A WGCNA-based cancer-associated fibroblast risk signature in colorectal cancer for prognosis and immunotherapy response
Highlight box
Key findings
• Our study identified a three-gene cancer-associated fibroblast (CAF)-related signature predicting colorectal cancer (CRC)prognosis and defined risk groups based on Riskscores.
• The signature showed robustness through multidimensional validations and correlation with clinical parameters.
• Low-risk patients exhibited enhanced sensitivity to immunotherapy and chemotherapy drugs, including 5-fluorouracil and oxaliplatin.
What is known and what is new?
• Existing knowledge: Gene signatures and biomarkers play a crucial role in CRC prognosis and personalized medicine.
• This manuscript adds: A novel CAF-related three-gene signature for prognostic prediction, risk stratification, and drug sensitivity in CRC.
What is the implication, and what should change now?
• The identified signature has potential clinical utility in guiding treatment decisions and improving patient outcomes.
• Low-risk patients may benefit from tailored immunotherapy and chemotherapy approaches.
• Further validation studies are needed to assess its integration into existing prognostic models.
Introduction
Background
Colorectal cancer (CRC) is the third-most prevalent and second-most deadly malignancy, with approximately 1.9 million new cases and nearly 900,000 deaths in 2020 (1). It is estimated that the incidence of CRC will rise to 3.2 million new cases and result in 1.6 million deaths within the next two decades (2). Early-stage patients have a relatively favorable prognosis with various treatment options such as local endoscopic excision, local surgical excision, and adjacent postoperative chemotherapy (3). However, the 5-year overall survival (OS) of patients in the advanced stage is still less than 40% due to metastases and treatment failure (4). Thus, it is necessary to investigate possible biomarkers and signatures to facilitate early diagnosis and provide a more targeted, personalized treatment.
Rationale and knowledge gap
Although cancer arises as a result of mutations occurring in cancer cells, non-mutant cells in the tumor microenvironment (TME) also play a significant role in cancer growth (5). Cancer-associated fibroblasts (CAFs), which comprise the majority of the stromal cells in the TME (6), regulate the development and spread of cancer through a variety of mechanisms (7). CAFs produce extracellular matrix (ECM) components such as collagen and fibronectin, as well as matrix metalloproteinases that degrade the ECM (8,9). Numerous studies have demonstrated that CAFs produce a variety of tumor-promoting molecules such as cytokines and chemokines, which promote angiogenesis and cancer cell proliferation (10-12). Additionally, prior studies discovered that CAFs encourage matrix deposition and crosslinking, thereby increasing the stiffness of tumor tissue (13,14). Consequently, increasing mechanical stress causes blood vessels to collapse, resulting in hypoxia, stimulating tumor invasiveness, and reducing treatment efficacy (15,16). Moreover, it has been demonstrated that CAFs contribute to poor immunotherapy response in CRC mouse models (17).
Over the past few years, there has been a significant expansion in gene sequencing technology. Techniques such as microarray and RNA-seq, in conjunction with multiple bioinformatics tools, have been widely used to investigate the underlying mechanisms of CRC. As a systematic bioinformatics algorithm, weighted gene co-expression network analysis (WGCNA) is a novel method for investigating the relationship between genes and clinical phenotypes (18). Multiple attempts have been made to identify CAF markers by WGCNA in a variety of cancer types, including breast cancer (19), bladder cancer (20), and renal cell carcinoma (21). However, different cancers have distinct CAF genes (22), and few researchers have conducted systematic analyses of the prognostic significance of CAF-related genes in CRC.
Objective
Therefore, this study aimed to develop a reliable, CAF-related mRNA signature panel for predicting OS and therapy response in CRC patients. By employing WGCNA, we explored the gene modules that are most relevant for CAFs. Then, we used the least absolute shrinkage and selection operator (LASSO) method to identify a CAF-related, three-gene signature (BOC, COLEC12, DACT3). On the basis of their Riskscores, we divided patients into two risk groups and validated the signature’s prognostic value and ability to predict drug sensitivity in an independent dataset. Thus, these three genes have the potential to serve as biomarkers of cancer progression and may help develop a novel anti-CAF therapeutic approach in CRC. We present this article in accordance with the TRIPOD reporting checklist (available at https://tcr.amegroups.com/article/view/10.21037/tcr-23-261/rc).
Methods
Public data acquisition
Figure 1 presents an overview of the workflow of this study. We obtained level 3 RNA-sequencing data from The Cancer Genome Atlas Colon Adenocarcinoma (TCGA-COAD) and The Cancer Genome Atlas Rectum Adenocarcinoma (TCGA-READ) via the R package TCGAbiolinks (23). The voom function in the R package limma (version 3.52.2) was used to normalize the raw counts for subsequent WGCNA analysis (24). The voom algorithm computes the mean variance of log counts and assigns a precision weight to each observation (25). Thereby, all bioinformatics workflows originally designed for microarray analysis can be applied to these data.
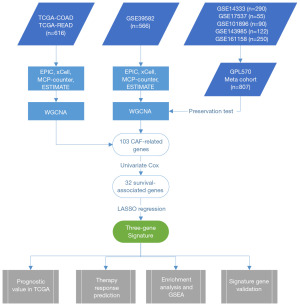
We incorporated several GPL570-based microarray datasets from the Gene Expression Omnibus (GEO) database. Using the R package GEOquery (version 2.64.2), we downloaded the raw .cel files from several CRC patient datasets (26). Then, we utilized the justRMA function within the R package affy (version 1.74.0) to read and normalize the raw data using the Robust Multichip Average (RMA) algorithm (27,28). The missing values were then filled in using the K-Nearest Neighbor (KNN) algorithm from the R package impute (version 1.70.0) (29). Lastly, we merged the datasets into a single meta-cohort using the ComBat algorithm from the R package sva (version 3.44.0) to eliminate batch effects across datasets (30). The probes were converted into gene symbols in accordance with the annotation files. The study was conducted in accordance with the Declaration of Helsinki (as revised in 2013).
CAF abundance estimation
The Microenvironment Cell Populations-counter (MCP-counter) algorithm (31), the Estimate the Proportion of Immune and Cancer cells (EPIC) algorithm (32), and the gene signature enrichment-based xCell algorithm (33) can all be used to estimate CAF abundances. Therefore, we downloaded the R package Immunedeconv (version 2.1.0) from GitHub and computed the CAF abundances for each sample using the aforementioned techniques (34). In addition, we applied the Estimation of Stromal and Immune cells in Malignant Tumor tissues using Expression data (ESTIMATE) program using the R package estimate (version 1.0.13) to construct the stromal score, which reflects the stromal infiltration levels of each sample (35).
Weighted gene co-expression network construction
Each dataset’s weighted co-expression network was constructed using the R package WGCNA (version 1.71) (18). Following data collection, we eliminated outlier samples based on their standardized connectivity (Z.K Score), a technique advised by WGCNA authors. After data preprocessing, we calculated Pearson’s correlation coefficient between any two genes and created a similarity matrix. In our study, we adopted the unsigned co-expression measure for the network, which indicates that positive and negative correlations cannot be distinguished. To create an adjacency matrix, we must next establish a soft thresholding power to which the expression similarity is elevated. To accomplish this, we computed the scale independence and average connection degree of modules with varying power using the gradient approach. Then, we selected the optimal power β to guarantee that the network was scale-free without sacrificing connection. Following this, we constructed a topological overlap matrix (TOM) based on the adjacency matrix and computed the dissimilarity matrix (1-TOM) for subsequent studies.
Identification of modules associated with CAFs
In the follow-up phase of the study, we employed a dynamic, hybrid, tree-cutting technique to construct a hierarchical clustering tree from a dissimilarity matrix to partition genes into many modules. The minimum size of the module was set at 50, and comparable modules were discovered and merged using a height cutoff of 0.25. As noted, the EPIC and Stromal scores for each sample were determined as clinical, principal characteristics. Principal component analysis (PCA) was used to derive the module eigengenes (MEs), the mathematically best summary of the module expression, for each module. Utilizing Pearson’s correlation test, we analyzed the relationship between module MEs and characteristics. The heatmap depicting the module-to-trait connection displayed the associated Pearson’s correlation coefficient and P values. We chose hub genes based on module membership and gene importance in modules substantially linked to CAF abundance.
Module preservation validation
We plotted a histogram of network connectivity and a corresponding log-log plot of the specified power using the scaleFreePlot function. The near-straight-line relationship showed the approximate scale-free topology. To assess the reproducibility of the modules, we conducted the preservation test using an independent dataset, the GPL570 meta-cohort. Module preservation statistics help determine if a certain module defined in the reference network is also present in another dataset (36). The creator of WGCNA offered two composite statistics for preservation. The first composite statistic, Zsummary, summarizes the individual Z statistic values for each module that result from the permutation test. Zsummary <2 implies no preservation, but Zsummary >10 suggests substantial preservation evidence. The alternative technique is median rank statistics, which is based on the observed preservation statistics’ ranks. A module with a lower median rank is often better preserved than one with a higher median rank.
Univariate and LASSO cox analysis
The common genes between CAF-related hub genes from two datasets were extracted for further analysis. We performed a univariate Cox regression analysis in GSE39582, using the R package survival (version 3.3.1) (37), to identify survival-associated genes. These predictive genes were then included in the LASSO-cox analysis. The LASSO regression is a regularization approach that picks a smaller number of variables, hence, promoting a simple and sparse model. LASSO regression applies an L1-norm penalty, which equals the absolute value of the magnitude of the coefficient (38). The R package glmnet (version 4.1.4) was applied to build an optimized and streamlined LASSO-cox model for predicting patient risk and prognosis (39). Ten-fold cross-validation was utilized to identify the ideal penalty parameter value. The Riskscore formula was as follows:
For additional validation, the CRC patients in GSE39582 and TCGA were separated into high-risk and low-risk groups based on their respective Riskscores.
Chemotherapy and immunotherapy responsepredictions
We calculated the chemotherapeutic response in various samples using the R package oncoPredict (version 0.2), which is based on the ridge regression model. oncoPredict is a significant improvement to pRRophetic that allows users to choose their own training datasets (40). In this work, we used the Genomics of Drug Sensitivity in Cancer 2.0 (GDSC2; https://www.cancerrxgene.org/) database (41) as reference data and extrapolated half-maximal inhibitory concentration (IC50) values of 198 distinct medications for each sample.
In addition, we used the Tumor Immune Dysfunction and Exclusion (TIDE) method to estimate the immune checkpoint blockade response rate (42). The prediction of the immune response of each sample in TCGA and GSE39582 was obtained from the website, http://tide.dfci.harvard.edu/, after data processing to fulfill the TIDE algorithm’s requirements. Furthermore, the IMvigor210 anti-PD-L1 cohort (43) was also retrieved and evaluated.
Enrichment analysis
We performed the Gene Ontology (GO) and Kyoto Encyclopedia of Genes and Genomes (KEGG) analyses of common hub genes using the R package clusterProfiler (version 4.4.4) (44). In addition, we performed Gene Set Enrichment Analysis (GSEA) to investigate the enriched pathways in both risk groups (45). The number of permutations was set to 1,000, and a false discovery rate (FDR) of less than 0.25 was deemed statistically significant.
Single-sample GSEA (ssGSEA) is an extension of GSEA that permits the computation of the enrichment score for each sample. We retrieved the h.all.v7.4.symbols gene set from the MSigDB database (https://www.gsea-msigdb.org) (46) for ssGSEA via the R package GSVA (version 1.44.4) (47). P value <0.05 and FDR <0.25 indicated statistical significance.
Statistical analysis
We used the R 4.2.1 programming environment throughout our study, depending on its fundamental features and specialized packages and modules. Microsoft’s open R version 4.0.2 (https://mran.microsof.com/open) was used to improve the performance of multithreaded processes via the Intel® oneAPI Math Kernel Library (oneMKL). Pairwise comparisons were performed using the Wilcoxon rank-sum test. Spearman’s test was used to identify correlations between the screened genes. The log-rank test was selected for OS comparisons by utilizing the R survival package (version 3.3.1). Kaplan-Meier plots were generated using the R package survminer (version 0.4.9) (48). Specific statistical methods for assessing transcriptome data are discussed in the preceding section. P value <0.05 was considered statistically significant.
Results
Data processing and assessment of CAF abundance
We searched the GEO database and randomly selected six datasets based on the GPL570 platform with relatively large sample sizes. We picked GSE39582 (n=566) for prognostic model construction because of its more comprehensive clinical information and higher data quality. For module preservation purposes, the remaining five datasets [GSE14333 (n=290), GSE17537 (n=55), GSE101896 (n=90), GSE143985 (n=122), and GSE161158 (n=250)] were merged into one meta-cohort. For TCGA data, we downloaded 616 tumor samples from TCGA-COAD and TCGA-READ and retrieved each sample’s raw counts of 19,934 mRNAs from 60,488 transcripts. After data normalization and preparation, we applied four methods to compute the CAF abundance and stromal score for each sample. As primary CAF-related metrics, we selected CAF abundance from the EPIC and stromal scores for the subsequent investigation. We separated the data into high-risk and low-risk groups on the basis of the optimal cutoff for each CAF parameter. The Kaplan-Meier plots of survival analysis demonstrated that groups with low CAF abundance had a more favorable prognosis than those with high CAF abundance (Figure 2). These findings suggest a correlation between CAFs and the OS of CRC patients, which warrants further research.
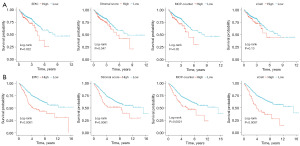
Identifying key genes related to CAFs by WGCNA
We performed WGCNA on the top 75% median absolute deviation expression profiles from the GSE39582 dataset and TCGA cohort. In GSE39582, the soft threshold was set to 5 based on the scale-independent plot (Figure 3A,3B). Subsequently, module identification was carried out and shown as a gene dendrogram (Figure 3C), where each branch of the cluster tree represents one gene and the color underneath it indicates the module to which the gene belongs. The module-to-trait relationship heatmap (Figure 3D) revealed that the magenta and turquoise modules were most relevant to CAF abundance. In these two modules, genes with a module membership >0.8 and gene significance >0.5 were identified as essential hub genes (Figure 3E).
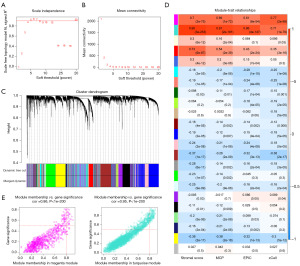
We picked 6 as the soft threshold for TCGA data (Figure S1A,S1B), and the gene dendrogram was drawn during the module identification process (Figure S1C). The black and magenta modules were identified as modules of interest (Figure S1D). Apart from GSE39582, we selected the hub genes from modules with a gene significance threshold of 0.3 (Figure S1E). Finally, we obtained 298 hub genes from the TCGA cohort and 152 hub genes from the GSE39582 dataset.
Module preservation validation
Five distinct datasets were utilized to verify the repeatability and preservation of modules discovered by WGCNA in GSE39582. After robust multichip average (RMA) normalization, we used the Combating Batch Effects When Combining Batches of Gene Expression Microarray Data (ComBat) method to minimize the batch effect between arrays. We later used Uniform Manifold Approximation and Projection (UMAP) analysis (Figure 4A), PCA (Figure 4B), and boxplot (Figure 4C) to visualize the results of this procedure. At the chosen, best soft threshold of 5, the histogram of connectivity and the log-log plot of the same histogram indicate that the scale-free topology is roughly fulfilled (Figure 4D). Based on their high Zsummary scores and low median rankings, the preservation analysis revealed that the magenta and turquoise modules were well conserved (Figure 4E).
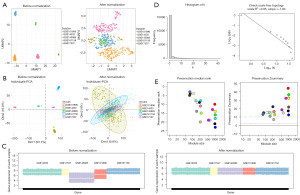
Functional analyses of CAF-related genes
As demonstrated in Figure 5A, the overlap of GSE39582 and TCGA included 103 common hub genes. In the GO analysis, ECM organization were enriched in biological process (BP), collagen-containing ECM were enriched in cellular component (CC), and ECM structure constituent were enriched in molecular function (MF) (Figure 5B). Meanwhile, KEGG analysis revealed that these hub genes were also strongly related to focal adhesion, protein digestion and absorption, and ECM-receptor interaction (Figure 5C).
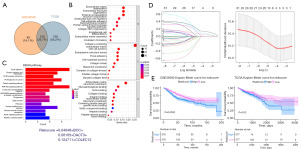
Identification and verification of the prognostic signature
For the development of prognostic models, we selected GSE39582 as the training set and TCGA as the test set. A preliminary univariate cox regression analysis found 32 OS-related genes out of 103 key hub genes in the training set (Figure S2). Next, LASSO regression was utilized to select the most suitable gene combination, resulting in the development of a three-gene prognostic signature (Figure 5D). The Riskscore was determined using the following formula:
All the patients in the training and test datasets were separated into low-risk and high-risk subgroups using the median of the Riskscores as the dividing line. In both datasets, Kaplan-Meier curve analysis revealed that high-risk patients had a substantially shorter OS than low-risk patients (GEO: P=0.0033; TCGA: P=0.03) (Figure 5E).
Correlation between CAF-based signature genes with known CAF markers
We computed Spearman correlation coefficients between the Riskscores and CAF abundance estimated by various techniques (Figure 6A). A positive and strong correlation existed between these parameters in both GSE39582 and TCGA datasets. In addition, we extracted the expression data of CAF marker genes summarized in prior publications (49). In both datasets, the expression of the signature genes was substantially linked to those of CAF indicators (Figure 6B). CAF markers tended to have a higher expression in the high-risk group than in the low-risk group, as represented by the heatmaps (Figure 6C,6D).
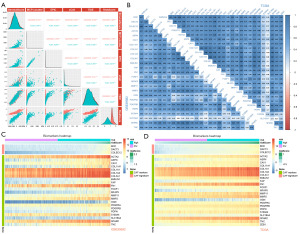
Correlation between Riskscores and clinicopathological characteristics
Further analysis of the prognostic significance of these signature genes in the TCGA cohort revealed that they were also inversely associated with the OS of CRC patients (Figure 7A). Regarding clinical parameters, we found that individuals with a lower Riskscore were more likely to have an advanced pathological T stage (P=0.02) and pathological N stage (P=9.77e-3) than those with a higher Riskscore. Although not statistically significant, we also noticed a similar trend in the American Joint Committee on Cancer staging of CRC patients (P=0.09) (Figure 7B). However, no significant difference in tumor metastasis was observed (P=0.21). While lacking statistical significance, a comparable trend was observed in GSE39582 dataset (Figure S3A).
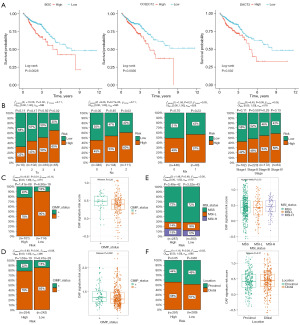
Subsequently, we assessed the CpG island methylator phenotype (CIMP) status of each patient, and found a statistically significant difference between the high- and low-CAF risk groups in CIMP in both cohorts (Figure 7C,7D). Additionally, we further analyzed the microsatellite instability (MSI) status in the TCGA cohort (Figure 7E) and chromosomal instability (CIN) status in the GSE39582 cohort (Figure S3B), respectively, due to a lack of pertinent data. However, no significant differences were found in these two comparisons. Moreover, upon investigating the tumor’s location, we discovered that the variations between proximal and distal CRC did not impact the Riskscore (Figure 7F, Figure S3C).
Chemotherapy sensitivity between different risk groups
We retrieved the IC50 values of first-line chemotherapeutic drugs for CRC, including 5-fluorouracil, oxaliplatin, and irinotecan, as well as the IC50 values of the target medications, gefitinib and afatinib. In both cohorts, high-risk patients were more resistant to oxaliplatin (GSE39582: P=8e-11; TCGA: p2.2e-16), gefitinib (GSE39582: P=2.3e-16; TCGA: P=7.3e-7), and afatinib (GSE39582: P=3.9e-16; TCGA: P=3.7e-7) than low-risk patients. In the TCGA cohort, greater sensitivity to 5-fluorouracil was observed in the low-risk group (P=5.6e-8), while there was no difference in the GSE39582 cohort (P=0.13). In the GSE39582 cohort, high-risk patients tended to have significantly decreased irinotecan resistance (P=0.0095), while this decreased irinotecan resistance was not significant in the TCGA cohort (P=0.059) (Figure 8A,8B).
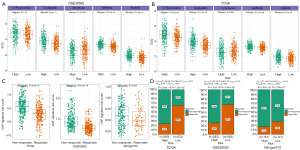
The role of the CAF-based signature in immunotherapy
We utilized TIDE to predict the immunotherapy outcomes in TCGA and GSE39582 datasets, and collected the response information of IMvigor210 trial cohort. With this information, we validated the Riskscore’s capacity to predict immunotherapy response. Figure 8C shows that the immunotherapy-resistant patients had a higher Riskscore (TCGA: P<2.2e-16; GSE39582: P<2.2e-16; IMvigor210: P=0.034). From another perspective, the patients in the low-risk group had a reasonably high sensitivity to immunotherapy as indicated by the chi-squared test (TCGA: P=5.38e-22, GSE39582; P=5.87e-23; IMvigor210: P=0.02) (Figure 8D).
Somatic variation and GSEA of the CAF-based gene signature
We utilized the R package maftools (version 2.12.0) to read and analyze the TCGA somatic mutation data. The waterfall plots incorporating the top 20, highly variable, mutant genes were separately generated for high- and low-risk groups. Figure 9A illustrates a significant overlap in the top 20 mutational gene profiles between high- and low-CAF-risk groups. However, specific genes like RYR3, DNAH11, NEB, and ABCA13 were unique to the high-CAF-risk group, while FBXW7, ADGRV1, ATM, and PCLO were exclusive to the low-CAF-risk group. In addition, the chi-square test revealed that the mutation rate of KRAS, a key oncogene and therapeutic target in CRC, was higher in the low-risk group (P<0.05).
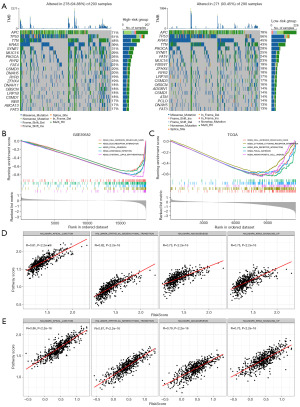
We performed GSEA on two datasets (TCGA and GSE39582) to further validate the activation of the associated signaling pathways implicated in the diverse CAF-related risk categories. Cell adhesion molecules, ECM-receptor interaction, and focal adhesion pathways were considerably enriched in both datasets (Figure 9B,9C). The ssGSEA also revealed a strong correlation between the CAF Riskscore and apical junctions, epithelial to mesenchymal transition, angiogenesis, and the KRAS signaling pathway (Figure 9D,9E).
Validation of cell lines and immunohistochemistry
We evaluated RNA-seq data from 35 fibroblast and 57 CRC cell lines. The heatmap (Figure 10A) and boxplot (Figure 10B) indicate that the signature genes (BOC, DACT3, and COLEC12) are predominantly expressed in the fibroblast cell lines. Immunohistochemistry of Human Protein Atlas database signature genes also validated protein expression in stromal tissue (Figure 10C).
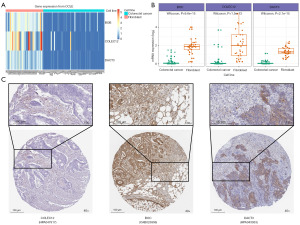
Discussion
Key findings
In recent years, researchers have reached a consensus that the TME is highly linked to both cancer progression (50) and therapeutic resistance (51). The tumor stroma is no longer viewed solely as physical support for mutated epithelial cells but as a crucial modulator. As a major component of the TME, CAFs collaborate with tumor cells and other TME components in an established solid tumor (52) and exert the greatest influence on nearly all TME activities in real time (53-55). Based on the CRC Consensus Molecular Subtype classification (CMS), the CMS4 (mesenchymal) group is prominent in transforming growth factor-beta (TGF-β) signaling activation, stromal invasion, ECM remodeling, and angiogenesis (56). This study discovered that genes upregulated in the mesenchymal subtype were prominently expressed by CAFs and other stromal cells (57). Lawrenson et al. demonstrated that the presence of senescent CAFs during the aging process significantly increased in-vitro and in-vivo proliferation and tumorigenicity (58). A recent study conducted by Nee and colleagues has revealed that preneoplastic stromal cells play a significant role in promoting breast tumorigenesis that is mediated by BRCA1 (59). They identified that pre-CAFs are responsible for the production of pro-tumorigenic factors, including MMP3, which promotes BRCA1-driven tumorigenesis.
In this study, we identified a novel gene signature associated with CAF infiltration that may serve as a potential prognostic marker and predictor of therapeutic response. Unlike the conventional differential gene expression (DEG) strategy for screening hub CAF markers (60), WGCNA assesses the connection between co-expressed genes and sample phenotypes. We utilized numerous deconvolution algorithms to assess the CAF abundance of each sample as its characteristic. Consistent with previous studies, we observed that CAF infiltration measured by three bioinformatics approaches (EPIC, xCell, and MCP-counter) and estimated stromal scores were inversely linked to the OS in CRC patients (61,62). Although some research employing the single-cell RNA-sequencing technique has been carried out on gene expression differences with respect to CAF infiltration (63), the heterogeneity of CAFs prevents a full comprehension of alterations in the transcriptomics (53,63,64). Through WGCNA analysis, we identified several co-expression modules that exhibited strong correlations with CAFs. These modules were enriched for genes involved in key biological processes associated with CAFs, which was confirmed by following GO and KEGG enrichment analyses. Thus, WGCNA is an innovative method to explore markers associated with CAFs and has been successfully applied to other cancer types (65,66).
Explanations of findings
Among the co-expression modules, we selected a specific module that exhibited the highest correlation with CAFs for further analysis. LASSO permits subset contraction for multicollinear data and biased estimates to minimize variance. By applying LASSO regression, we constructed a gene risk signature that effectively captured the most informative genes associated with CAFs, while penalizing irrelevant or redundant genes. Finally, we successfully demonstrated a three-gene prognostic (BOC, COLEC12, DACT3) signature. By examining the expression levels of the signature genes in tumor samples, it may be possible to stratify patients based on their CAF-related risk profiles. After that, we performed several analyses to explore the difference between CAFs risk groups. Besides the advanced TNM stage and dismal prognosis, we observed a statistically significant difference in CIMP between high- and low-CAF risk groups. However, there was no similar tendency with respect to MSI and CIN. A study conducted by researchers at Umeå University has reported that CIMP-negative tumors exhibit heightened levels of fibronectin expression, whereas CIMP-high tumors show a decreased expression of E-cadherin (67). These findings indicated that the different tumor subgroups in fact induce different phenotypes in CAFs, resulting in CIMP-specific markers. Combining with existing researches, our findings may provide insights into the role of epigenetic regulation in the TME and its association with CAFs.
Comparison with similar researches
CAFs have been implicated in modulating the immune microenvironment and influencing the response to chemotherapy and immunotherapy. Previous studies found that CAFs affected oxaliplatin and 5FU sensitivity in CRC cells. CRC development and treatment resistance are promoted by platinum-based medication accumulation in CAFs (68,69). In our study, the Riskscore chemotherapy sensitivity analysis may influence the selection of 5-fluorouracil/leucovorin combined with oxaliplatin (FOLFOX) or 5-fluorouracil/leucovorin combined with irinotecan (FOLFIRI) chemotherapy. Based on the forecast from the TIDE algorithm, the gene signature may also provide valuable insights into the interplay between CAFs and the immune response, potentially serving as a predictive tool for immunotherapy response. Moreover, the mutation rate of KRAS differed between high- and low-risk groups, and ssGSEA revealed a significant correlation between the CAF risk score and the KRAS signaling hallmark. Further research is required to determine the relevance of the KRAS pathway in CAF-related risk groups.
Several reports have documented the roles of the three identified markers in this well-established signature in tumor progression and the TME. BOC is a member of the Robo-related Ig/fibronectin superfamily and interacts with the Sonic Hedgehog (SHH) pathway (70). Prior research has highlighted the significance of the Hedgehog signaling pathway in modulating cancer properties and the TME. As a receptor and pathway activator, BOC binds SHH with high-affinity via a specific fibronectin repeat that is essential for activity (71,72). Previous research has established that BOC mediates SHH responsiveness in pancreatic fibroblasts and promotes pancreatic tumor growth (73). Furthermore, it has been proposed that BOC inactivation inhibits the proliferation and development of early medulloblastoma to advanced stages (74). DACT3 is a vital regulator of Wnt/β-catenin signaling and a therapeutic target for controlling Wnt/β-catenin signaling in CRC. Recent evidence suggests that CAF-derived β-catenin accumulation may be a relatively early-stage event in carcinogenesis. For instance, many CAFs infiltrate into invasive tissue in the context of elevated β-catenin levels (75). DACT3 expression has been connected to prognosis and proven to be associated with the pathological stage of colon cancer, according to prior bioinformatics analyses (76). Proteomic analysis has revealed that the stromal factor COLEC12 is a high-affinity, inhibitory collagen receptor LAIR1 ligand (77). LAIR1 is broadly expressed in immune cell subsets (78) and leads to poor prognosis in a variety of malignancies (79,80). A recent study suggests that inhibition of LAIR-1 and TGF-β signaling can help remodel the TME and enable PD-L1-mediated tumor eradication (81). In spite of this, as the functional validation of the three genes implicated in the signature of CRC is limited, more research on these three CAF-related biomarkers is necessary.
Limitations and actions needed
Our study demonstrates promising potential in predicting patient outcomes, such as disease progression, treatment response, and OS. However, it is essential to acknowledge the limitations of our gene signature and its implications. Firstly, although we employed rigorous statistical and bioinformatics methods for developing gene signature, the functional validation of the three genes implicated in the signature is limited. Subsequent research endeavors ought to focus on remedying these constraints and augmenting its clinical value in patients with CRC. Prospective validation of the gene signature using other independent patient cohorts is crucial to ascertain its robustness and generalizability. Individual functional validation of the genes comprising the signature could serve as a valuable asset in the development of targeted therapeutic approaches against CAF-mediated processes.
Conclusions
Through comprehensive bioinformatics analysis, we discovered BOC, COLEC12, and DACT3 as new prognostic CAF biomarkers in CRC. A CAF-related signature was constructed and validated using these three indicators, which could precisely predict prognosis, chemotherapeutic resistance, and immunotherapy responses in patients with CRC. This model might give insights into targeting CAFs in CRC therapy.
Acknowledgments
This research represents partial fulfillment of the requirements for a Master’s degree for Jinhui Hu.
Funding: None.
Footnote
Reporting Checklist: The authors have completed the TRIPOD reporting checklist. Available at https://tcr.amegroups.com/article/view/10.21037/tcr-23-261/rc
Peer Review File: Available at https://tcr.amegroups.com/article/view/10.21037/tcr-23-261/prf
Conflicts of Interest: All authors have completed the ICMJE uniform disclosure form (available at https://tcr.amegroups.com/article/view/10.21037/tcr-23-261/coif). The authors have no conflicts of interest to declare.
Ethical Statement: The authors are accountable for all aspects of the work in ensuring that questions related to the accuracy or integrity of any part of the work are appropriately investigated and resolved. The study was conducted in accordance with the Declaration of Helsinki (as revised in 2013).
Open Access Statement: This is an Open Access article distributed in accordance with the Creative Commons Attribution-NonCommercial-NoDerivs 4.0 International License (CC BY-NC-ND 4.0), which permits the non-commercial replication and distribution of the article with the strict proviso that no changes or edits are made and the original work is properly cited (including links to both the formal publication through the relevant DOI and the license). See: https://creativecommons.org/licenses/by-nc-nd/4.0/.
References
- Sung H, Ferlay J, Siegel RL, et al. Global Cancer Statistics 2020: GLOBOCAN Estimates of Incidence and Mortality Worldwide for 36 Cancers in 185 Countries. CA Cancer J Clin 2021;71:209-49. [Crossref] [PubMed]
- Morgan E, Arnold M, Gini A, et al. Global burden of colorectal cancer in 2020 and 2040: incidence and mortality estimates from GLOBOCAN. Gut 2023;72:338-44. [Crossref] [PubMed]
- Shaukat A, Kahi CJ, Burke CA, et al. ACG Clinical Guidelines: Colorectal Cancer Screening 2021. Am J Gastroenterol 2021;116:458-79. [Crossref] [PubMed]
- Ciardiello F, Ciardiello D, Martini G, et al. Clinical management of metastatic colorectal cancer in the era of precision medicine. CA Cancer J Clin 2022;72:372-401. [Crossref] [PubMed]
- Wang M, Zhao J, Zhang L, et al. Role of tumor microenvironment in tumorigenesis. J Cancer 2017;8:761-73. [Crossref] [PubMed]
- Mao X, Xu J, Wang W, et al. Crosstalk between cancer-associated fibroblasts and immune cells in the tumor microenvironment: new findings and future perspectives. Mol Cancer 2021;20:131. [Crossref] [PubMed]
- Sahai E, Astsaturov I, Cukierman E, et al. A framework for advancing our understanding of cancer-associated fibroblasts. Nat Rev Cancer 2020;20:174-86. [Crossref] [PubMed]
- Kalluri R. The biology and function of fibroblasts in cancer. Nat Rev Cancer 2016;16:582-98. [Crossref] [PubMed]
- Bonnans C, Chou J, Werb Z. Remodelling the extracellular matrix in development and disease. Nat Rev Mol Cell Biol 2014;15:786-801. [Crossref] [PubMed]
- Koliaraki V, Pallangyo CK, Greten FR, et al. Mesenchymal Cells in Colon Cancer. Gastroenterology 2017;152:964-79. [Crossref] [PubMed]
- Hu C, Zhang Y, Wu C, et al. Heterogeneity of cancer-associated fibroblasts in head and neck squamous cell carcinoma: opportunities and challenges. Cell Death Discov 2023;9:124. [Crossref] [PubMed]
- Ying F, Chan MSM, Lee TKW. Cancer-Associated Fibroblasts in Hepatocellular Carcinoma and Cholangiocarcinoma. Cell Mol Gastroenterol Hepatol 2023;15:985-99. [Crossref] [PubMed]
- Nguyen EV, Pereira BA, Lawrence MG, et al. Proteomic Profiling of Human Prostate Cancer-associated Fibroblasts (CAF) Reveals LOXL2-dependent Regulation of the Tumor Microenvironment. Mol Cell Proteomics 2019;18:1410-27. [Crossref] [PubMed]
- Mohammadi H, Sahai E. Mechanisms and impact of altered tumour mechanics. Nat Cell Biol 2018;20:766-74. [Crossref] [PubMed]
- Jain RK, Martin JD, Stylianopoulos T. The role of mechanical forces in tumor growth and therapy. Annu Rev Biomed Eng 2014;16:321-46. [Crossref] [PubMed]
- DuFort CC, DelGiorno KE, Hingorani SR. Mounting Pressure in the Microenvironment: Fluids, Solids, and Cells in Pancreatic Ductal Adenocarcinoma. Gastroenterology 2016;150:1545-1557.e2. [Crossref] [PubMed]
- Tauriello DVF, Palomo-Ponce S, Stork D, et al. TGFβ drives immune evasion in genetically reconstituted colon cancer metastasis. Nature 2018;554:538-43. [Crossref] [PubMed]
- Langfelder P, Horvath S. WGCNA: an R package for weighted correlation network analysis. BMC Bioinformatics 2008;9:559. [Crossref] [PubMed]
- Xu Y, Zhang Z, Zhang L, et al. Novel module and hub genes of distinctive breast cancer associated fibroblasts identified by weighted gene co-expression network analysis. Breast Cancer 2020;27:1017-28. [Crossref] [PubMed]
- Du Y, Jiang X, Wang B, et al. The cancer-associated fibroblasts related gene CALD1 is a prognostic biomarker and correlated with immune infiltration in bladder cancer. Cancer Cell Int 2021;21:283. [Crossref] [PubMed]
- Liu B, Chen X, Zhan Y, et al. Identification of a Gene Signature for Renal Cell Carcinoma-Associated Fibroblasts Mediating Cancer Progression and Affecting Prognosis. Front Cell Dev Biol 2021;8:604627. [Crossref] [PubMed]
- Biffi G, Tuveson DA. Diversity and Biology of Cancer-Associated Fibroblasts. Physiol Rev 2021;101:147-76. [Crossref] [PubMed]
- Colaprico A, Silva TC, Olsen C, et al. TCGAbiolinks: an R/Bioconductor package for integrative analysis of TCGA data. Nucleic Acids Res 2016;44:e71. [Crossref] [PubMed]
- Ritchie ME, Phipson B, Wu D, et al. limma powers differential expression analyses for RNA-sequencing and microarray studies. Nucleic Acids Res 2015;43:e47. [Crossref] [PubMed]
- Law CW, Chen Y, Shi W, et al. voom: Precision weights unlock linear model analysis tools for RNA-seq read counts. Genome Biol 2014;15:R29. [Crossref] [PubMed]
- Davis S, Meltzer PS. GEOquery: a bridge between the Gene Expression Omnibus (GEO) and BioConductor. Bioinformatics 2007;23:1846-7. [Crossref] [PubMed]
- Gautier L, Cope L, Bolstad BM, et al. affy--analysis of Affymetrix GeneChip data at the probe level. Bioinformatics 2004;20:307-15. [Crossref] [PubMed]
- Irizarry RA, Bolstad BM, Collin F, et al. Summaries of Affymetrix GeneChip probe level data. Nucleic Acids Res 2003;31:e15. [Crossref] [PubMed]
- Yadav ML, Roychoudhury B. Handling missing values: A study of popular imputation packages in R. Knowledge-Based Systems. 2018;160:104-18. [Crossref]
- Leek JT, Johnson WE, Parker HS, et al. The sva package for removing batch effects and other unwanted variation in high-throughput experiments. Bioinformatics 2012;28:882-3. [Crossref] [PubMed]
- Becht E, Giraldo NA, Lacroix L, et al. Estimating the population abundance of tissue-infiltrating immune and stromal cell populations using gene expression. Genome Biol. 2016;17:218. Erratum in: Genome Biol 2016;17:249. [Crossref] [PubMed]
- Racle J, de Jonge K, Baumgaertner P, et al. Simultaneous enumeration of cancer and immune cell types from bulk tumor gene expression data. Elife 2017;6:e26476. [Crossref] [PubMed]
- Aran D, Hu Z, Butte AJ. xCell: digitally portraying the tissue cellular heterogeneity landscape. Genome Biol 2017;18:220. [Crossref] [PubMed]
- Sturm G, Finotello F, List M. Immunedeconv: An R Package for Unified Access to Computational Methods for Estimating Immune Cell Fractions from Bulk RNA-Sequencing Data. Methods Mol Biol 2020;2120:223-32. [Crossref] [PubMed]
- Yoshihara K, Shahmoradgoli M, Martínez E, et al. Inferring tumour purity and stromal and immune cell admixture from expression data. Nat Commun 2013;4:2612. [Crossref] [PubMed]
- Langfelder P, Luo R, Oldham MC, et al. Is my network module preserved and reproducible? PLoS Comput Biol 2011;7:e1001057. [Crossref] [PubMed]
- Therneau TM, Lumley T. Package ‘survival’. R Top Doc 2015;128:28-33.
- Tibshirani R. Regression shrinkage and selection via the lasso. J R Stat Soc Series B (Methodological) 1996;58:267-88. [Crossref]
- Hastie T, Qian J. Glmnet vignette. Stanford September 13, 2016. Available online: https://hastie.su.domains/Papers/Glmnet_Vignette.pdf
- Maeser D, Gruener RF, Huang RS. oncoPredict: an R package for predicting in vivo or cancer patient drug response and biomarkers from cell line screening data. Brief Bioinform 2021;22:bbab260. [Crossref] [PubMed]
- Yang W, Soares J, Greninger P, et al. Genomics of Drug Sensitivity in Cancer (GDSC): a resource for therapeutic biomarker discovery in cancer cells. Nucleic Acids Res 2013;41:D955-61. [Crossref] [PubMed]
- Jiang P, Gu S, Pan D, et al. Signatures of T cell dysfunction and exclusion predict cancer immunotherapy response. Nat Med 2018;24:1550-8. [Crossref] [PubMed]
- Balar AV, Galsky MD, Rosenberg JE, et al. Atezolizumab as first-line treatment in cisplatin-ineligible patients with locally advanced and metastatic urothelial carcinoma: a single-arm, multicentre, phase 2 trial. Lancet 2017;389:67-76. [Crossref] [PubMed]
- Wu T, Hu E, Xu S, et al. clusterProfiler 4.0: A universal enrichment tool for interpreting omics data. Innovation (Camb) 2021;2:100141. [Crossref] [PubMed]
- Subramanian A, Tamayo P, Mootha VK, et al. Gene set enrichment analysis: a knowledge-based approach for interpreting genome-wide expression profiles. Proc Natl Acad Sci U S A 2005;102:15545-50. [Crossref] [PubMed]
- Liberzon A, Birger C, Thorvaldsdóttir H, et al. The Molecular Signatures Database (MSigDB) hallmark gene set collection. Cell Syst 2015;1:417-25. [Crossref] [PubMed]
- Hänzelmann S, Castelo R, Guinney J. GSVA: gene set variation analysis for microarray and RNA-seq data. BMC Bioinformatics 2013;14:7. [Crossref] [PubMed]
- Kassambara A, Kosinski M, Biecek P, et al. survminer: Drawing Survival Curves using'ggplot2'. R package version 0.4.9. 2021. 2021.
- Han C, Liu T, Yin R. Biomarkers for cancer-associated fibroblasts. Biomark Res 2020;8:64. [Crossref] [PubMed]
- Hinshaw DC, Shevde LA. The Tumor Microenvironment Innately Modulates Cancer Progression. Cancer Res 2019;79:4557-66. [Crossref] [PubMed]
- Trédan O, Galmarini CM, Patel K, et al. Drug resistance and the solid tumor microenvironment. J Natl Cancer Inst 2007;99:1441-54. [Crossref] [PubMed]
- De P, Aske J, Dey N. Cancer-Associated Fibroblast Functions as a Road-Block in Cancer Therapy. Cancers (Basel) 2021;13:5246. [Crossref] [PubMed]
- Kanzaki R, Pietras K. Heterogeneity of cancer-associated fibroblasts: Opportunities for precision medicine. Cancer Sci 2020;111:2708-17. [Crossref] [PubMed]
- Houthuijzen JM, Jonkers J. Cancer-associated fibroblasts as key regulators of the breast cancer tumor microenvironment. Cancer Metastasis Rev 2018;37:577-97. [Crossref] [PubMed]
- Borriello L, Nakata R, Sheard MA, et al. Cancer-Associated Fibroblasts Share Characteristics and Protumorigenic Activity with Mesenchymal Stromal Cells. Cancer Res 2017;77:5142-57. [Crossref] [PubMed]
- Guinney J, Dienstmann R, Wang X, et al. The consensus molecular subtypes of colorectal cancer. Nat Med 2015;21:1350-6. [Crossref] [PubMed]
- Isella C, Terrasi A, Bellomo SE, et al. Stromal contribution to the colorectal cancer transcriptome. Nat Genet 2015;47:312-9. [Crossref] [PubMed]
- Lawrenson K, Grun B, Benjamin E, et al. Senescent fibroblasts promote neoplastic transformation of partially transformed ovarian epithelial cells in a three-dimensional model of early stage ovarian cancer. Neoplasia 2010;12:317-25. [Crossref] [PubMed]
- Nee K, Ma D, Nguyen QH, et al. Preneoplastic stromal cells promote BRCA1-mediated breast tumorigenesis. Nat Genet 2023;55:595-606. [Crossref] [PubMed]
- Wang J, Akter R, Shahriar MF, et al. Cancer-Associated Stromal Fibroblast-Derived Transcriptomes Predict Poor Clinical Outcomes and Immunosuppression in Colon Cancer. Pathol Oncol Res 2022;28:1610350. [Crossref] [PubMed]
- Paulsson J, Micke P. Prognostic relevance of cancer-associated fibroblasts in human cancer. Semin Cancer Biol 2014;25:61-8. [Crossref] [PubMed]
- Saigusa S, Toiyama Y, Tanaka K, et al. Cancer-associated fibroblasts correlate with poor prognosis in rectal cancer after chemoradiotherapy. Int J Oncol 2011;38:655-63. [Crossref] [PubMed]
- Zheng H, Liu H, Ge Y, et al. Integrated single-cell and bulk RNA sequencing analysis identifies a cancer associated fibroblast-related signature for predicting prognosis and therapeutic responses in colorectal cancer. Cancer Cell Int 2021;21:552. [Crossref] [PubMed]
- Louault K, Li RR, DeClerck YA. Cancer-Associated Fibroblasts: Understanding Their Heterogeneity. Cancers (Basel) 2020;12:3108. [Crossref] [PubMed]
- Feng S, Xu Y, Dai Z, et al. Integrative Analysis From Multicenter Studies Identifies a WGCNA-Derived Cancer-Associated Fibroblast Signature for Ovarian Cancer. Front Immunol 2022;13:951582. [Crossref] [PubMed]
- Zheng H, Liu H, Li H, et al. Weighted Gene Co-expression Network Analysis Identifies a Cancer-Associated Fibroblast Signature for Predicting Prognosis and Therapeutic Responses in Gastric Cancer. Front Mol Biosci 2021;8:744677. [Crossref] [PubMed]
- Lundberg I. Fibroblasts and ECM in colorectal cancer: Analysis of subgroup specific protein expression and matrix arrangement. 2012.
- Gonçalves-Ribeiro S, Díaz-Maroto NG, Berdiel-Acer M, et al. Carcinoma-associated fibroblasts affect sensitivity to oxaliplatin and 5FU in colorectal cancer cells. Oncotarget 2016;7:59766-80. [Crossref] [PubMed]
- Linares J, Sallent-Aragay A, Badia-Ramentol J, et al. Long-term platinum-based drug accumulation in cancer-associated fibroblasts promotes colorectal cancer progression and resistance to therapy. Nat Commun 2023;14:746. [Crossref] [PubMed]
- Okada A, Charron F, Morin S, et al. Boc is a receptor for sonic hedgehog in the guidance of commissural axons. Nature 2006;444:369-73. [Crossref] [PubMed]
- Tenzen T, Allen BL, Cole F, et al. The cell surface membrane proteins Cdo and Boc are components and targets of the Hedgehog signaling pathway and feedback network in mice. Dev Cell 2006;10:647-56. [Crossref] [PubMed]
- Kang JS, Gao M, Feinleib JL, et al. CDO: an oncogene-, serum-, and anchorage-regulated member of the Ig/fibronectin type III repeat family. J Cell Biol 1997;138:203-13. [Crossref] [PubMed]
- Mathew E, Zhang Y, Holtz AM, et al. Dosage-dependent regulation of pancreatic cancer growth and angiogenesis by hedgehog signaling. Cell Rep 2014;9:484-94. [Crossref] [PubMed]
- Mille F, Tamayo-Orrego L, Lévesque M, et al. The Shh receptor Boc promotes progression of early medulloblastoma to advanced tumors. Dev Cell 2014;31:34-47. [Crossref] [PubMed]
- Zhou L, Yang K, Randall Wickett R, et al. Dermal fibroblasts induce cell cycle arrest and block epithelial-mesenchymal transition to inhibit the early stage melanoma development. Cancer Med 2016;5:1566-79. [Crossref] [PubMed]
- Zhou XG, Huang XL, Liang SY, et al. Identifying miRNA and gene modules of colon cancer associated with pathological stage by weighted gene co-expression network analysis. Onco Targets Ther 2018;11:2815-30. [Crossref] [PubMed]
- Keerthivasan S, Şenbabaoğlu Y, Martinez-Martin N, et al. Homeostatic functions of monocytes and interstitial lung macrophages are regulated via collagen domain-binding receptor LAIR1. Immunity 2021;54:1511-1526.e8. [Crossref] [PubMed]
- Meyaard L. The inhibitory collagen receptor LAIR-1 (CD305). J Leukoc Biol 2008;83:799-803. [Crossref] [PubMed]
- Joseph C, Alsaleem MA, Toss MS, et al. The ITIM-Containing Receptor: Leukocyte-Associated Immunoglobulin-Like Receptor-1 (LAIR-1) Modulates Immune Response and Confers Poor Prognosis in Invasive Breast Carcinoma. Cancers (Basel) 2020;13:80. [Crossref] [PubMed]
- Ramos MIP, Tian L, de Ruiter EJ, et al. Cancer immunotherapy by NC410, a LAIR-2 Fc protein blocking human LAIR-collagen interaction. Elife 2021;10:e62927. [Crossref] [PubMed]
- Horn LA, Chariou PL, Gameiro SR, et al. Remodeling the tumor microenvironment via blockade of LAIR-1 and TGF-β signaling enables PD-L1-mediated tumor eradication. J Clin Invest 2022;132:e155148. [Crossref] [PubMed]