Novel potential urinary biomarkers for effective diagnosis and prognostic evaluation of high-grade bladder cancer
Highlight box
Key findings
• Through identifying differentially expressed genes in urine and tumor tissue samples from patients with HGBC, we developed an optimal risk model to predict patient outcomes and screened out eighteen hub genes as novel potential urinary biomarkers for effective diagnosis of HGBC.
What is known and what is new?
• High-grade bladder cancer has a higher malignant potential, recurrence and progression rate compared to low-grade phenotype, and its early symptoms are often vague.
• We identified reliable oncological urinary biomarkers for effective diagnosis and prognostic evaluation for high-grade bladder cancer, which is a promising alternative for the noninvasive diagnosis.
What is the implication, and what should change now?
• Novel urinary biomarkers with satisfactory sensitivity contributing to high diagnostic accuracy are needed urgently. Our findings may provide some evidence for the future non-invasive detection with high diagnostic accuracy for bladder cancer from new insights.
Introduction
With 81,180 new cases annually, bladder cancer (BC) ranks as the tenth most common cancer globally and is considered a leading cause of cancer-related fatalities (1). The most frequent symptom is painless haematuria, which is commonly the only observed symptom of BC (2). As with many cancers, the initial signs and symptoms of BC are often non-specific, presenting a diagnostic difficulty in differentiating them from benign conditions, which often leads to delayed diagnosis until the tumor has grown to a certain size or even metastasized and becomes incurable before being definitively diagnosed. High-grade bladder cancer (HGBC) is characterized by tumor cells exhibiting abnormal cell morphology, significant atypia, a high rate of cell division, and rapid growth, which can lead to invasion and metastasis to other tissues and organs. In comparison to low-grade bladder cancer (LGBC), HGBC tends to result in a poorer prognosis and shorter survival time (3). High-grade tumors necessitate a concurrent cystoscopy and urinary cytology test every three months for the first two years. Despite its efficacy, cystoscopy is invasive, painful, expensive, and time-consuming. Furthermore, urinary cytology tests often exhibit unsatisfactory sensitivity (4).
Urine testing is a non-invasive, simple and reproducible method that plays a crucial role in monitoring and treating bladder cancer. It improves early detection, reduces unnecessary pain and cost for patients, and promotes timely treatment and management that can enhance the prognosis and quality of life of patients. Recently, there have been several proposed potential urinary biomarkers for BC diagnosis at the DNA, RNA, and protein levels (5). The US Food and Drug Administration has approved several urinary detection methods for clinical use, such as Nuclear Matrix Protein 22 (NMP22), Bladder Tumor Antigen (BTA), UroVysion, and Immunocyt. However, these markers often exhibit low specificity and high false positive rates, and are only approved for use with cystoscopy (6). Therefore, more novel urinary biomarkers with satisfactory sensitivity contributing to high diagnostic accuracy are needed urgently.
With the emergence of high-throughput microarray and next-generation sequencing technologies, bioinformatics has become a crucial tool for managing vast amounts of data. It has been used to identify novel prognostic markers, metastasis markers, and recurrence prediction markers from genomics, transcriptomics, and proteomics data (7). In this study, we extracted urine sample data from the GEO database to identify differentially expressed genes (DEGs) associated with the pathogenesis and prognosis of HGBC. After screening for DEGs using data extracted from 387 HGBC and 19 adjacent normal bladder tissues in The Cancer Genome Atlas (TCGA) database, we identified 176 genes that were common between the two datasets, suggesting that these genes were highly expressed in the urine of HGBC patients as well as in the tumor tissues. Subsequently we conducted a functional enrichment analysis, followed by constructing a protein-protein interaction (PPI) network to identify the hub genes. Next, a competing endogenous RNA (ceRNA) regulation network was constructed to predict the interactions among hub genes, microRNAs (miRNAs) and long non-coding RNAs (lncRNAs) to provide new perspectives for evaluating the urinary critical genes regulatory network in HGBC. Finally, we assessed the expression of the selected genes in BC cells through quantitative real-time reverse transcription-polymerase chain reaction (qRT-PCR), followed by exploring possible co-expressed genes and their potential functions at the cellular level utilizing the CCLE database. We present this article in accordance with MDAR reporting checklist (available at https://tcr.amegroups.com/article/view/10.21037/tcr-23-98/rc).
Methods
Data mining and collection
GSE68020 dataset and its corresponding platform annotation files were obtained from the GEO database (https://www.ncbi.nlm.nih.gov/geo) (8), which contained of the data of 50 urine sediment samples, including 30 HGBC and 20 benign samples assessed using urine cytology. The clinical information of patients with HGBC and relevant messenger RNA (mRNA), lncRNA and miRNA expression data were downloaded from TCGA Bladder Carcinoma (TCGA-BLCA) study of the official TCGA website (https://cancergenome.nih.gov/). The samples containing incomplete clinical information were excluded. The Gene Expression Profiling Interactive Analysis (GEPIA) platform (http://gepia2.cancer-pku.cn/#index) was used to validate the expression of candidate genes in BC samples (9). Furthermore, the CCLE database (https://portals.broadinstitute.org/ccle/about) (10) were used to analyze the expression of specific genes in 25 different urinary tract cancer cell lines. The study was conducted in accordance with the Declaration of Helsinki (as revised in 2013).
Data preprocessing and identification of DEGs
The probes lacking gene expression profile annotations were subjected to filtration using the Bioconductor Affy package in R (R v.4.1.0) (11), securing the reproducibility, transparency and efficiency of development (12). The probe ID assigned to each gene was converted into its corresponding gene symbol using the Affymetrix Human Genome U133 Plus 2.0 Array annotation data (hgu133plus2.db) and Genome wide annotation for Human, version: 3.7 (org. Hs.eg.db) package from Bioconductor (https://www.bioconductor.org/). For multiple probe IDs associated with a single gene symbol, the representative expression value of the gene was determined by calculating the average expression value across these probes, to ensure a consolidated and reliable assessment of the gene expression level. The DEGs were screened using the Linear Models for Microarray Analysis package (13) with cut off criteria of |logFC (fold change)| ≥0.5 and P value <0.05.
Functional enrichment analysis for DEGs
The Disease Ontology Semantic and Enrichment analysis (DOSE) (14) and clusterProfiler (15) packages were used in the statistical R programming language (Version 3.6.2) to explore the information associated with GO terms and for implementing the KEGG pathway enrichment analysis. To ensure precise visual representation, the ggplot2 and pROC packages were utilized for generating high-quality graphs. Gene Set Enrichment Analysis (GSEA) 4.0.3 was implemented to determine whether a priori defined set of genes significantly differed between two biological states, (16). The functional gene set file “c2.cp.kegg.v7.0.symbols.gmt” was employed to aggregate precise and well-defined signaling information. The number of substitutions per analysis was set at 1,000, and gene sets with P value <0.05 were recognized as significantly enriched.
PPI network construction and module analysis
The initial PPI network was constructed utilizing the Search Tool for the Retrieval of Interacting Genes (STRING) (version 11.5; https://string-db.org) online platform (17). After setting the minimum value for the highest confidence to 0.4, data on the unrelated proteins were eliminated. Molecular Complex Detection (MCODE) (version 1.4.2), a cluster algorithmic-based software in Cytoscape, was applied to identify densely connected regions for a given network based on topology (18). Using the Cytoscape visualization software (version 3.7.1), we generated the final PPI networks. The identification of the most significant module was accomplished via the MCODE algorithm. The data underwent filtration based on the following criteria: MCODE score >5, maximum depth =100, degree cut-off =2, node score cut-off =0.2, and k-score =2.
Cell culture and treatment
The human HGBC cell lines T24 and J82, and the normal urothelial cell SVHUC1 were purchased from the Cell Resource Center of the Shanghai Institutes for Biological Sciences, Chinese Academy of Sciences (Shanghai, China). We cultured all cell lines in Roswell Park Memorial Institute (RPMI) 1640 medium with 100 U/mL penicillin, 100 µg/mL streptomycin, and 10% fetal bovine serum at 5% CO2 in a 37 ℃ humidified culture environment.
RNA isolation and qRT-PCR
Total RNA was isolated using the Total RNA Extractor (TRIzol) reagent (Life Technologies, Carlsbad, CA, USA) according to the manufacturer’s instructions. The SYBR Premix Ex Taq II (Perfect Real-Time) kit (TaKaRa Bio, Shiga, Japan) was used and qRT-PCR was performed as follows: 95 ℃ for 30 s, and 39 cycles of 95 ℃ for 5 s and 60 ℃ for 30 s. At the completion of each PCR run, a DNA dissociation analysis, including the generation of a melting curve, was performed to verify the absence of primer dimers, mixed-amplicon populations, and nonspecific products. The relative expression of genes was quantified using the comparative threshold cycle (2−ΔΔCt) method based on data obtained from a minimum of three independent experiments. Expression of the beta-actin gene was utilized as an internal reference to normalize the expression of target genes. The primer sequences were as follows: human DKC1, forward 5'-TTCTGGACAAGCATGGGAAG-3' and reverse 5'-CAGCAAGTGCCGTCTCTA-3'; human SNRPG, forward 5'-GAG GCCAATGCTCAGAGAAG-3' and reverse 5'-AGGGAGGT TAAGGCACACCT -3'; beta-actin, forward 5'-AAACGTGCTGCTGACCGAG-3' and reverse 5'-TAGCACAGCCTGGATAGCAAC-3'.
The ceRNA network construction
To further evaluate the hub genes regulatory network in HGBC, we constructed a ceRNA regulation network. The potential miRNAs associated with hub genes were identified using the TargetScan (http://www.targetscan.org/) and miRDB (http://www.mirdb.org/mmiRDB/) databases. Differentially expressed long non-coding RNAs (DElncRNAs) (|logFC| >2.0) and differentially expressed microRNAs (DEmiRNAs) (|logFC| >1.0) with adjusted P value <0.05 were identified using the edgeR package in the R statistical environment. MiRcode (http://www.mircode.org/) was used to predict the miRNAs associated with DElncRNAs, and information on miRNAs unrelated to the hub genes was excluded from the network. We integrated these data and used Cytoscape to visualize the interaction among lncRNA-miRNA-mRNA.
Statistical analysis
Box plots were employed to depict the distribution of expression differences for discrete variables. The chi-squared test was utilized to evaluate the statistical significance of the relationship between gene expression and clinical data. Survival curves were generated using the Kaplan-Meier method, and the log-rank test was employed to assess the statistical significance. Univariate Cox analysis was conducted to identify significant variables, followed by multivariate Cox analysis to assess the prognostic significance of gene expression in overall survival (OS). Based on individual mRNA expression level and the regression coefficient obtained from multivariate Cox analysis, a personalized risk score was calculated for each patient. Risk score is calculated as follows: ExpmRNA1 × βmRNA1 + ExprnRNA2 × βmRNA2 + ⋯ + ExpmRNAn × βmRNAn (Exp represents each mRNA expression level, and β represents each mRNA regression coefficient). The optimal risk model was determined using the Akaike Information Criterion (AIC) as the evaluation criterion (19). Patients were divided into high-risk group and low-risk group according to the median value of risk scores. The anticipated power of the prognostic gene signature was evaluated by calculating the area under the curve (AUC) of the receiver operating characteristic (ROC) curves. A statistically significant value was one with a P value <0.05.
Results
Microarray data normalization and identification of DEGs
The clinical characteristics of the samples from the GSE68020 dataset are presented in Table S1. DEGs identification was performed after standardizing the microarray results (Figure 1). A total of 560 genes including 375 up-regulated and 185 down-regulated genes were identified as DEGs when comparing the urine sediment samples from HGBC patients with the benign ones (available online: https://cdn.amegroups.cn/static/public/tcr-23-98-1.xlsx).
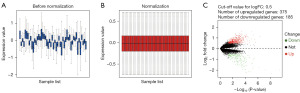
Function enrichment analysis for DEGs
A total of 319 and 32 remarkably enriched GO terms (adj. P value ≤0.05) were obtained for the up-regulated and down-regulated DEGs, respectively (Figure 2). The up-regulated genes were mainly enriched in the process of regulation of mitosis, cell cycle, stem cell differentiation, immune response, response to hypoxia, apoptotic, WNT and NF-κB signaling. The down-regulated DEGs were mainly enriched in the biological process of cornification, humoral immune response, keratinocyte and epidermal cell differentiation (available online: https://cdn.amegroups.cn/static/public/tcr-23-98-2.xlsx). The integrated DEGs were primarily involved in five pathways, namely ribosome, proteasome, biosynthesis of amino acids, HIF-1 signaling pathway and 2-Oxocarboxylic acid metabolism (Table 1), which were all related to the tumorigenesis and progression of BC.
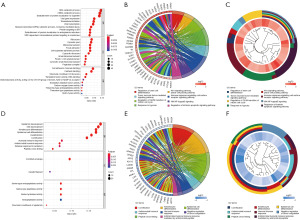
Table 1
Pathway ID | Pathway description | Count | P value | Gene ID |
---|---|---|---|---|
hsa03010 | Ribosome | 22 | 1.21E−06 | RPL22, RPS3, RPS12, RPL15, RPS6, FAU, RPS20, RPS3A, RPS19, RPL13A, RPL6, RPL4, RPS14, RPS11, RPL30, RPS27, RPL14, RPS18, MRPL11, RPS5, RPS8, RPSA |
hsa03050 | Proteasome | 9 | 1.32E−03 | PSME4, PSMB1, PSMB2, PSMA5, PSMD7, PSMB5, PSMB4, PSMB6, PSMC3 |
hsa01230 | Biosynthesis of amino acids | 10 | 1.08E−02 | GPT2, ENO1, ENO2, BCAT2, TKT, GOT2, IDH1, GAPDH, ACY1, ALDOB |
hsa04066 | HIF-1 signaling pathway | 12 | 1.08E−02 | ENO1, IFNGR2, ENO2, RPS6, TFRC, LDHA, SLC2A1, EIF4E2, GAPDH, PLCG2, HMOX1, ALDOB |
hsa01210 | 2-Oxocarboxylic acid metabolism | 5 | 1.08E−02 | GPT2, BCAT2, GOT2, IDH1, ACY1 |
Through KEGG enrichment analysis on the DEGs identified in GSE68020, five pathways were found to be significantly enriched (adj. P value ≤0.05). KEGG, Kyoto Encyclopedia of Genes and Genomes; DEG, differentially expressed gene.
PPI network construction and module analysis
The PPI network of the DEGs was constructed, as presented in Figure 3A. Using the MCODE algorithm, we identified sixteen functional modules from the PPI network, and subsequently determined the top four modules, as illustrated in Figure 3B-3E. The GO terms and KEGG pathway enrichment analyses on the genes in each of the top four modules were performed (available online: https://cdn.amegroups.cn/static/public/tcr-23-98-3.xlsx). The results showed that the genes in module 1 are mainly enriched in the regulation of RNA processing and stabilization; the genes in module 2 are mainly enriched in keratinocyte and epidermal cell differentiation; the genes in module 3 are mainly enriched in the tumor necrosis factor-mediated signaling pathway, regulation of cell cycle, response to hypoxia, WNT signaling; and the genes in module 4 are mainly enriched in stem cell differentiation, immune response, response to hypoxia, WNT and NF-κB signaling pathway.
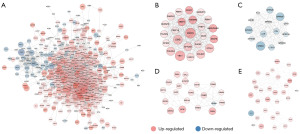
Construction of the optimal risk model for HGBC
We extracted the clinical and gene expression data from the 387 cases of HGBC in the TCGA database, and developed an optimal risk model for HGBC using the 560 identified urinary DEGs. The resulting model consisted of eight genes (Table 2). Of the eight genes, CRYAB, EMP1, EFTUD2, FASN, KDELR2, VWF and GAPDH showed positive coefficients, while BLCAP showed negative coefficient. The median value of the risk score of each sample was calculated to be 1.019, which was used as the cut-off value. According to the survival curve (Figure 4A), the five-year survival rate for patients in the high-risk group (190 patients) was 26.0%, with a 95% confidence interval (CI) of 18.2–37.1%, while the low-risk group (191 patients) had a five-year survival rate of 57.8%, with a 95% CI of 48.5–68.8%. The ROC curve was plotted simultaneously, and the AUC was 0.724 (Figure 4B), suggesting our model can effectively predict patient survival. The expression data of the eight genes were sorted in ascending order of the risk score and depicted in a heatmap (Figure 4C).
Table 2
Gene | Description | Coefficient | P value |
---|---|---|---|
CRYAB | Crystallin alpha B | 0.14 | 6.29E−03 |
EMP1 | Epithelial membrane protein 1 | 0.18 | 4.31E−03 |
EFTUD2 | Elongation factor Tu GTP binding domain containing 2 | 0.41 | 5.49E−02 |
FASN | Fatty acid synthase | 0.37 | 1.92E−05 |
KDELR2 | KDEL Endoplasmic Reticulum Protein Retention Receptor 2 | 0.42 | 9.95E−03 |
VWF | Von Willebrand factor | 0.20 | 2.15E−02 |
BLCAP | Bladder cancer associated protein | −0.22 | 2.82E−02 |
GAPDH | Glyceraldehyde-3-phosphate dehydrogenase | 0.26 | 3.83E−02 |
Likelihood ratio test=69.22 on 8 df, P=7.036e−12, n=381.
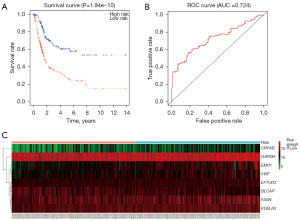
Identification and analysis of hub genes
Using the data extracted from 387 HGBC tissues and 19 adjacent non-cancer bladder tissues in the TCGA database, 7,858 DEGs (4,906 up-regulated and 2,952 down-regulated) were identified. The expression data of the DEGs were depicted by a volcano plot and a heatmap (Figure S1). The DEGs obtained from both GSE62080 and the TCGA database were intersected, and then 176 genes (106 up-regulated and 70 down-regulated, listed in Table S2) that exhibited similar expression trends in both datasets were identified after removing genes with discordant regulation. These genes have shown potential as a distinguisher between HGBC and benign cells in both urine sediments as well as solid tissue samples. A PPI network of the 176 genes was constructed (Figure 5A), and the top 30 genes in the PPI network according to the node degree were identified (Figure 5B). The MCODE algorithm was used to obtain the most significant module with 9 nodes and 36 edges (Figure 5C). As shown in Figure 5B, there were a total of 24 genes with a node degree ≥10 in the PPI network. Eighteen of them exhibited significant differential expression in both urine and tumor tissue samples from HGBC patients (with the filer criteria of |Log2 FC| >0.5 in the GSE62080 dataset and |Log2 FC| >0.75 in the TCGA-HGBC dataset). These 18 genes were further determined to be hub genes and listed in Table 3. Following GO and KEGG enrichment analyses of these hub genes, 227 remarkably enriched GO terms and three pathways (RNA polymerase, proteasome and ribosome biogenesis in eukaryotes) were obtained (with a adj. P value ≤0.05, listed in Table S3). Survival analysis was subsequently performed on these 18 hub genes, showing that overexpression of EFTUD2, LOR and EBNA1BP2 were associated with a worse OS of BC patients (Figure 6), while abnormal expression of others did not show significant prognostic value.
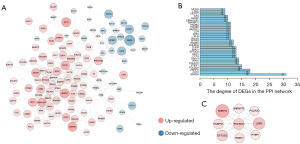
Table 3
Gene symbol | Gene description | Degree | logFC (GSE62080) | logFC (TCGA) |
---|---|---|---|---|
SNRPG | Small nuclear ribonucleoprotein polypeptide G | 18 | 1.33 | 0.87 |
CCT2 | Chaperonin containing TCP1 subunit 2 | 18 | 0.74 | 1.01 |
POLR2H | RNA polymerase II subunit H | 15 | 0.90 | 1.14 |
RPN1 | Ribophorin I | 14 | 1.03 | 0.96 |
DKC1 | Dyskerin pseudouridine synthase 1 | 13 | 1.16 | 0.94 |
EFTUD2 | Elongation factor Tu GTP binding domain containing 2 | 13 | 0.88 | 0.87 |
EMG1 | N1-specific pseudouridine methyltransferase | 13 | 0.82 | 1.00 |
POLR2G | RNA polymerase II subunit G | 12 | 0.58 | 0.92 |
SPRR3 | Small proline rich protein 3 | 12 | −2.16 | −3.67 |
PSMB2 | Proteasome subunit beta 2 | 11 | 0.72 | 0.86 |
PABPN1 | Poly(A) binding protein nuclear 1 | 11 | 0.70 | 0.80 |
CKS1B | CDC28 protein kinase regulatory subunit 1B | 11 | 0.57 | 1.57 |
PSMB4 | Proteasome subunit beta 4 | 11 | 0.57 | 0.80 |
FLG | Filaggrin | 11 | −0.58 | −1.41 |
LOR | Loricrin | 11 | −1.42 | −1.59 |
EBNA1BP2 | EBNA1 binding protein 2 | 10 | 1.20 | 0.80 |
ILF2 | Interleukin enhancer binding factor 2 | 10 | 0.81 | 0.80 |
H2AFZ | H2A histone family member Z | 10 | 0.79 | 0.78 |
FC, fold change; TCGA, The Cancer Genome Atlas.
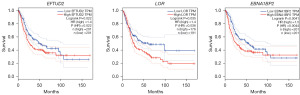
Construction of the ceRNA network
580 crucial DElncRNAs (465 up-regulated and 115 down-regulated) in 387 HGBC and 19 nearby non-cancer bladder tissues, as well as 353 significant DEmiRNAs (279 up-regulated and 74 down-regulated) in 391 HGBC and 19 nearby non-cancer bladder tissues, were discovered using information taken from the TCGA database (Figure S2). Combining the results from the TargetScan and miRDB databases, a total of 642 miRNAs related to the hub genes were predicted (Table S4). Following that, we identified the DEmiRNAs that exhibited interactions with both hub genes and DElncRNAs (available online: https://cdn.amegroups.cn/static/public/tcr-23-98-4.xlsx), and utilized them to build a ceRNA network consisting of 48 lncRNA nodes, 16 miRNA nodes, and 18 mRNA nodes (Figure 7A). Furthermore, we selected respectively the down-regulated miRNAs and up-regulated miRNAs in the ceRNA network to construct the specific ceRNA regulation network (Figure 7B). The results revealed that FLG could be down-regulated by hsa-miR-508 and hsa-miR-32B, and DKC1 could be up-regulated by hsa-miR-150, which was mediated by multiple differentially expressed lncRNAs.
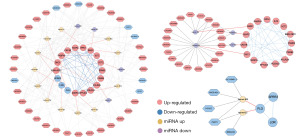
Analysis of SNRPG and DKC1 at the cellular level of BC
The urine samples of HGBC patients showed SNRPG, EBNA1BP2, and DKC1 as the top three up-regulated hub genes. The tumor tissues also depicted considerable upregulation of these genes, where SNRPG and DKC1 portrayed higher upregulation than EBNA1BP2. Analysis of the PPI network revealed that SNRPG had the highest node degree at 18, while EBNA1BP2 demonstrated a degree of 10, which ranked 16th, lower than DKC1 (node degree =13). Thus, we selected SNRPG and DKC1 as the urine biomarkers with the most potential for diagnostic potential for subsequent analysis.
Using data obtained from the CCLE and GEPIA databases, SNRPG and DKC1 were found to be overexpressed in multiple tumor cell lines and tissue types (Figure S3). Based on the analysis using data extracted from the CCLE database, the co-expressed genes for SNRPG (412 positively and 189 negatively) and DKC1 (648 positively and 283 negatively) were identified with a correlation coefficient >0.5 or <−0.5 and P value <0.01 (available online: https://cdn.amegroups.cn/static/public/tcr-23-98-5.xlsx). The top 20 positively and negatively co-expressed genes for SNRPG and DKC1 were respectively illustrated in a heatmap (Figure 8A,8B). Notably, strong correlations were observed between SNRPG and other hub genes, including DKC1, CCT2, EMG, ILF2 and EFTUD2 (Figure 8C). DKC1 also showed a high correlation with EMG1, ILF2, CCT2, H2AFZ and EFTUD2 (Figure 8D). The upregulation of SNRPG and DKC1 in HGBC cells were verified using qRT-PCR (Figure 8E). Additionally, the representative signaling pathways significantly enriched in SNRPG and DKC1 up-regulation or down-regulation phenotypes were determined using GSEA (Figure 9 and Table S5).
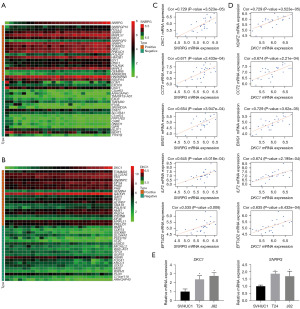
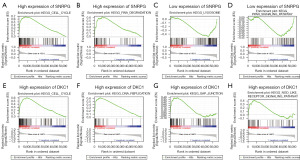
Discussion
Urine sampling is easily available and cost-effective, making it a promising non-invasive method to diagnose BC. However, obtaining urine biomarkers with high sensitivity and specificity is an issue that needs further attention. Herein, we analyzed data on urine samples obtained from GSE62080 to identify reasonable DEGs associated with HGBC. The dysregulated DEGs were significantly enriched in various tumor-associated biological processes, such as regulation of mitosis, cell cycle, stem cell differentiation, immune response, response to hypoxia, apoptotic, WNT and NF-κB signaling. KEGG pathway analysis showed that the DEGs were mainly enriched in ribosome, proteasome, biosynthesis of amino acids, HIF-1 signaling pathway and 2-Oxocarboxylic acid metabolism. The interplay between ribosome synthesis and major signaling pathways is of high relevance to tumor biology. Inhibiting the ribosomal function or biosynthesis has toxic effects on tumor growth (20). In addition to the activity and structure of ribosomes, proteasome and acid metabolism are also altered in tumor cells. Amino acid synthesis is essential for cell proliferation, especially in a nutrient-poor microenvironment (21). Hypoxia can strongly activate HIF-1, which is responsible for tumor progression and treatment resistance. Direct targeting HIF-1 signaling could potentially resolve the major barriers related to treatment-refractory cancers (22).
The PPI analysis is vital for comprehending the molecular mechanisms underlying the development of diseases. In this study, revealing PPIs enables the identification of crucial hub genes implicated in the pathogenesis of HGBC. The basic modules of the PPI network and the genes that make up each of them were identified using the MCODE method. These modules not only contribute to several tumorigenesis-related biological process, but also performed essential roles in the processes of keratinocyte and epidermal cell differentiation, regulation of RNA processing and stabilization, tumor necrosis factor-mediated signaling pathway, etc. Subsequently, by analyzing the effect of DEGs on the survival of patients with HGBC, an optimal risk model consisting of eight genes was developed to predict the patient outcome, including CRYAB, EMP1, EFTUD2, FASN, KDELR2, VWF, GAPDH and BLCAP. Overexpressed or mutant CRYAB (23), EMP1 (24), EFTUD2 (25), FASN (26), KDELR2 (27), VWF (28) and GAPDH (29) have been found to promote tumor growth and cell motility. In various malignancies, an increase in these genes commonly indicates poor prognosis, which is consistent with our predictions in BC. The overexpression of BLCAP is relevant to tumor growth inhibition and promotion of tumor cell apoptosis. BLCAP expression is frequently down-regulated in BC, correlating inversely with patient survival as a significant prognostic factor (30). As predicted, BLCAP can be viewed as a protective factor, contributing to longer survival.
After intersecting the DEGs obtained from both GSE62080 and the TCGA-HGBC datasets, 176 genes that exhibited similar expression trends in both datasets were identified. These genes have shown potential as a distinguisher between HGBC and benign cells in both urine sediments and solid tissue samples. Subsequently, by performing PPI analysis and observing the fold changes of DEGs expression in urine and tissue samples, we identified 18 hub genes, including SNRPG, CCT2, POLR2H, RPN1, DKC1, EFTUD2, EMG1, POLR2G, SPRR3, PSMB2, PABPN1, CKS1B, PSMB4, FLG, LOR, EBNA1BP2, ILF2, and H2AFZ. These hub genes exert critical functions in the biological regulation of RNA polymerase, proteasome and ribosome biogenesis in eukaryotes, and the process of RNA splicing, regulation of cell cycle, cell differentiation and telomere maintenance, and various tumor-related pathways, such as fibroblast growth factor receptor signaling, tumor necrosis factor-mediated signaling, and WNT signaling pathway.
We then constructed a ceRNA network using the hub genes. By predicting the interactions among hub genes, miRNAs and lncRNAs, we can gain further insight into the transcriptional-level regulatory mechanism involved in the pathogenesis of HGBC. Of the down-regulated hub genes, only FLG was predicted to be directly regulated by miRNAs, namely hsa-miR-508 and hsa-miR-32. Lenherr et al. demonstrated that miR-32 is down-regulated during the progress from high-grade non-muscle invasive BC to muscle-invasive phenotype, and serves as a crucial mediator in the survival of BC patients (31). There is currently no report available on the expression of miR-508 in BC. Hsa-miR-150 was significantly down-regulated and had a broad interaction network with various differently expressed lncRNAs in our HGBC ceRNA network. One potential mechanism for hsa-miR-150 to carry out its biological function is by interacting with DKC1. Studies have shown that miR-150 is significantly down-regulated in prostate cancer (32), and could be targeted by lncSNHG14, leading to BC progression (33).
SNRPG, EBNA1BP2, and DKC1 were the three most up-regulated hub genes detected in urine samples of HGBC patients, and considerable upregulation was also observed in HGBC tumor tissues. Through PPI analysis, SNRPG was found had the highest degree at 18, DKC1 had a degree of 13, while EBNA1BP2 ranked lower with a degree of 10. Thus, SNRPG and DKC1 were selected as the urine biomarkers with the most potential for diagnostic potential for subsequent analysis. Both SNRPG and DKC1 exhibit a remarkable overexpression in various tumor tissues and malignant cell lines (Figure S3). We further confirmed the high expression of SNRPG and DKC1 in HGBC cells. SNRPG is indispensable for small nuclear ribonucleoprotein biogenesis in both major and minor mRNA splicing pathways, which has been reported to have a rather broad interaction network (34). Although accumulating evidence of varying expression in various cancers makes SNRPG a promising anti-tumor target in PPI-focused drug technology, its oncogenic role stills need to be further explored (35). Moreover, DKC1 has been found to be up-regulated in many human cancers, such as colorectal cancer, prostate cancer, breast cancer, neuroblastoma, and hepatocellular carcinoma, and frequently predicts poor prognosis (36). Meng et al. have previously reported that DKC1 correlates with BC progression via linc01116-DKC1-HOXD8 signaling axis (37).
Further co-expression analysis in BC cell lines revealed that SNRPG and DKC1 exhibit high correlations with other hub genes. Specifically, SNRPG was found to have a strong correlation with DKC1, CCT2, EMG1, ILF2 and EFTUD2, while DKC1 showed a high correlation with EMG1, ILF2, CCT2, H2AFZ and EFTUD2. Multiple metabolism-related signaling, cell cycle, NOD like receptor signaling and RNA degradation pathway were predicted to be involved in phenotypes with high SNRPG and DKC1 expression. NOD-like receptor signaling plays significant role in inflammation-associated tumorigenesis, such as inflammatory bowel disease-associated colorectal cancer, hepatitis associated hepatocellular carcinoma, H. pylori infection-associated gastric cancer, schistosomiasis-associated BC (38). The mRNA degradation pathway acts as a crucial role on oncogene expression and functions of cancer cells (39). Lysosome and PPAR signaling pathway were significantly enriched in phenotypes with low SNRPG expression. Although no relative report has been made on the correlation between SNRPG expression levels and the lysosome and PPAR signaling pathway, nevertheless, data are available on the participation of PPAR ligands in the modulation of immune responses to tumors (40,41).
This study has some limitations. Firstly, further experiments are necessary to validate our findings and investigate the biological function of the hub genes. Besides, the relatively limited sample size and lack of data from different genetic backgrounds may affect the gene expression for HGBC. Moreover, characteristics including gender, age, clinical stage were not accounted in our study, which would result in some biological information loss. To improve the clinical application value of the biomarkers, extensive validation research is required to determine the diagnostic and prognostic value of these hub genes. These research findings will provide theoretical basis and data support to develop highly specific and sensitive non-invasive urine detection techniques for HGBC.
Conclusions
Through identifying DEGs in urine and tumor tissue samples from HGBC patients, we developed an optimal risk model to predict patient outcomes. Additionally, we obtained 18 hub genes and their corresponding ceRNA regulatory network were obtained, which may provide theoretical basis for the development of effective non-invasive detection and treatment strategies. Further research is necessary to explore the clinical applications of these findings.
Acknowledgments
Funding: This work was supported by
Footnote
Reporting Checklist: The authors have completed the MDAR reporting checklist. Available at https://tcr.amegroups.com/article/view/10.21037/tcr-23-98/rc
Peer Review File: Available at https://tcr.amegroups.com/article/view/10.21037/tcr-23-98/prf
Conflicts of Interest: All authors have completed the ICMJE uniform disclosure form (available at https://tcr.amegroups.com/article/view/10.21037/tcr-23-98/coif). The authors have no conflicts of interest to declare.
Ethical Statement: The authors are accountable for all aspects of the work in ensuring that questions related to the accuracy or integrity of any part of the work are appropriately investigated and resolved. The study was conducted in accordance with the Declaration of Helsinki (as revised in 2013).
Open Access Statement: This is an Open Access article distributed in accordance with the Creative Commons Attribution-NonCommercial-NoDerivs 4.0 International License (CC BY-NC-ND 4.0), which permits the non-commercial replication and distribution of the article with the strict proviso that no changes or edits are made and the original work is properly cited (including links to both the formal publication through the relevant DOI and the license). See: https://creativecommons.org/licenses/by-nc-nd/4.0/.
References
- Siegel RL, Miller KD, Fuchs HE, et al. Cancer statistics, 2022. CA Cancer J Clin 2022;72:7-33. [Crossref] [PubMed]
- Lenis AT, Lec PM, Chamie K, et al. Bladder Cancer: A Review. JAMA 2020;324:1980-91. [Crossref] [PubMed]
- Yun SJ, Kim SK, Kim WJ. How do we manage high-grade T1 bladder cancer? Conservative or aggressive therapy? Investig Clin Urol 2016;57:S44-51. [Crossref] [PubMed]
- Crocetto F, Barone B, Ferro M, et al. Liquid biopsy in bladder cancer: State of the art and future perspectives. Crit Rev Oncol Hematol 2022;170:103577. [Crossref] [PubMed]
- Tan WS, Tan WP, Tan MY, et al. Novel urinary biomarkers for the detection of bladder cancer: A systematic review. Cancer Treat Rev 2018;69:39-52. [Crossref] [PubMed]
- Soorojebally Y, Neuzillet Y, Roumiguié M, et al. Urinary biomarkers for bladder cancer diagnosis and NMIBC follow-up: a systematic review. World J Urol 2023;41:345-59. [Crossref] [PubMed]
- Jiang P, Sinha S, Aldape K, et al. Big data in basic and translational cancer research. Nat Rev Cancer 2022;22:625-39. [Crossref] [PubMed]
- Edgar R, Domrachev M, Lash AE. Gene Expression Omnibus: NCBI gene expression and hybridization array data repository. Nucleic Acids Res 2002;30:207-10. [Crossref] [PubMed]
- Tang Z, Kang B, Li C, et al. GEPIA2: an enhanced web server for large-scale expression profiling and interactive analysis. Nucleic Acids Res 2019;47:W556-60. [Crossref] [PubMed]
- Barretina J, Caponigro G, Stransky N, et al. The Cancer Cell Line Encyclopedia enables predictive modelling of anticancer drug sensitivity. Nature 2012;483:603-7. [Crossref] [PubMed]
- Gautier L, Cope L, Bolstad BM, et al. affy--analysis of Affymetrix GeneChip data at the probe level. Bioinformatics 2004;20:307-15. [Crossref] [PubMed]
- Carvalho BS, Irizarry RA. A framework for oligonucleotide microarray preprocessing. Bioinformatics 2010;26:2363-7. [Crossref] [PubMed]
- Gentleman RC, Carey VJ, Bates DM, et al. Bioconductor: open software development for computational biology and bioinformatics. Genome Biol 2004;5:R80. [Crossref] [PubMed]
- Yu G, Wang LG, Yan GR, et al. DOSE: an R/Bioconductor package for disease ontology semantic and enrichment analysis. Bioinformatics 2015;31:608-9. [Crossref] [PubMed]
- Yu G, Wang LG, Han Y, et al. clusterProfiler: an R package for comparing biological themes among gene clusters. OMICS 2012;16:284-7. [Crossref] [PubMed]
- Subramanian A, Tamayo P, Mootha VK, et al. Gene set enrichment analysis: a knowledge-based approach for interpreting genome-wide expression profiles. Proc Natl Acad Sci U S A 2005;102:15545-50. [Crossref] [PubMed]
- Franceschini A, Szklarczyk D, Frankild S, et al. STRING v9.1: protein-protein interaction networks, with increased coverage and integration. Nucleic Acids Res 2013;41:D808-15. [Crossref] [PubMed]
- Bandettini WP, Kellman P, Mancini C, et al. MultiContrast Delayed Enhancement (MCODE) improves detection of subendocardial myocardial infarction by late gadolinium enhancement cardiovascular magnetic resonance: a clinical validation study. J Cardiovasc Magn Reson 2012;14:83. [Crossref] [PubMed]
- Aho K, Derryberry D, Peterson T. Model selection for ecologists: the worldviews of AIC and BIC. Ecology 2014;95:631-6. [Crossref] [PubMed]
- Eberhard R, Stergiou L, Hofmann ER, et al. Ribosome synthesis and MAPK activity modulate ionizing radiation-induced germ cell apoptosis in Caenorhabditis elegans. PLoS Genet 2013;9:e1003943. [Crossref] [PubMed]
- Vettore L, Westbrook RL, Tennant DA. New aspects of amino acid metabolism in cancer. Br J Cancer 2020;122:150-6. [Crossref] [PubMed]
- Fallah J, Rini BI. HIF Inhibitors: Status of Current Clinical Development. Curr Oncol Rep 2019;21:6. [Crossref] [PubMed]
- Tao X, Cheng L, Li Y, et al. Expression of CRYAB with the angiogenesis and poor prognosis for human gastric cancer. Medicine (Baltimore) 2019;98:e17799. [Crossref] [PubMed]
- Lin B, Zhang T, Ye X, et al. High expression of EMP1 predicts a poor prognosis and correlates with immune infiltrates in bladder urothelial carcinoma. Oncol Lett 2020;20:2840-54. [Crossref] [PubMed]
- Tu M, He L, You Y, et al. EFTUD2 maintains the survival of tumor cells and promotes hepatocellular carcinoma progression via the activation of STAT3. Cell Death Dis 2020;11:830. [Crossref] [PubMed]
- Abdelrahman AE, Rashed HE, Elkady E, et al. Fatty acid synthase, Her2/neu, and E2F1 as prognostic markers of progression in non-muscle invasive bladder cancer. Ann Diagn Pathol 2019;39:42-52. [Crossref] [PubMed]
- Liao Z, She C, Ma L, et al. KDELR2 Promotes Glioblastoma Tumorigenesis Targeted by HIF1a via mTOR Signaling Pathway. Cell Mol Neurobiol 2019;39:1207-15. [Crossref] [PubMed]
- Patmore S, Dhami SPS, O'Sullivan JM. Von Willebrand factor and cancer; metastasis and coagulopathies. J Thromb Haemost 2020;18:2444-56. [Crossref] [PubMed]
- Sirover MA. Pleiotropic effects of moonlighting glyceraldehyde-3-phosphate dehydrogenase (GAPDH) in cancer progression, invasiveness, and metastases. Cancer Metastasis Rev 2018;37:665-76. [Crossref] [PubMed]
- Gromova I, Svensson S, Gromov P, et al. Identification of BLCAP as a novel STAT3 interaction partner in bladder cancer. PLoS One 2017;12:e0188827. [Crossref] [PubMed]
- Lenherr SM, Tsai S, Silva Neto B, et al. MicroRNA Expression Profile Identifies High Grade, Non-Muscle-Invasive Bladder Tumors at Elevated Risk to Progress to an Invasive Phenotype. Genes (Basel) 2017;8:77. [Crossref] [PubMed]
- Paunescu IA, Bardan R, Marcu A, et al. Biomarker Potential of Plasma MicroRNA-150-5p in Prostate Cancer. Medicina (Kaunas) 2019;55:564. [Crossref] [PubMed]
- Li J, Wang AS, Wang S, et al. LncSNHG14 promotes the development and progression of bladder cancer by targeting miRNA-150-5p. Eur Rev Med Pharmacol Sci 2019;23:1022-9. [PubMed]
- Stark C, Breitkreutz BJ, Reguly T, et al. BioGRID: a general repository for interaction datasets. Nucleic Acids Res 2006;34:D535-9. [Crossref] [PubMed]
- Mabonga L, Kappo AP. The oncogenic potential of small nuclear ribonucleoprotein polypeptide G: a comprehensive and perspective view. Am J Transl Res 2019;11:6702-16. [PubMed]
- Elsharawy KA, Mohammed OJ, Aleskandarany MA, et al. The nucleolar-related protein Dyskerin pseudouridine synthase 1 (DKC1) predicts poor prognosis in breast cancer. Br J Cancer 2020;123:1543-52. [Crossref] [PubMed]
- Meng L, Xing Z, Guo Z, et al. LINC01106 post-transcriptionally regulates ELK3 and HOXD8 to promote bladder cancer progression. Cell Death Dis 2020;11:1063. [Crossref] [PubMed]
- Liu P, Lu Z, Liu L, et al. NOD-like receptor signaling in inflammation-associated cancers: From functions to targeted therapies. Phytomedicine 2019;64:152925. [Crossref] [PubMed]
- Burgess HM, Mohr I. Cellular 5'-3' mRNA exonuclease Xrn1 controls double-stranded RNA accumulation and anti-viral responses. Cell Host Microbe 2015;17:332-44. [Crossref] [PubMed]
- Zeng W, Yin X, Jiang Y, et al. PPARα at the crossroad of metabolic-immune regulation in cancer. FEBS J 2022;289:7726-39. [Crossref] [PubMed]
- Wagner N, Wagner KD. Peroxisome Proliferator-Activated Receptors and the Hallmarks of Cancer. Cells 2022;11:2432. [Crossref] [PubMed]