Transcriptional profiles reveal histologic origin and prognosis across 33 The Cancer Genome Atlas tumor types
Highlight box
Key findings
• We established a new pipeline to investigate the tumor expression characteristics through data mining of tumor tissue-specific gene sets (TTSGs).
• We confirmed that there is correlation between this signature index with cell stemness and immune microenvironments, which might impact on progress and prognosis of certain cancers.
What is known and what is new?
• There has been obvious progress in researches on the DNA mutational signatures that can be used for evaluating tumor prognosis, drug resistance, and tumor cell percentage.
• We presented a new method to find TTSGs with a size of 200 genes between different tumor tissues and to reflect and quantify tumor tissue specificity and stemness.
What is the implication, and what should change now?
• The signature could be used as biomarkers in tumor diagnosis or for prognosis prediction.
Introduction
Malignant tumors are diverse and complex diseases with molecular changes on genomic, transcriptomic, proteomic and epigenetic levels and are highly variable in histological features and clinical prognoses (1). This complexity creates a challenge in developing tumor molecular signatures and identifying methods that are clinically useful with respect to prognosis or prediction (2,3). In recent years, with the rapid development of next generation sequencing (NGS), there has been obvious progress in researches on the DNA mutational signatures of somatic mutations that can be used for evaluating tumor prognosis, drug resistance, and tumor cell percentage (4,5). However, due to the spatiotemporal complexity of gene expression and tumor evolution, tumor molecular signature studies at the transcriptome level are relatively unfolding (6).
The Cancer Genome Atlas (TCGA) is an open access cancer database that provides an integrated platform for studying tumors from multiple perspectives in large cohorts (7). Based on TCGA, an overall understanding of pan-cancer levels in the development of tumors was obtained, which contributed to the study of mRNA signatures (8). Some progress has been made in the study of tumor mRNA signatures for cell-of-origin patterns, oncogenic processes, mRNA methylation and signaling pathways through TCGA data (9-12). These studies have not only extended the current understanding of the nature of tumors but also provided a reference for clinical diagnosis, treatment and prognosis (13). On the other hand, advances in single-cell RNA sequencing (scRNA-seq) have allowed for novel perspectives of transcriptome research (14). SCPortalen is a single-cell centric database constructed for data mining, in which the expression data from scRNA-seq are collected and rearranged for a more convenient accession (15).
The widespread application of deep learning algorithms in recent years has brought new methods for performing data-intensive bioinformatics, which have been applied to medical image recognition, clinical data mining and gene expression studies (16). t-distributed Stochastic Neighbor Embedding (t-SNE) is a non-parametric algorithm for dimensionality reduction that is particularly well suited for the visualization of high-dimensional datasets (17). t-SNE was used for the visualization of normal tissue expression data (18,19). The k-nearest neighbors (kNN) algorithm, among the simplest of all machine learning algorithms as a clustering method used for classification and regression, was applied for the classification of large cohorts of tumors (20). These two methods are large data-based and appropriate for TCGA expression data.
Using existing TCGA and single-cell sequencing data, we sought to find a new set of tumor molecular features that are more effective, intuitive, and able to be related to clinical outcomes. It was reaveled that one of the biological characteristics of tumors is the reduction in tissue specificity and gain of cellular dedifferentiation (21). Here, we present a new method to find tumor tissue-specific gene sets (TTSGs) with a size of 200 genes between different tumor tissues and to reflect tumor tissue stemness, immune microenvironment and radiotherapy resistance. We present this article in accordance with the MDAR reporting checklist (available at https://tcr.amegroups.com/article/view/10.21037/tcr-23-234/rc).
Methods
Data preparation
RNA-seq whole-transcriptome expression data for all 33 cancer cohorts from TCGA project (https://tcga-data.nci.nih.gov/tcga/), including 9,875 tumors and 721 normal solid tissues, were obtained via the Genomic Data Commons (GDC) client tool. The three main types of tumor tissues contained in TCGA were primary tumor, metastatic tumor, and recurrent tumor. The abbreviations and total number of specimens of each tumor types were shown in Table 1. Only primary tumors of 33 tumor types and metastatic tumors of skin cutaneous melanoma (SKCM) were with more than 20 samples recorded in TCGA and were included in this study. All TCGA tumors were divided into seven pan-cancer groups according to previous studies (9,22). For all cancer cohorts, the raw expression fragments per kilobase per million mapped reads (FPKM) data for all 60,483 transcripts were collected and transformed into an m × n gene expression matrix where rows (m) are mRNA transcript Ensembl IDs and columns (n) were samples.
Table 1
Pan-cancer type | Abbreviations | Primary tumor (n) | Normal tissue (n) | Histology type (full name) |
---|---|---|---|---|
Pan-squamous | BLCA | 411 | 19 | Bladder urothelial carcinoma |
CESC | 304 | 3 | Cervical squamous cell carcinoma and endocervical adenocarcinoma | |
ESCA | 161 | 11 | Esophageal carcinoma | |
HNSC | 500 | 44 | Head and neck squamous cell carcinoma | |
LUSC | 501 | 49 | Lung squamous cell carcinoma | |
Pan-gyn | BRCA | 1,097 | 113 | Breast invasive carcinoma |
OV | 374 | 0 | Ovarian serous cystadenocarcinoma | |
UCEC | 547 | 35 | Uterine corpus endometrial carcinoma | |
UCS | 57 | 0 | Uterine carcinosarcoma | |
Pan-adenocarcinoma | CHOL | 36 | 0 | Cholangiocarcinoma |
COAD | 469 | 41 | Colon adenocarcinoma | |
LUAD | 524 | 59 | Lung adenocarcinoma | |
PAAD | 177 | 4 | Pancreatic adenocarcinoma | |
PRAD | 498 | 52 | Prostate adenocarcinoma | |
READ | 166 | 10 | Rectum adenocarcinoma | |
STAD | 375 | 32 | Stomach adenocarcinoma | |
Pan-hem | DLBC | 48 | 0 | Lymphoid neoplasm diffuse large B cell lymphoma |
LAML | 151 | 0 | Acute myeloid leukemia | |
THYM | 119 | 2 | Thymoma | |
Pan-neuronal | GBM | 155 | 5 | Glioblastoma multiforme |
LGG | 511 | 0 | Brain lower-grade glioma | |
PCPG | 178 | 3 | Pheochromocytoma and paraganglioma | |
SKCM* | 101 | 0 | Skin cutaneous melanoma | |
UVM | 80 | 0 | Uveal melanoma | |
Pan-kidney | KICH | 65 | 24 | Kidney chromophobe |
KIRC | 534 | 73 | Kidney renal clear cell carcinoma | |
KIRP | 288 | 32 | Kidney renal papillary cell carcinoma | |
Others | ACC | 79 | 0 | Adrenocortical carcinoma |
LIHC | 371 | 50 | Liver hepatocellular carcinoma | |
MESO | 87 | 0 | Mesothelioma | |
SARC | 259 | 2 | Sarcoma | |
TGCT | 150 | 0 | Testicular germ cell tumors | |
THCA | 502 | 58 | Thyroid carcinoma |
*, 369 metastatic tumor samples of SKCM also included. TCGA, The Cancer Genome Atlas.
The respective survival status, gender, clinic stage and outcome information for all cases were extracted from TCGA clinical data. The single-cell RNA-seq FPKM data of 124 individual libraries of human pre-implantation embryos and human embryonic stem cells (hESCs) were downloaded from the SCPortalen database (http://single-cell.clst.riken.jp/) under accession number GSE36552 (23). This study was conducted in accordance with the Declaration of Helsinki (as revised in 2013).
Tumor tissue-specific gene selection
To obtain an mRNA molecular signature that can reflect the specificity of tumor histology types, a gene set was structured with the following characteristics: the difference in expression levels within each tumor type was relatively insignificant, but the expression levels between different tumor types were significantly different. This group of genes reduced the impacts of age, gender, ethnicity, environment, and tumor stage on the tumor transcriptome, and the differences were mainly reflected in distinct histological features. It was significant to further study the molecular characteristics of different tumors and their clinical value for diagnosis and prognosis.
First, the mean, standard deviation (SD), and coefficient of variance (CV) of FPKM were calculated for each transcript in all samples from each tumor, and the transcripts with CV <25% in ascending order of CV and an average FPKM >1.0 were selected to establish subsets of each tumor. The intersection of each tumor subset was called for the next step of filtering. Second, the mean, SD, and CV of the FPKM values of each gene at this intersection were calculated. The CV in descending order of the first 200 genes was screened to form TTSGs. The number of genes in TTSGs varied, and different sizes of TTSGs were tested according to the analysis.
After z-score normalization of the expression matrix by TTSGs, a heatmap of 9,875 tumor expression data for 200 TTSGs was generated to display the expression profiles and features of each tumor. To outline the potential functional features of TTSGs, a gene ontology (GO) enrichment analysis was performed to reveal the functional distribution characteristics of TTSGs in three gene ontologies of biological processes, molecular functions and cellular components. Pathway enrichment analysis based on the Kyoto Encyclopedia of Genes and Genomes (KEGG) was performed to find multiple cell pathways in TTSGs. P value <0.05 was used as the cut-off.
Visualization of tumor classification using t-SNE
The t-SNE algorithm was a non-parametric and non-linear dimensionality reduction method published in 2008 for the visualization of high-dimensional data. Compared with principal component analysis (PCA), t-SNE had a shorter history in the study of tumor expression profiles and a rapid development trend in recent years (24).
To visualize the classification of tumors, t-SNE was performed to project the expression of TTSGs in each individual sample to a 2-dimensional map. For the t-SNE analysis, different sizes from 50 to 200 of TTSGs were tested to validate the classification effect of this model. Dimensionality reduction and visualization using PCA were also performed for comparison with the t-SNE method. The performance of t-SNE was fairly robust under different settings of the perplexity, which depends on the density of the data. In our study, the key parameter perplexity of the t-SNE model was set to 30–80 to fit an optimal embedding and classification effect. t-SNE was repeated with the same data and parameters to select the visualization with the optimal classification effect as the final visualization.
Validation of classification capability using kNN
To estimate the potential capability of TTSGs for tumor molecule signature identification, a kNN model was established for tumor discriminant analysis. The training group and validation group, which contained 70% and 30% of each tumor sample, respectively, were selected randomly and with no return. The kNN model was fitted using training group data and subsequently used to predict the tumor categories of specimens from the validation group. The accuracy of the prediction for the validation group from each tumor was generated by calculating the ratio of correctly predicted counts and the number of all samples. Parameter k was set to 3 to adjust for highest discriminant accuracy.
Dissimilarity between embryos and tumors
To explore the relationship between tissue specificity, differentiation and prognosis, the single-cell sequencing data of human pre-implantation embryos was downloaded as a reference for comparison with tumor cells. The developmental stages of embryo cells included oocyte (3 cells), zygote (3 cells), 2-cell stage (6 cells), 4-cell stage (12 cells), 8-cell stage (24 cells), morula stage (16 cells), late blastocyst (30 cells) and hESC (34 cells) and calculated the Euclidean distance of TTSGs from undifferentiated early embryos to each sample. The median expression FPKM of these 124 TTSGs was set as an undifferentiated reference sample, which represented the overall expression levels of TTSGs in embryo cells. The multidimensional Euclidean distance between the expression of TTSGs of the remaining tumor samples and the reference embryo cells was defined as TTSG index and calculated by using the following equation:
where n was the size of TTSGs, and x was the FPKM value of each gene in TTSGs from each sample. Additionally, y was the median FPKM of TTSGs in all embryo cells. The higher the dissimilarity, the higher the difference between the expression features of embryos and tumors.
For each tumor, the samples were divided into two groups based on their dissimilarity: the top 50% in the high group and the bottom 50% in the low group. The overall survival difference between the two groups was compared using log-rank test, and two-tailed t-test. P value <0.05 was considered statistically significant in this study.
Correlation between cell stemness, immune cell fraction and TTSG index
The mRNA signature index (mRNAsi) represents the quantification of cell stemness and dedifferentiation using one-class logistic regression (OCLR) (25), a machine learning algorithm that provides a scalable approach to generate cell type signatures (22). To evaluate the association of the mRNAsi with embryo dissimilarity, these two signature indexes were compared according to tumor types.
The mRNAsi for each sample was scored based on Spearman’s rank correlation coefficient between the FPKM of each sample and the mRNA signature weight, which consisted of 12,965 genes selected using OCLR (https://gdc.cancer.gov/about-data/publications/PanCanStemness-2018). CIBERSORT method was used to evaluate fractions of 22 types of immune cells and total leukocytes (26). The correlation of each immune cell fraction and immune cell activation with mRNAsi and embryo tumor distance was calculated to compare the characteristics of immune microenvironments.
Statistical analysis and available software
R 3.6.2 (https://www.r-project.org/) was used in this study to aggregate, organize, matrix compute, and visualize all data. T-test, log-rank test, kNN and visualization of results was performed using R. t-SNE was performed using the R package Rtsne 0.15. All applied software and adapted data resources were shown in Table S1.
Results
Selection of TTSGs
The clinical information and a total of 60,483 transcript profiles, including mRNA and long non-coding RNA (lncRNA), for 9,875 tumor samples from 33 cancer types were accessed from TCGA database (Table 1).
A set of genes was screened and selected from TTSGs by the average and CV distribution of mRNA expression profiles from different tumors. According to our method, there was no clear-cut limit to the size of the TTSGs; however, the effect and reliability of the classification model might be reduced by too small TTSGs. A total of 200 genes were selected as the upper limit of the TTSGs for further analysis. Detailed information for each TTSG can be found in table available at https://cdn.amegroups.cn/static/public/10.21037tcr-23-234-1.xlsx. To investigate the gene selection of TTSGs and other cancer related tumor signatures, the Cancer Driver gene set (Bailey et al. 2018) (27), the Pathogenic Germline Variants gene set (Huang et al. 2018) (28) and the ESTIMATE gene set (Yoshihara et al. 2013) (29) were chosen for comparison (Figure 1A). The first two signatures were DNA mutation based, and the ESTIMATE signature was mRNA expression based. TTSGs as a gene set for classification had less intersection with mutational signatures.
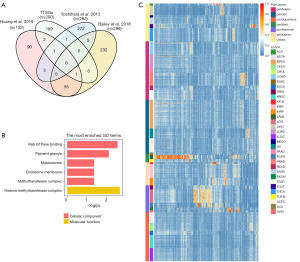
In order to outline the potential function of the TTSGs, GO enrichment analysis and pathway analysis were performed for the 200 genes. The enriched GO terms (P<0.05) were shown in Figure 1B. None of the cell component (CC) GO terms or KEGG pathways were enriched. The GO enrichment of TTSGs indicates that there was dispersed functional distribution within TTSGs. Expression levels of TTSGs in all cancer types were shown in Figure 1C.
Classification and visualization of tumors
To classify and visualize tumors using TTSGs, t-SNE was performed to reduce the high-dimensional data for TTSG expression FPKM values to 2-dimensional data. The size of the TTSGs was set to 50, 100 and 200 to explore the classification capability at different sizes of TTSGs for comparing t-SNE with PCA. When the size of the TTSGs was 50, some tumors were gathered into clusters by t-SNE but could not be separated from each other, and the classification was unclear. The classification effect of t-SNE was optimal when 200 genes were used. The tumors were separated with poor effects by the PCA method and were not more clearly separated when the size of the TTSGs increased (Figure S1).
All individual samples of each tumor type were embedded by t-SNE. Each tumor type was clearly divided into separate clusters, except for head and neck squamous cell carcinoma (HNSC), lung squamous cell carcinoma (LUSC), cervical squamous cell carcinoma and endocervical adenocarcinoma (CESC), sarcoma (SARC), lung adenocarcinoma (LUAD) and pancreatic adenocarcinoma (PAAD), which were dispersed into their respective regions but could not be completely separated into independent clusters, suggesting that these tumor types have similar TTSG expression profiles (Figure 2A). Stomach adenocarcinoma (STAD) and esophageal carcinoma (ESCA), rectum adenocarcinoma (READ) and colon adenocarcinoma (COAD), had overlapped clusters, indicating a high degree of similarity in their TTSG expression profiles. A few outliers existed in the graph, especially in the central region, revealing that this classification method is difficult to correctly classify a few samples with special expression values. In the pan-cancer view, pan-squamous cancers were embedded into a co-cluster while other pan-cancer types were distributed separately (Figure 2B).
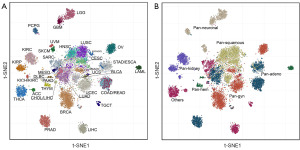
Normal tissue samples of tumor origin were embedded together with tumors of the same histological origin. The classification using TTSGs was also effective when distinguishing normal and primary tumors. Separated clusters of normal tissue were formed in LUAD/LUSC, COAD/READ, breast invasive carcinoma (BRCA) and pan-kidney tumors. Normal liver tissue was clustered but embedded into liver hepatocellular carcinoma (LIHC) tumor clusters. Metastatic tumors of SKCM were mixed into primary tumors, indicating that there were indistinct expression features for t-SNE to form independent clusters (Figure 3A).
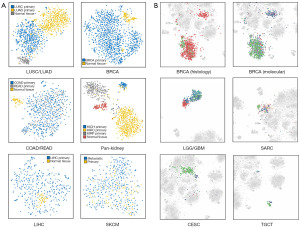
However, the pathology or molecular types of some tumors from TCGA were heterogeneous. For example, CESC consisted of adenosquamous, squamous cell carcinoma and adenocarcinoma, each type characterized by different expression profiles. To reveal the classification potential of subtypes of tumors, outlier clusters generated within BRCA, CESC, brain lower-grade glioma (LGG)/glioblastoma multiforme (GBM), SARC and testicular germ cell tumors (TGCTs) were illustrated (Figure 3B). The samples with molecular subtypes of basal-like breast cancers (ER−, PR−, HER-2−) were enriched for the sub-cluster of infiltrating ductal carcinoma, close to LUAD and LUSC, while luminal and HER-2-amplified subtypes were co-clustered. LGG was classified into three types: astrocytoma, oligoastrocytoma and oligodendroglioma, and projected to clusters close to GBM. SARC formed an isolated cluster mainly consisting of desmoid tumor, while other types of SARC showed scattered and mixed plots. By classification of the pathology types, a more distinct identification of inter-tumor expression features was observed, indicating the classification capacity of subtypes of tumors by TTSGs.
kNN discrimination analysis
As a supervised deep learning algorithm, kNN was used for discrimination analysis of the tumor samples (Figure 4A). Tumors with an accuracy rate lower than 0.9 include CESC (0.839), cholangiocarcinoma (CHOL) (0.846), COAD (0.816), HNSC (0.838), kidney chromophobe (KICH) (0.838), LUSC (0.858), PAAD (0.664) and READ (0.822). The accuracy of other tumors was higher than 0.9. The mismatching rate between two tumors higher than 10% involved COAD to READ (0.148) and READ to COAD (0.178). Similar to the results of the classification via t-SNE, mismatching between READ, COAD, ESCA and STAD reflected the similarity of TTSG expression in the above tumors, while the discrimination of other tumors was relatively accurate. The matrix of accuracy of each tumor discrimination was shown in table available at https://cdn.amegroups.cn/static/public/10.21037tcr-23-234-2.xlsx.
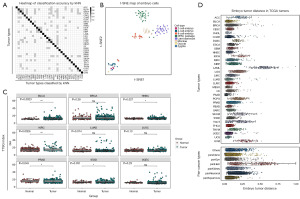
TTSG index
The expression levels of TTSGs in 124 embryo cells were dimensionally reduced and visualized by t-SNE (Figure 4B). A clear and progressive development pathway was observed from oocyte to late blastocyst cells, revealing that the changes in expression features in embryo development were reflected in TTSGs, which were significantly distinct in tumors.
However, the TTSG index was significantly lower in normal tissues of lung, bladder, endometrium, rectum/colon, prostate and stomach than in tumors derived from these tissues (Figure 4C). These observations, which conflicted with the expectations of the analysis, revealed that there was a restriction on comparing tumors with non-tumor tissues using TTSG index.
Euclidean distances between each tumor sample and the expression of TTSGs in morula and blastocyst stage cells were calculated as the dissimilarity between tumors and embryos (Figure 4D). The TTSG indexes of each analyzed sample were shown in table available at https://cdn.amegroups.cn/static/public/10.21037tcr-23-234-3.xlsx, suggesting that pan-squamous cancers had lower embryo distances than other pan-cancer types (P=2.7e−4). The overall survival outcomes are shown in Figure 5 and table available at https://cdn.amegroups.cn/static/public/10.21037tcr-23-234-4.xlsx. Samples with dissimilarities of less and larger than the median embryo tumor distance in the same tumor were used as the low and high groups. There were relatively small dissimilarities in PAAD, HNSC, bladder urothelial carcinoma (BLCA), LUAD and LUSC, which had poor outcomes. The dissimilarity in adrenocortical carcinoma (ACC), KICH, pheochromocytoma and paraganglioma (PCPG) and TGCT with fewer deaths was relatively large. Both GBM and LGG are of neuroglial cell origin, but the dissimilarity in GBM is smaller than that in LGG. CESC, GBM, kidney renal clear cell carcinoma (KIRC), kidney renal papillary cell carcinoma (KIRP), LGG, LIHC and thymoma (THYM) had significant differences (P<0.05) in the overall survival status of the low and high groups, while the other groups had no significant difference.
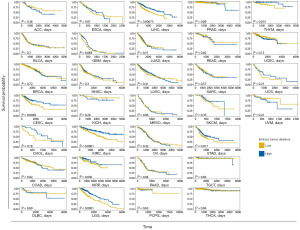
Comparing cell stemness and TTSG index
The mRNAsi of cell stemness was calculated for each case through Spearman’s rank correlation coefficient and compared with TTSG index. Although the mRNAsi was higher in tissues with more stemness and cell dedifferentiation, middle to strong correlations (r>0.4, P<0.05) between TTSG index and mRNAsi were observed in ACC, GBM, KIRC, LGG, LUSC, PCPG, TGCT, and THYM (Figure 6A). There was no significant relationship (|r|<0.2) between the two signature indexes in BRCA, COAD, lymphoid neoplasm diffuse large B cell lymphoma (DLBC), ESCA, KIRP, acute myeloid leukemia (LAML), LIHC, LUAD, READ, thyroid carcinoma (THCA), uterine corpus endometrial carcinoma (UCEC) and uveal melanoma (UVM).
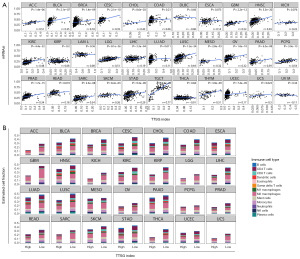
Relationship between TTSG index with immune microenvironment and radiation therapy resistance
To investigate the characteristics of tumor infiltrating immune cells in different embryo tumor distance levels, Immune cell fraction in the high (top half) and low (bottom half) groups of TTSG index were calculated using the CIBERSORT method. The total leucocyte fraction was significantly higher in the low group in all tumor types, except UCEC and LUAD. The increase in the total leucocyte fraction mainly consisted of macrophage M2 cells, which were considered as promoters of tumorigenesis and angiogenesis and were closely related to immunosuppression and prognosis (30). In addition to macrophage M2 cells, other immune cells were also enriched in the high TTSG index group (Figure 6B). Compared with the mRNAsi, the TTSG index had a lower correlation with CD4 memory T cell activation and a higher correlation with dendritic cell activation (Figure 7A). Tissues with higher TTSG index were enriched in dendritic cell activation, which played an important role in presenting tumor antigen and promoting anti-tumor immunity (Figure 7B).
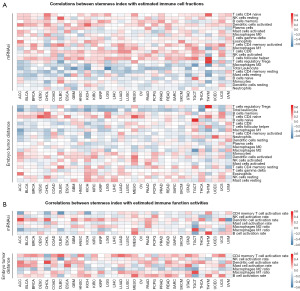
The outcomes of patients with or without radiation therapy in high and low groups were further explored. By survival analysis, in BRCA, GBM, HNSC, STAD and uterine carcinosarcoma (UCS), patients with higher TTSG index had longer overall survival times compared to patients with lower TTSG index (Figure 8). The radiation therapy effect of CESC and LGG was reduced in low group. These results suggested that the TTSG index was correlated with radiation therapy resistance.
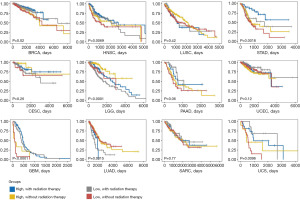
Discussion
The aim of this study was to establish a method for describing tumor expression profiling and segregating samples into biologically relevant groups based upon quantitative dimensions. In the current work, we found a new tumor molecular signature composed of TTSGs that reflected tumor expression profiling in the TCGA database. In particular, the potential effect of TTSGs in tumor identification and classification was validated by visualization and classification of tumor samples using t-SNE and kNN. Furthermore, by calculating the Euclidean distances as the dissimilarity of tumor and embryo cells, it was suggested that TTSGs were valuable in the outcome evaluation of several tumors.
In our observation of the pan-cancer 2D map projected from TTSGs, samples of BRCA, GBM, HNSC, KIRC, LAML, LGG, LIHC, ovarian serous cystadenocarcinoma (OV), PCPG, prostate adenocarcinoma (PRAD), SKCM, THCT, THCA, THYM, and UVM were separated from all other tumor types. In contrast, PAAD, SARC, CESC, and BLCA formed a co-clustering group that was isolated from other tumors. Notably, some tumors with similar origins were difficult to separate and distinguish, for example, COAD and READ, LGG and GBM, STAD and ESCA (adenocarcinoma). The co-cluster of squamous cancers that were not distinguished clearly indicated that squamous cancers had similar molecular features and should be classified by an integrative multiplatform (31,32). It was indicated that the TTSGs were not only able to distinguish different types of tumor samples but also able to uncover potential histological and molecular subtypes within some tumor types. There was unambiguous separation from the molecular types of BRCA: from the HER2-amp subtype and luminal subtype to a basal-like subtype, confirming that there was a large distinction of features between basal-like breast cancer and other molecular types, which could lead to different prognoses and outcomes (33-35).
Several studies have focused on the identification of mRNA biomarkers and the classification of TCGA tumor types in recent years. For a pan-cancer scale view of tumor expression profiling, many clustering and classification algorithms were applied to identify molecular characteristics. Roche et al. selected a set of specific biomarkers and background classification genes and sorted five human tumor types using t-SNE (36). Martínez et al. classified 12 TCGA tumor types using hierarchical clustering according expression data of 1,500 genes (37). Li et al. established a classification strategy based on GA/KNN to classify 9,096 tumor samples from 31 tumor types (20). Hoadley et al. analyzed TCGA data from a 4-experiment platform and found that cell-of-origin dominated the molecular classification of 10,000 tumors from 33 types of cancer using the iCluster and TumorMap algorithm (9). Compared with these studies focused on classification and visualization of tumors, in our study, there are two points of difference. First, the size of the gene set was limited to 200, and all TCGA tumor types were analyzed to explore the minimum size of the gene set for pan-cancer classification. Our results confirmed that tumor expression profiling can be reflected in a small number of genes that represent tumor tissue origin. Second, the visualization method of t-SNE in our study is insensitive to outliers and fitted for large-scale data (38). In our study, t-SNE was effective for processing RNA data, especially when embedding a pan-cancer map of a large sample size. Surprisingly, TTSG expression profiling progressed in a sequential developmental direction from oocytes to late blastocyst cells using the t-SNE method in a 2D space. This observation was confirmed by the original experiment and analysis of the scRNA-seq research, which projected the cells to a 3D space using PCA (23).
Euclidean distances are usually used for quantifying the difference between two vectors of high-dimension data and are applied as the basic approach in some data mining algorithms, for example, hierarchical clustering and ridge regression (39-41). Survival outcomes were subsequently impacted by the dissimilarity in BRCA, KIRC, KIRP, LGG, LIHC, STAD and THYM. There are two potential reasons to explain the irrelevant results observed in other tumors. First, the prognosis of tumors is dominated by multiple factors that have increased weight in cancer treatment, such as therapy scheme, accessibility of surgery, metastasis occurrence and immune microenvironment (42). In our findings, the TTSG index is related to tumor radiation therapy and the immune microenvironment, which are impact factors of overall survival (43). Second, the clinical data were mostly completed in the past 10 years, and the patient follow-up period was not long enough to accumulate adequate differences in survival outcomes of tumor cohorts (44).
The stemness feature of cancer cells, which indicates the degree of oncogenic dedifferentiation, can be evaluated by an mRNAsi based on 12,945 genes (22). However, there is a weak positive correlation between the mRNAsi and the embryo tumor distance in a majority of the 33 types of TCGA tumors, especially in pan-neuronal tumors. This result means there is a conflict within these two signatures potentially because of different immune microenvironments and algorithms. In our research, overall survival outcomes in gliomas (LGG/GBM) of different TTSG index levels conformed with the observation of mRNAsi.
There were several limitations in this study. Firstly, this study is a reanalysis of TCGA data only in silico, without validation and implement using histologic or clinic experiments. Secondly, advanced feature selection algorithms, such as neural network algorithms, were not implemented in selection of TTSG. In future work, experimental verification and algorithm attempts should be conducted to obtain further insights.
Conclusions
In this study, a new pipeline is established to investigate the tumor expression characteristics through data mining of a group of genes with tumor tissue specificity (TTSGs). There is correlation between this signature index with cell stemness and immune microenvironments which might impact on progress and prognosis of certain cancers.
Acknowledgments
The authors thank Zhuyan Huang and Song Xu from Longgang District Maternity & Child Healthcare Hospital of Shenzhen City for providing computing servers to store and process data, and thank TCGA project for its open access to massive cancer resources.
Funding: This work was supported by grants from
Footnote
Reporting Checklist: The authors have completed the MDAR reporting checklist. Available at https://tcr.amegroups.com/article/view/10.21037/tcr-23-234/rc
Peer Review File: Available at https://tcr.amegroups.com/article/view/10.21037/tcr-23-234/prf
Conflicts of Interest: All authors have completed the ICMJE uniform disclosure form (available at https://tcr.amegroups.com/article/view/10.21037/tcr-23-234/coif). The authors have no conflicts of interest to declare.
Ethical Statement: The authors are accountable for all aspects of the work in ensuring that questions related to the accuracy or integrity of any part of the work are appropriately investigated and resolved. The study was conducted in accordance with the Declaration of Helsinki (as revised in 2013).
Open Access Statement: This is an Open Access article distributed in accordance with the Creative Commons Attribution-NonCommercial-NoDerivs 4.0 International License (CC BY-NC-ND 4.0), which permits the non-commercial replication and distribution of the article with the strict proviso that no changes or edits are made and the original work is properly cited (including links to both the formal publication through the relevant DOI and the license). See: https://creativecommons.org/licenses/by-nc-nd/4.0/.
References
- Cancer Genome Atlas Research Network. Integrated genomic characterization of endometrial carcinoma. Nature 2013;497:67-73. [Crossref] [PubMed]
- Alexandrov LB, Nik-Zainal S, Wedge DC, et al. Signatures of mutational processes in human cancer. Nature 2013;500:415-21. [Crossref] [PubMed]
- Büttner FA, Winter S, Stühler V, et al. A novel molecular signature identifies mixed subtypes in renal cell carcinoma with poor prognosis and independent response to immunotherapy. Genome Med 2022;14:105. [Crossref] [PubMed]
- Zengin T, Önal-Süzek T. Analysis of genomic and transcriptomic variations as prognostic signature for lung adenocarcinoma. BMC Bioinformatics 2020;21:368. [Crossref] [PubMed]
- Kamps R, Brandão RD, Bosch BJ, et al. Next-Generation Sequencing in Oncology: Genetic Diagnosis, Risk Prediction and Cancer Classification. Int J Mol Sci 2017;18:308. [Crossref] [PubMed]
- Conesa A, Madrigal P, Tarazona S, et al. A survey of best practices for RNA-seq data analysis. Genome Biol 2016;17:13. [Crossref] [PubMed]
- Cancer Genome Atlas Research Network. The Cancer Genome Atlas Pan-Cancer analysis project. Nat Genet 2013;45:1113-20. [Crossref] [PubMed]
- Muzzi JCD, Magno JM, Cardoso MA, et al. Adrenocortical Carcinoma Steroid Profiles: In Silico Pan-Cancer Analysis of TCGA Data Uncovers Immunotherapy Targets for Potential Improved Outcomes. Front Endocrinol (Lausanne) 2021;12:672319. [Crossref] [PubMed]
- Hoadley KA, Yau C, Hinoue T, et al. Cell-of-origin patterns dominate the molecular classification of 10,000 tumors from 33 types of cancer. Cell 2018;173:291-304.e6. [Crossref] [PubMed]
- Ding L, Bailey MH, Porta-Pardo E, et al. Perspective on oncogenic processes at the end of the beginning of cancer genomics. Cell 2018;173:305-320.e10. [Crossref] [PubMed]
- Sanchez-Vega F, Mina M, Armenia J, et al. Oncogenic Signaling Pathways in The Cancer Genome Atlas. Cell 2018;173:321-337.e10. [Crossref] [PubMed]
- Pu Y, Lu X, Yang X, et al. Estimating the prognosis of esophageal squamous cell carcinoma based on The Cancer Genome Atlas (TCGA) of m6A methylation-associated genes. J Gastrointest Oncol 2022;13:1-12. [Crossref] [PubMed]
- Hutter C, Zenklusen JC. The Cancer Genome Atlas: Creating Lasting Value beyond Its Data. Cell 2018;173:283-5. [Crossref] [PubMed]
- Kim C, Gao R, Sei E, et al. Chemoresistance Evolution in Triple-Negative Breast Cancer Delineated by Single-Cell Sequencing. Cell 2018;173:879-893.e13. [Crossref] [PubMed]
- Abugessaisa I, Noguchi S, Böttcher M, et al. SCPortalen: human and mouse single-cell centric database. Nucleic Acids Res 2018;46:D781-7. [Crossref] [PubMed]
- Koohy H. The rise and fall of machine learning methods in biomedical research. F1000Res 2017;6:2012. [Crossref] [PubMed]
- Xu W, Jiang X, Hu X, et al. Visualization of genetic disease-phenotype similarities by multiple maps t-SNE with Laplacian regularization. BMC Med Genomics 2014;7:S1. [Crossref] [PubMed]
- Taskesen E, Reinders MJ. 2D representation of transcriptomes by t-SNE exposes relatedness between human tissues. PLoS One 2016;11:e0149853. [Crossref] [PubMed]
- Jia X, Liu Y, Han Q, et al. Multiple-cumulative probabilities used to cluster and visualize transcriptomes. FEBS Open Bio 2017;7:2008-20. [Crossref] [PubMed]
- Li L, Weinberg CR, Darden TA, et al. Gene selection for sample classification based on gene expression data: study of sensitivity to choice of parameters of the GA/KNN method. Bioinformatics 2001;17:1131-42. [Crossref] [PubMed]
- Ng SW, Mitchell A, Kennedy JA, et al. A 17-gene stemness score for rapid determination of risk in acute leukaemia. Nature 2016;540:433-7. [Crossref] [PubMed]
- Malta TM, Sokolov A, Gentles AJ, et al. Machine Learning Identifies Stemness Features Associated with Oncogenic Dedifferentiation. Cell 2018;173:338-354.e15. [Crossref] [PubMed]
- Yan L, Yang M, Guo H, et al. Single-cell RNA-Seq profiling of human preimplantation embryos and embryonic stem cells. Nat Struct Mol Biol 2013;20:1131-9. [Crossref] [PubMed]
- Li W, Cerise JE, Yang Y, et al. Application of t-SNE to human genetic data. J Bioinform Comput Biol 2017;15:1750017. [Crossref] [PubMed]
- Sokolov A, Paull EO, Stuart JM. One-class detection of cell states in tumor subtypes. Pac Symp Biocomput 2016;21:405-16. [Crossref] [PubMed]
- Newman AM, Liu CL, Green MR, et al. Robust enumeration of cell subsets from tissue expression profiles. Nat Methods 2015;12:453-7. [Crossref] [PubMed]
- Bailey MH, Tokheim C, Porta-Pardo E, et al. Comprehensive characterization of cancer driver genes and mutations. Cell 2018;173:371-385.e18. [Crossref] [PubMed]
- Huang KL, Mashl RJ, Wu Y, et al. Pathogenic germline variants in 10,389 adult cancers. Cell 2018;173:355-370.e14. [Crossref] [PubMed]
- Yoshihara K, Shahmoradgoli M, Martínez E, et al. Inferring tumour purity and stromal and immune cell admixture from expression data. Nat Commun 2013;4:2612. [Crossref] [PubMed]
- Lan J, Sun L, Xu F, et al. M2 macrophage-derived exosomes promote cell migration and invasion in colon cancer. Cancer Res 2019;79:146-58. [Crossref] [PubMed]
- Hoadley KA, Yau C, Wolf DM, et al. Multiplatform analysis of 12 cancer types reveals molecular classification within and across tissues of origin. Cell 2014;158:929-44. [Crossref] [PubMed]
- Campbell JD, Yau C, Bowlby R, et al. Genomic, pathway network, and immunologic features distinguishing squamous carcinomas. Cell Rep 2018;23:194-212.e6. [Crossref] [PubMed]
- Totoki Y, Saito-Adachi M, Shiraishi Y, et al. Multiancestry genomic and transcriptomic analysis of gastric cancer. Nat Genet 2023;55:581-94. [Crossref] [PubMed]
- Horr C, Buechler SA. Breast Cancer Consensus Subtypes: A system for subtyping breast cancer tumors based on gene expression. NPJ Breast Cancer 2021;7:136. [Crossref] [PubMed]
- Berger AC, Korkut A, Kanchi RS, et al. A comprehensive pan-cancer molecular study of gynecologic and breast cancers. Cancer Cell 2018;33:690-705.e9. [Crossref] [PubMed]
- Roche KE, Weinstein M, Dunwoodie LJ, et al. Sorting five human tumor types reveals specific biomarkers and background classification genes. Sci Rep 2018;8:8180. [Crossref] [PubMed]
- Martínez E, Yoshihara K, Kim H, et al. Comparison of gene expression patterns across 12 tumor types identifies a cancer supercluster characterized by TP53 mutations and cell cycle defects. Oncogene 2015;34:2732-40. [Crossref] [PubMed]
- Dimitriadis G, Neto JP, Kampff AR. t-SNE visualization of large-scale neural recordings. Neural Comput 2018;30:1750-74. [Crossref] [PubMed]
- Shen X, Alam M, Fikse F, et al. A novel generalized ridge regression method for quantitative genetics. Genetics 2013;193:1255-68. [Crossref] [PubMed]
- Pagnuco IA, Pastore JI, Abras G, et al. Analysis of genetic association using hierarchical clustering and cluster validation indices. Genomics 2017;109:438-45. [Crossref] [PubMed]
- Ghosh A, Barman S. Application of Euclidean distance measurement and principal component analysis for gene identification. Gene 2016;583:112-20. [Crossref] [PubMed]
- Taarnhøj GA, Christensen IJ, Lajer H, et al. Risk of recurrence, prognosis, and follow-up for Danish women with cervical cancer in 2005-2013: a national cohort study. Cancer 2018;124:943-51. [Crossref] [PubMed]
- Sorin M, Rezanejad M, Karimi E, et al. Single-cell spatial landscapes of the lung tumour immune microenvironment. Nature 2023;614:548-54. [Crossref] [PubMed]
- Liu J, Lichtenberg T, Hoadley KA, et al. An integrated TCGA pan-cancer clinical data resource to drive high-quality survival outcome analytics. Cell 2018;173:400-416.e11. [Crossref] [PubMed]