Crossref] [PubMed]
- Shi W, Feng L, Dong S, et al. Exploration of prognostic index based on immune-related genes in patients with liver hepatocellular carcinoma. Biosci Rep 2020;40:BSR20194240. [Crossref] [PubMed]
- Sun JR, Kong CF, Xiao KM, et al. Integrated Analysis of lncRNA-Mediated ceRNA Network Reveals a Prognostic Signature for Hepatocellular Carcinoma. Front Genet 2020;11:602542. [Crossref] [PubMed]
- Gu CY, Dai B, Zhu Y, et al. The novel transcriptomic signature of angiogenesis predicts clinical outcome, tumor microenvironment and treatment response for prostate adenocarcinoma. Mol Med 2022;28:78. [Crossref] [PubMed]
- Tu Z, Wu L, Wang P, et al. N6-Methylandenosine-Related lncRNAs Are Potential Biomarkers for Predicting the Overall Survival of Lower-Grade Glioma Patients. Front Cell Dev Biol 2020;8:642. [Crossref] [PubMed]
- Luo C, Wang S, Shan W, et al. A Whole Exon Screening-Based Score Model Predicts Prognosis and Immune Checkpoint Inhibitor Therapy Effects in Low-Grade Glioma. Front Immunol 2022;13:909189. [Crossref] [PubMed]
- Wei RL, Zhang LW, Li JG, et al. Behavior-Oriented Nomogram for the Stratification of Lower-Grade Gliomas to Improve Individualized Treatment. Front Oncol 2020;10:538133. [Crossref] [PubMed]
- Sui Z, Wu X, Du L, et al. Characterization of the Immune Cell Infiltration Landscape in Esophageal Squamous Cell Carcinoma. Front Oncol 2022;12:879326. [Crossref] [PubMed]
- Song J, Lin Y, Zhang J, et al. Effect of the Age-Adjusted Charlson Comorbidity Index on the Survival of Esophageal Squamous Cell Carcinoma Patients after Radical Esophagectomy. J Clin Med 2022;11:6737. [Crossref] [PubMed]
- Chong X, Peng R, Sun Y, et al. Identification of Key Genes in Gastric Cancer by Bioinformatics Analysis. Biomed Res Int 2020;2020:7658230. [Crossref] [PubMed]
- Yu K, Liu D, Chen Y, et al. Unraveling the genetic architecture of grain size in einkorn wheat through linkage and homology mapping and transcriptomic profiling. J Exp Bot 2019;70:4671-88. [Crossref] [PubMed]
- Song X, Xin S, Zhang Y, et al. Identification and Quantification of Iron Metabolism Landscape on Therapy and Prognosis in Bladder Cancer. Front Cell Dev Biol 2022;10:810272. [Crossref] [PubMed]
- Wang B, Zhong JL, Li HZ, et al. Diagnostic and therapeutic values of PMEPA1 and its correlation with tumor immunity in pan-cancer. Life Sci 2021;277:119452. [Crossref] [PubMed]
- Ge Y, Wei F, Du G, et al. The association of sex-biased ATRX mutation in female gastric cancer patients with enhanced immunotherapy-related anticancer immunity. BMC Cancer 2021;21:240. [Crossref] [PubMed]
- Wang Y, Wang Z, Sun J, et al. Identification of HCC Subtypes With Different Prognosis and Metabolic Patterns Based on Mitophagy. Front Cell Dev Biol 2021;9:799507. [Crossref] [PubMed]
- Trojano G, Olivieri C, Tinelli R, et al. Conservative treatment in early stage endometrial cancer: a review. Acta Biomed 2019;90:405-10. [PubMed]
- Hu Q, Egranov SD, Lin C, et al. Long noncoding RNA loss in immune suppression in cancer. Pharmacol Ther 2020;213:107591. [Crossref] [PubMed]
- Winkle M, El-Daly SM, Fabbri M, et al. Noncoding RNA therapeutics – challenges and potential solutions. Nat Rev Drug Discov 2021;20:629-51. [Crossref] [PubMed]
- Du Y, Wang L, Chen S, et al. lncRNA DLEU1 contributes to tumorigenesis and development of endometrial carcinoma by targeting Mtor. Mol Carcinog 2018;57:1191-200. [Crossref] [PubMed]
- Zhong Y, Wang Y, Dang H, et al. LncRNA AFAP1-AS1 contributes to the progression of endometrial carcinoma by regulating miR-545-3p/VEGFA pathway. Mol Cell Probes 2020;53:101606. [Crossref] [PubMed]
- Wang J, Li Z, Wang X, et al. The tumor suppressive effect of long non-coding RNA FRMD6-AS2 in uteri corpus endometrial carcinoma. Life Sci 2020;243:117254. [Crossref] [PubMed]
- Voli F, Valli E, Lerra L, et al. Intratumoral Copper Modulates PD-L1 Expression and Influences Tumor Immune Evasion. Cancer Res 2020;80:4129-44. [Crossref] [PubMed]
- Atakul T, Altinkaya SO, Abas BI, et al. Serum Copper and Zinc Levels in Patients with Endometrial Cancer. Biol Trace Elem Res 2020;195:46-54. [Crossref] [PubMed]
- Jiang Y, Huo Z, Qi X, et al. Copper-induced tumor cell death mechanisms and antitumor theragnostic applications of copper complexes. Nanomedicine (Lond) 2022;17:303-24. [Crossref] [PubMed]
- Wang F, Lin H, Su Q, et al. Cuproptosis-related lncRNA predict prognosis and immune response of lung adenocarcinoma. World J Surg Oncol 2022;20:275. [Crossref] [PubMed]
- Zhang S, Li S, Guo JL, et al. Integrated Analysis of lncRNA-Associated ceRNA Network Identifies Two lncRNA Signatures as a Prognostic Biomarker in Gastric Cancer. Dis Markers 2021;2021:8886897. [Crossref] [PubMed]
- Gao F, Feng J, Yao H, et al. LncRNA SBF2-AS1 promotes the progression of cervical cancer by regulating miR-361-5p/FOXM1 axis. Artif Cells Nanomed Biotechnol 2019;47:776-82. [Crossref] [PubMed]
- Niland S, Riscanevo AX, Eble JA. Matrix Metalloproteinases Shape the Tumor Microenvironment in Cancer Progression. Int J Mol Sci 2021;23:146. [Crossref] [PubMed]
- Shi M, Luo F, Shao T, et al. Positive Correlation Between LTA Expression and Overall Immune Activity Suggests an Increased Probability of Survival in Uterine Corpus Endometrial Carcinoma. Front Cell Dev Biol 2022;9:793793. [Crossref] [PubMed]
- Li G, Wang Y, Cheng Y. IL2RB Is a Prognostic Biomarker Associated with Immune Infiltrates in Pan-Cancer. J Oncol 2022;2022:2043880. [Crossref] [PubMed]
- Zhao L, Fu X, Han X, et al. Tumor mutation burden in connection with immune-related survival in uterine corpus endometrial carcinoma. Cancer Cell Int 2021;21:80. [Crossref] [PubMed]
- du Bois A, Pfisterer J, Burchardi N, et al. Combination therapy with pegylated liposomal doxorubicin and carboplatin in gynecologic malignancies: a prospective phase II study of the Arbeitsgemeinschaft Gynäekologische Onkologie Studiengruppe Ovarialkarzinom (AGO-OVAR) and Kommission Uterus (AGO-K-Ut). Gynecol Oncol 2007;107:518-25. [Crossref] [PubMed]
- Nimeiri HS, Oza AM, Morgan RJ, et al. A phase II study of sorafenib in advanced uterine carcinoma/carcinosarcoma: a trial of the Chicago, PMH, and California Phase II Consortia. Gynecol Oncol 2010;117:37-40. [Crossref] [PubMed]
- Xiao Z, Liu F, Cheng J, et al. B-Raf inhibitor vemurafenib counteracts sulfur mustard-induced epidermal impairment through MAPK/ERK signaling. Drug Chem Toxicol 2023;46:226-35. [Crossref] [PubMed]
- Li J, Zhu H, Yang Q, et al. Identification of Molecular Subtypes and Potential Small-Molecule Drugs for Esophagus Cancer Treatment Based on m6A Regulators. J Oncol 2022;2022:5490461. [Crossref] [PubMed]
- Mai S, Xiong G, Diao D, et al. Case report: Crizotinib is effective in a patient with ROS1-rearranged pulmonary inflammatory myofibroblastic tumor. Lung Cancer 2019;128:101-4. [Crossref] [PubMed]
- Ren J, Yuan Q, Liu J, et al. Identifying the role of transient receptor potential channels (TRPs) in kidney renal clear cell carcinoma and their potential therapeutic significances using genomic and transcriptome analyses. BMC Med Genomics 2022;15:156. [Crossref] [PubMed]
- Liu S, Rong G, Li X, et al. Diosgenin and GSK126 Produce Synergistic Effects on Epithelial-Mesenchymal Transition in Gastric Cancer Cells by Mediating EZH2 via the Rho/ROCK Signaling Pathway. Onco Targets Ther 2020;13:5057-67. [Crossref] [PubMed]
- Chen B, Hong W, Tang Y, et al. Protective effect of the NAC and Sal on zinc oxide nanoparticles-induced reproductive and development toxicity in pregnant mice. Food Chem Toxicol 2020;143:111552. [Crossref] [PubMed]
- Wang Y, Zhang H, Shen W, et al. Effectiveness and tolerability of targeted drugs for the treatment of metastatic castration-resistant prostate cancer: a network meta-analysis of randomized controlled trials. J Cancer Res Clin Oncol 2018;144:1751-68. [Crossref] [PubMed]
- Wang SC, Yu CY, Wu YC, et al. Chidamide and mitomycin C exert synergistic cytotoxic effects against bladder cancer cells in vitro and suppress tumor growth in a rat bladder cancer model. Cancer Lett 2022;530:8-15. [Crossref] [PubMed]
- Coronel JA, Cetina Ldel C, Cantú D, et al. A randomized comparison of cisplatin and oral vinorelbine as radiosensitizers in aged or comorbid locally advanced cervical cancer patients. Int J Gynecol Cancer 2013;23:884-9. [Crossref] [PubMed]
- Jaidee R, Kongpetch S, Senggunprai L, et al. Phenformin inhibits proliferation, invasion, and angiogenesis of cholangiocarcinoma cells via AMPK-Mtor and HIF-1A pathways. Naunyn Schmiedebergs Arch Pharmacol 2020;393:1681-90. [Crossref] [PubMed]