Risk factors and a prognostic model for patients with borderline resectable locally advanced T3–4N0–1 non-small cell lung cancer: a population-based study
Highlight box
Key findings
• An accurate prognostic model was developed to predict individual overall survival (OS) for patients with borderline resectable locally advanced T3–4N0–1 non-small cell lung cancer (NSCLC). Our findings indicated that neoadjuvant therapy can significantly improve the OS of patients diagnosed with T3–4N1 NSCLC, but not T3–4N0.
What is known and what is new?
• The treatment strategy recommended by the 3.2023 version NCCN guidelines for patients with borderline resectable locally advanced T3–4N0–1 NSCLC is still under debate, likely due to the complex composition of this patient population;
• This study developed a multivariable prognostic model to estimate the individual risk or prognosis and further revealed neoadjuvant therapy can improve the OS of patients diagnosed with T3–4N1 NSCLC, but not T3–4N0.
What is the implication, and what should change now?
• This model can be used as a clinical decision tool to predict and improve the prognosis of patients with borderline resectable locally advanced T3–4N0–1 NSCLC.
Introduction
Lung cancer is one of the leading causes of cancer death across the world (1). Among all patients diagnosed with lung cancer, nearly 85% were pathologically defined as non-small cell lung cancer (NSCLC) (2). T3–4 invasion resectable NSCLC without mediastinal nodal involvement (N0–1) is considered as borderline resectable locally advanced NSCLC, and the optimal treatment and prognosis of these patients are still under debate (3-6). Actually, the prognosis of patients with borderline resectable locally advanced T3–4N0–1 NSCLC is remarkable heterogeneous, which can be attributed to the intricate composition of this patient population (7). The 3.2023 version of National Comprehensive Cancer Network (NCCN) guidelines has recommended the same therapeutic strategies [Therapy Non-Small Cell Lung Cancer-7 (NSCL-7)] for this population, including postoperative systemic therapy or systemic therapy both before and after surgery (8). The 5-year overall survival (OS) rate for this therapeutic strategy is around 50% (3). According to the recommendation of NCCN guideline, postoperative systemic therapy has been proven beneficial for all patients with T3–4N0–1 NSCLC. Neoadjuvant chemotherapy has been reported to effectively downgrade the tumor-node-metastasis (TNM) stage of selected patients, increase the likelihood of achieving R0 resection and further improve their prognosis (9). R0 resection and achieving a complete or near-complete pathological response to neoadjuvant chemotherapy (defined as the complete radiologic disappearance of all measurable or assessable disease) has shown an OS rate of 60% (10,11). However, neoadjuvant therapy may also delay or prevent surgery by inducing adverse reactions such as grade 3/4 neutropenia (54%/23%), nausea (27%/2%), esophagitis (17%/3%), and pneumonitis (17%/1%) (12). Furthermore, another clinical study reported that neoadjuvant chemotherapy did not improve the prognosis of patients with T3N0M0 NSCLC, despite a higher rate of R0 resection compared to the control group (13). Therefore, whether patients with borderline resectable locally advanced T3–4N0–1 NSCLC could benefit from neoadjuvant chemotherapy is still obscure (5,13).
TNM staging system as the classical predicting tool for prognosis, does not show sufficient accuracy in prognostic prediction (14). Nomogram is a form of line graph showing the proportions of different variables included in a specific formula, so that the corresponding value for each variable lies on a straight line that intersects all the proportions (15). Currently, medical nomograms are widely used for patient’s risk stratification and show better prognostic prediction accuracy than TNM system (16,17). Actually, the prognosis of patients with borderline resectable locally advanced T3–4N0–1 NSCLC is remarkable heterogeneous (5,13). There is currently no effective model to stratify prognosis and predict outcomes of these patients. Therefore, it is necessary to establish an accurate prognostic model to comprehensively assess risk factors and survival rate of these patients, and further guide clinical practice.
This population-based study collected and analyzed the clinical characteristics and survival data from the Surveillance, Epidemiology, and End Results (SEER) Database of the National Cancer Institute. To achieve rapid assessment in clinical settings, we developed a prognostic nomogram to effectively predict the OS for patients diagnosed with borderline resectable locally advanced T3–4N0–1 NSCLC. Furthermore, we preformed subgroup analysis to identify the specific population subgroup that may benefit from neoadjuvant chemotherapy. We present this article in accordance with the TRIPOD reporting checklist (available at https://tcr.amegroups.com/article/view/10.21037/tcr-23-519/rc).
Methods
Data and cohort selection
From 2010 to 2015, patients pathologically diagnosed with T3–4 invasion, N0–1 NSCLC were extracted from the SEER database, and re-staged according to the 8th edition of the American Joint Committee on Cancer (AJCC) Cancer Staging Manual (18). Detailed criteria for TNM re-staging are descripted in the Appendix 1. In total, 9,087 records met our inclusion criteria: no distant metastasis (M0), surgery performed and invasion. And we excluded patients: (I) with more than one primary tumor (n=2,550); (II) without specific positive histology (n=85); (III) whose primary tumor site was not on the lung lobes (n=313) and (IV) with incomplete clinical information (data on race, primary site, laterality, diagnostic confirmation, surgical procedure, lymph node dissection, grade classification, and systemic treatment were missing, n=1,007). (V) Besides, perioperative deaths (died within 1 month, n=78) were excluded to avoid the interference caused by surgery and other confounders.
As a result, 5,054 patients were finally enrolled in this study cohort. In order to develop and verify the nomogram, the study cohort was divided into a training cohort (70%) and a validation cohort (30%) based on the random number method. This study was conducted in accordance with the Declaration of Helsinki (as revised in 2013).
Covariates
The following information of patients were extracted and categorized from the SEER database: age (<60, 60–69, 70–79, ≥80 years), gender (female, male), race (White, Black, others), primary site (left lower lobe, right lower lobe, left upper lobe, right middle lobe, right upper lobe), surgical procedure (lobectomy, pneumonectomy, others), lymph node removal (none, 1–3, ≥4), differentiation grade (I, II, III, IV), T stage (T3, T4), N stage (N0, N1), neoadjuvant (no, yes) and adjuvant chemotherapy (no, yes). Histological type was divided into lung adenocarcinoma (LUAD), lung squamous cell carcinoma (LUSC) and others (based on SEER database variable “Histology ICD-O-3” (International Classification of Diseases for Oncology).
Outcomes
Primary outcomes were determined as 1-, 3-, and 5-year OS. OS was defined as the time period (months) from the date of diagnosis to the date of death or the last contact. The associations between OS and risk factors were assessed by Cox proportional hazards analyses.
Statistical analyses
Variable selection
Cox proportional hazards regression analyses were conducted in the training cohort (n=3,538) to identify the significant prognostic factors. Age at diagnosis, gender, race, histology, primary site, surgical procedure, lymph node removal, differentiation grade, T stage, N stage, neoadjuvant chemotherapy and adjuvant chemotherapy were included in the univariate cox regression analyses. Odds ratio (OR) and corresponding 95% confidential interval (CI) were applied to assess the effect of each potential prognostic risk factor (19). Then, we combined the significance of univariate cox analysis with clinical importance to screen variables into the multivariate regression model. Finally, the correlation among these variables was assessed, and the least absolute shrinkage and selection operator (LASSO) regression method was employed to mitigate potential multicollinearity.
Development and validation of the nomogram
Following the variable selection process, the prognostic factors were used to develop a visualized dynamic nomogram, where each factor was assigned a score ranging from 0 to 100 (20). The nomogram visually depicts the predicted 1-, 3-, and 5-year OS for each individual based on the cumulative scores of the factors. This established nomogram was verified by measuring discrimination and calibration. Concordance index (C-index) is a statistic tool which quantifies the model’s ability to accurately predict the OS rate based on the individual risk scores (21,22). C-index and the area under the receiver operating characteristic (ROC) curve (AUC) (23) were utilized to assess the discrimination efficiency by bootstrap validation with 1,000 times of resampling from each cohort. Higher C-index and larger AUC were indicative of better prediction accuracy. Calibration curves were applied to assess the uniformity between the actual and the predicted survival probability (24).
Risk stratification and survival analysis
The risk score for each individual was calculated by the summation effects of each risk factor. Based on the median of the risk score, patients were then classified into high-risk or low-risk group (25). Kaplan-Meier curves were plotted to visualize the survival differences between the high-risk and low-risk groups (26). In addition, subgroup survival analyses were conducted to explore the potential subgroups that may benefit from neoadjuvant chemotherapy.
All the above process were carried out in R 4.1.0 (https://www.R-project.org/). The construction and validation of the nomogram were operated with the “rms”, “foreign”, “survival” and “survival ROC” R packages (27). The Kaplan-Meier survival curve was plotted with “survminer” R package. A two-sided significance level was set as P value <0.05 for all statistical testing. We applied the Bonferroni correction method to adjust for multiple hypothesis testing in the results of the univariate analysis, using a significance threshold of P<0.004. P values ranging from 0.004 to 0.05 were considered as suggestive evidence.
Results
Demographic characteristics
The study design is demonstrated in Figure 1. From 2010 to 2015, 5,054 eligible records were eventually enrolled in this study cohort from SEER database. Among these records, 3,538 patients were included in the training cohort, and 1,516 patients were included in the validation cohort. In the training cohort, the median age of patients was 68 years old, and the median follow-up time was 44 (1 to 107) months. Among these patients, there were more males (54.18%) than females. LUAD (56.76%) was the most common pathological subtype. Lobectomy (78.27%) was the most preferred surgical procedure. A total of 305 (8.62%) patients received neoadjuvant chemotherapy, and 1,678 (47.43%) patients received adjuvant chemotherapy. In the validation cohort, the median age of patients was 68 years old, and the median follow-up time was 43 (1 to 107) months. Among these patients, 803 (52.97%) were males, 855 (56.40%) patients diagnosed with LUAD. A total of 1,191 (78.56%) patients underwent lobectomy. Besides, 108 (7.12%) patients received neoadjuvant chemotherapy, and 683 (45.05%) patients received adjuvant chemotherapy. The demographic details were shown in Table 1.
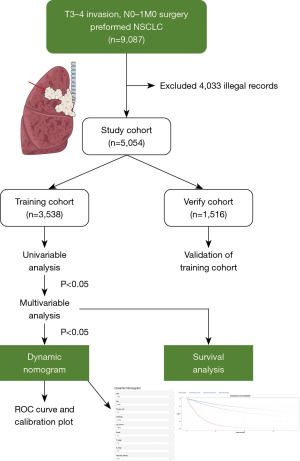
Table 1
Characteristics | Training cohort (n=3,538), n (%) | Validation cohort (n=1,516), n (%) |
---|---|---|
Age (years), median [IQR] | 68 [61–75] | 68 [61–76] |
Gender | ||
Male | 1,917 (54.18) | 803 (52.97) |
Female | 1,621 (45.82) | 713 (47.03) |
Race | ||
White | 2,950 (83.38) | 1,274 (84.04) |
Black | 344 (9.72) | 135 (8.91) |
Others | 244 (6.90) | 107 (7.06) |
Primary site | ||
Left lower lobe | 519 (14.67) | 238 (15.70) |
Right lower lobe | 632 (17.86) | 260 (17.15) |
Left upper lobe | 978 (27.64) | 408 (26.91) |
Right middle lobe | 150 (4.24) | 77 (5.08) |
Right upper lobe | 1,259 (35.59) | 533 (35.16) |
Histology | ||
LUAD | 2,008 (56.76) | 855 (56.40) |
LUSC | 1,204 (34.03) | 494 (32.59) |
Others | 326 (9.21) | 167 (11.02) |
Surgery | ||
Lobectomy | 2,769 (78.27) | 1,191 (78.56) |
Pneumonectomy | 351 (9.92) | 143 (9.43) |
Others | 418 (11.81) | 182 (12.01) |
LN remove (n) | ||
None | 235 (6.64) | 92 (6.07) |
1–3 | 417 (11.79) | 179 (11.81) |
≥4 | 2,886 (81.57) | 1,245 (82.12) |
Grade | ||
I | 457 (12.92) | 208 (13.72) |
II | 1,365 (38.58) | 553 (36.48) |
III | 1,638 (46.30) | 723 (47.69) |
IV | 78 (2.20) | 32 (2.11) |
T stage | ||
T3 | 2,340 (66.14) | 1,024 (67.55) |
T4 | 1,198 (33.86) | 492 (32.45) |
N stage | ||
N0 | 2,511 (70.97) | 1,098 (72.43) |
N1 | 1,027 (29.03) | 418 (27.57) |
Neoadjuvant chemotherapy | ||
No | 3,233 (91.38) | 1,408 (92.88) |
Yes | 305 (8.62) | 108 (7.12) |
Adjuvant chemotherapy | ||
No | 1,860 (52.57) | 833 (54.95) |
Yes | 1,678 (47.43) | 683 (45.05) |
IQR, interquartile range; LUAD, lung adenocarcinoma; LUSC, lung squamous cell carcinoma; LN, lymph node; T, tumor; N, node.
Variable selection
Univariate cox proportional hazards analyses of the training cohort revealed seven risk factors having significant impact on OS of patients with borderline resectable locally advanced T3–4N0–1 NSCLC, including age (P<0.001), gender (P<0.001), surgical procedure (P<0.001), lymph node removal (P<0.001), differentiation grade (P<0.001), N stage (P<0.001) and histology (P<0.001). In addition, suggestive evidence indicated an association between primary site (P<0.05), T stage (P<0.05) and adjuvant chemotherapy (P<0.05) with OS. These potential factors were further utilized for multivariate cox regression analyses. The results of multivariate cox regression model demonstrated that age (P<0.001), gender (P<0.001), primary site (P<0.05), lymph node removal (P<0.001), differentiation grade (P<0.001), T stage (P<0.001), N stage (P<0.001), histology (P<0.001) and adjuvant chemotherapy (P<0.01) were independent prognostic factors (Table 2, Figure 2). No correlation was observed among these variables (Figure S1). The LASSO regression results suggested that all the nine variables were important and there was no bias from multicollinearity (Figure S2).
Table 2
Characteristics | Univariate | Multivariate | |||
---|---|---|---|---|---|
OR (95% CI) | P value | OR (95% CI) | P value | ||
Age (years) | |||||
<60 | Reference | Reference | |||
60–69 | 1.125 (0.9856–1.284) | 0.081 | 1.105 (0.966–1.263) | 0.146 | |
70–79 | 1.444 (1.269–1.642) | <0.001 | 1.455 (1.274–1.663) | <0.001 | |
≥80 | 1.947 (1.648–2.300) | <0.001 | 2.004 (1.684–2.386) | <0.001 | |
Gender | |||||
Female | Reference | Reference | |||
Male | 1.432 (1.306–1.570) | <0.001 | 1.349 (1.228–1.482) | <0.001 | |
Race | |||||
White | Reference | – | – | ||
Black | 0.974 (0.835–1.137) | 0.740 | – | – | |
Others | 0.980 (0.820–1.170) | 0.822 | – | – | |
Primary site | |||||
Left lower lobe | Reference | Reference | |||
Right lower lobe | 1.132 (0.971–1.320) | 0.113 | 1.212 (1.038–1.414) | <0.05 | |
Left upper lobe | 0.968 (0.839–1.118) | 0.659 | 0.957 (0.828–1.106) | 0.548 | |
Right middle lobe | 0.717 (0.547–0.940) | <0.05 | 0.790 (0.601–1.037) | 0.090 | |
Right upper lobe | 0.921 (0.802–1.058) | 0.244 | 0.986 (0.857–1.135) | 0.847 | |
Histology | |||||
LUAD | Reference | Reference | |||
LUSC | 1.414 (1.285–1.555) | <0.001 | 1.216 (1.100–1.345) | <0.001 | |
Others | 1.050 (0.888–1.241) | 0.572 | 0.979 (0.815–1.175) | 0.819 | |
Surgery | |||||
Lobectomy | Reference | Reference | |||
Pneumonectomy | 1.271 (1.098–1.471) | <0.001 | 1.139 (0.974–1.332) | 0.103 | |
Others | 1.079 (0.940–1.240) | 0.282 | 0.937 (0.789–1.113) | 0.457 | |
LN remove (n) | |||||
None | Reference | Reference | |||
1–3 | 0.883 (0.720–1.083) | 0.233 | 0.817 (0.655–1.020) | 0.074 | |
≥4 | 0.755 (0.637–0.894) | <0.001 | 0.626 (0.509–0.769) | <0.001 | |
Grade | |||||
I | Reference | Reference | |||
II | 1.501 (1.268–1.777) | <0.001 | 1.386 (1.161–1.655) | <0.001 | |
III | 1.986 (1.687–2.340) | <0.001 | 1.870 (1.572–2.223) | <0.001 | |
IV | 3.008 (2.213–4.087) | <0.001 | 3.287 (2.380–4.541) | <0.001 | |
T stage | |||||
T3 | Reference | Reference | |||
T4 | 1.318 (1.201–1.446) | <0.05 | 1.273 (1.157–1.400) | <0.001 | |
N stage | |||||
N0 | Reference | Reference | |||
N1 | 1.347 (1.223–1.483) | <0.001 | 1.332 (1.199–1.480) | <0.001 | |
Neoadjuvant chemotherapy | |||||
No | Reference | – | – | ||
Yes | 1.140 (0.974–1.333) | 0.102 | – | – | |
Adjuvant chemotherapy | |||||
No | Reference | Reference | |||
Yes | 0.913 (0.834–0.998) | <0.05 | 0.862 (0.783–0.949) | <0.01 |
OR, odds ratio; CI, confidence interval; LUAD, lung adenocarcinoma; LUSC, lung squamous cell carcinoma; LN, lymph node; T, tumor; N, node.
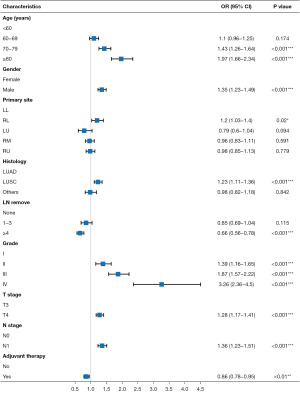
Nomogram construction and validation
To accurately predict the 1-, 3-, 5-year OS of patients with borderline resectable locally advanced T3–4N0–1 NSCLC, a nomogram was developed incorporating the identified independent prognostic factors (Figure 3). Moreover, we developed a dynamic interactive nomogram to facilitate convenient individual risk prediction [Dynamic Nomogram (shinyapps.io)]. The AUC value of the model for predicting 1-, 3-, and 5-year OS were 0.71, 0.67 and 0.66, respectively (Figure 4A). These results indicated that the nomogram achieved a good predictive accuracy. Validation cohort also demonstrated a good consistency (Figure 4B). Apart from this, the calibration curves of OS also showed the inspiring uniformities of this nomogram in both the training cohort (Figure 4C) and the validation cohort (Figure 4D).
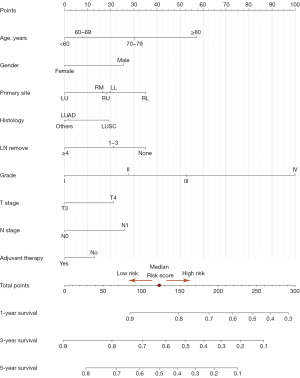
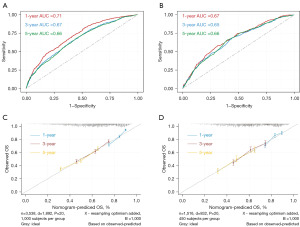
Risk stratification and survival analysis
Patients were divided into high-risk group and low-risk group based on the median risk score of 122.6. Low risk group exhibited significantly better OS compared to the high-risk group in both the training cohort (Figure 5A) and the validation cohort (P<0.001) (Figure 5B). These findings suggested that the developed model had excellent prognostic predictive ability.
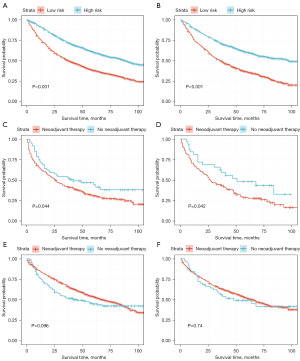
Considering the heterogeneous composition of this patient population, we further conducted subgroup analysis. Our result demonstrated that neoadjuvant chemotherapy application was significantly associated with a better OS in patients diagnosed with T3–4N1 NSCLC (P<0.05) (Figure 5C). The validation cohort showed consistent result (P<0.05) (Figure 5D). However, no significant correlation was observed between neoadjuvant chemotherapy application and OS in patients diagnosed with T3–4N0 NSCLC, neither in the training cohort (P=0.096) (Figure 5E) nor the validation cohort (P=0.74) (Figure 5F).
Discussion
Patients diagnosed with T3–4 invasion, N0–1 resectable NSCLC are considered as borderline resectable locally advanced NSCLC (3,6). Due to the presence of other significant potential risk factors, the assessment of prognosis based on only the TNM staging system is no longer sufficiently accurate (14). It is still a great challenge for clinicians to stratify the prognosis of these borderline resectable patients. Nomogram is a comprehensive assessment tool that integrates multiple risk factors to predict outcomes. It has been demonstrated to possess superior prognostic prediction ability compared to traditional TNM staging in various types of tumors (28-30). Furthermore, nomograms have been successfully employed to predict the subset of patients who may derive benefits from specific therapies (31,32). We developed a prognostic nomogram to comprehensively predict the long-term prognosis of patients with borderline resectable locally advanced T3–4N0–1 NSCLC in this study. In addition, our result revealed that patients diagnosed with T3–4N1 resectable NSCLC can benefit from neoadjuvant chemotherapy.
To the best of our knowledge, this is the first study focusing on the prognosis of patients with borderline resectable locally advanced T3–4N0–1 NSCLC. The calibration curves showed an excellent coherence both in the training cohort and the validation cohort, which demonstrated that the nomogram had satisfying prognostic forecasting abilities. Besides, dynamic interactive nomogram can serve as a convenient tool for estimating individual risk in patients with borderline resectable locally advanced T3–4N0–1 NSCLC.
According to univariate and multivariate analyses, we identified nine independent prognostic factors including age, gender, primary site, lymph node removal, differentiation grade, T stage, N stage, histology and adjuvant chemotherapy for patients diagnosed with T3–4 invasion, N0–1 resectable NSCLC. Same as results shown in previous studies, elder age (≥70), male and higher T/N stage were independent factors of unfavorable prognosis (33-35). The results of this study showed that the primary site in right lower lobe was associated with shorter OS, which was consistent with findings from previous studies (36,37). The reasons for this could be attributed to the complexity in the anatomy of the lower lobe lymph system, and the increasing risk of major complications (especially arrhythmias) and mortality for right-sided resection (38). Besides, we found that lymph node removal ≥4 was significantly associated with increased OS. The reason might be that lymph node removal ≥4 was related to more accurate staging and less false-negative staging (39,40). Adenocarcinoma has shown to exhibit better prognosis than squamous cell carcinoma. This is because most adenocarcinomas are well differentiated, while squamous cell carcinomas tend to be poorly differentiated (41). In addition, adenocarcinomas typically originate in the peripheral regions of the lungs, while squamous cell carcinomas tend to arise in more proximal areas. The relatively less surgical damage in peripheral lung cancer may contribute to better treatment outcomes (42).
According to our findings, adjuvant chemotherapy application was associated with better prognosis in patients with T3–4 invasion, N0–1 resectable NSCLC, which was consistent with previous studies (43). However, despite being recommended as an important treatment in the 3.2023 version NCCN guideline, neoadjuvant chemotherapy did not demonstrate the desired effect on prognosis. Previous studies have also reported conflicting results regarding the effectiveness of neoadjuvant chemotherapy in similar patient population (5,13). Considering the heterogeneous composition of this patient population, we preformed subgroup analyses to further explore the potential benefits of neoadjuvant chemotherapy in specific subgroups. It was observed that neoadjuvant chemotherapy could significantly improve the OS of patients diagnosed with T3–4N1 NSCLC, while in the subgroup of patient with T3–4N0 NSCLC, an opposite trend was observed (P>0.05). Due to the inclusion of only surgically treated patients in our study, we were unable to evaluate whether neoadjuvant chemotherapy could serve as a useful tool for improving patient selection for surgery. However, the conclusion we can draw is that the combination of neoadjuvant chemotherapy can improve OS in patients with resectable T3–4N1 NSCLC who undergo surgery. The reason might be that neoadjuvant chemotherapy decreased the nodal stage and increased the R0 resection rate in T3–4N1 patients. However, in the T3–4N0 subgroup, the potential negative effects of neoadjuvant chemotherapy might outweigh the benefits. Another population-based study revealed the same result (44).
Neoadjuvant immunotherapy has been employed in various types of cancer (45,46). Since our data did not include information on immunotherapy, the potential benefits of neoadjuvant immunotherapy in T3–4N0 patients still require further discussion. A recent meta-analysis has reported that neoadjuvant immunotherapy achieves a higher rate of major pathological response (MPR) in patients diagnosed with stage II/III NSCLC compared to neoadjuvant chemotherapy alone. Furthermore, MPR has been found to be associated with improved OS [hazard ratio (HR) 0.80, 95% CI: 0.72–0.88, P<0.001] (45). This suggests that neoadjuvant immunotherapy seems to be beneficial for patients diagnosed with both T3–4N0 and T3–4N1 NSCLC. It is worth noting that factors influencing the achievement of MPR should be taken into consideration before administering neoadjuvant immunotherapy (47,48).
There are some limitations in our study. Firstly, this is a retrospective population-based cohort study, the bias caused by data integrity and homogeneity was inevitable. Secondly, some potential factors that may have impact on the findings were unavailable from the SEER database, such as smoking, comorbidities, complications, R0 resection, genetic mutation, immunotherapy and targeted therapy were also unavailable from SEER database.
Conclusions
In conclusion, we have developed an accurate prognostic nomogram as a clinical decision tool to predict individual 1-, 3-, and 5-year OS for patients with borderline resectable locally advanced T3–4N0–1 NSCLC. Neoadjuvant chemotherapy could significantly improve the OS of patients diagnosed with T3–4N1 NSCLC, but not patients with T3–4N0 NSCLC. Further prospective studies including more variables are still needed.
Acknowledgments
Funding: This study was supported by
Footnote
Reporting Checklist: The authors have completed the TRIPOD reporting checklist. Available at https://tcr.amegroups.com/article/view/10.21037/tcr-23-519/rc
Peer Review File: Available at https://tcr.amegroups.com/article/view/10.21037/tcr-23-519/prf
Conflicts of Interest: All authors have completed the ICMJE uniform disclosure form (available at https://tcr.amegroups.com/article/view/10.21037/tcr-23-519/coif). LL reports that this study is supported by the 1.3.5 Project for Disciplines of Excellence, West China Hospital, Sichuan University (No. ZYGD18021 to LL). CG reports that this study is supported by the Science and Technology Project of the Health Planning Committee of Sichuan, China (No. 21PJ001 to CG). LX reports that this study is supported by the Key Projects of Sichuan Province (No. 2022YFS0208 to LX). The other authors have no conflicts of interest to declare.
Ethical Statement: The authors are accountable for all aspects of the work in ensuring that questions related to the accuracy or integrity of any part of the work are appropriately investigated and resolved. This study was conducted in accordance with the Declaration of Helsinki (as revised in 2013).
Open Access Statement: This is an Open Access article distributed in accordance with the Creative Commons Attribution-NonCommercial-NoDerivs 4.0 International License (CC BY-NC-ND 4.0), which permits the non-commercial replication and distribution of the article with the strict proviso that no changes or edits are made and the original work is properly cited (including links to both the formal publication through the relevant DOI and the license). See: https://creativecommons.org/licenses/by-nc-nd/4.0/.
References
- Siegel R, Ma J, Zou Z, et al. Cancer statistics, 2014. CA Cancer J Clin 2014;64:9-29. [Crossref] [PubMed]
- Jemal A, Siegel R, Xu J, et al. Cancer statistics, 2010. CA Cancer J Clin 2010;60:277-300. [Crossref] [PubMed]
- Dickhoff C, Senan S, Schneiders FL, et al. Ipilimumab plus nivolumab and chemoradiotherapy followed by surgery in patients with resectable and borderline resectable T3-4N0-1 non-small cell lung cancer: the INCREASE trial. BMC Cancer 2020;20:764. [Crossref] [PubMed]
- Haque W, Verma V, Butler EB, et al. Post-treatment mortality after definitive chemoradiotherapy versus trimodality therapy for locally advanced non-small cell lung cancer. Lung Cancer 2019;127:76-83. [Crossref] [PubMed]
- Kanzaki R, Ose N, Funaki S, et al. The Outcomes of Induction Chemoradiotherapy Followed by Surgery for Clinical T3-4 Non-Small Cell Lung Cancer. Technol Cancer Res Treat 2019;18:1533033819871327. [Crossref] [PubMed]
- Nguyen NA, Isfahanian N, Pond G, et al. A Novel Neoadjuvant Therapy for Operable Locally Invasive Non-Small-Cell Lung Cancer. Phase I Study of Neoadjuvant Stereotactic Body Radiotherapy. LINNEARRE I (NCT02433574). Clin Lung Cancer 2017;18:436-440.e1. [Crossref] [PubMed]
- Uramoto H, Shimokawa H, Hanagiri T, et al. Factors predicting the surgical outcome in patients with T3/4 lung cancer. Surg Today 2014;44:2249-54. [Crossref] [PubMed]
- Ettinger DS, Wood DE, Aisner DL, et al. NCCN Guidelines Insights: Non-Small Cell Lung Cancer, Version 2.2021. J Natl Compr Canc Netw 2021;19:254-66. [Crossref] [PubMed]
- Blumenthal GM, Bunn PA Jr, Chaft JE, et al. Current Status and Future Perspectives on Neoadjuvant Therapy in Lung Cancer. J Thorac Oncol 2018;13:1818-31. [Crossref] [PubMed]
- Rusch VW, Giroux DJ, Kraut MJ, et al. Induction chemoradiation and surgical resection for superior sulcus non-small-cell lung carcinomas: long-term results of Southwest Oncology Group Trial 9416 (Intergroup Trial 0160). J Clin Oncol 2007;25:313-8. [Crossref] [PubMed]
- Vos CG, Hartemink KJ, Blaauwgeers JL, et al. Trimodality therapy for superior sulcus tumours: evolution and evaluation of a treatment protocol. Eur J Surg Oncol 2013;39:197-203. [Crossref] [PubMed]
- Albain KS, Swann RS, Rusch VW, et al. Radiotherapy plus chemotherapy with or without surgical resection for stage III non-small-cell lung cancer: a phase III randomised controlled trial. Lancet 2009;374:379-86. [Crossref] [PubMed]
- Anderson KL Jr, Mulvihill MS, Yerokun BA, et al. Induction chemotherapy for T3N0M0 non-small-cell lung cancer increases the rate of complete resection but does not confer improved survival. Eur J Cardiothorac Surg 2017;52:370-7. [Crossref] [PubMed]
- Wang Y, Li J, Xia Y, et al. Prognostic nomogram for intrahepatic cholangiocarcinoma after partial hepatectomy. J Clin Oncol 2013;31:1188-95. [Crossref] [PubMed]
- Grimes DA. The nomogram epidemic: resurgence of a medical relic. Ann Intern Med 2008;149:273-5. [Crossref] [PubMed]
- Duijm M, Oomen-de Hoop E, van Voort van der Zyp N, et al. The development and external validation of an overall survival nomogram in medically inoperable centrally located early-stage non-small cell lung carcinoma. Radiother Oncol 2021;156:223-30. [Crossref] [PubMed]
- Zuo Z, Zhang G, Song P, et al. Survival Nomogram for Stage IB Non-Small-Cell Lung Cancer Patients, Based on the SEER Database and an External Validation Cohort. Ann Surg Oncol 2021;28:3941-50. [Crossref] [PubMed]
- Lababede O, Meziane MA. The Eighth Edition of TNM Staging of Lung Cancer: Reference Chart and Diagrams. Oncologist 2018;23:844-8.
- Prentice RL, Kalbfleisch JD. Hazard rate models with covariates. Biometrics 1979;35:25-39. [Crossref] [PubMed]
- Iasonos A, Schrag D, Raj GV, et al. How to build and interpret a nomogram for cancer prognosis. J Clin Oncol 2008;26:1364-70. [Crossref] [PubMed]
- Brentnall AR, Cuzick J. Use of the concordance index for predictors of censored survival data. Stat Methods Med Res 2018;27:2359-73. [Crossref] [PubMed]
- Longato E, Vettoretti M, Di Camillo B. A practical perspective on the concordance index for the evaluation and selection of prognostic time-to-event models. J Biomed Inform 2020;108:103496. [Crossref] [PubMed]
- Linden A. Measuring diagnostic and predictive accuracy in disease management: an introduction to receiver operating characteristic (ROC) analysis. J Eval Clin Pract 2006;12:132-9. [Crossref] [PubMed]
- Austin PC, Harrell FE Jr, van Klaveren D. Graphical calibration curves and the integrated calibration index (ICI) for survival models. Stat Med 2020;39:2714-42. [Crossref] [PubMed]
- Rich JT, Neely JG, Paniello RC, et al. A practical guide to understanding Kaplan-Meier curves. Otolaryngol Head Neck Surg 2010;143:331-6. [Crossref] [PubMed]
- D'Arrigo G, Leonardis D, Abd ElHafeez S, et al. Methods to Analyse Time-to-Event Data: The Kaplan-Meier Survival Curve. Oxid Med Cell Longev 2021;2021:2290120. [Crossref] [PubMed]
- Zhou ZR, Wang WW, Li Y, et al. In-depth mining of clinical data: the construction of clinical prediction model with R. Ann Transl Med 2019;7:796. [Crossref] [PubMed]
- Lin JX, Wang ZK, Hong QQ, et al. Assessment of Clinicopathological Characteristics and Development of an Individualized Prognostic Model for Patients With Hepatoid Adenocarcinoma of the Stomach. JAMA Netw Open 2021;4:e2128217. [Crossref] [PubMed]
- Mao Y, Wang X, Xi L, et al. Prediction values of tertiary lymphoid structures in the prognosis of patients with left- and right-sided colon cancer: a multicenter propensity score-matched study. Int J Surg 2023;109:2344-58. [Crossref] [PubMed]
- Wang X, Zheng X, Zhu J, et al. A nomogram and risk classification system for predicting cancer-specific survival in tall cell variant of papillary thyroid cancer: a SEER-based study. J Endocrinol Invest 2023;46:893-901. [Crossref] [PubMed]
- Jiang Y, Liang Z, Chen K, et al. A dynamic nomogram combining tumor stage and magnetic resonance imaging features to predict the response to induction chemotherapy in locally advanced nasopharyngeal carcinoma. Eur Radiol 2023;33:2171-84. [Crossref] [PubMed]
- Wo Y, Peng Y, Wu Z, et al. Individualized prediction of survival benefit from postoperative radiotherapy for patients with malignant pleural mesothelioma. Cancer Med 2023;12:12452-61. [Crossref] [PubMed]
- Hsu CL, Chen JH, Chen KY, et al. Advanced non-small cell lung cancer in the elderly: the impact of age and comorbidities on treatment modalities and patient prognosis. J Geriatr Oncol 2015;6:38-45. [Crossref] [PubMed]
- Visbal AL, Williams BA, Nichols FC 3rd, et al. Gender differences in non-small-cell lung cancer survival: an analysis of 4,618 patients diagnosed between 1997 and 2002. Ann Thorac Surg 2004;78:209-15; discussion 215. [Crossref] [PubMed]
- Xu L, Su H, She Y, et al. Which N Descriptor Is More Predictive of Prognosis in Resected Non-small Cell Lung Cancer: The Number of Involved Nodal Stations or the Location-Based Pathological N Stage? Chest 2021;159:2458-69. [Crossref] [PubMed]
- Ueda K, Murakami J, Tanaka T, et al. Postoperative Complications and Cancer Recurrence: Impact on Poor Prognosis of Lower Lobe Cancer. Ann Thorac Surg 2020;109:1750-6. [Crossref] [PubMed]
- Martin J, Ginsberg RJ, Abolhoda A, et al. Morbidity and mortality after neoadjuvant therapy for lung cancer: the risks of right pneumonectomy. Ann Thorac Surg 2001;72:1149-54. [Crossref] [PubMed]
- Harpole DH, Liptay MJ, DeCamp MM Jr, et al. Prospective analysis of pneumonectomy: risk factors for major morbidity and cardiac dysrhythmias. Ann Thorac Surg 1996;61:977-82. [Crossref] [PubMed]
- Donington JS. Survival After Sublobar Resection Versus Lobectomy for Clinical Stage IA Lung Cancer: Analysis From the National Cancer Database. J Thorac Oncol 2015;10:1513-4. [Crossref] [PubMed]
- Zhou H, Tapias LF, Gaissert HA, et al. Lymph Node Assessment and Impact on Survival in Video-Assisted Thoracoscopic Lobectomy or Segmentectomy. Ann Thorac Surg 2015;100:910-6. [Crossref] [PubMed]
- Wang BY, Huang JY, Chen HC, et al. The comparison between adenocarcinoma and squamous cell carcinoma in lung cancer patients. J Cancer Res Clin Oncol 2020;146:43-52. [Crossref] [PubMed]
- Sánchez-Danés A, Blanpain C. Deciphering the cells of origin of squamous cell carcinomas. Nat Rev Cancer 2018;18:549-61. [Crossref] [PubMed]
- Artal Cortés Á, Calera Urquizu L, Hernando Cubero J. Adjuvant chemotherapy in non-small cell lung cancer: state-of-the-art. Transl Lung Cancer Res 2015;4:191-7. [PubMed]
- Pu CY, Rodwin S, Nelson B, et al. Approach to Resectable N1 Non-Small Cell Lung Cancer: An Analysis of the National Cancer Database. J Surg Res 2021;259:145-53. [Crossref] [PubMed]
- Chen Y, Qin J, Wu Y, et al. Does major pathological response after neoadjuvant Immunotherapy in resectable non-small-cell lung cancers predict prognosis? a systematic review and meta-analysis. Int J Surg 2023;109:2794-807. [Crossref] [PubMed]
- Li S, Yu W, Xie F, et al. Neoadjuvant therapy with immune checkpoint blockade, antiangiogenesis, and chemotherapy for locally advanced gastric cancer. Nat Commun 2023;14:8. [Crossref] [PubMed]
- Sun X, Liu W, Sun L, et al. Maturation and abundance of tertiary lymphoid structures are associated with the efficacy of neoadjuvant chemoimmunotherapy in resectable non-small cell lung cancer. J Immunother Cancer 2022;10:e005531. [Crossref] [PubMed]
- Zhao ZR, Lin ZC, Shen JF, et al. Neoadjuvant Immunotherapy in Oncogene-Positive Non-Small Cell Lung Cancer: A Multicenter Study. Ann Thorac Surg 2023;116:703-10. [Crossref] [PubMed]