A comprehensive pan-cancer analysis identifies a novel glycolysis score and its hub genes as prognostic and immunological biomarkers
Highlight box
Key findings
• The abnormal glycolysis score in tumor correlates with clinical stage and prognosis. High glycolysis score is strongly associated with tumor mutation burden, microsatellite instability, immune checkpoints and an immunosuppressive microenvironment especially in colon adenocarcinoma. The single-nucleotide variation, copy number variation, and DNA methylation of 12 glycolytic key genes affect their expression level and patients’ prognosis. Glycolysis score might predict clinical response to immunotherapy. Twelve key genes are aberrantly expressed in endometrial cancer cells.
What is known and what is new?
• Glycolysis plays significant roles in tumor survival and progression.
• We comprehensively analyzed molecular expression, clinical implications, and immune features of glycolysis score and 12 hub genes among pan-cancer.
What is the implication, and what should change now?
• Glycolysis score and glycolytic key genes could be served as promising biomarkers aiding diagnosis, predicting prognosis and immunotherapy response for some tumor patients. More experiments and clinical trials are needed to verify this result.
Introduction
Background
Glycolysis is a critical pathway of glucose metabolism, providing intermediates for cells to generate energy under hypoxic conditions. Normally, mitochondrial oxidative phosphorylation is the main glucose metabolism pathway to satisfy cellular energy demands. On the other hand, cancer cells adjust their metabolism to meet the demand of biosynthetic energy for their rapid growth, metastasis, and proliferation (1). Even under normoxic conditions, glycolysis is hyperactive in cancer cells, consuming glucose heavily, and producing more lactic acid and less adenosine triphosphate (ATP), a phenomenon known as the “Warburg effect” (2) or aerobic glycolysis. It is well established that cancer is a disease with complex metabolic perturbations and metabolic reprogramming is one of the hallmarks of cancer cells, especially for aerobic glycolysis (3). Previous studies have shown that the transformation from oxidative phosphorylation to glycolysis in tumors could suppress apoptosis by weakening mitochondrial function (4-7). Aerobic glycolysis produces an acidic and hypoxic microenvironment that promotes tumorigenesis, proliferation, and survival and is positively associated with many types of cancer outcomes and clinical prognosis (8,9). In addition, increased lactate levels create a suitable acidic tumor microenvironment (TME) that induces extracellular matrix decomposition and promotes tumor invasion and metastasis (10). Thus, glycolysis plays a pivotal role in the proliferation, growth, and survival of cancer cells.
Immune evasion is also regarded as an important hallmark of cancer, and emerging reports indicate that it is closely related to tumor metabolism (11,12). The accumulation of lactic acid produced by glycolysis leads to the acidic state of the tumor immune microenvironment (TIM), which plays an immune suppressive role (12). Meanwhile, the growth of tumor cells requires a large amount of energy, which can also lead to the low oxygen and low energy state of TIM (13). This microenvironment has a great impact on the human immune system, which can affect the infiltration and functions of immune cells, and promote the immune escape of tumor cells (14,15). Currently, tumor immunotherapy has become a trending topic. Immune-checkpoint therapy is being used for the treatment of diverse cancers, such as cytotoxic T-lymphocyte-associated protein 4 (CTLA-4), and programmed cell death protein 1 (PD-1). Nevertheless, a large number of patients still have limited or no response to current immunotherapy. Moreover, certain genetic heterogeneities, such as tumor mutation burden (TMB), microsatellite instability (MSI), or single-nucleotide variation (SNV) and DNA copy number variation (CNV), have been found to be associated with the response to immunotherapy.
Rationale and knowledge gap
An effective cancer therapeutic approach should consider multiple factors, including integrate targeting more than one hallmark of cancer and genetic alterations. Although it is known that glycolysis affects the development of some tumors, most previous studies have focused on a single gene in a certain tumor type. The mechanisms underlying the impacts of glycolysis on the progression of tumors, prognosis of patients, as well as the association of glycolytic genes with tumor immunity have not been systematically investigated for all cancer types.
Objective
In this study, we aim to evaluate the glycolysis process in 33 human cancer types in the form of glycolysis score, and to explore the role of glycolysis score in prognosis and immune regulation based on multi-omics data. In addition, we comprehensively evaluate the genetics and epigenetics alterations of 12 glycolysis key genes, and their potential impact on prognosis among 33 tumor types, providing an overall picture of glycolysis in cancer for future reference. We present this study in accordance with the REMARK reporting checklist (16) (available at https://tcr.amegroups.com/article/view/10.21037/tcr-23-325/rc).
Methods
Datasets acquisition and processing
This study was conducted in accordance with the Declaration of Helsinki (as revise in 2013). Gene expression data and associated clinical data from a total of 11,160 patients for 33 kinds of tumors, and 712 corresponding normal samples were collected from the UCSC XENA database (http://xena.ucsc.edu/). The RNAseq data from The Cancer Genome Atlas (TCGA) were normalized and log2 transformed. UCSC XENA database was also available to examine gene copy number, methylation, and somatic mutation. The glycolysis gene set was defined based on hallmark gene sets from MSigDB (http://www.gsea-msigdb.org/gsea/downloads.jsp). According to the expression of each gene in the glycolysis gene set in each sample, the single sample gene set enrichment analysis (ssGSEA) was performed using the R Gene Set Variation Analysis (GSVA) package by calling the gsva function with parameter method = “ssgsea”. The ssGSEA score of each sample was then used as its glycolysis score.
Differential expression and prognosis analysis
The R package “limma” was used to analyze the differences in glycolysis score between tumor and their corresponding normal tissues in each cancer type. For prognosis analysis, we first used the “survival” and “survminer” R packages to automatically calculate the optimal cutoff value of glycolysis score in each tumor. Next, patients with different tumor types who had complete survival data were divided into high-score and low-score groups according to the corresponding best cutoff value of glycolysis scores. Kaplan-Meier (K-M) survival plots were then generated to compare the survival time differences. The overall survival (OS) time was computed from the date of diagnosis to all-cause death, and disease-free survival (DFS) time was defined as the interval from to recurrence or death. Furthermore, we compared the distribution of glycolysis scores between different clinical stages in tumor samples of all cancers.
Gene set enrichment analysis
Pearson correlation analysis was performed to identify genes that were positively or negatively correlated with glycolysis in tumor datasets. Then, based on pathways acquired from the reactome database (https://www.kegg.jp/kegg/pathway.html), we conducted Gene Set Enrichment Analysis (GSEA) by “clusterpofiler” R package to explore the downstream pathways of glycolysis.
TME and immune infiltration analysis
To explore the effects of glycolysis on the TME, three biological processes connected to TME, which were reported by Zeng et al. and utilized by Li et al. (17,18), were applied, including immune-related pathways, stromal-related pathways, and DNA repair-related pathways. Subsequently, three diverse approaches were applied for the aim of exploring the abundance of infiltrating immune cells within 33 cancers. Firstly, immune infiltration data were obtained from the Tumor Immune Estimation Resource 2.0 database (TIMER2.0, http://timer.comp-genomics.org/) and were analyzed to estimate the relevance between glycolysis score and the degree of infiltrating immune cells in all TCGA cancers cohorts. Afterwards, we attained the immune infiltration data from the ImmuCellAI database (http://bioinfo.life.hust.edu.cn/ImmuCellAI#!/) and previous published article (19) to revalidate the associations between the degree of glycolysis and immune-related cells.
TMB and MSI
We conducted a thorough analysis to explore the connections between glycolysis score and TMB and MSI in 33 cancer types. The somatic mutation data (alteration frequency and copy number) were derived from the UCSC XENA database, and the MSI data were obtained and processed using the pipeline described by Bonneville et al. (20). Following that, Pearson correlation analyses were performed and lollipop diagrams were generated to summarize the correlation between glycolysis score and TMB and MSI.
Immune checkpoint (ICP) analysis
We obtained the expression data of five well-known ICP-related genes, including CTLA4, LAG3, CD274, TIGIT, and PDCD1, in 33 tumor tissue samples from the TCGA. The expression levels of ICP genes and glycolysis score were analyzed by the Spearman correlation method. Results were shown as circle maps, with green representing negative correlation and red representing positive correlation.
Characteristics of molecular-level changes of glycolysis genes
SNV, CNV, and methylation data for 33 cancers among the studies were retrieved from the Gene Set Cancer Analysis (GSCA, http://bioinfo.life.hust.edu.cn/GSCA/#/) (21). Correlation analysis was used to explore the correlations between CNV and methylation and expression level of key genes. Paired t-test was used to identify differential methylation between the tumor and normal tissue. Genomic data and survival data were subsequently integrated to perform survival analysis using the survival R package.
Drug sensitivity analysis
We collected the half-maximal inhibitory concentration (IC50) values of small molecules from the GSCA and Genomics of Drug Sensitivity in Cancer (GDSC, https://www.cancerrxgene.org) databases. Combining the drug sensitivity data with the mRNA expression profile, Pearson correlation analysis was used to examine the correlation between glycolysis key genes and IC50 of different drugs. The above results were summarized graphically as a heat map.
Predicting response to immunotherapy
Two immunotherapeutic data sets with detailed response data and survival information were retrieved to assess the ability of glycolysis score to predict immunotherapy efficacy. IMvigor210 cohort included patients with metastatic urothelial cancer who were treated with anti-programmed cell death ligand 1 (anti-PD-L1) agent (atezolizumab) (22). GSE91061 dataset acquired from the Gene Expression Omnibus (GEO) (http://www.ncbi.nlm.nih.gov/geo) is a database of advanced melanoma treated with PD-1 inhibitor (nivolumab).
Cell culture and quantitative real-time polymerase chain reaction (qRT-PCR)
The human Ishikawa, HEC-1A, KLE endometrial cancer cell lines, normal endometrial epithelial cells (EECs) were cultured in Dulbecco’s Modified Eagle Medium (DMEM) containing 10% fetal bovine serum (Bio-Channel; Shanghai, China) and 1% (vol/vol) penicillin-streptomycin. TRIzol reagent (CWBio; Jiangsu, China) was used to extract RNA from cells. Then, RNA was reverse-transcribed into cDNA using a Hifair II 1st Strand cDNA Synthesis Kit (Yeason, Shanghai, China), and amplified by real-time PCR assays using Hieff UNICON qPCR SYBR Green Mix (Yeason, Shanghai, China). GAPDH was used as the internal reference. The primers used in this investigation were synthesized by TSINGKE Biotechnology (Beijing, China). The relative RNA expression level was calculated using the 2−∆∆CT method. Three replicate wells were run in each experiment, and the experiment was replicated three times.
Statistical analysis
Wilcoxon Rank Sum Test was used to analyze glycolysis score differences between groups. Spearman or Pearson correlation analysis was used to calculate the correlation between glycolysis score and infiltration of immune cells, TMB, MSI and drug sensitivity. A log-rank K-M method was performed to compare survival outcomes. All data were statistically analyzed by R software (version 4.0.3). The differences were considered significant at P<0.05 (*), P<0.01 (**), P<0.001 (***), and P<0.0001 (****).
Results
Glycolysis score showed great differences in pan-cancer
We first performed ssGSEA to calculate the glycolysis score for each individuals using the gene expression data. Each cancer showed a wide range of glycolysis score, and all have some individuals with high glycolysis score (Figure 1). Colon adenocarcinoma (COAD) has the highest glycolysis score on average across all cancers, whereas acute myeloid leukemia (LAML) has the lowest. The glycolysis process was generally more active in cancer groups with glycolysis score was notably increased in 15 tumor tissues when compared with normal tissues, including BLCA, BRCA, CESC, COAD, ESCA, HNSC, KIRC, KIRP, LIHC, LUAD, LUSC, READ, STAD, THCA, and UCEC (please see Appendix 1 for the complete list of the TCGA cancer-type abbreviations). On the contrary, a lower glycolysis score was found in KICH and PRAD patients (Figure 2).
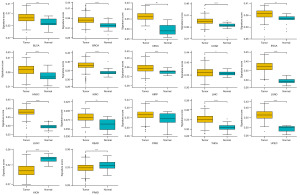
Pan-cancer analysis of the prognostic value of glycolysis score
Patients with zero glycolysis score and incomplete survival data were filtered out and a total of 9,737 patients were included in survival analysis. Then we separated tumor cases into high and low glycolysis score groups to investigate the relationship between glycolysis score and the clinical fate of tumor patients. As Figure 3 demonstrated, high glycolysis score was identified to be linked with a poor OS in a wide range of tumor types. It was noteworthy that high level of glycolysis score did not appear to have a protective function in any type of cancer according to our analysis. We speculated that this relationship was due to the advanced clinical stage, so we further assessed the clinical significance of glycolysis score in different cancer clinical stages. The results confirmed that glycolysis score was associated with tumor stage in 10 tumor types: BLCA (Stage II =130, III =140, IV =133), BRCA (Stage I =182, II =617, III =248, IV =20), HNSC (Stage I =27, II =82, III =93, IV =316), KICH (Stage I =21, II =25, III =14, IV =6), KIRC (Stage I =266, II =57, III =123, IV =81), KIRP (Stage I =177, II =25, III =52, IV =16), LIHC (Stage I =169, II =86, III =85, IV =5), LUAD (Stage I =274, II =122, III =83, IV =26), PAAD (Stage I =21, II =147, III =3, IV =4), and THCA (Stage I =283, II =52, III =112, IV =55), in which glycolysis score was relatively greater as the clinical stage progressed (Figure S1).
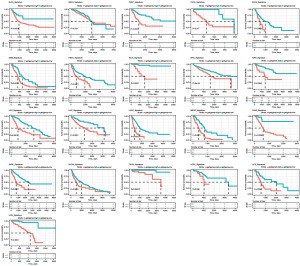
Potential biological pathways of glycolysis in carcinogenesis
To uncover the potential oncogenesis mechanism underneath the glycolysis process, we screened out the top 50 genes that are most relevant to the glycolysis score to perform the GSEA. The top 20 related pathways in BLCA, COAD, ESCA, HNSC, LGG, and LIHC were presented in Figure 4. As shown, among the cancers mentioned above, the signaling pathways correlated with glycolysis score-related genes included the innate immune system, signaling by interleukin, cytokine signaling in the immune system, adaptive immune system, Class I major histocompatibility complex (MHC) mediated antigen processing & presentation, MAPK1/MAPK3 signaling, MAPK family signaling cascades, and signaling by WNT. Therefore, it could be inferred that glycolysis may be involved in pathways associated with tumor physiology and play an important role in immune regulation.
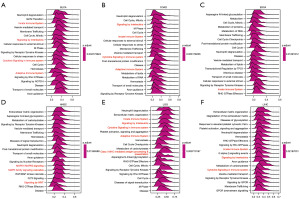
Correlation of glycolysis with the TIM
Together with tumor cells, a large number of immunocytes and stromal cells in tumor tissue form the TME, and continuous communications between these cells exert pivotal functions during the onset and progression of neoplasm (23,24). The association between glycolysis gene expression levels and the TME-related pathways were examined in 33 TCGA tumor samples. In the majority of malignancies, the glycolysis score was closely associated with TME, except for UCS, DLBC, and SARC. The glycolysis score was mainly positive with stromal and mismatch repair-related pathways in diverse tumor types, however, both positive and negative correlations were observed when immune-related pathways were analyzed (Figure 5). It is worth noticing that the glycolysis score demonstrates a strong positive correlation with immune-related pathways, including ICPs, antigen processing mechanisms, and CD8+ T effectors in LGG, UVM, OV, KICH, LIHC, and COAD.
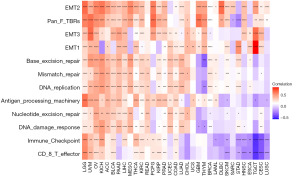
Considering that glycolysis might play an important role in the regulations of TME and immunity, we further explored the correlations between glycolysis score and the level of immune cell infiltration in the TCGA tumor cohorts through three different analytical methods. The results from the TIMER2.0 database (Figure 6) showed that, overall, glycolysis score was notably positively related to the infiltration levels of multiple immune cells including tumor-associated macrophages (TAM), cancer-associated fibroblast (CAF), and neutrophils lineages. Contrarily, negative associations were discovered between glycolysis score and B cells, CD8+ T cells, and natural killer (NK) cells abundance. Importantly, when other methods were used for verifying the above relationship, similar conclusions were obtained. Result from a published article and ImmuCellAI database was that glycolysis score has a positive association with TAM, well negative association with CD8+ T cells and NK cells for most cancer types. For instance, the glycolysis score was positively correlated with macrophage infiltration in BRCA, LGG, LUAD, and STAD in all three datasets. In BRCA, KIRP, LGG, LUAD, LUSC, STAD, and UCEC, glycolysis score was negatively associated with CD8+ T cells and NK cells infiltration levels. Collectively, all the above results further strengthen the immunosuppressive roles of glycolysis in modulating TME.
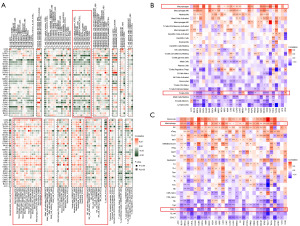
Correlation of glycolysis score with TMB and MSI
As shown in Figure 7, the association between glycolysis score and TMB achieved a significant positive correlation in 10 out of 33 cancers. COAD, READ and UCEC had relatively high coefficients, while PRAD had the lowest coefficients, which indicated that higher glycolysis score is related to higher mutation status in COAD, READ, and UCEC (particularly COAD), but adversely related to lower mutation in PRAD. As for MSI, a highly positive correlation with glycolysis score was observed merely in KICH, COAD, STAD, and THYM. Of note, COAD was the single malignancy in which glycolysis score was closely associated with both TMB and MSI through an overall cohort of tumors. Considering that COAD obtained the highest glycolysis scores, it prompted us to make a deeper investigation of the mutational landscape in COAD patients.
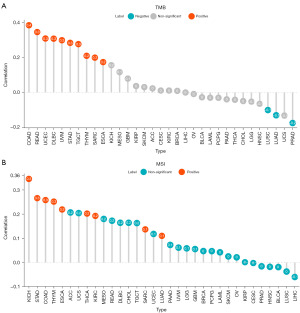
Comparison of mutation frequencies between different glycolysis groups in COAD patients
We conducted a thorough analysis of mutation data from the TCGA COAD cohort. As demonstrated in Figure 8A,8B, the TMB of COAD patients was considerably high with 277 (98.93%) samples possessing somatic mutations. The mutation frequency in the high-score group was higher compared to that in the low-score group: in COAD high glycolysis score group, the mutation frequencies for the top 10 genes increased substantially, with all being greater than 20%, and seven of them greater than 40%. Whereas, in the low score group, only four genes had mutation frequencies greater than 40%. The mutation of gene TTN, APC, KRAS, and TP53 were commonly observed in both groups. A total of 12 genes were identified that had significantly different mutation frequencies (Figure 8C) and all differentially mutated genes were significantly enriched in the high glycolysis score cohort.
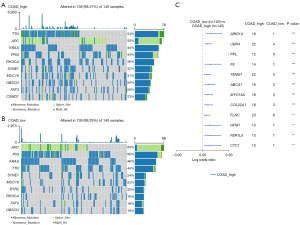
Correlation of glycolysis score with ICP
ICP genes are tightly linked to immune cell infiltration and immunotherapy response (25,26). From the series of findings above, we could deduce that the glycolysis score was correlated with the level of immune infiltration and higher glycolysis score seemed to be associated with inhibited immune status in tumor tissues, so we further explored whether there was a relationship between glycolysis score and the expression of five well-recognized checkpoint genes. As the results showed, correlation analysis indicated a positive connection between glycolysis score and expression of checkpoint genes in diverse cancer types, which was more obviously in LGG, OV, UVM, KICH, LIHC, and COAD (Figure 9). The glycolysis was significantly associated with ICP gene CD274 in LGG, OV, and COAD, with TIGIT in KIHC, with LAG3 in UVM and KICH, with CTLA4 in OV and KICH, and with PDCD1 in all cancer types mentioned above. The findings provided further evidences that glycolysis score was involved in the regulation of the tumor immune response via ICP activity modulation.
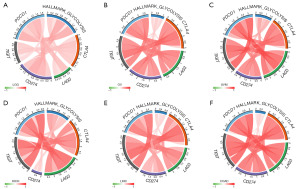
Somatic alteration landscape of 12 glycolysis key genes across pan-cancer
To further investigate the possible mechanism by which glycolysis affects patient prognosis, the Gene Expression Profiling Interactive Analysis 2.0 (GEPIA2.0) database was used to display the correlation between 200 glycolysis genes and OS in pan-cancers (Figure S2). The number of tumor types in which a gene was regarded as a risk factor was subtracted from the number of tumor types in which the same gene was a protective factor to obtain a value. Genes whose values were greater than or equal to 8 were defined as the key genes of glycolysis and were analyzed in the next step. Finally, 12 key genes were screened out for following up in-depth studies: COL5A1, HMMR, PLOD1, P4HA1, GPC1, STC1, SLC16A3, B4GALT2, CDK1, AURKA, TPI1, and CENPA.
According to differential expression analysis based on the GSCA database, we found glycolysis key genes were expressed at a high level in various tumor tissues and almost all key genes were over-expressed in BRCA, HNSC, KIRC, LUAD, LUSC, and COAD. The SNV analysis demonstrated that COL5A1 was the most frequently mutated glycolysis gene across all types of human neoplasm, with the mutation rate being over 20% (22.22%) in SKCM. Additionally, SKCM, UCEC, COAD, and STAD were the most prominent hyper-mutated types across all tumor types. The mutation frequencies of genes COL5A1, HMMR, PLOD1, P4HA1, and GPC1 were relatively high in these tumors. According to survival analysis, it is noteworthy that BRCA sufferers with COL5A1 mutation seem to have a worse OS and STAD sufferers with B4GALT2 mutation seem to have a worse progress-free survival compared to wild-type suffers. In contrast, the PFS of SKCM suffers with CDK1 mutant and UCEC suffers with HMMR and COL5A1 mutant was better than that of the suffers with wild type (Figure 10).
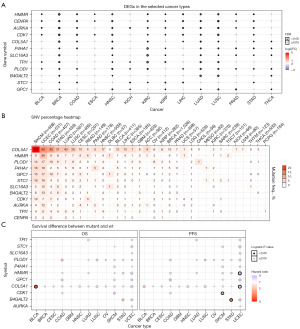
CNVs are the major aberrations that lead to changes in gene expression during tumorigenesis and tumor growth. Our analysis indicated that glycolysis key genes had relatively high CNV frequencies in most cancer types, but few copy number alterations occurred in LAML and THCA compared with other cancers (Figure 11A). The frequency of copy number amplification was generally higher than that of copy number deletion, with heterozygous amplification as the most common mutation among 12 key genes, followed by heterozygous deletion. In addition, genes TPI1 and AURKA had higher amplification mutation frequencies whereas genes PLOD1, STC1, and P4HA1 were more likely to have deletion mutation in the pan-cancer analysis. The correlation analysis between CNV and mRNA expression revealed that CNV was positively connected with the expressions level of the majority of glycolysis key genes (Figure 11B) across 33 cancer types, especially for B4GALT2, TPI1, and AURKA. The exploration of the survival association of glycolysis key genes CNV revealed that ACC, KIRP, LGG, UCEC, and KICH patients with glycolysis genes CNV were more likely to suffer from a poor prognosis (Figure 11C).
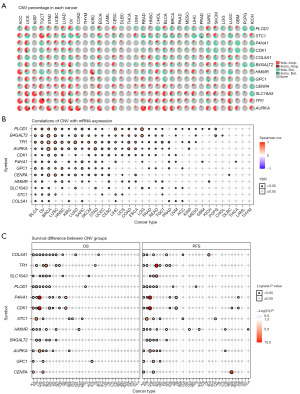
The abnormal methylation of regulatory regions also contributes to the aberrant expression of tumor genes and has a direct impact on both the formation and progression of tumors (27,28). Thus, we analyzed the methylation of the glycolysis key gene to determine epigenetic regulation. The difference in methylation level of key genes between normal and tumor tissues was measured first. As shown in Figure 12A, hypermethylated genes were more common than hypomethylated genes in COAD, PRAD, and UCEC subtypes, while in KIRP and LUSC cohorts, there were more hypomethylated genes than hypermethylated genes. SLC16A3 and P4HA1 were hypomethylated in most cancer types, whereas GPC1 and COL5A1 were more likely to be hypermethylated. Correlation analysis demonstrated that there was a negative correlation between gene expression and their methylation level, which is more obvious in genes SLC16A3, B4GALT2, and COL5A1 (Figure 12B). Survival analysis showed that THYM suffers with CDK1 hypermethylation, DLBC suffers with COL5A1 hypermethylation, PCPG suffers with GPC1 hypermethylation and UVM suffers with B4GALT2 hypermethylation seemed to have a shorter survival duration (Figure 12C).
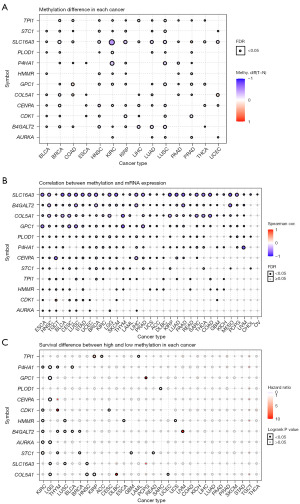
Drug sensitivity analysis
Genomic alterations play an important role in carcinogenesis, disease progression, as well as resistance, and response to targeted therapy. To investigate the role of glycolysis genes in chemo- or targeted therapy, we integrated drug IC50 data and gene expression profiles of cancer cell lines obtained from GSCA database. Correlation analysis results showed that the high level of B4GALT2, AURKA, GPC1, and PLOD1 were correlated with increasing drug resistance to most compounds in both GDSC and CTRP databases (Figure 13), indicating patients with the higher expression level of these glycolysis genes might exhibit drug-resistance when treated with these drugs. On the other hand, increased expression of CENPA and CDK1 was negatively correlated with sensitivity to the majority of drugs, implying that patients with high CENPA and CDK1 expression may be more susceptible to antitumor treatment and these genes may serve as new targets for oncology therapy.
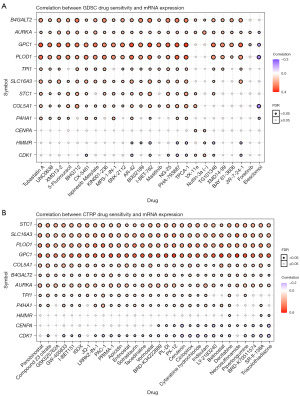
Glycolysis score predicts response to immunotherapy
Given that the immune checkpoint inhibitors (ICIs), represented by anti-PD-1/L1 agents, are the new frontier in anti-tumor treatment, we sought to explore whether glycolysis score could be used as a predictive biomarker for response to immunotherapy. Patients from IMvigor210 and GSE91061 datasets undergoing anti-PD-L1 or anti-PD-1 therapy were used to investigate the relationship between the glycolysis score and immune response. As Figure 14A demonstrated, the K-M survival analysis revealed that in anti-PD-L1 (IMvigor210) cohort, patients with a low glycolysis score exhibited markedly clinical benefits and a significantly prolonged survival time. Similar OS result was observed in the anti-PD-1 (GSE91061) cohort (Figure 14B). Furthermore, in both anti-PD1 and anti-PD-L1 cohorts, a higher response rate was observed in patients with low glycolysis scores compared with those with high glycolysis scores (Figure 14C,14D).
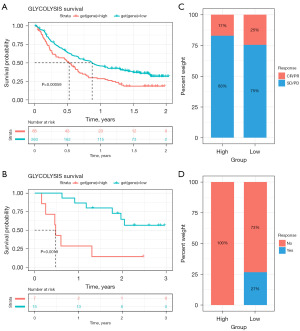
Validation of glycolysis key genes mRNA expression in cell lines
We validated the mRNA expression level of 12 glycolysis key genes in human UCEC (Ishikawa, HEC-1A, KLE) and normal endometrial cell lines (EEC) by RT-qPCR. The results showed that most of these genes were differentially expressed between tumor cells and normal cells (Figure 15). Compared to normal endometrial cells, the UCEC cancer cell lines showed significant high expression of STC1, B4GALT2, B4HA1, CENPA, HMMR, and CDK1. The trend for high PLOD1, TPI1, and AURKA expression approached but did not reach statistical significance. Contrarily, COL5A1 gene expression was significantly downregulated in UCEC cell lines.
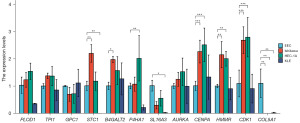
Discussion
Key findings
We explored the profile of the glycolysis score across all TCGA tumor types, with COAD was found to score highest across pan-cancer. In most tumor types, glycolysis score was significantly elevated in tumor tissues compared with normal tissues. Patients with high glycolysis score also had a relatively high mortality risk than those with low score. A high glycolysis score was associated with decreased immunostimulatory NK cells and CD8+ T cells infiltration, well increased immunosuppressive M2-TAM cells infiltration. Other immune-related signatures, including TMB, MSI and ICPs, were all closely interacted with glycolysis score. SNV, CNV, and DNA methylation of 12 glycolysis key genes occurred at different frequencies and showed different impacts on survival outcomes. Furthermore, two immunotherapy cohorts were used to valid the predictive and prognostic value of glycolysis score for immunotherapy outcomes. Finally, 12 key genes were confirmed differentially expressed in normal endometrial and three endometrial cancer cell lines.
Strengths and limitations
The current study performed a comprehensive multi-omics analysis of the glycolytic process in term of glycolysis score and provided an overall picture of glycolysis in pan-cancer for future reference. Nevertheless, it should be pointed out that this study is a retrospective study based on multiple public databases and the above valuable findings are mainly based on the results of bioinformatics analysis. These conclusions require additional experimental confirmation.
Comparison with similar researches
A body of studies focusing on a specific tumor type have revealed that glycolysis-related genes were overexpressed in a variety of tumors and were linked with a poor prognosis of cancer (29-31). Those results were borne out in our study. Previous literatures have indicated that reprogramming of glycolysis metabolism could interact with the TME and immune reaction and is closely related to the occurrence and development of tumors (32,33). Cascone et al. demonstrated that the elevated expression of glycolysis-related genes was associated with poor infiltration and impaired tumor cell-killing ability of T cell. Inhibition of glycolysis enhanced T cell mediated antitumor immunity both in vitro and in vivo (34). However, another study including 14 tumor types suggested that highly glycolytic tumors tend to present an immune-stimulatory TME with a significantly increased ratio between immune-stimulatory CD8+ T cells and immune-inhibitory CD4+ regulatory T cells (35). In the present pan-cancer analysis, we confirmed the immunosuppressive role of glycolysis score: In the majority of tumors, the glycolysis score had a negative correlation with tumor-antagonizing cells, such as NK cells and CD8+ T cells. Contrarily, a positive correlation was observed between glycolysis score and the level of TAMs infiltration across pan-cancer.
Explanations of findings
It is well established that cells in a living body rely mainly on oxidative glucose metabolism for the generation of energy. When cells become cancerous, energy generation by oxidative phosphorylation of glucose is inefficient for the drastically increased demand of tumor cells. As a result, the process of glucose metabolism in tumor cells are reprogrammed and significantly different from that in normal cells (36), which are considered hallmark features of the tumor (37). This phenomenon might be responsible for the significantly elevated glycolysis score in tumor tissues compared with normal tissues. The high glycolysis score is associated with poor prognosis in the majority of tumors, suggesting glycolysis score deserve more attention for its prognostic value.
The TME is the constitutive element in cancer immunity (38). Now, it has become increasingly clear that TME influences the invasion and metastasis of cancer cells and impacts them as they escape. Considering that glucose metabolism as the essential supporter of cancer cell viability and malignant processes, it is important to delve a little deeper into the involvement of the glycolysis process in immune regulation. Our study strongly suggested that the glycolysis process played a broad regulatory role in tumor immunity and were positively related to some crucial TME-related pathways.
TAMs are the predominant tumor-infiltrating immune cell population within the TME. Most macrophages accumulated in the TME are M2 subtypes, which can establish an immunosuppressive TME facilitating tumor cells to evade immune surveillance and metastasis (39,40). NK cells exert an antitumor effect directly by inducing the apoptotic death of tumor cells, and/or indirectly by recruiting dendritic cells to tumor sites to trigger adaptive T cell responses (41). CD8+ T cells are the main effector cells that participate in the immune response which can recognize and kill tumor cells directly. The decrease in the infiltration number of NK cells and CD8+ T cells may also assist tumor cells in evading immune destruction. Hence, the highly active glycolysis process may participate in tumor immune escape and contribute to tumor progression by altering immune infiltration, and that perhaps explained why patients with high glycolysis score showed worse prognosis in terms of tumor immunity.
Immunotherapy represents great signs of progress and breakthroughs in cancer treatment (42). TMB and MSI are two emerging biomarkers associated with immunotherapy response. Currently, it is considered that tumor patients with a high TMB are more susceptible to ICIs, such as PD-1/PD-L1 inhibition (43,44). Our study provided evidence for the underlying associations between glycolysis, TMB, and MSI, reflecting that glycolysis score was positively correlated with TMB and MSI in COAD, STAD, ESCA, and THYM. Among them, the COAD cohort attracted our attention for its highest glycolysis score and strongest correlation with TMB, which implies COAD patients with high glycolysis score may benefit more from ICP blockades. We surmised whether COAD patients with greater glycolysis score also have higher mutation frequency, and we next confirmed this hypothesis using mutational analysis. Subsequently, we performed a correlation analysis of the glycolysis score with five major ICPs. We found that the glycolysis score exhibited positive correlations with the expression of immunosuppressive checkpoint genes in LGG, OV, UVM, KICH, LIHC, and COAD, suggesting that patients with high glycolysis score could respond favorably to ICI therapy in these tumor types. These results presented above strongly indicated that glycolysis score might be considered a useful biomarker for predicting the response to immunotherapy in certain cancer types, especially in COAD as its positive correlations with TMB and expression of ICP molecules. Nevertheless, such associations also implied more frequent mutations of genes in high glycolysis score patients, which may be another reason for their worse survival outcomes.
Among 200 genes included in glycolysis gene set, we further screened 12 key genes based on their impact on the prognosis of patients. Not only that, SNV, CNV, methylation, as well as the effects of these genetic variations on gene expression and prognosis were analyzed across pan-cancer in depth. Glycolysis key genes mutations were frequent in SKCM, UCEC, and COAD, with the highest mutation frequency of 22.22%. The mutation of these glycolysis key genes may result in differential protein expression levels in different cancers. Mutations of glycolysis key genes were not related to the survival of patients, except for mutated COL5A1 and B4GALT2, which were poor prognostic factors in BLCA and STAD, respectively. CNV analysis demonstrated that amplification and deletion of glycolysis key genes in pan-cancer. We additionally found that CNV has a positive effect whereas methylation has a negative effect on the mRNA expression level of glycolysis genes in different tumors. Generally, according to survival analysis of various tumor types, the occurrence of CNV in glycolysis key genes was related to worse patient survival while hypermethylation in key genes was mainly associated with better survival, except hypermethylated CDK1, COL5A1, GPC1, and B4GALT2. The phenomenon of hypomethylation of genes was observed in a wide variety of tumors and has been thought to be an apparently ubiquitous feature of cancer (45). Furthermore, pieces of evidence have shown that global hypomethylation could be associated with poor survival outcomes in tumor patients (46,47). Similar conclusions were reached from the present study.
The immunotherapy response was further assessed by IMvigor210 and GSE91061 cohorts. Low glycolysis score patients had a better prognosis and responded better to immunotherapy. These findings highlight the prognostic and predictive value of glycolysis score for individualized immunotherapy.
Finally, we examined the mRNA expression levels of 12 glycolysis key genes in human cell lines and confirmed the aberrant expression of these genes in UCEC cell lines compared to normal endometrial cell lines.
Implications and actions needed
The glycolysis score might be used as a predictor for clinical prognosis and responsiveness to tumor immunotherapy to help physicians decide curative strategies more efficiently. Further insights into the prognostic and immunomodulatory value of glycolysis score and glycolytic hub genes require rigorous experimental validation and more prospective clinical trials.
Conclusions
The current study comprehensively analyzed molecular expression, clinical implications, and immune features of glycolysis score and 12 glycolytic key genes in pan-cancer. The results revealed that glycolysis score differs in tumor versus normal tissues and correlates with clinical stage and prognosis. Of note, high glycolysis score seemed to be strongly associated with TMB and MSI and immunosuppressive microenvironment in tumors, especially in COAD patients. The genomic alteration profiles of 12 glycolytic key genes regarding the SNV, CNV, and methylation revealed that genomic alterations of these genes have effects on their expression level and impact upon the prognosis of patients. Our study reveals the potential role of glycolysis score and glycolytic key genes as promising indicators for the prognosis and immunotherapeutic efficacy of cancer patients.
Acknowledgments
Funding: This work was supported by grants from
Footnote
Reporting Checklist: The authors have completed the REMARK reporting checklist. Available at https://tcr.amegroups.com/article/view/10.21037/tcr-23-325/rc
Peer Review File: Available at https://tcr.amegroups.com/article/view/10.21037/tcr-23-325/prf
Conflicts of Interest: All authors have completed the ICMJE uniform disclosure form (available at https://tcr.amegroups.com/article/view/10.21037/tcr-23-325/coif). All authors report receiving funding from the National Natural Science Foundation of China (No. 82272710) and the Health Care Scientific and Technology Project of Sichuan Province (No. 2022-1701). The authors have no other conflicts of interest to declare.
Ethical Statement: The authors are accountable for all aspects of the work in ensuring that questions related to the accuracy or integrity of any part of the work are appropriately investigated and resolved. This study was conducted in accordance with the Declaration of Helsinki (as revise in 2013).
Open Access Statement: This is an Open Access article distributed in accordance with the Creative Commons Attribution-NonCommercial-NoDerivs 4.0 International License (CC BY-NC-ND 4.0), which permits the non-commercial replication and distribution of the article with the strict proviso that no changes or edits are made and the original work is properly cited (including links to both the formal publication through the relevant DOI and the license). See: https://creativecommons.org/licenses/by-nc-nd/4.0/.
References
- Schwartz L, Supuran CT, Alfarouk KO. The Warburg Effect and the Hallmarks of Cancer. Anticancer Agents Med Chem 2017;17:164-70. [Crossref] [PubMed]
- Vander Heiden MG, Cantley LC, Thompson CB. Understanding the Warburg effect: the metabolic requirements of cell proliferation. Science 2009;324:1029-33. [Crossref] [PubMed]
- Marcucci F, Rumio C. On the Role of Glycolysis in Early Tumorigenesis-Permissive and Executioner Effects. Cells 2023;12:1124. [Crossref] [PubMed]
- Xu D, Jin J, Yu H, et al. Chrysin inhibited tumor glycolysis and induced apoptosis in hepatocellular carcinoma by targeting hexokinase-2. J Exp Clin Cancer Res 2017;36:44. [Crossref] [PubMed]
- Martinez-Outschoorn UE, Pavlides S, Sotgia F, et al. Mitochondrial biogenesis drives tumor cell proliferation. Am J Pathol 2011;178:1949-52. [Crossref] [PubMed]
- Martinez-Outschoorn UE, Sotgia F, Lisanti MP. Power surge: supporting cells "fuel" cancer cell mitochondria. Cell Metab 2012;15:4-5. [Crossref] [PubMed]
- Sotgia F, Martinez-Outschoorn UE, Howell A, et al. Caveolin-1 and cancer metabolism in the tumor microenvironment: markers, models, and mechanisms. Annu Rev Pathol 2012;7:423-67. [Crossref] [PubMed]
- Lu J. The Warburg metabolism fuels tumor metastasis. Cancer Metastasis Rev 2019;38:157-64. [Crossref] [PubMed]
- Lebelo MT, Joubert AM, Visagie MH. Warburg effect and its role in tumourigenesis. Arch Pharm Res 2019;42:833-47. [Crossref] [PubMed]
- Sutendra G, Michelakis ED. Pyruvate dehydrogenase kinase as a novel therapeutic target in oncology. Front Oncol 2013;3:38. [Crossref] [PubMed]
- Chang CH, Qiu J, O'Sullivan D, et al. Metabolic Competition in the Tumor Microenvironment Is a Driver of Cancer Progression. Cell 2015;162:1229-41. [Crossref] [PubMed]
- Kareva I, Hahnfeldt P. The emerging "hallmarks" of metabolic reprogramming and immune evasion: distinct or linked? Cancer Res 2013;73:2737-42. [Crossref] [PubMed]
- Olivares-Illana V, Riveros-Rosas H, Cabrera N, et al. A guide to the effects of a large portion of the residues of triosephosphate isomerase on catalysis, stability, druggability, and human disease. Proteins 2017;85:1190-211. [Crossref] [PubMed]
- Cao J, Zeng F, Liao S, et al. Effects of glycolysis on the polarization and function of tumor associated macrophages Int J Oncol 2023;62:70. (Review). [Crossref] [PubMed]
- Qiu X, Li Y, Zhang Z. Crosstalk between oxidative phosphorylation and immune escape in cancer: a new concept of therapeutic targets selection. Cell Oncol (Dordr) 2023;46:847-65. [Crossref] [PubMed]
- McShane LM, Altman DG, Sauerbrei W, et al. Reporting recommendations for tumor marker prognostic studies (REMARK). J Natl Cancer Inst 2005;97:1180-4. [Crossref] [PubMed]
- Zeng D, Li M, Zhou R, et al. Tumor Microenvironment Characterization in Gastric Cancer Identifies Prognostic and Immunotherapeutically Relevant Gene Signatures. Cancer Immunol Res 2019;7:737-50. [Crossref] [PubMed]
- Li Z, Li Y, Shen L, et al. Molecular characterization, clinical relevance and immune feature of m7G regulator genes across 33 cancer types. Front Genet 2022;13:981567. [Crossref] [PubMed]
- Thorsson V, Gibbs DL, Brown SD, et al. The Immune Landscape of Cancer. Immunity 2019;51:411-2. [Crossref] [PubMed]
- Bonneville R, Krook MA, Kautto EA, et al. Landscape of Microsatellite Instability Across 39 Cancer Types. JCO Precis Oncol 2017;2017:PO.17.00073.
- Liu CJ, Hu FF, Xia MX, et al. GSCALite: a web server for gene set cancer analysis. Bioinformatics 2018;34:3771-2. [Crossref] [PubMed]
- Mariathasan S, Turley SJ, Nickles D, et al. TGFβ attenuates tumour response to PD-L1 blockade by contributing to exclusion of T cells. Nature 2018;554:544-8. [Crossref] [PubMed]
- Lei X, Lei Y, Li JK, et al. Immune cells within the tumor microenvironment: Biological functions and roles in cancer immunotherapy. Cancer Lett 2020;470:126-33. [Crossref] [PubMed]
- Ohtani H. Focus on TILs: prognostic significance of tumor infiltrating lymphocytes in human colorectal cancer. Cancer Immun 2007;7:4. [PubMed]
- Topalian SL, Drake CG, Pardoll DM. Immune checkpoint blockade: a common denominator approach to cancer therapy. Cancer Cell 2015;27:450-61. [Crossref] [PubMed]
- Petitprez F, Meylan M, de Reyniès A, et al. The Tumor Microenvironment in the Response to Immune Checkpoint Blockade Therapies. Front Immunol 2020;11:784. [Crossref] [PubMed]
- Mehdi A, Rabbani SA. Role of Methylation in Pro- and Anti-Cancer Immunity. Cancers (Basel) 2021;13:545. [Crossref] [PubMed]
- Koch A, Joosten SC, Feng Z, et al. Analysis of DNA methylation in cancer: location revisited. Nat Rev Clin Oncol 2018;15:459-66. [Crossref] [PubMed]
- D'Souza L, Bhattacharya D. Plasma cells: You are what you eat. Immunol Rev 2019;288:161-77. [Crossref] [PubMed]
- Huang G, Chen S, Washio J, et al. Glycolysis-Related Gene Analyses Indicate That DEPDC1 Promotes the Malignant Progression of Oral Squamous Cell Carcinoma via the WNT/β-Catenin Signaling Pathway. Int J Mol Sci 2023;24:1992. [Crossref] [PubMed]
- Cui Y, Leng C. A glycolysis-related gene signatures in diffuse large B-Cell lymphoma predicts prognosis and tumor immune microenvironment. Front Cell Dev Biol 2023;11:1070777. [Crossref] [PubMed]
- Choudhary N, Osorio RC, Oh JY, et al. Metabolic Barriers to Glioblastoma Immunotherapy. Cancers (Basel) 2023;15:1519. [Crossref] [PubMed]
- Xiao C, Tian H, Zheng Y, et al. Glycolysis in tumor microenvironment as a target to improve cancer immunotherapy. Front Cell Dev Biol 2022;10:1013885. [Crossref] [PubMed]
- Cascone T, McKenzie JA, Mbofung RM, et al. Increased Tumor Glycolysis Characterizes Immune Resistance to Adoptive T Cell Therapy. Cell Metab 2018;27:977-987.e4. [Crossref] [PubMed]
- Jiang Z, Liu Z, Li M, et al. Increased glycolysis correlates with elevated immune activity in tumor immune microenvironment. EBioMedicine 2019;42:431-42. [Crossref] [PubMed]
- Kim J, DeBerardinis RJ. Mechanisms and Implications of Metabolic Heterogeneity in Cancer. Cell Metab 2019;30:434-46. [Crossref] [PubMed]
- Dong Y, Tu R, Liu H, et al. Regulation of cancer cell metabolism: oncogenic MYC in the driver's seat. Signal Transduct Target Ther 2020;5:124. [Crossref] [PubMed]
- Yu H, Chen Z, Ballman KV, et al. Correlation of PD-L1 Expression with Tumor Mutation Burden and Gene Signatures for Prognosis in Early-Stage Squamous Cell Lung Carcinoma. J Thorac Oncol 2019;14:25-36. [Crossref] [PubMed]
- Sadhukhan P, Seiwert TY. The role of macrophages in the tumor microenvironment and tumor metabolism. Semin Immunopathol 2023;45:187-201. [Crossref] [PubMed]
- Zhang Q, Sioud M. Tumor-Associated Macrophage Subsets: Shaping Polarization and Targeting. Int J Mol Sci 2023;24:7493. [Crossref] [PubMed]
- Garrido G, Schrand B, Rabasa A, et al. Tumor-targeted silencing of the peptide transporter TAP induces potent antitumor immunity. Nat Commun 2019;10:3773. [Crossref] [PubMed]
- Hu H, Chen Y, Tan S, et al. The Research Progress of Antiangiogenic Therapy, Immune Therapy and Tumor Microenvironment. Front Immunol 2022;13:802846. [Crossref] [PubMed]
- Yarchoan M, Hopkins A, Jaffee EM. Tumor Mutational Burden and Response Rate to PD-1 Inhibition. N Engl J Med 2017;377:2500-1. [Crossref] [PubMed]
- Samstein RM, Lee CH, Shoushtari AN, et al. Tumor mutational load predicts survival after immunotherapy across multiple cancer types. Nat Genet 2019;51:202-6. [Crossref] [PubMed]
- Timp W, Feinberg AP. Cancer as a dysregulated epigenome allowing cellular growth advantage at the expense of the host. Nat Rev Cancer 2013;13:497-510. [Crossref] [PubMed]
- Ma L, Li C, Yin H, et al. The Mechanism of DNA Methylation and miRNA in Breast Cancer. Int J Mol Sci 2023;24:9360. [Crossref] [PubMed]
- Casarotto M, Lupato V, Giurato G, et al. LINE-1 hypomethylation is associated with poor outcomes in locoregionally advanced oropharyngeal cancer. Clin Epigenetics 2022;14:171. [Crossref] [PubMed]