A deep learning-based model (DeepMPM) to help predict survival in patients with malignant pleural mesothelioma
Highlight box
Key findings
• A deep learning-based model can provide good prediction of survival in patients with malignant pleural mesothelioma (MPM).
What is known and what is new?
• MPM is a rare disease with limited treatment and a poor prognosis.
• Our newly devised artificial intelligence deep learning tool could predict MPM survival.
What is the implication, and what should change now?
• Deep learning can aid clinical in decision-making.
• Multimode combination therapy is needed for patients with MPM.
Introduction
Malignant pleural mesothelioma (MPM) is a rare malignant tumor derived from pleural mesothelial cells and accounts for 5% of pleural tumors (1). The rate of diagnostic accuracy differs significantly between developed and developing nations, which can influence certain outcomes, including survival (2). According to the classification criteria for pleural tumors published by World Health Organization (WHO) in 2015, the histological subtypes of MPM mainly include epithelioid type, sarcomatoid type, and biphasic (mixed) type, among which epithelioid type is the most common (3). The emergence of MPM has been recently linked to asbestos exposure. Although asbestos has been banned in many countries, the impact of asbestos lingers, leading to a steady increase in the number of MPM cases globally (4). Patients with MPM have a poor prognosis, with the median overall survival (OS) being about 1 year and the overall 5-year survival rate being about 5% (5). Given MPM’s poor prognosis, it would be beneficial to precisely predict survival in patients with MPM to identify potentially advantageous strategies or avoid unnecessary medical complications. Moreover, the pathological classifications of MPM, including the 3 main subtypes of epithelioid, biphasic, and sarcomatoid, have an impact on prognosis. Epithelioid MPM is associated with the most favorable outcomes (median OS about 16 months), sarcomatoid with the worst outcomes (OS around 5 months), and biphasic MPM with intermediate outcomes. Although these pathological subtypes hold some prognostic value, they do not fully account for the complex variability in clinical characteristics related to the survival of patients with MPM.
This study aimed to help predict MPM survival outcomes to aid treatment decisions using a deep learning model based on a combination of tumor, node, and metastasis (TNM) stage; age; sex; history of malignancy; pathology; and treatment methods (surgery, radiotherapy, and chemotherapy).
Despite the TNM staging system of the American Joint Committee on Cancer (AJCC) being widely used among clinicians, it has been argued that it is insufficiently accurate in predicting MPM prognosis due to its sole reliance on TNM status (6). Subsequently, Cox proportional hazard (CPH) regression-based nomograms have been developed by researchers to enhance precision, allowing for better predictive performance than TNM staging (7-9). However, these models show some limitations in time-to-event prediction because the results based on a linear hypothesis cannot reflect the role of nonlinear variables. The rapid development of machine learning and artificial neural networks (ANNs) in recent years have provided considerable advantages for solving multifactor and nonlinear problems (10). Katzman et al. developed DeepSurv theory, combining deep learning and CPH algorithm to predict time-dependent data, which could more precisely incorporate the effects of covariates (11). Many clinical survival prediction issues have been addressed with this algorithm. She et al. (12) developed a deep learning model based on DeepSurv, which showed a good performance in predicting non-small cell lung cancer-specific survival, with an area under the receiver operating characteristics curve (AUC) in the training and validation groups of 0.739 and 0.742, respectively. Similarly, Yu et al. (13) used DeepSurv to develop a deep learning model in rectal adenocarcinoma-specific survival prediction. The consistency indices for training and validation were 0.824 and 0.821, respectively, demonstrating the considerable potential of DeepSurv.
In this study, we analyzed the data of patients with MPM from 2000 to 2019 in the Surveillance, Epidemiology, and End Results (SEER) database. For the first time, we developed and validated a MPM prognosis model based on a neural network, which was formulated into a Windows desktop tool for convenient use by clinicians. We present this article in accordance with the TRIPOD reporting checklist (available at https://tcr.amegroups.com/article/view/10.21037/tcr-23-422/rc).
Methods
Study design and patient data
A search was conducted of the SEER 17 Registries database (2000–2019), yielding the raw data of 13,754 samples obtained after screening with the following inclusion criteria: (I) International Classification of Diseases for Oncology, Third Edition (ICD-O-3) codes for histology and behavior of malignancy were 9050/3 and 9053/3, respectively; and (II) primary sites were the pleura, lung, or mediastinum. These samples were then screened by the following exclusion criteria: (I) without complete AJCC seventh edition TNM stage (mainly diagnosed as MPM before 2010) and (II) records with missing values. Finally, the raw data of 3,130 samples were included in this study. Subsequently, all data were separated into 2 cohorts according to time of diagnosis time: a training cohort (diagnosed in the 2010–2016 period) and a test cohort (diagnosed in the 2017–2019). The training cohort was used to train and construct a deep learning survival prediction model for malignant pleural mesothelioma (DeepMPM), which was validated by training and test cohort. Finally, all data were used to build the CPH regression model to determine the risk factors and protective factors of MPM. All data were submitted on November 2021, and the follow-up cutoff date was December 31, 2019, according to SEER research data description (Figure 1).
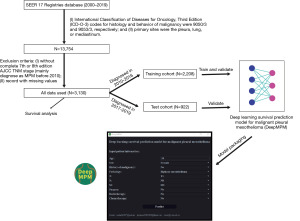
The study was conducted in accordance with the Declaration of Helsinki (as revised in 2013). Given that all data were retrieved from the publicly available SEER database and patients’ private information has been concealed and is not traceable, this retrospective cohort was exempted from ethical considerations and written consent.
Predictive variables and data preprocessing
Based on clinical experience, age, sex, history of malignancy, pathology, TNM stage (according to the AJCC seventh edition), surgery, radiotherapy, and chemotherapy were chosen as predictive clinical features. Due to the SEER record rules, patients more than 100 years old were recorded as being 100 years old. Similarly, a survival time less than 1 month was recorded as 1 month.
Model training and evaluation
Age was considered a numerical variable, and the other clinical features were regarded as categorical variables. The numerical variable was standardized (subtracting the population mean from an individual raw score and then dividing the difference by the population standard deviation). Categorical variables were converted into dummy variables, which involved replacing certain records with numbers, for example, female was indicated with 0 and male with 1. The test cohort was then handled according to the criteria of the training cohort. DeepMPM was trained with Python software (Python Software Foundation; https://www.python.org).
A model that behaved well in the training set but poorly in the test set was said to be overfitted, while if the opposite was true, the model was considered to be underfitted. To avoid overfitting, we have employed 10% dropout layers and an early stopping function, which could automatically cease training if there was no improvement after several epochs. To avoid underfitting, batch norm and batch training were adopted. AUC was applied to evaluate model performance: an outstanding model has an AUC near to the 1 while a poor model has an AUC near 0.5. The model was evaluated by both the training cohort and test cohort. The bootstrap method was used to calculate the 95% confidence interval (CI) of the AUC.
Statistical analysis
All clinical features were included in the survival analysis conducted with CPH regression and Kaplan-Meier curve on R software (https://www.r-project.org). A 2-sided P value <0.05 was considered statistically significant.
Results
Demographic characteristics
A total of 3,130 patients were included in the study, with 2,208 patients in the training cohort and 922 patients in the test cohort. The median age in the training cohort was 73 years while that in the test cohort was 74 years. The training cohort had 472 (21.38%) females and 1,736 (78.62%) males, while the test cohort had 214 (23.21%) females and 708 (76.79%) males. In the 2 cohorts, most patients with MPM had no history of malignant tumors. In the training cohort, 337 (15.26%) patients were diagnosed with biphasic mesothelioma, with 1,452 (65.76%) epithelioid mesotheliomas and 419 (18.98%) sarcomatoid mesotheliomas. Meanwhile, in the test cohort, 147 (15.94%) patients were diagnosed with biphasic mesothelioma, with 624 (67.68%) epithelioid mesotheliomas and 151 (16.38%) sarcomatoid mesotheliomas. Most patients in both cohorts were staged as T1, N0, or M0. In the training cohort, 812 (36.78%) patients underwent surgery, 327 (14.81%) received radiotherapy, and 1,257 (56.93%) received chemotherapy. Meanwhile, in the test cohort, 296 (32.10%) patients underwent surgery, 127 (13.77%) received radiotherapy, and 567 (61.50%) received chemotherapy. The median survival time was 10 months in the training cohort and 8 months in test cohort (Table 1).
Table 1
Clinical features | Training cohort (n=2,208) | Test cohort (n=922) |
---|---|---|
Age (years) | 73 [66, 79] | 74 [68, 80] |
Sex | ||
Male | 1,736 (78.62) | 708 (76.79) |
Female | 472 (21.38) | 214 (23.21) |
History of malignancy | ||
No | 1,662 (75.27) | 664 (72.02) |
Yes | 546 (24.73) | 258 (27.98) |
Pathology | ||
Epithelioid mesothelioma | 1,452 (65.76) | 624 (67.68) |
Sarcomatoid mesothelioma | 419 (18.98) | 151 (16.38) |
Biphasic mesothelioma | 337 (15.26) | 147 (15.94) |
T | ||
T1 | 696 (31.52) | 322 (34.92) |
T2 | 496 (22.46) | 182 (19.74) |
T3 | 409 (18.52) | 147 (15.94) |
T4 | 607 (27.49) | 271 (29.39) |
N | ||
N0 | 1,446 (65.49) | 614 (66.59) |
N1 | 118 (5.34) | 146 (15.84) |
N2 | 581 (26.31) | 143 (15.51) |
N3 | 63 (2.85) | 19 (2.06) |
M | ||
M0 | 1,835 (83.11) | 663 (71.91) |
M1 | 373 (16.89) | 259 (28.09) |
Surgery | ||
No | 1,396 (63.22) | 626 (67.90) |
Yes | 812 (36.78) | 296 (32.10) |
Radiotherapy | ||
No | 1,881 (85.19) | 795 (86.23) |
Yes | 327 (14.81) | 127 (13.77) |
Chemotherapy | ||
No | 951 (43.07) | 355 (38.50) |
Yes | 1,257 (56.93) | 567 (61.50) |
Survival time (months) | 10 [4, 22] | 8 [3, 16] |
Data are presented as median [interquartile range] or n (%).
Model performance and application
DeepMPM had an AUC of 0.7037 (95% CI: 0.7030–0.7045) in the training cohort and an AUC of 0.7076 (95% CI: 0.7067–0.7086) in the test cohort (Table 2). DeepMPM showed a satisfactory performance in predicting the prognosis of patients with MPM. We therefore formatted it into a Windows 64-bit executable file for clinicians to use (available on Google Drive: https://drive.google.com/file/d/1F3an8yOPu38ptrid0ztwhhNZd48zpT_Q/view?usp=share_link; once the installation is complete, users can launch DeepMPM by clicking “DeepMPM.exe”). In this version, after the patient’s clinical information is input and the “Predict” button is clicked, the model automatically performs analysis and calculation, and the user’s default browser is opened to show the patient’s survival curve (Figure 2). The curves are interactive and can display the survival probability of a given patient in specific months.
Table 2
Performance | Training cohort | Test cohort |
---|---|---|
AUC | 0.7037 | 0.7076 |
Low 95% CI | 0.7030 | 0.7067 |
High 95% CI | 0.7045 | 0.7086 |
AUC, area under the receiver operating characteristics curve; CI, confidence interval.
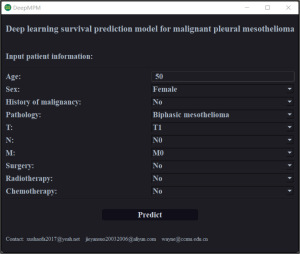
Survival analysis
All clinical features used were incorporated to conduct survival analysis. Age [hazard ratio (HR) =1.02; 95% CI: 1.01–1.02; P<0.001], male sex (HR =1.17; 95% CI: 1.06–1.29; P=0.002), sarcomatoid mesothelioma (HR =1.46; 95% CI: 1.28–1.67; P<0.001), T4 (HR =1.28; 95% CI: 1.16–1.43; P<0.001), N2 (HR =1.36; 95% CI: 1.23–1.49; P<0.001), and M1 (HR =1.48; 95% CI: 1.33–1.64; P<0.001) were identified as risk factors. Epithelioid mesothelioma (HR =0.59; 95% CI: 0.53–0.66; P<0.001), surgery (HR =0.75; 95% CI: 0.69–0.82; P<0.001), radiotherapy (HR =0.71; 95% CI: 0.63–0.80; P<0.001), and chemotherapy (HR =0.61; 95% CI: 0.56–0.66; P<0.001) were identified as protective factors (Figure 3A). In terms of treatment modalities, patients administered surgery plus radiotherapy had the best prognosis, followed by those treated with the combination of surgery, radiotherapy, and chemotherapy (Figure 3B).
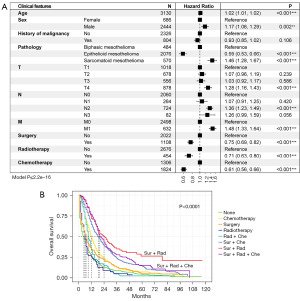
Discussion
MPM is a rare invasive malignant tumor with poor prognosis and a median OS of only about 12 months. Surgery is the first-choice treatment in early-stage MPM and mainly includes pleurectomy/decortication (P/D) or extrapleural pneumonectomy (EPP) (14,15). A meta-analysis showed that patients undergoing P/D had a significantly lower 5-year OS than did those undergoing EPP (HR =0.76; 95% CI: 0.62–0.94; P<0.001), as well as a lower 30-day mortality and postoperative complications rate (16). For unresectable MPM, antifolate drugs pemetrexed plus cisplatin have been shown to improve the survival of patients (17). Pemetrexed combined with platinum chemotherapy is also the first-line treatment recommended by the American Society of Clinical Oncology (ASCO) (18). In a multicenter phase III randomized trial (IFCT-GFPC-0701 MAPS) (19), the median OS of patients with MPM in the bevacizumab combined chemotherapy group was prolonged by 2.7 months (18.8 and 16.1 months, respectively; HR =0.77; P=0.0167) compared with the chemotherapy alone group, and the median progression-free survival (PFS) was 9.2 and 7.3 months in these two groups, respectively (HR =0.61; P<0.0001). In addition, immune checkpoint inhibitors (ICIs) have been shown to have a significant tumor growth inhibition effect in a variety of malignant tumors. Many studies on cytotoxic T-lymphocyte-associated protein 4 (CTLA-4) and programmed cell death protein 1 (PD-1) have been conducted. An open, multicenter, randomized phase III clinical trial (CheckMate-743) (20) showed that compared with standard chemotherapy (pemetrexed plus cisplatin or carboplatin), nivolumab plus ipilimumab can significantly reduce the risk of death in unresectable patients with MPM by 26%. The median OS of the patients in a nivolumab plus ipilimumab group was 4 months longer than that in a chemotherapy group (18.1 and 14.1 months, respectively; HR =0.74, 96.6% CI: 0.60–0.91; P=0.0020), and thus nivolumab plus ipilimumab is recommended as the first-line treatment by the National Comprehensive Cancer Network (NCCN) guidelines. Although MPM was traditionally considered to be resistant to radiotherapy, a study showed that radiotherapy can produce positive therapeutic effects (21). Radiotherapy has been used as a palliative treatment for chest pain, bronchial or esophageal obstruction, and other MPM-related conditions (such as brain or bone metastases). In a prospective, single-arm trial, the median OS of patients treated with high-dose hemithoracic radiotherapy after EPP was 23.9–39.4 months, which was independent of chemotherapy reaction, indicating that intensity-modulated radiotherapy (IMRT) could benefit patients after EPP (21). EPP combined with neoadjuvant or adjuvant chemotherapy and adjuvant radiotherapy was reported to be an extensive and active treatment method, with a median OS of 12.8–46.9 months, a disease-free survival (DFS) time of 10–16.3 months, a perioperative mortality rate of 0–12.5%, and a total perioperative morbidity rate of 50–82.6% (22). Genomics studies have shown there to be no clear driver gene mutation in tumor tissues of patients with MPM, with the inactivation of tumor suppressor genes being dominant (23). A previous study reported the failure of MPM targeted therapy, as it was far more difficult to correct the inactivation of tumor suppressor genes than to target the tumor driver genes (23). Fortunately, other research has revealed that individuals with the germline mutations of BRCA1-associated protein 1 (BAP1) or other genes live longer and respond well to targeted treatment (24-27).
Generally speaking, the benefits conferred by the current treatment methods are not significant, especially for patients with unresectable MPM, and inevitably lead to various drug-related adverse events. Therefore, accurate prediction of the survival of patients with MPM could provide a reference for clinical treatment decision-making.
A nomogram, a graphic prediction tool based on a CPH model, has been widely used to predict the survival outcome of patients with cancer (28-31). By integrating independent prognostic factors with TNM staging, a nomogram can stratify the prognosis of patients with cancer (32). However, simple linear assumptions make it difficult to fully explain the nonlinear relationship between clinical features and survival outcomes (11). Therefore, a richer survival model is needed to better fit survival data with nonlinear risk-log functions. In recent years, with the rapid development of artificial intelligence, deep learning has been continuously improved and successfully applied to clinical (33-37), pathology (38-40), imaging (41-44), and genetic data (45,46). Deep learning combined with existing imaging technology has been used in the diagnosis of MPM and the measurement of pleural effusion or tumor volume, achieving satisfactory results (47-49). This method can fully reveal the potential nonlinear relationships in data and can thus also be applied to survival analysis in patients with cancer. DeepSurv is a configurable feed-forward deep neural network with CPH developed by Katzman et al., and its advantage is that it does not consider the prior selection or domain expertise (11). In this study, we aimed to build a survival model for patients with MPM based on DeepSurv theory and information from the SEER database. To our knowledge, this was the first time that a deep learning algorithm has been used to predict the survival of patients with MPM.
In this study, we found that the median age of patients with MPM was old (73 years in the training cohort and 74 years in the test cohort), and the proportion of male was 4 times that of females, which may be related to the longer incubation period of MPM and the predominance of men in asbestos-related work (50,51). Additionally, the study found that epithelioid MPM was the most common type, and a forest plot demonstrated that the HRs of epithelioid MPM and sarcomatoid MPM were 0.59 (95% CI: 0.53–0.66) and 1.46 (95% CI: 1.28–1.67), respectively. A previous study also reported that the prognosis of epithelioid patients with MPM was the best among the 3 subtypes (52). Positive lymph node status, except that in N2, was also significantly associated with prognosis, and we found that the proportion of patients with MPM lymph node metastasis was nearly 35%, which was roughly the same as that previously reported (53). However, it remains unclear whether the location and number of lymph nodes are also principal prognostic factors, and further research is needed in this area. Regarding the treatment of MPM, a forest plot showed that surgery, radiotherapy, and chemotherapy are all protective factors for patients with MPM. Kaplan-Meier survival curve showed that the median OS of patients with MPM treated with surgery combined with radiotherapy was the longest (reaching 24 median months), followed by those treated with triple therapy (surgery combined with radiotherapy and chemotherapy; median OS of 20 months) and those treated with surgery combined with chemotherapy (19 months). However, the median OS of a single treatment method was less than 1 year. These findings thus suggest that multimode combination therapy may provide greater survival benefits for patients with MPM than may monotherapy. Although our study found that surgery combined with radiotherapy may be a better choice (P<0.0001), but further validation in prospective clinical trials is needed to confirm this interpretation. Fortunately, immunotherapy is bringing hope to patients with MPM. As mentioned above, in the CheckMate-743 study (20), the OS of immunotherapy was higher compared with that of chemotherapy (18.1 vs. 14.1 months; HR =0.74; 96.6% CI: 0.60–0.91; P=0.002), making immunotherapy the potential first-line treatment for unresectable patients with MPM. BAP1, the most prevalent mutated gene in MPM, has recently emerged as a promising therapeutic target. According to studies by Okonska et al. (54) and Guazzelli et al. (55), mesothelioma cells with functioning BAP1 are more responsive to gemcitabine treatment. In an effort to provide patients better care, several phase II clinical trials are now in progress (ClinicalTrials.gov identifier NCT02860286 and NCT03531840).
In summary, we selected sex, age, malignant tumor history, TNM stage, chemotherapy, surgery, and radiotherapy as clinical characteristics to model and predict the survival of patients with MPM. The model’s AUC was 0.7037 (95% CI: 0.7030–0.7045) in the training cohort and 0.7076 (95% CI: 0.7067–0.7086) in the test cohort, indicating that DeepMPM has a satisfactory performance in predicting the prognosis of patients with MPM. Finally, we packaged this model into a desktop tool for convenient use, hoping that it can serve as a reference for clinicians and researchers. Families of patients and medical professionals may approximately estimate the survival probability using the model, allowing them to assess the prognosis and select a specific course of treatment. Patients with low survival probabilities may require more care and supportive treatment, while those with relatively high survival probabilities may be recommended a combination of treatments (refer to our forest map), including surgery, chemotherapy, and radiotherapy.
Some limitations to our study should be mentioned. First, some characteristic variables of patients with MPM in the SEER database that might have important impact on the prognosis of patients with MPM, such as surgical methods, chemotherapy schemes, lung function, and biomarkers, were missing. Second, large-scale prospective multicenter data are needed for further verification of our findings.
Conclusions
A prognosis prediction tool based on a deep learning neural network performed well in predicting the survival of patients with MPM. Moreover, multimode combination therapy might confer greater survival benefits to these patients than single-mode therapy.
Acknowledgments
We are grateful for the help of the support team of the Surveillance, Epidemiology, and End Results (SEER) database at the National Cancer Institute.
Funding: This work was supported by
Footnote
Reporting Checklist: The authors have completed the TRIPOD reporting checklist. Available at https://tcr.amegroups.com/article/view/10.21037/tcr-23-422/rc
Peer Review File: Available at https://tcr.amegroups.com/article/view/10.21037/tcr-23-422/prf
Conflicts of Interest: All authors have completed the ICMJE uniform disclosure form (available at https://tcr.amegroups.com/article/view/10.21037/tcr-23-422/coif). The authors have no conflicts of interest to declare.
Ethical Statement: The authors are accountable for all aspects of the work in ensuring that questions related to the accuracy or integrity of any part of the work are appropriately investigated and resolved. This study was conducted in accordance with the Declaration of Helsinki (as revised in 2013). Given that all data were retrieved from the publicly available SEER database and patients’ private information has been concealed and is not traceable, this retrospective cohort was exempted from ethical considerations and written consent.
Open Access Statement: This is an Open Access article distributed in accordance with the Creative Commons Attribution-NonCommercial-NoDerivs 4.0 International License (CC BY-NC-ND 4.0), which permits the non-commercial replication and distribution of the article with the strict proviso that no changes or edits are made and the original work is properly cited (including links to both the formal publication through the relevant DOI and the license). See: https://creativecommons.org/licenses/by-nc-nd/4.0/.
References
- Remon J, Reguart N, Corral J, et al. Malignant pleural mesothelioma: new hope in the horizon with novel therapeutic strategies. Cancer Treat Rev 2015;41:27-34. [Crossref] [PubMed]
- Carbone M, Adusumilli PS, Alexander HR Jr, et al. Mesothelioma: Scientific clues for prevention, diagnosis, and therapy. CA Cancer J Clin 2019;69:402-29. [Crossref] [PubMed]
- Travis WD, Brambilla E, Nicholson AG, et al. The 2015 World Health Organization Classification of Lung Tumors: Impact of Genetic, Clinical and Radiologic Advances Since the 2004 Classification. J Thorac Oncol 2015;10:1243-60. [Crossref] [PubMed]
- Kwak KM, Paek D, Hwang SS, et al. Estimated future incidence of malignant mesothelioma in South Korea: Projection from 2014 to 2033. PLoS One 2017;12:e0183404. [Crossref] [PubMed]
- Shavelle R, Vavra-Musser K, Lee J, et al. Life Expectancy in Pleural and Peritoneal Mesothelioma. Lung Cancer Int 2017;2017:2782590. [Crossref] [PubMed]
- Abdel-Rahman O. Challenging a dogma; AJCC 8th staging system is not sufficient to predict outcomes of patients with malignant pleural mesothelioma. Lung Cancer 2017;113:128-33. [Crossref] [PubMed]
- Wang S, Ma K, Chen Z, et al. A Nomogram to Predict Prognosis in Malignant Pleural Mesothelioma. World J Surg 2018;42:2134-42. [Crossref] [PubMed]
- Zhuo M, Zheng Q, Chi Y, et al. Survival analysis via nomogram of surgical patients with malignant pleural mesothelioma in the Surveillance, Epidemiology, and End Results database. Thorac Cancer 2019;10:1193-202. [Crossref] [PubMed]
- Chen S, Yu W, Shao S, et al. Establishment of predictive nomogram and web-based survival risk calculator for malignant pleural mesothelioma: A SEER database analysis. Front Oncol 2022;12:1027149. [Crossref] [PubMed]
- Wainberg M, Merico D, Delong A, et al. Deep learning in biomedicine. Nat Biotechnol 2018;36:829-38. [Crossref] [PubMed]
- Katzman JL, Shaham U, Cloninger A, et al. DeepSurv: personalized treatment recommender system using a Cox proportional hazards deep neural network. BMC Med Res Methodol 2018;18:24. [Crossref] [PubMed]
- She Y, Jin Z, Wu J, et al. Development and Validation of a Deep Learning Model for Non-Small Cell Lung Cancer Survival. JAMA Netw Open 2020;3:e205842. [Crossref] [PubMed]
- Yu H, Huang T, Feng B, et al. Deep-learning model for predicting the survival of rectal adenocarcinoma patients based on a surveillance, epidemiology, and end results analysis. BMC Cancer 2022;22:210. [Crossref] [PubMed]
- Wolf AS, Flores RM. Updates in Staging and Management of Malignant Pleural Mesothelioma. Surg Oncol Clin N Am 2020;29:603-12. [Crossref] [PubMed]
- Gelzinis TA. The 2019 ERS/ESTS/EACTS/ESTRO Guidelines on the Management of Patients With Malignant Pleural Mesothelioma. J Cardiothorac Vasc Anesth 2021;35:378-88. [Crossref] [PubMed]
- Danuzzo F, Maiorca S, Bonitta G, et al. Systematic Review and Meta-Analysis of Pleurectomy/Decortication versus Extrapleural Pneumonectomy in the Treatment of Malignant Pleural Mesothelioma. J Clin Med 2022;11:5544. [Crossref] [PubMed]
- Vogelzang NJ, Rusthoven JJ, Symanowski J, et al. Phase III study of pemetrexed in combination with cisplatin versus cisplatin alone in patients with malignant pleural mesothelioma. J Clin Oncol 2003;21:2636-44. [Crossref] [PubMed]
- Kindler HL, Ismaila N, Armato SG 3rd, et al. Treatment of Malignant Pleural Mesothelioma: American Society of Clinical Oncology Clinical Practice Guideline. J Clin Oncol 2018;36:1343-73. [Crossref] [PubMed]
- Zalcman G, Mazieres J, Margery J, et al. Bevacizumab for newly diagnosed pleural mesothelioma in the Mesothelioma Avastin Cisplatin Pemetrexed Study (MAPS): a randomised, controlled, open-label, phase 3 trial. Lancet 2016;387:1405-14. [Crossref] [PubMed]
- Baas P, Scherpereel A, Nowak AK, et al. First-line nivolumab plus ipilimumab in unresectable malignant pleural mesothelioma (CheckMate 743): a multicentre, randomised, open-label, phase 3 trial. Lancet 2021;397:375-86. [Crossref] [PubMed]
- de Perrot M, Wu L, Wu M, et al. Radiotherapy for the treatment of malignant pleural mesothelioma. Lancet Oncol 2017;18:e532-42. [Crossref] [PubMed]
- Batirel HF. Extrapleural pneumonectomy (EPP) vs. pleurectomy decortication (P/D). Ann Transl Med 2017;5:232. [Crossref] [PubMed]
- Cakiroglu E, Senturk S. Genomics and Functional Genomics of Malignant Pleural Mesothelioma. Int J Mol Sci 2020;21:6342. [Crossref] [PubMed]
- Carbone M, Pass HI, Ak G, et al. Medical and Surgical Care of Patients With Mesothelioma and Their Relatives Carrying Germline BAP1 Mutations. J Thorac Oncol 2022;17:873-89. [Crossref] [PubMed]
- Panou V, Gadiraju M, Wolin A, et al. Frequency of Germline Mutations in Cancer Susceptibility Genes in Malignant Mesothelioma. J Clin Oncol 2018;36:2863-71. [Crossref] [PubMed]
- Hassan R, Morrow B, Thomas A, et al. Inherited predisposition to malignant mesothelioma and overall survival following platinum chemotherapy. Proc Natl Acad Sci U S A 2019;116:9008-13. [Crossref] [PubMed]
- Pastorino S, Yoshikawa Y, Pass HI, et al. A Subset of Mesotheliomas With Improved Survival Occurring in Carriers of BAP1 and Other Germline Mutations. J Clin Oncol 2018; Epub ahead of print. [Crossref] [PubMed]
- Wu YY, Liu PF. A nomogram to predict survival in young patients with stage IV breast cancer: A SEER-based population study. Asian J Surg 2023;46:1856-8. [Crossref] [PubMed]
- Wang S, Liu Y, Yao Z, et al. A Commentary on “Construction of a nomogram to predict overall survival for patients with M1 stage of colorectal cancer: A retrospective cohort study” (Int J Surg 2019;72:96-101). Int J Surg 2022;106:106914. [Crossref] [PubMed]
- Ren C, Ma Y, Jin J, et al. Development and external validation of a dynamic nomogram to predict the survival for adenosquamous carcinoma of the pancreas. Front Oncol 2022;12:927107. [Crossref] [PubMed]
- Yang B, Liu C, Wu R, et al. Development and Validation of a DeepSurv Nomogram to Predict Survival Outcomes and Guide Personalized Adjuvant Chemotherapy in Non-Small Cell Lung Cancer. Front Oncol 2022;12:895014. [Crossref] [PubMed]
- Balachandran VP, Gonen M, Smith JJ, et al. Nomograms in oncology: more than meets the eye. Lancet Oncol 2015;16:e173-80. [Crossref] [PubMed]
- Gu J, Tong T, Xu D, et al. Deep learning radiomics of ultrasonography for comprehensively predicting tumor and axillary lymph node status after neoadjuvant chemotherapy in breast cancer patients: A multicenter study. Cancer 2023;129:356-66. [Crossref] [PubMed]
- Wang CW, Lin KY, Lin YJ, et al. A Soft Label Deep Learning to Assist Breast Cancer Target Therapy and Thyroid Cancer Diagnosis. Cancers (Basel) 2022;14:5312. [Crossref] [PubMed]
- Sartoretti E, Gennari AG, Maurer A, et al. Opportunistic deep learning powered calcium scoring in oncologic patients with very high coronary artery calcium (≥ 1000) undergoing 18F-FDG PET/CT. Sci Rep 2022;12:19191. [Crossref] [PubMed]
- Chen S, Zang Y, Xu B, et al. An Unsupervised Deep Learning-Based Model Using Multiomics Data to Predict Prognosis of Patients with Stomach Adenocarcinoma. Comput Math Methods Med 2022;2022:5844846. [Crossref] [PubMed]
- Wang H, Liu Y, Xu N, et al. Development and validation of a deep learning model for survival prognosis of transcatheter arterial chemoembolization in patients with intermediate-stage hepatocellular carcinoma. Eur J Radiol 2022; Epub ahead of print. [Crossref] [PubMed]
- Wen-Zhi G, Tai T, Zhixin F, et al. Prediction of pathological staging and grading of renal clear cell carcinoma based on deep learning algorithms. J Int Med Res 2022;50:3000605221135163. [Crossref] [PubMed]
- She Y, He B, Wang F, et al. Deep learning for predicting major pathological response to neoadjuvant chemoimmunotherapy in non-small cell lung cancer: A multicentre study. EbioMedicine 2022;86:104364. [Crossref] [PubMed]
- Jarkman S, Karlberg M, Pocevičiūtė M, et al. Generalization of Deep Learning in Digital Pathology: Experience in Breast Cancer Metastasis Detection. Cancers (Basel) 2022;14:5424. [Crossref] [PubMed]
- Xuan P, Bi H, Cui H, et al. Graph based multi-scale neighboring topology deep learning for kidney and tumor segmentation. Phys Med Biol 2022;67: [Crossref] [PubMed]
- Nwosu L, Li X, Qian L, et al. Calibrated bagging deep learning for image semantic segmentation: A case study on COVID-19 chest X-ray image. PloS One 2022;17:e0276250. [Crossref] [PubMed]
- Jung W, Lee HS, Seo M, et al. MR-self Noise2Noise: self-supervised deep learning-based image quality improvement of submillimeter resolution 3D MR images. Eur Radiol 2023;33:2686-98. [Crossref] [PubMed]
- Li X, Yang L, Jiao X. Comparison of Traditional Radiomics, Deep Learning Radiomics and Fusion Methods for Axillary Lymph Node Metastasis Prediction in Breast Cancer. Acad Radiol 2023;30:1281-7. [Crossref] [PubMed]
- Montepietra D, Cecconi C, Brancolini G. Combining enhanced sampling and deep learning dimensionality reduction for the study of the heat shock protein B8 and its pathological mutant K141E. RSC Adv 2022;12:31996-2011. [Crossref] [PubMed]
- Liao M, Zhao JP, Tian J, et al. iEnhancer-DCLA: using the original sequence to identify enhancers and their strength based on a deep learning framework. BMC Bioinformatics 2022;23:480. [Crossref] [PubMed]
- Kitajima K, Matsuo H, Kono A, et al. Deep learning with deep convolutional neural network using FDG-PET/CT for malignant pleural mesothelioma diagnosis. Oncotarget 2021;12:1187-96. [Crossref] [PubMed]
- Gudmundsson E, Straus CM, Li F, et al. Deep learning-based segmentation of malignant pleural mesothelioma tumor on computed tomography scans: application to scans demonstrating pleural effusion. J Med Imaging (Bellingham) 2020;7:012705. [Crossref] [PubMed]
- Kidd AC, Anderson O, Cowell GW, et al. Fully automated volumetric measurement of malignant pleural mesothelioma by deep learning AI: validation and comparison with modified RECIST response criteria. Thorax 2022;77:1251-9. [Crossref] [PubMed]
- Beckett P, Edwards J, Fennell D, et al. Demographics, management and survival of patients with malignant pleural mesothelioma in the National Lung Cancer Audit in England and Wales. Lung Cancer 2015;88:344-8. [Crossref] [PubMed]
- Huang X, Shu C, Chen L, et al. Impact of sex, body mass index and initial pathologic diagnosis age on the incidence and prognosis of different types of cancer. Oncol Rep 2018;40:1359-69. [Crossref] [PubMed]
- Meyerhoff RR, Yang CF, Speicher PJ, et al. Impact of mesothelioma histologic subtype on outcomes in the Surveillance, Epidemiology, and End Results database. J Surg Res 2015;196:23-32. [Crossref] [PubMed]
- Ricciardi S, Carleo F, Jaus MO, et al. Malignant Pleural Mesothelioma Nodal Status: Where Are We at? J Clin Med 2021;10:5177. [Crossref] [PubMed]
- Okonska A, Bühler S, Rao V, et al. Functional Genomic Screen in Mesothelioma Reveals that Loss of Function of BRCA1-Associated Protein 1 Induces Chemoresistance to Ribonucleotide Reductase Inhibition. Mol Cancer Ther 2020;19:552-63. [Crossref] [PubMed]
- Guazzelli A, Meysami P, Bakker E, et al. BAP1 Status Determines the Sensitivity of Malignant Mesothelioma Cells to Gemcitabine Treatment. Int J Mol Sci 2019;20:429. [Crossref] [PubMed]