A new prognostic model for glioblastoma multiforme based on coagulation-related genes
Highlight box
Key findings
• There is a potential correlation between the expression of 5 prognostic biomarker genes (PLAUR, GP6, C5AR1, SERPINA5, F2RL2) and the advancement of glioma.
What is known and what is new?
• A correlation exists between coagulation impairment and the processes of glioblastoma multiforme (GBM) invasion, metastasis, as well as the overall prognosis of patients. Within the glioma microenvironment, thrombin is generated through the activation of protease-activated receptors by thrombin. The involvement of coagulation factors, such as thrombin and tissue factors, is pivotal in the onset and progression of GBM.
• A comprehensive screening was conducted to identify 5 biomarkers that exhibit a significant association with the progression of GBM.
What is the implication, and what should change now?
• In the future, we will persist in conducting further research on the role of these 5 biomarkers in the pathogenesis of GBM, with the potential to alter the incidence and progression of glioma through these mechanisms.
Introduction
Gliomas are primary malignant tumors originating from glial cells in the central nervous system, comprising about 75% of brain tumors (1,2). In clinical terms, gliomas are divided into low-grade gliomas (LGGs) and glioblastoma multiformes (GBMs). GBMs grow rapidly, whereas LGGs grow slowly and can be resolved through surgical resection. Generally, patients with LGGs have a good prognosis (3). In contrast, GBM is a type of grade IV malignant glioma that is aggressive and resistant to treatment. GBM accounts for 50% of primary brain tumors in adults and results in over 15,000 fatalities annually in the United States (4). The development of a treatment plan for GBM patients requires a multidisciplinary approach (5). Although there have been multiple innovations in the treatment of GBM, it remains one of the most complex and difficult cancers to treat (6). Despite improvements in surgical resection rates, chemotherapy rates, and radiation rates, patients diagnosed with GBM continue to experience low survival rates, with a median overall survival (OS) of less than 2 years (7,8). In view of the limited treatment options available for GBM, new prognostic models are urgently needed to accurately and conveniently predict survival for patients with GBM.
One of the most important innate defense mechanisms is the coagulation system, especially tissue factor (TF) (9). The functions of these host-protected pathways are used by tumor cells for shaping the tumor microenvironment (TME), which they rely on for sustained metastasis. Research findings indicate that individuals with malignant tumors exhibit hypercoagulation and hyperfibrinolysis, denoting an augmented propensity for clot formation and heightened production of fibrin during the initial stages of the disease. This observation implies a correlation between coagulation impairment and the processes of tumor invasion, metastasis, as well as the overall prognosis of patients (10). Evidence has suggested that FGB of fibrinopeptide A increases when the clotting pathway is activated by tumors, resulting in fibrinolysis and the release of D-dimers. Coagulation, fibrinolysis, and platelet activation pathways can be blocked effectively to prevent tumor progression (11). In the meantime, it is possible to prevent metastatic tumor proliferation by inhibiting prothrombin activator, thrombin, and fibrin stages of clotting cascades and blocking selective platelet activation pathways (12). Invasive GBM is associated with hypercoagulable status, and the data support the hypothesis that plasma hypercoagulable status is associated with adverse outcomes in GBM patients (13). Currently, several biomarkers associated with coagulation disorders have shown significant prognostic associations in various cancers (14,15). The research conducted by Meli et al. demonstrated that the activation of thrombin and PAR-1 induces the production of pro-inflammatory factors, thereby promoting the proliferation of glioma (16). Thrombin, a protease, triggers the activation of a group of receptors known as protease-activated receptors (PARs), which include PAR-1, PAR-3, and PAR-4. Literature has reported a significant elevation in the incidence of venous thrombosis among glioma patients (17), which is attributed to the release of thrombin outside the blood vessels due to an imbalance in coagulation/fibrinolysis in patients with varying degrees of malignancy (18). Glioma stem cells (GSCs) activate platelets in a plasma-independent manner by utilizing both endogenous and extrinsic coagulation cascade factors. The production of thrombin by GSCs results in platelet activation, which in turn leads to the release of glioma platelets and subsequent promotion of vascular production of GBM endothelial cells. This process contributes to the complexity of the glioma vascular network (19). Within the glioma microenvironment, thrombin is generated through the activation of PARs by thrombin. Hence, the involvement of coagulation factors, such as thrombin and TFs, is pivotal in the onset and progression of glioma (20).
In this study, a first attempt was made to identify the prognostic effect and potential mechanism of coagulation-related genes (CRGs) in GBM based on the GBM-related public datasets, and a nomogram was constructed for clinical utilization of the CRGs-based prognostic signature. Besides, correlation of the signature and TME was systematically examined. The study aimed to provide reference for further treatment and prognostic prediction for GBM patients. We present this article in accordance with the TRIPOD reporting checklist (available at https://tcr.amegroups.com/article/view/10.21037/tcr-23-322/rc).
Methods
Data sources
Transcriptome sequencing data of TCGA-GBM dataset (including 5 healthy samples and 154 GBM samples) were downloaded from The Cancer Genome Atlas (TCGA) database (https://portal.gdc.cancer.gov/), wherein 153 GBM samples had complete clinical information. Moreover, RNA sequencing (RNA-seq) data of CGGA-GBM cohort were derived from the Chinese Glioma Genome Atlas (CGGA) database (http://www.cgga.org.cn/), wherein 657 case samples had associated follow-up information. CRGs within 2 coagulation pathways (hsa04610 and hsa04611) were collected from the Kyoto Encyclopedia of Genes and Genomes (KEGG) database (https://www.kegg.jp/kegg/pathway.html) (21). This study was conducted in accordance with the Declaration of Helsinki (as revised in 2013).
Identification of the differentially expressed CRGs (DECRGs)
Differentially expressed genes (DEGs) between GBM and control groups in TCGA-GBM dataset were identified using limma R package (Version 3.48.3; https://cran.r-project.org/) (22). The following thresholds were adopted: |log2fold change (FC)| >1, P<0.05, and Ave Expr >1. Ggplot2 (Version 3.3.5) and pheatmap (Version 1.0.12) within R (23) were conducted to visualize gene expression of DEGs. Following overlapping the DEGs and CRGs, the DECRGs were selected for further analysis.
Construction and evaluation of the prognostic signature
Survival-related DECRGs were screened by the univariate and multivariate Cox proportional hazards regression analyses to construct the prognostic signature. Firstly, a total of 153 patients with complete clinical information in TCGA-GBM dataset were divided into a training set (n=108) and a testing set (n=45) with a ratio of 7:3. The risk score of each patient was calculated with the ‘predict.coxph’ function of survival R package (Version 3.2-13) (24), then the populations in the training set and testing set were stratified into high- and low-risk groups according to the median value of risk score. Kaplan-Meier (K-M) curves between two risk groups were plotted by survminer (Version 0.4.9) (25). Receiver operating characteristic (ROC) curve analysis was used to further evaluate the predictive accuracy of the prognostic signature. Similarly, the CGGA-GBM dataset (n=657) was chosen to independently validate the prognostic performance of the signature. Besides, clinical phenotype data in the training set, that is, treatment type, age, gender, and the prognostic signature, were analyzed using univariate and multivariate statistical analyses to investigate the independent prognostic factors of GBM patients. The nomogram model with the endpoints of OS was constructed by integrating the signature and clinical factors with a P value <0.05.
Function enrichment analyses of signature genes
To interpret the signature genes-related underlying mechanisms, gene set enrichment analysis (GSEA) was used to determine gene sets with significantly difference between high- and low-risk groups (|NES| >1, NOM P<0.05, q<0.25). Signature genes were uploaded to the Metascape database (https://metascape.org/gp/index.html#/main/step1) (26) to excavate the enriched Gene Ontology (GO), KEGG pathways. Furthermore, single cell analysis of signature genes was performed through the cancer single-cell state atlas (CancerSEA) database (27).
Immune infiltration in TME of GBM
Considering the complex TME in GBM, the connections between 22 immune cells activity and the prognostic signature were investigated using the Cell-type Identification By Estimating Relative Subsets Of RNA Transcripts (CIBERSORT) analysis (28). Moreover, the immune score, Estimation of Stromal and Immune cells in Malignant Tumor tissues using Expression data (ESTIMATE) score, and stromal score were calculated by the ESTIMATE algorithm (29).
Statistical analysis
All analyses were conducted using R language software. If not specified above, P<0.05 was regarded as statistically significant.
Results
Identification of DECRGs
Following the differential expressed analysis in TCGA-GBM dataset (Figure 1A,1B), a total of 78 DECRGs between GBM and control groups were identified by overlapping 5,556 DEGs and 202 CDGs (Figure 1, Table S1).
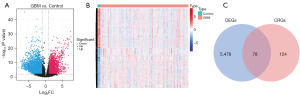
Development and validation of the prognostic signature of GBM
In order to explore the prognostic role of DECRGs, a total of five survival-related DECRGs (PLAUR, GP6, C5AR1, SERPINA5, F2RL2) (P<0.05) were identified to establish the prognostic signature (Figure 2A,2B; Tables S2,S3). Then, the high- and low-risk groups in the training set and testing set were classified according to the medium-risk score. As shown in Figure 3A-3F, patients with a high risk score had worse survival states and poor prognosis. The area under the ROC curve (AUC) values for survival prediction at 1–5 years were greater than 0.6, suggesting the prognostic signature had excellent efficiency. Meanwhile, it was further validated that the prognostic signature involved had a robust accuracy for survival prediction in CGGA-GBM dataset (Figure 3G-3I).
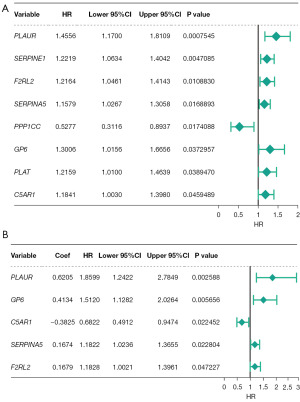
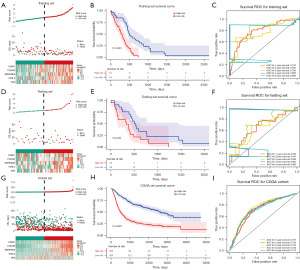
Results of the independent prognostic analyses suggested that only risk score and treatment type had independent prognostic value (Figure 4A,4B, Tables S4,S5). Therefore, a nomogram was drawn with the C-index of 0.6721 (Figure 4C). The calibration curve of the nomogram demonstrated a good agreement between predicted probabilities and the actual observed outcome of OS at 1- and 3-years (Figure 4D).
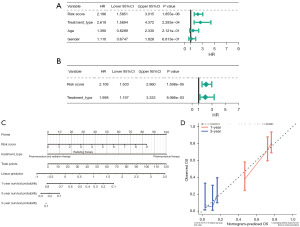
Biological process (BP) and pathway of signature genes
We conducted three enrichment analyses to explain the biological significance of signature genes, that is, GSEA analysis, function and pathway enrichment analysis, and single-cell analysis. The GSEA results indicated that a total of 6,385 GO and 174 KEGG pathways were significantly enriched in high- and low-risk groups, such as the BP of humoral immune response, the cellular components (CC) of external side of plasma membrane, and the molecular functions (MF) of cytokine activity (Figure 5A, available online: https://cdn.amegroups.cn/static/public/tcr-23-322-1.xlsx). According to the prediction results from Metascape database, these signature genes were markedly enriched in response to wounding, blood coagulation, and hemostasis (Figure 5B, Tables S6,S7). In addition, single-cell analysis of signature genes demonstrated that F2RL2 and SERPINA5 were significantly negatively correlated with invasion process, whereas PLAUR was closely related to the functions of metastasis, inflammation, and hypoxia (Figure 5C, Table S8).
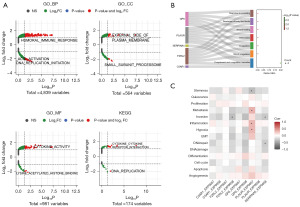
Exploration of immune microenvironment and generation of TME scores
From the perspective of immune infiltration analysis, the infiltration levels of 22 immune cells are displayed in Figure 6A, in which 5 innate immune cells demonstrated a distinct difference between high- and low-risk groups, namely, M2 macrophages, resting mast cells, neutrophils, activated natural killer (NK) cells, and resting memory CD4+ T cells. Simultaneously, the patients in high-risk group presented higher stromal score, immune score, and ESTIMATE score than those in the low-risk group (Figure 6B).
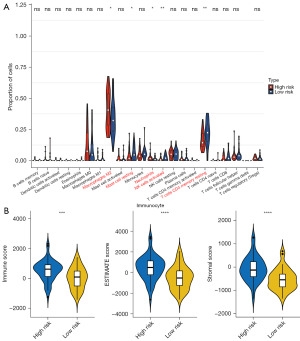
Discussion
The development of imaging and directional biopsy techniques has enabled rapid diagnosis of GBM. However, the current findings on molecular genetic characteristics, including IDH mutation, PTEN mutation, EGFR amplification, and TP53 mutation, have not changed the prognosis of GBM; patients with GBM relapse quickly after surgery and have a very short survival. As molecular genomics develops rapidly, conventional diagnostics and treatments may be improved with the help of specific diagnostic and prognostic biomarkers. As a result, this study investigated the impact of CRGs on the prognosis of GBM patients, and various bioinformatics analyses were used to identify key CRGs with excellent prognostic value in GBM. Subsequently, prognostic risk models for GBM patients were constructed using PLAUR, GP6, C5AR1, SERPINA5, and F2RL2. Besides, the characteristics of TME infiltration in different risk populations were explored, thus providing new ideas for the treatment of patients with GBM.
Malignant tumors can provoke changes in the blood vessels, stroma, and microenvironment when they are genetically altered. All changes in inflammation, angiogenesis, and tissue repair procedures can contribute to the local and systemic activation of the clotting system. BPs of tumor growth, initiation, dormancy, invasion, angiogenesis, metastasis, and therapeutic response are affected by abnormal coagulation function in malignant tumors. A driver mutation in key oncogenes that is synergistic with hypoxia, differentiation, and other factors, alters the expression of TFs and a number of hemostat-associated molecules, including transmembrane receptors such as protease-activating receptors (PAR-1, PAR-2) and clotting factors (FII and FVII) (30).
Evidence suggests that subpopulations of specific malignancies, including GBM, differ in their expression profiles of CRGs (20,21,31). In GBM, the coagulation cascade interacts with endothelial cells and angiogenic activity to drive growth and invasion. A clotting cascade alone cannot explain glioma progression. Throughout the course of GBM, a variety of complex interactions trigger the coagulation cascade, including tumor hypoxia, upregulation of VEGR expression, and increases in both tumor cell-specific TF expression and inducible TF expression in numerous intrinsic regulatory pathways (32). It remains unclear whether the coagulation cascade leads to GBM progression or whether GBM progression leads to hypercoagulability. This study showed that all five prognostic biomarker genes (PLAUR, GP6, C5AR1, SERPINA5, F2RL2) were highly expressed among patients at high risk, which might be involved in the development of GBM through the coagulation pathways. Several studies have reported an association between PLAUR and tumor progression. Using immunohistochemistry and real-time quantitative polymerase chain reaction (qRT-PCR) (33,34), PLAUR expression was very high in GBM, but virtually undetectable in LGG, indicating that PLAUR may be one of the characteristic genes of GBM. A study showed that PLAU and PLAUR expression was positively correlated with invasion, angiogenesis, epithelial-mesenchymal transformation, tumor stem cell-like characteristics, and metastasis in GBM (35). PLAUR activation promotes extracellular protease cascades involved in tumor matrix remodeling and cell migration. It has not been reported whether GP6 is differentially expressed in gliomas. Breast cancer tissue has high levels of GP6, which are significantly higher than those in adjacent tissues (36). A genetic signature associated with clots has been studied in breast cancer, which suggests that GP6 may also influence GBM processes through angiogenesis. CA5R1 is a receptor for complement CA5, and cancer survivors with high expression have a poorer prognosis (37). Based on immunohistochemistry and transcription-level expression validation by RT-PCR, C5AR1 was highly expressed in GBM (38). Complement and coagulation play an Important role in bodily defense. Each plays a different role in physiology and pathophysiology of the systemic circulation, but their functions are interconnected (39). The regulatory role of SERPINA5 in hemostasis and thrombosis has been observed in various organs. However, the expression relationship of SERPINA5 in GBM remains unreported, despite its implication in numerous diseases such as osteoarthritis (40), gastric cancer (41), and thyroid cancer (42). Researchers found that LGG patients with high SERPINA5 expression had a poor prognosis (43). In other words, SERPINA5 is highly expressed in LGG patients, and LGG may progress to GBM. Although it is rare to find a report on coagulation F2RL2 in studies on gliomas, F2RL2 was revealed to be a promising biomarker for glioma in a recent analysis. PAR3 plays a key role in inflammatory reactions and immune responses, as well as tumorigenesis and metastasis in many types of cancer, including gliomas. PAR3 is encoded by F2RL2, which is a G-protein-coupled receptor (GPCR). As a result, we hypothesized that F2RL2 is related to PAR3 through inflammatory processes in primary gliomas (44). In conclusion, there may be a close relation between all five prognostic biomarker genes (PLAUR, GP6, C5AR1, SERPINA5, F2RL2) and the progression of glioma.
The potential value in predicting the prognosis of GBM for these characteristic genes was explored. With ROC analysis, it is suggested that AUCs at 1, 3 and 5 years were all greater than 0.6. Randomly, patients were grouped into high- and low-risk groups according to their median risk scores. Low-risk survivors had longer survival times than high-risk survivors, supporting the validity of the risk model. Moreover, risk models as well as treatment modalities were considered independent predictors of GBM survival. A nomogram for clinical use was also validated.
Based on the results of the GO and KEEG functional enrichment analysis of the 5 prognostic biomarkers, almost all of them were related to blood coagulation. It appears that coagulation plays an important role in GBM progression. In single-cell functional analysis, biomarkers F2RL2, PLAUR, and SERPINA5 were significantly correlated with cell invasion, metastasis, inflammation, repair, and other functions. According to the tumor immune microenvironment analysis, high-risk patients had significantly higher matrix scores, immune scores, as well as ESTIMATE composite scores. As compared to the low-risk group, there were significant differences between the five types of immune cells: macrophages M2, mast cells resting, neutrophils, NK cells activated, and T cells CD4 memory resting. According to these results, the immune microenvironment appears to play a significant role in GBM development. The interaction between the TME, coagulation, inflammation, and immunization plays an important role throughout the tumor life cycle (45).
In the study for GBM, CRGs were independently identified for the first time as having a prognostic effect through the bioinformatic analysis. The relationship between the prognostic signature and immune cell infiltration in GBM was investigated as well. It is essential to observe the characteristics of GBM from an entirely new perspective in order to understand its potential pathogenesis. It has been demonstrated that PLAUR and C5AR1 are involved in glioma cell growth (35,36). The GP6, SERPINA5, and F2RL2 genes need to be studied in more clinical experiments, as well as further mechanistic studies to verify the prognostic utility of the CRGs-based risk model. In the future, we will continue to investigate the prognostic role of these genes, which are essential steps in predicting GBM disease progression and managing treatment. In the future, we will persist in conducting further research on the role of these 5 biomarkers in the pathogenesis of GBM, with the potential to alter the incidence and progression of glioma through these mechanisms.
Conclusions
This study aimed to conduct an analysis of coagulation-related prognostic signatures, resulting in the identification of 5 biomarkers (PLAUR, GP6, C5AR1, SERPINA5, and F2RL2) through the screening process. Additionally, the study explored the potential impact of these signature genes on GBM progression, offering valuable insights that could potentially contribute to the development of molecular targeted therapies for GBM.
Acknowledgments
Funding: This work was supported by grants from
Footnote
Reporting Checklist: The authors have completed the TRIPOD reporting checklist. Available at https://tcr.amegroups.com/article/view/10.21037/tcr-23-322/rc
Peer Review File: Available at https://tcr.amegroups.com/article/view/10.21037/tcr-23-322/prf
Conflicts of Interest: All authors have completed the ICMJE uniform disclosure form (available at https://tcr.amegroups.com/article/view/10.21037/tcr-23-322/coif). The authors have no conflicts of interest to declare.
Ethical Statement: The authors are accountable for all aspects of the work in ensuring that questions related to the accuracy or integrity of any part of the work are appropriately investigated and resolved. This study was conducted in accordance with the Declaration of Helsinki (as revised in 2013).
Open Access Statement: This is an Open Access article distributed in accordance with the Creative Commons Attribution-NonCommercial-NoDerivs 4.0 International License (CC BY-NC-ND 4.0), which permits the non-commercial replication and distribution of the article with the strict proviso that no changes or edits are made and the original work is properly cited (including links to both the formal publication through the relevant DOI and the license). See: https://creativecommons.org/licenses/by-nc-nd/4.0/.
References
- Lapointe S, Perry A, Butowski NA. Primary brain tumours in adults. Lancet 2018;392:432-46. [Crossref] [PubMed]
- Wang D, He MQ, Fan DQ. RARRES1 is a novel immune-related biomarker in GBM. Am J Transl Res 2019;11:5655-63. [PubMed]
- Sturm D, Pfister SM, Jones DTW. Pediatric Gliomas: Current Concepts on Diagnosis, Biology, and Clinical Management. J Clin Oncol 2017;35:2370-7. [Crossref] [PubMed]
- Atif F, Yousuf S, Espinosa-Garcia C, et al. Progesterone Modulates Mitochondrial Functions in Human Glioblastoma Cells. Mol Neurobiol 2021;58:3805-16. [Crossref] [PubMed]
- Kirstein A, Schmid TE, Combs SE. The Role of miRNA for the Treatment of MGMT Unmethylated Glioblastoma Multiforme. Cancers (Basel) 2020;12:1099. [Crossref] [PubMed]
- Li Y, Wu H, Chen G, et al. Arming Anti-EGFRvIII CAR-T With TGFβ Trap Improves Antitumor Efficacy in Glioma Mouse Models. Front Oncol 2020;10:1117. [Crossref] [PubMed]
- Tan AC, Ashley DM, López GY, et al. Management of glioblastoma: State of the art and future directions. CA Cancer J Clin 2020;70:299-312. [Crossref] [PubMed]
- Feng E, Sui C, Wang T, et al. Temozolomide with or without Radiotherapy in Patients with Newly Diagnosed Glioblastoma Multiforme: A Meta-Analysis. Eur Neurol 2017;77:201-10. [Crossref] [PubMed]
- Khorana AA, Soff GA, Kakkar AK, et al. Rivaroxaban for Thromboprophylaxis in High-Risk Ambulatory Patients with Cancer. N Engl J Med 2019;380:720-8. [Crossref] [PubMed]
- Bauer AT, Gorzelanny C, Gebhardt C, et al. Interplay between coagulation and inflammation in cancer: Limitations and therapeutic opportunities. Cancer Treat Rev 2022;102:102322. [Crossref] [PubMed]
- Dirix LY, Oeyen S, Buys A, et al. Coagulation/fibrinolysis and circulating tumor cells in patients with advanced breast cancer. Breast Cancer Res Treat 2022;192:583-91. [Crossref] [PubMed]
- Lazar S, Goldfinger LE. Platelets and extracellular vesicles and their cross talk with cancer. Blood 2021;137:3192-200. [Crossref] [PubMed]
- Navone SE, Guarnaccia L, Locatelli M, et al. Significance and Prognostic Value of The Coagulation Profile in Patients with Glioblastoma: Implications for Personalized Therapy. World Neurosurg 2019;121:e621-9. [Crossref] [PubMed]
- Li M, Wu Y, Zhang J, et al. Prognostic value of pretreatment plasma fibrinogen in patients with colorectal cancer: A systematic review and meta-analysis. Medicine (Baltimore) 2019;98:e16974. [Crossref] [PubMed]
- Zhang Y, Cao J, Deng Y, et al. Pretreatment plasma fibrinogen level as a prognostic biomarker for patients with lung cancer. Clinics (Sao Paulo) 2020;75:e993. [Crossref] [PubMed]
- Meli R, Raso GM, Cicala C, et al. Thrombin and PAR-1 activating peptide increase iNOS expression in cytokine-stimulated C6 glioma cells. J Neurochem 2001;79:556-63. [Crossref] [PubMed]
- Melnichnikova O, Zhilenkova Y, Sirotkina O, et al. Circulating Small Extracellular Vesicles Profiling and Thrombin Generation as Potential Markers of Thrombotic Risk in Glioma Patients. Front Cardiovasc Med 2022;9:789937. [Crossref] [PubMed]
- Wojtukiewicz MZ, Mysliwiec M, Matuszewska E, et al. Imbalance in Coagulation/Fibrinolysis Inhibitors Resulting in Extravascular Thrombin Generation in Gliomas of Varying Levels of Malignancy. Biomolecules 2021;11:663. [Crossref] [PubMed]
- Campanella R, Guarnaccia L, Cordiglieri C, et al. Tumor-Educated Platelets and Angiogenesis in Glioblastoma: Another Brick in the Wall for Novel Prognostic and Targetable Biomarkers, Changing the Vision from a Localized Tumor to a Systemic Pathology. Cells 2020;9:294. [Crossref] [PubMed]
- Tripathy S, Singh S, Banerjee M, et al. Coagulation proteases and neurotransmitters in pathogenicity of glioblastoma multiforme. Int J Neurosci 2022; Epub ahead of print. [Crossref] [PubMed]
- He Q, Yang J, Jin Y. Immune infiltration and clinical significance analyses of the coagulation-related genes in hepatocellular carcinoma. Brief Bioinform 2022;23:bbac291. [Crossref] [PubMed]
- Wu Z, Lu Z, Li L, et al. Identification and Validation of Ferroptosis-Related LncRNA Signatures as a Novel Prognostic Model for Colon Cancer. Front Immunol 2022;12:783362. [Crossref] [PubMed]
- Zhang MY, Huo C, Liu JY, et al. Identification of a Five Autophagy Subtype-Related Gene Expression Pattern for Improving the Prognosis of Lung Adenocarcinoma. Front Cell Dev Biol 2021;9:756911. [Crossref] [PubMed]
- Zhou RS, Zhang EX, Sun QF, et al. Integrated analysis of lncRNA-miRNA-mRNA ceRNA network in squamous cell carcinoma of tongue. BMC Cancer 2019;19:779. [Crossref] [PubMed]
- Li R, Yin YH, Ji XL, et al. Pan-Cancer Prognostic, Immunity, Stemness, and Anticancer Drug Sensitivity Characterization of N6-Methyladenosine RNA Modification Regulators in Human Cancers. Front Mol Biosci 2021;8:644620. [Crossref] [PubMed]
- Zhou Y, Zhou B, Pache L, et al. Metascape provides a biologist-oriented resource for the analysis of systems-level datasets. Nat Commun 2019;10:1523. [Crossref] [PubMed]
- Yuan H, Yan M, Zhang G, et al. CancerSEA: a cancer single-cell state atlas. Nucleic Acids Res 2019;47:D900-8. [Crossref] [PubMed]
- Lin X, Zhou T, Hu S, et al. Prognostic significance of pyroptosis-related factors in lung adenocarcinoma. J Thorac Dis 2022;14:654-67. [Crossref] [PubMed]
- Tong Y, Peng M, Li J, et al. Comprehensive Analyses of Stromal-Immune Score-Related Competing Endogenous RNA Networks In Colon Adenocarcinoma. Dis Markers 2022;2022:4235305. [Crossref] [PubMed]
- Wojtukiewicz MZ, Hempel D, Sierko E, et al. Endothelial Protein C Receptor (EPCR), Protease Activated Receptor-1 (PAR-1) and Their Interplay in Cancer Growth and Metastatic Dissemination. Cancers (Basel) 2019;11:51. [Crossref] [PubMed]
- Mandoj C, Tomao L, Conti L. Coagulation in Brain Tumors: Biological Basis and Clinical Implications. Front Neurol 2019;10:181. [Crossref] [PubMed]
- Wojtukiewicz MZ, Mysliwiec M, Matuszewska E, et al. Heterogeneous Expression of Proangiogenic and Coagulation Proteins in Gliomas of Different Histopathological Grade. Pathol Oncol Res 2021;27:605017. [Crossref] [PubMed]
- Wang LJ, Lv P, Lou Y. A Novel TAF-Related Signature Based on ECM Remodeling Genes Predicts Glioma Prognosis. Front Oncol 2022;12:862723. [Crossref] [PubMed]
- Tan YQ, Li YT, Yan TF, et al. Six Immune Associated Genes Construct Prognostic Model Evaluate Low-Grade Glioma. Front Immunol 2020;11:606164. [Crossref] [PubMed]
- Li J, Fan H, Zhou X, et al. Prognostic Significance and Gene Co-Expression Network of PLAU and PLAUR in Gliomas. Front Oncol 2022;11:602321. [Crossref] [PubMed]
- Guo L, Jing Y. Construction and Identification of a Novel 5-Gene Signature for Predicting the Prognosis in Breast Cancer. Front Med (Lausanne) 2021;8:669931. [Crossref] [PubMed]
- Qiu H, Li Y, Cheng S, et al. A Prognostic Microenvironment-Related Immune Signature via ESTIMATE (PROMISE Model) Predicts Overall Survival of Patients With Glioma. Front Oncol 2020;10:580263. [Crossref] [PubMed]
- Ye S, Yang B, Zhang T, et al. Identification of an Immune-Related Prognostic Signature for Glioblastoma by Comprehensive Bioinformatics and Experimental Analyses. Cells 2022;11:3000. [Crossref] [PubMed]
- Berkowitz S, Chapman J, Dori A, et al. Complement and Coagulation System Crosstalk in Synaptic and Neural Conduction in the Central and Peripheral Nervous Systems. Biomedicines 2021;9:1950. [Crossref] [PubMed]
- Zhu T, Tan Q, Xin X, et al. Proteomic analysis of human articular cartilage unravels the dyscoagulation in osteoarthritis and the potential value of serpinA5 as a biomarker for osteoarthritis. Proteomics Clin Appl 2022;16:e2100117. [Crossref] [PubMed]
- Fan M, Xiong X, Han L, et al. SERPINA5 promotes tumour cell proliferation by modulating the PI3K/AKT/mTOR signalling pathway in gastric cancer. J Cell Mol Med 2022;26:4837-46. [Crossref] [PubMed]
- Moshtaghioun SM, Fazel-Yazdi N, Mandegari M, et al. Evaluation the Presence of SERPINA5 (Exon 3) and FTO rs9939609 Polymorphisms in Papillary Thyroid Cancer Patients. Asian Pac J Cancer Prev 2021;22:3641-6. [Crossref] [PubMed]
- Zeng WJ, Yang YL, Wen ZP, et al. Identification of gene expression and DNA methylation of SERPINA5 and TIMP1 as novel prognostic markers in lower-grade gliomas. PeerJ 2020;8:e9262. [Crossref] [PubMed]
- Lvu W, Fei X, Chen C, et al. In silico identification of the prognostic biomarkers and therapeutic targets associated with cancer stem cell characteristics of glioma. Biosci Rep 2020;40:BSR20201037. [Crossref] [PubMed]
- Ruf W, Graf C. Coagulation signaling and cancer immunotherapy. Thromb Res 2020;191:S106-11. [Crossref] [PubMed]