Establishment and verification of a prognostic risk score model based on immune genes for hepatocellular carcinoma in an Asian population
Highlight box
Key findings
• We have found 15 genes significantly associated with prognosis of hepatocellular carcinoma (HCC) in an Asian population and a prognostic model was constructed based on the above genes.
What is known and what is new?
• It is well known that HCC is highly malignant and has a poor prognosis, and the current assessment of prognosis mainly relies on serum alpha-fetoprotein levels and imaging tools.
• In this study, a new prognostic model was constructed for HCC population based on immunogenes and achieved good predictive value.
What is the implication, and what should change now?
• The prognostic model constructed in this study had some predictive value, and MICB/PSMD14/TRAF3/SP1/NDRG1/HDAC1/HRAS/NRAS/SEMA5B/GMFB/ACVR2B/BRD8/MMP12/KITLG/DCK, which are genes involved in the construction of the model, also have the potential to become new targets for HCC treatment in Asian populations. And we still need more in vivo and ex vivo experimental studies to explore and validate it.
Introduction
Liver cancer ranks as the sixth most common cancer worldwide and possesses the fourth highest fatality rate within cancer-related deaths. Hepatocellular carcinoma (HCC) emerges as the predominant type of liver cancer, accounting for around 90% of cases (1). The prevalence and mortality of HCC reach their apex in Asia and Africa. Projections anticipate HCC to ascend to the position of the third highest contributor to cancer-related deaths by 2030 (2). The underlying causes of HCC vary across regions. Hepatitis B virus (HBV) assumes the foremost role in instigating HCC in most parts of Asia (excluding Japan), South America, and Africa. Meanwhile, Western Europe, North America, Japan, and Central and Eastern Europe primarily attribute HCC to hepatitis C virus (HCV) and excessive alcohol consumption (3).
The pathogenesis of HCC involves intricate stages of complexity. Mutations in genes such as TERT, TP53, CTNNB1, abnormalities in Wnt-β-catenin pathway, oxidative stress, MAPK and other cell signaling pathways, autophagy-related pathways, and immune-related effects are all involved in the development of HCC (4-8). The immune system has a significant interplay with the onset of HCC and assumes a pivotal role in its therapeutic approaches. In recent years, immunotherapies such as programmed death 1 (PD-1), programmed death ligand-1 (PD-L1), cytotoxic T-lymphocyte-associated protein 4 (CTLA4) immune checkpoint inhibitors, as well as chimeric antigen receptor (CAR) T and T cell receptor (TCR) T have become popular emerging options for the treatment of HCC. It has been shown in the previous study that the combination of atezolizumab and bevacizumab is effective in improving overall survival as well as progression-free survival in patients with advanced HCC compared to the conventional first-line standard treatment of sorafenib (9). Biomarkers of treatment response in HCC remain limited, and elevated serum alpha-fetoprotein (AFP) level is a definitive marker of poor prognosis, associated with activation of the vascular endothelial growth factor (VEGF) pathway (10).
The current diagnosis of HCC relies on imaging and histopathology. In recent years, liquid biopsy also promises to become an emerging tool for liver cancer diagnosis, with researchers actively pursuing novel biomarkers to advance HCC detection. For example, six cell cycle-linked genes (PLK1, CDC20, HSP90AA1, CHEK1, HDAC1, and NDC80) were chosen to create a prognostic model and shows a good prognostic capacity (11).
Therefore, it is clinically important to explore the role of immune genes in HCC. Given the notable regional diversity in the causative factors of HCC, coupled with the marked prevalence of HCC in Asia. In this study, we opted to investigate a population from Asia, and constructed a prognostic risk score model for the Asian HCC population based on immune genes by analyzing the transcriptomic data and clinical data of Asian patients with HCC from the The Cancer Genome Atlas (TCGA) database. And the study provides new perspectives for the discovery of prognosis assessment methods, new targets for immunotherapy and biomarkers of HCC. We present this article in accordance with the TRIPOD reporting checklist (available at https://tcr.amegroups.com/article/view/10.21037/tcr-23-128/rc).
Methods
The analysis procedure is shown in Figure 1.
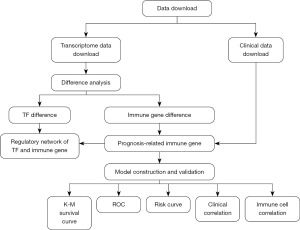
Data acquisition
The transcriptome sequencing data and comprehensive clinical profiles of 161 HCC patients originating from the Asian region were retrieved from The Cancer Genome Atlas Liver Hepatocellular Carcinoma (TCGA-LIHC) project in May 2022 via the TCGA website (https://portal.gdc.cancer.gov/). This encompassed transcriptome data, which included sequencing details of 6 normal samples and 160 tumor samples, alongside comprehensive clinical information meticulously delineated in Table 1.
Table 1
Clinical feature | Classification | Number | Proportion (%) |
---|---|---|---|
Age (years) | ≤65 | 125 | 77.64 |
>65 | 35 | 21.74 | |
Unknown | 1 | 0.62 | |
Gender | Female | 34 | 21.12 |
Male | 127 | 78.88 | |
Grade | G1 | 14 | 8.70 |
G2 | 64 | 39.75 | |
G3 | 71 | 44.10 | |
G4 | 12 | 7.45 | |
Stage | I | 81 | 50.31 |
II | 36 | 22.36 | |
III | 41 | 25.47 | |
IV | 1 | 0.62 | |
Unknown | 2 | 1.24 | |
TNM | |||
T | T1 | 82 | 50.93 |
T2 | 36 | 22.36 | |
T3 | 37 | 22.98 | |
T4 | 6 | 3.73 | |
N | N0 | 149 | 92.55 |
N1 | 1 | 0.62 | |
NX | 11 | 6.83 | |
M | M0 | 153 | 95.03 |
M1 | 1 | 0.62 | |
MX | 7 | 4.35 | |
Survival state | Survival | 117 | 72.67 |
Dead | 44 | 27.33 |
HCC, hepatocellular carcinoma; TNM, tumor-node-metastasis.
Immune genes were downloaded through the ImmPort database (https://immport.org/shared/home), and 318 relevant transcription factors (TFs) were obtained from the cistrome website (http://cistrome.org/). In addition, the information of 6 immune cells: B cells, CD4+ T cells, CD8+ T cells, dendritic cells, macrophages and neutrophils were obtained from the Tumor Immune Estimation Resource (TIMER) website (https://cistrome.shinyapps.io/timer/). This study was conducted in accordance with the Declaration of Helsinki (as revised in 2013).
Differential gene expression analysis
After obtaining the transcriptome information through the TCGA database, genes exhibiting negligible expression levels in both normal and tumor samples were subsequently excluded. The differentiation analysis was executed utilizing the wilcoxTest function within the R programming “environment.setting” the filtering conditions to fdr ≤0.05 (fdr is the value of the correction of P value using fdr method) and |logFC| ≥1:
thus, the genes with differences were screened out. Next, the expression of immune genes acquired from ImmPort database were extracted from the transcriptome data, and the selection of differentially expressed immune genes was carried out employing the identical filtering criteria and methodologies.
Enrichment analysis
The Gene Ontology (GO) and Kyoto Encyclopedia of Genes and Genomes (KEGG) enrichment analysis of differential immune genes obtained above were performed using the enrichplot package in R language based on the GO and the KEGG. The GOs and KEGG pathways associated with each distinct gene were identified through the backend database org.Hs.eg.db. Subsequently, the differentially expressed genes underwent enrichment analysis using Fisher’s exact test, followed by P value computation. GO terms and KEGG pathways conforming to the stipulated criteria were then sifted based on the thresholds of P≤0.05 and q≤0.05 (where q-values denote corrected P values). Furthermore, the GO enrichment analysis was categorized into biological process (BP), cellular component (CC), and molecular function (MF) classifications.
Prognosis-related immune gene analysis
Differential immune genes and survival time (those with follow-up less than 90 days were removed, which were not significant for the analysis) were merged, and prognosis-related immune genes were extracted by univariate Cox analysis through the SURVIVAL package in R, setting a significant filtering criterion of P<0.001.
Analysis of TF and immune gene regulatory network
Differential TF analysis
After obtaining the TFs through the cistrome website, the TFs with differences were filtered out by the same method illustrated in “Differential gene expression analysis”.
TF and immune gene correlation analysis
The correlation coefficient filtering criterion was set to 0.7, and P value to 0.001. The intersect function in R was applied to take the intersection of the two files of differential TF expression and prognosis-related immune gene expression, and the TF as well as immune gene expression of the same samples were output. The “cor.test” in R was used to check the correlation between differential TFs and immune genes, as a result, the correlation coefficients of them were acquired.
Combined with the prognostic analysis results obtained in 1.3, immune genes with hazard ratio (HR) >1 were added high-risk markers, and those with HR <1 were labeled as low-risk immune genes. As for TF, there was no need to be distinguished between high- and low-risk. Thus, we output the regulatory network node attribute file, and the regulatory network of TFs and immune genes was mapped based on this file by applying cytoscape software.
Construction and validation of an immune gene-based prognostic model
The R Survival package was employed to establish the prognostic model utilizing immune-related genes. Initially, the coxph function was employed to extract survival time and survival status, thereby conducting the preliminary construction of the cox model. Subsequently, model refinement ensued via the step function, which systematically eliminated genes exhibiting high correlation. Following this, the optimized model parameters were ascertained through a comprehensive summary analysis:
unction and the coefficients (coef), HR values, 95% confidence interval (CI) of HR values and P values were all output. After obtaining the model coefficients, the predict function was used to calculate the risk score of patients:
the depiction showcases the relationship between gene expression (denoted as “Exp”) and the median risk score derived from the developed prognostic model. Subsequently, patients were stratified into high-risk and low-risk groups based on the median threshold.
The distinction analysis between high- and low-risk groups was executed through the “survdiff” function within the survival package. Subsequently, the “ggsurvplot” function facilitated the generation of Kaplan-Meier (K-M) survival curves, enabling a comparative assessment of the survival statuses within these risk groups. Employing the “survivalROC” package, the receiver operating characteristic (ROC) curve was plotted, and the prognostic model’s accuracy was evaluated based on the area under the curve (AUC). Furthermore, the “pheatmap” package’s plot function was employed to visualize the risk curve. To assess the independent prognostic value of the risk score, univariate and multivariate Cox regression analyses were conducted.
Clinical correlation analysis
All the clinical attributes extracted from the TCGA database were categorized into distinct groups: age (≤65 vs. >65 years), gender (male vs. female), pathological grade (G1–2 vs. G3–4), pathological stage (stage I–II vs. stage III–IV), T stage (T1–2 vs. T3–4), M stage (M0 vs. M1), and N stage (N0 vs. N1). The immune genes incorporated within the model, as well as the risk scores, were scrutinized against each clinical trait, and their correlations were meticulously analyzed using the “beeswarm” package within R. A significance level of P<0.05 was adopted, indicating a potential association between the immune gene expression or risk score and the corresponding clinical trait.
Immune cell correlation analysis
The intersect function in R was applied to take the intersection of the immune cell file acquired from the TIMER database and the risk score file obtained from the immune gene prognostic model in 1.5. And the correlation coefficient and P value were obtained by correlating the immune cell level with the risk score through “cor.test” in R, P<0.05 means the risk score was correlated with the immune cells.
Statistical analysis
In this study, all analyses were completed using RStudio-4.1.0 version. WilcoxTest was applied for difference analysis and correlation analysis was performed by “cor.test”. Cox regression analysis was harnessed for the formulation of the prognostic model, while the K-M survival curve coupled with the log-rank test served to evaluate the survival disparities between the high-risk and low-risk patient cohorts. Further bolstering the assessment, univariate and multivariate Cox regression independent prognostic analyses, in tandem with the ROC curve, were instrumental in appraising the model’s predictive prowess.
Results
Differential genes and prognosis-related immune genes
Six thousand seven hundred and seventy-one differential genes (Figure 2A,2B) as well as 265 differential immune genes (Figure 2C,2D) were obtained by differential analysis through wilcoxTest. Combined with survival time and state, 40 prognosis-related immune genes were screened out through univariate cox analysis (Figure 2E).
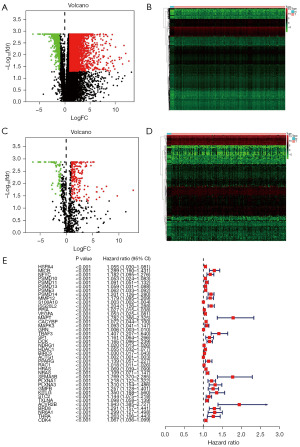
Enrichment analysis
Enrichment analyses encompassing GO and KEGG were executed employing the 265 selected differential immune genes. Figure 3A,3B portrays the foremost 30 outcomes of GO enrichment analysis, categorized across BP, CC, and MF. The GO functional enrichment findings underscored a predominant enrichment of differentially expressed immune genes associated with the positive regulation of the MAPK cascade, cytokine-mediated signaling pathway, proteasome accessory complex, external side of plasma membrane, receptor ligand activity, growth factor activity, etc.
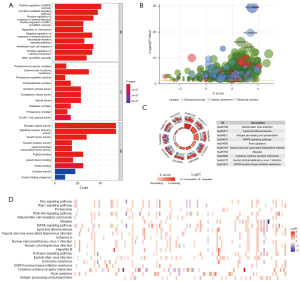
The KEGG enrichment analysis unveiled notable enrichments of the differential immune genes, prominently encompassing Epstein-Barr virus infection, lipid and atherosclerosis, antigen processing and presentation, MAPK signaling pathway, axon guidance, etc. (Figure 3C,3D).
TF and immune gene regulatory network
Differential analysis was performed by wilcoxTest to obtain 123 differential TFs (Figure 4A,4B), and Figure 5 shows the regulatory relationship between TFs and immune genes.
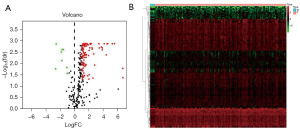
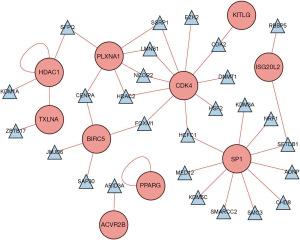
Construction and validation of the prognostic model based on immune genes
Model construction
The risk score model for predicting prognosis was constructed by multivariate Cox regression analysis (Table 2): risk score = (0.204138821 × ExpMICB) + (0.132083415 × ExpPSMD14) + (0.347452557 × ExpTRAF3) + (−0.186333147 × ExpSP1) + (0.019496354 × ExpNDRG1) + (−0.038473968 × ExpHDAC1) + (0.038113834 × ExpHRAS) + (0.088094449 × ExpNRAS) + (0.649885818 × ExpSEMA5B) + (0.12019319 × ExpGMFB) + (0.528385329 × ExpACVR2B) + (0.176246154 × ExpBRD8) + (−0.213311477 × ExpMMP12) + (−0.245472873 × ExpKITLG) + (0.141294643 × ExpDCK). Utilizing the established model, the patients’ risk scores were computed, leading to their stratification into high- and low-risk groups based on the median value (Figure 6).
Table 2
ID | coef | HR | HR.95L | HR.95H | P value |
---|---|---|---|---|---|
MICB | 0.204139 | 1.226468 | 0.980605 | 1.533976 | 0.073714 |
PSMD14 | 0.132083 | 1.141204 | 1.016646 | 1.281022 | 0.025096 |
TRAF3 | 0.347453 | 1.415457 | 1.068684 | 1.874753 | 0.015382 |
SP1 | −0.18633 | 0.829997 | 0.682364 | 1.009571 | 0.062231 |
NDRG1 | 0.019496 | 1.019688 | 1.006543 | 1.033004 | 0.003229 |
HDAC1 | −0.03847 | 0.962257 | 0.923509 | 1.002630 | 0.066548 |
HRAS | 0.038114 | 1.038849 | 0.991445 | 1.088521 | 0.109729 |
NRAS | 0.088094 | 1.092091 | 1.033284 | 1.154245 | 0.001813 |
SEMA5B | 0.649886 | 1.915322 | 1.218577 | 3.010446 | 0.004851 |
GMFB | 0.120193 | 1.127715 | 0.959314 | 1.325677 | 0.145229 |
ACVR2B | 0.528385 | 1.696191 | 1.125172 | 2.557000 | 0.011632 |
BRD8 | 0.176246 | 1.192732 | 1.006169 | 1.413887 | 0.042273 |
MMP12 | −0.21331 | 0.807904 | 0.705287 | 0.925453 | 0.002086 |
KITLG | −0.24547 | 0.782335 | 0.590356 | 1.036743 | 0.087492 |
DCK | 0.141295 | 1.151764 | 0.959701 | 1.382264 | 0.129006 |
coef, coefficients; HR, hazard ratio; 95L, lowest value of 95% confidence interval; 95H, highest value of 95% confidence interval.
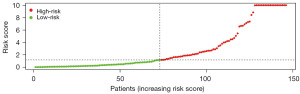
Model validation
K-M survival curve was plotted to compare the survival time of the high- and low-risk groups, and it was found that the survival rate of the high-risk group was significantly lower than that of the low-risk group (Figure 7), with a 5-year survival rate of 45.9% (95% CI: 33.2–63.6%) in the high-risk group and 83.4% (95% CI: 70.4–98.9%) in the low-risk group. The ROC curve of risk score was plotted and an AUC value of 0.901 was obtained, indicating the high accuracy of the model (Figure 8). Figure 9A shows a progressive increase in mortality with increasing risk score, indicating that the high-risk group has a poor prognosis. Figure 9B shows the heat map of the expression of 15 genes involved in the construction of the prognostic model in the high- and low-risk groups. The P values of risk score were found to be less than 0.05 by both univariate and multivariate Cox independent prognostic analysis, indicating that the risk score calculated by the prognostic model can be regarded as an independent prognostic factor (Figure 10A,10B).
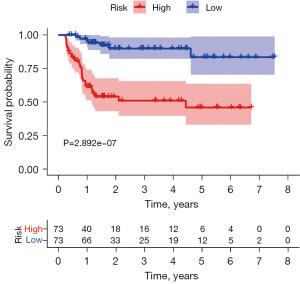
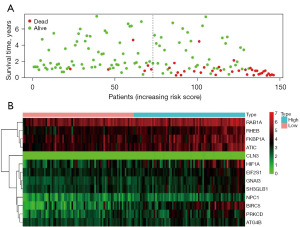
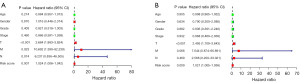
Clinical correlation analysis
A comprehensive comparison was conducted between the 15 immune genes integral to the model construction and the risk score against each clinical trait. As a result, the genes associated with age were found to be ACVR2B, NRAS and SEMA5B, those associated with stage were BRD8, DCK, KITLG, NRAS, SEMA5B and TRAF3, those associated with grade were HDAC1, SEMA5B, SP1 and TRAF3, and those associated with T stage were BRD8, DCK, KITLG, NRAS, SEMA5B and TRAF3 (Figure 11).
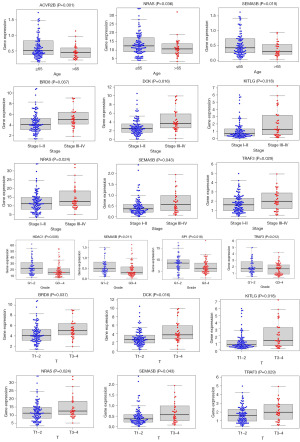
Immune cell correlation analysis
The risk score was positively correlated with the content of all six immune cells including B cell, CD4+ T cell, CD8+ T cell, dendritic cells, macrophages and neutrophils, which had the highest correlation with neutrophil content, with cor =0.541 (Figure 12).
Discussion
HCC is characterized by complex mechanisms of occurrence and poor prognosis. The tumor microenvironment, which is closely related to the function of immunity, plays an important role in the process of this disease. Therefore, the analysis of the effect of immune genes in HCC has been regarded of clinical significance for prognosis prediction and therapeutic target selection. In addition, the etiology of HCC varies around the world and there are large regional differences.
So, in this study, we analyzed the immune gene profile of Asian HCC population and screened 15 immune genes closely associated with prognosis: MICB/PSMD14/TRAF3/SP1/NDRG1/HDAC1/HRAS/NRAS/SEMA5B/GMFB/ACVR2B/BRD8/MMP12/KITLG/DCK. And these genes were applied to construct a risk score prognostic model.
Subsequently, through the visualization of K-M survival curves, a notable disparity emerged: the low-risk value group exhibited a significantly higher survival rate compared to the high-risk value group (P<0.001). Moreover, the outcomes of both univariate and multivariate Cox independent prognostic analyses reinforced the potential of the risk score as an autonomous prognostic indicator for HCC. The construction of a ROC curve further confirmed the model’s efficacy, with an AUC of 0.901, underscoring its considerable predictive capability.
MICB encodes a protein that functions as a ligand for the NKG2D type II receptor. Engagement of this ligand triggers the cytolytic response of immune cells such as natural killer (NK) cells, CD8+ T cells, and gammadelta T cells, all of which express the NKG2D receptor. The previous study have shown that MICB was highly expressed in human HCC and the expression level was significantly and negatively correlated with tumor-node-metastasis (TNM) stage (12).
PSMD14 encodes a protein which is a component of the 19S regulatory cap complex of the 26S proteasome and mediates substrate deubiquitination. Elevated expression of PSMD14 has been ascertained within HCC tissues. The augmented presence of PSMD14 demonstrated a discernible correlation with vascular invasion, tumor multiplicity, recurrence patterns, and notably, dismal tumor-free and overall survival outcomes among HCC patients (13).
The protein arising from TRAF3’s genetic coding belongs to the esteemed TNF receptor associated factor (TRAF) protein family. This particular protein intricately engages in the signal transduction process of CD40, a significant member of the TNFR family that plays a pivotal role in orchestrating the activation of the immune response. It also plays a role in the regulation of antiviral response and cell death initiated by LTbeta ligation. TRAF3 has been found as a novel hepatic ischemia/reperfusion mediator that promotes liver damage and inflammation via TAK1-dependent activation of the JNK and NF-κB pathways (14).
SP1 encodes a sequence-specific DNA-binding protein which regulates the activity of target gene promoters. Elevated expression of SP1 has been identified in both HCC tissues and cell lines. It has been shown that USP39 promotes tumorigenesis by promoting the deubiquitination pathway of SP1 protein, stabilizing it and prolonging its half-lif (15). The development of oxaliplatin resistance in hepatocellular carcinoma treatment is also associated with SP1, and the LINC01134/SP1/p62 axis regulates oxaliplatin resistance by altering cell viability, apoptosis, and mitochondrial homeostasis in vitro and in vivo, thereby affecting the therapeutic efficacy of hepatocellular carcinoma (16).
NDRG1, a constituent of the NDRG gene family, has been highlighted for its pivotal involvement in diverse cellular processes, including growth regulation, differentiation, stress response, and hormonal signaling. Previous investigations have unveiled distinct alterations in NDRG1 expression within the context of liver cancer, wherein the upregulation of NDRG1 expression exhibits a direct positive correlation with the progression of carcinogenesis. These findings suggest that the strategic targeting of NDRG1 could offer a promising avenue for the treatment and potential cure of such malignancies (17).
HDAC1, the product of HDAC1 gene, is classified within the histone deacetylase/acuc/apha family and serves as a constituent of the histone deacetylase complex. This complex plays a pivotal role in orchestrating cellular processes of proliferation and differentiation. The intricate involvement of HDAC1 in HCC’s initiation and progression has been firmly established. Remarkably, research has demonstrated that the utilization of HDAC1 inhibitors exerts a notable inhibitory effect on the proliferation and migratory potential of HCC cells (18).
HRAS belongs to the Ras oncogene family, whose members are related to the transforming genes of mammalian sarcoma retroviruses. The products encoded by these genes function in signal transduction pathways. It was reported that the expression levels of HRAS in HCC cell lines were strongly up-regulated compared to normal cells. And the fact that the influence of HRAS on survival was more obvious in a subgroup of Asian HCC patients may reflect differences in the etiology of liver disease (19).
NRAS is an oncogene encoding a membrane protein that shuttles between the Golgi apparatus and the plasma membrane. The protein encoded by this gene possesses inherent GTPase activity and is activated through interaction with a guanine nucleotide-exchange factor, while its activity is countered by a GTPase activating protein, leading to its deactivation. NRAS protein was significantly upregulated in HCC tissues compared with corresponding nontumorous liver tissues. It was found that increased NRAS expression levels correlated with liver cancer development, metastatic progression, and proliferation (20).
SEMA5B is a constituent of the semaphorins, a cohort of soluble proteins known for orchestrating cellular differentiation, morphology, functionality, and intercellular communications. Its pivotal function extends to the modulation of tumor proliferation, dissemination, along with its involvement in bone metastasis and disorders affecting microvasculature (21).
GMFB functions as a growth and differentiation factor for both glial cells and neurons, showcasing its intricate interplay with inflammatory responses and neurodegenerative processes. Investigations have highlighted the substantial upregulation of GMFB expression in individuals afflicted by HCC, demonstrating a notable correlation with key parameters such as the TNM stage and histopathological grade of the disease. Furthermore, elevated GMFB expression emerges as a prominent predictor of unfavorable overall survival, particularly among male patients as opposed to their female counterparts (22).
ACVR2B encodes a protein which is an activin type 2 receptor. It is dimeric growth and differentiation factor which belongs to the transforming growth factor-beta (TGF-beta) superfamily of structurally related signaling proteins. And it signals through a heteromeric complex of receptor serine kinases. Studies have shown that ACVR2B expression is increased in HCC and is associated with poor prognosis (23,24).
The gene product of BRD8 engages with the thyroid hormone receptor in a manner contingent upon ligand presence, thereby amplifying the thyroid hormone-triggered activation originating from thyroid response elements. Notably, it encompasses a bromodomain and is conjectured to function as an enhancer of nuclear receptor coactivation. BRD8 was over-expressed in HCC and was significantly associated with clinical cancer stages and pathological tumor grades. And high mRNA expressions of BRD8 was promising candidate biomarkers in HCC patients (25,26).
MMP12 encodes a protein engaged in extracellular matrix breakdown during physiological processes like embryonic development, reproduction, and tissue remodeling. It also plays a role in diseases such as arthritis and metastasis. Higher levels of MMP-12 expression indicated a poorer prognosis in patients with HCC. PD-L1 expression was positively correlated with MMP-12 expression, indicating that MMP-12 may promote the development of HCC through the up-regulation of PD-L1 (27,28).
KITLG acts as a ligand for the receptor-type protein-tyrosine kinase KIT. It is crucial in regulating cell survival, proliferation, hematopoiesis, stem cell maintenance, gametogenesis, mast cell development, migration, and function, as well as in melanogenesis. The protein expression of KITLG was enhanced in HCC tissues relative to that in normal hepatic tissues (29,30).
DCK mainly phosphorylates deoxycytidine (dC), converting it into dC monophosphate. Recent biomedical studies have explored DCK’s potential as a therapeutic target for various cancers. Research indicates that DCK expression correlates with patient outcomes and tumor-infiltrating cell levels in HCC. Elevated DCK levels also relate to marker genes of Tregs and exhaustion-related inhibitory receptors, implying its involvement in immunosuppression and immune evasion (31,32).
The study’s limitations include several factors. Initially, the data were sourced from public databases, where the sample size was constrained and missing data were prevalent. Additionally, the retrospective nature of the study introduced various biases. To ensure a more precise verification of the prognostic model’s predictive capacity, larger-scale prospective clinical trials are required. Furthermore, the precise involvement of the genes incorporated into the model in HCC’s pathogenesis remains uncertain.
The prognostic model we have constructed calculates the risk value of each patient more objectively, and based on the median value, we can easily know whether the patient belongs to the high- or low-risk group, and the survival curve can predict the 3- or 5-year survival rate. If this model is extended to the clinic later, we need to use gene sequencing to determine the amount of target genes to calculate the risk value of each patient and group them for prognosis prediction. Gene sequencing has high equipment and technical requirements and is expensive, which is one of the difficulties of this project.
Although the above limitations objectively exist, a series of validation such as K-M survival curve, COX independent prognostic analysis and ROC curve showed that the prognostic model constructed in this study had some predictive value, and MICB/PSMD14/TRAF3/SP1/NDRG1/HDAC1/HRAS/NRAS/SEMA5B/GMFB/ACVR2B/BRD8/MMP12/KITLG/DCK, which are genes involved in the construction of the model, also have the potential to become new targets for HCC treatment in Asian populations.
Conclusions
We have found 15 genes significantly associated with prognosis, namely MICB/PSMD14/TRAF3/SP1/NDRG1/HDAC1/HRAS/NRAS/SEMA5B/GMFB/ACVR2B/BRD8/MMP12/KITLG/DCK, and a prognostic risk score model was constructed based on the above genes. A series of validation such as K-M survival curve, Cox independent prognostic analysis and ROC curve showed that the prognostic model constructed in this study had certain predictive value.
Acknowledgments
Funding: The current study was supported by grants from
Footnote
Reporting Checklist: The authors have completed the TRIPOD reporting checklist. Available at https://tcr.amegroups.com/article/view/10.21037/tcr-23-128/rc
Peer Review File: Available at https://tcr.amegroups.com/article/view/10.21037/tcr-23-128/prf
Conflicts of Interest: All authors have completed the ICMJE uniform disclosure form (available at https://tcr.amegroups.com/article/view/10.21037/tcr-23-128/coif). The authors have no conflicts of interest to declare.
Ethical Statement: The authors are accountable for all aspects of the work in ensuring that questions related to the accuracy or integrity of any part of the work are appropriately investigated and resolved. This study was conducted in accordance with the Declaration of Helsinki (as revised in 2013).
Open Access Statement: This is an Open Access article distributed in accordance with the Creative Commons Attribution-NonCommercial-NoDerivs 4.0 International License (CC BY-NC-ND 4.0), which permits the non-commercial replication and distribution of the article with the strict proviso that no changes or edits are made and the original work is properly cited (including links to both the formal publication through the relevant DOI and the license). See: https://creativecommons.org/licenses/by-nc-nd/4.0/.
References
- McGlynn KA, Petrick JL, London WT. Global epidemiology of hepatocellular carcinoma: an emphasis on demographic and regional variability. Clin Liver Dis 2015;19:223-38. [Crossref] [PubMed]
- Rahib L, Smith BD, Aizenberg R, et al. Projecting cancer incidence and deaths to 2030: the unexpected burden of thyroid, liver, and pancreas cancers in the United States. Cancer Res 2014;74:2913-21. Erratum in: Cancer Res 2014;74:4006. [Crossref] [PubMed]
- International Agency for Research on Cancer. GLOBOCAN 2020. IARC. Available online: https://gco.iarc.fr/today/online-analysis-map?v=2020&mode=population&mode_population=continents&population=900&populations=900&key=asr&sex=0&cancer=11&type=0&statistic=5&prevalence=0&population_groupearth&color_palette=default&map_scale=quantile&map_nb_colors=5&continent=0&rotate=%255B10%252C0%255D
- Llovet JM, Kelley RK, Villanueva A, et al. Hepatocellular carcinoma. Nat Rev Dis Primers 2021;7:6. [Crossref] [PubMed]
- Schulze K, Imbeaud S, Letouzé E, et al. Exome sequencing of hepatocellular carcinomas identifies new mutational signatures and potential therapeutic targets. Nat Genet 2015;47:505-11. [Crossref] [PubMed]
- He S, Tang S. WNT/β-catenin signaling in the development of liver cancers. Biomed Pharmacother 2020;132:110851. [Crossref] [PubMed]
- Qian H, Chao X, Williams J, et al. Autophagy in liver diseases: A review. Mol Aspects Med 2021;82:100973. [Crossref] [PubMed]
- Gentles AJ, Newman AM, Liu CL, et al. The prognostic landscape of genes and infiltrating immune cells across human cancers. Nat Med 2015;21:938-45. [Crossref] [PubMed]
- Galle PR, Finn RS, Qin S, et al. Patient-reported outcomes with atezolizumab plus bevacizumab versus sorafenib in patients with unresectable hepatocellular carcinoma (IMbrave150): an open-label, randomised, phase 3 trial. Lancet Oncol 2021;22:991-1001. [Crossref] [PubMed]
- Montal R, Andreu-Oller C, Bassaganyas L, et al. Molecular portrait of high alpha-fetoprotein in hepatocellular carcinoma: implications for biomarker-driven clinical trials. Br J Cancer 2019;121:340-3. [Crossref] [PubMed]
- Wang J, Li Y, Zhang C, et al. Characterization of diagnostic and prognostic significance of cell cycle-linked genes in hepatocellular carcinoma. Transl Cancer Res 2021;10:4636-51. [Crossref] [PubMed]
- Fang L, Gong J, Wang Y, et al. MICA/B expression is inhibited by unfolded protein response and associated with poor prognosis in human hepatocellular carcinoma. J Exp Clin Cancer Res 2014;33:76. [Crossref] [PubMed]
- Lv J, Zhang S, Wu H, et al. Deubiquitinase PSMD14 enhances hepatocellular carcinoma growth and metastasis by stabilizing GRB2. Cancer Lett 2020;469:22-34. [Crossref] [PubMed]
- Hu J, Zhu XH, Zhang XJ, et al. Targeting TRAF3 signaling protects against hepatic ischemia/reperfusions injury. J Hepatol 2016;64:146-59. [Crossref] [PubMed]
- Dong X, Liu Z, Zhang E, et al. USP39 promotes tumorigenesis by stabilizing and deubiquitinating SP1 protein in hepatocellular carcinoma. Cell Signal 2021;85:110068. [Crossref] [PubMed]
- Ma L, Xu A, Kang L, et al. LSD1-Demethylated LINC01134 Confers Oxaliplatin Resistance Through SP1-Induced p62 Transcription in HCC. Hepatology 2021;74:3213-34. [Crossref] [PubMed]
- Luo Z, Fan Y, Liu X, et al. MiR-188-3p and miR-133b Suppress Cell Proliferation in Human Hepatocellular Carcinoma via Post-Transcriptional Suppression of NDRG1. Technol Cancer Res Treat 2021;20:15330338211033074. [Crossref] [PubMed]
- Al-Yhya N, Khan MF, Almeer RS, et al. Pharmacological inhibition of HDAC1/3-interacting proteins induced morphological changes, and hindered the cell proliferation and migration of hepatocellular carcinoma cells. Environ Sci Pollut Res Int 2021;28:49000-13. [Crossref] [PubMed]
- Dietrich P, Freese K, Mahli A, et al. Combined effects of PLK1 and RAS in hepatocellular carcinoma reveal rigosertib as promising novel therapeutic "dual-hit" option. Oncotarget 2017;9:3605-18. [Crossref] [PubMed]
- Dietrich P, Gaza A, Wormser L, et al. Neuroblastoma RAS Viral Oncogene Homolog (NRAS) Is a Novel Prognostic Marker and Contributes to Sorafenib Resistance in Hepatocellular Carcinoma. Neoplasia 2019;21:257-68. [Crossref] [PubMed]
- Xiong Z, Wu J, Sun Y, et al. Variants in multiple genes are associated with esophageal cancer risk in a Chinese Han population: A case-control study. J Gene Med 2020;22:e3266. [Crossref] [PubMed]
- Sun W, Hu C, Wang T, et al. Glia Maturation Factor Beta as a Novel Biomarker and Therapeutic Target for Hepatocellular Carcinoma. Front Oncol 2021;11:744331. [Crossref] [PubMed]
- Ishikawa S, Kai M, Murata Y, et al. Genomic organization and mapping of the human activin receptor type IIB (hActR-IIB) gene. J Hum Genet 1998;43:132-4. [Crossref] [PubMed]
- Nie Y, Jiao Y, Li Y, et al. Investigation of the Clinical Significance and Prognostic Value of the lncRNA ACVR2B-As1 in Liver Cancer. Biomed Res Int 2019;2019:4602371. [Crossref] [PubMed]
- Monden T, Wondisford FE, Hollenberg AN. Isolation and characterization of a novel ligand-dependent thyroid hormone receptor-coactivating protein. J Biol Chem 1997;272:29834-41. [Crossref] [PubMed]
- Chen YR, Ouyang SS, Chen YL, et al. BRD4/8/9 are prognostic biomarkers and associated with immune infiltrates in hepatocellular carcinoma. Aging (Albany NY) 2020;12:17541-67. [Crossref] [PubMed]
- Belaaouaj A, Shipley JM, Kobayashi DK, et al. Human macrophage metalloelastase. Genomic organization, chromosomal location, gene linkage, and tissue-specific expression. J Biol Chem 1995;270:14568-75. [Crossref] [PubMed]
- Gao H, Zhou X, Li H, et al. Role of Matrix Metallopeptidase 12 in the Development of Hepatocellular Carcinoma. J Invest Surg 2021;34:366-72. [Crossref] [PubMed]
- Gaudet P, Livstone MS, Lewis SE, et al. Phylogenetic-based propagation of functional annotations within the Gene Ontology consortium. Brief Bioinform 2011;12:449-62. [Crossref] [PubMed]
- Hu B, Yang XB, Sang XT. Molecular subtypes based on immune-related genes predict the prognosis for hepatocellular carcinoma patients. Int Immunopharmacol 2021;90:107164. [Crossref] [PubMed]
- Nathanson DA, Armijo AL, Tom M, et al. Co-targeting of convergent nucleotide biosynthetic pathways for leukemia eradication. J Exp Med 2014;211:473-86. [Crossref] [PubMed]
- Song D, Wang Y, Zhu K, et al. DCK is a promising prognostic biomarker and correlated with immune infiltrates in hepatocellular carcinoma. World J Surg Oncol 2020;18:176. [Crossref] [PubMed]