Genes associated with inflammation for prognosis prediction for clear cell renal cell carcinoma: a multi-database analysis
Introduction
The association between inflammatory responses and tumours was first proposed in the 19th century (1). Since then, many studies on the relationship between inflammatory responses and tumours have been carried out. As early as 2002, an article from Nature related the origin of many tumours to the site of infection, especially with chronic inflammation (2). When the body is infected and inflammation occurs, tissue loss and repair occur, and this process greatly increases the possibility of tumorigenesis (3). More than 15% of malignant tumours are directly related to infections (4). For example, the development of bladder cancer is strongly associated with schistosomiasis infection. As a result, new treatments for bladder cancer have been developed (1). Further studies have found that the inflammatory response is involved in various stages of tumorigenesis, but the relationship between inflammation-related genes and the prognosis of clear cell renal cell carcinoma (ccRCC) patients is poorly understood (5). With the increase in the number of studies on inflammatory responses, various inflammatory cells and factors in the tumour microenvironment can be used as important prognostic indicators for tumour patients (6). Some indicator combinations are considered more valuable, such as the systemic immune-inflammation index (SII), neutrophil-to-lymphocyte ratio (NLR), platelet-to-lymphocyte ratio (PLR), or lymphocyte-to-monocytes ratio (LMR) (7-9). A study of 440,000 samples found that higher SII, NLR, and PLR scores were associated with a greater risk of kidney cancer, while the LMR showed the opposite (10).
Inflammatory cells and inflammatory factors each play important roles in the disease progression of ccRCC. A large number of studies have focused on macrophages, lymphocytes, and interleukins (11-13). Tumour-associated macrophages are very active in the tumour microenvironment (14), which in different microenvironments, can differentiate into two phenotypes, M1 and M2, among which the M2 phenotype is considered to be closely related to tumorigenesis (15,16). ccRCC can promote the polarisation of M2 macrophages through exosomes, and polarised M2 macrophages can further promote the progression of ccRCC (14). However, a higher dendritic cell number and macrophage infiltration in patients with ccRCC can show a worse prognosis (17). The expression level of FCER1G combined with the expression of CD68 is expected to be the prognostic index of patients with ccRCC after surgery (18). LAPTM4B and TRAF2 can promote the polarisation of M2 macrophages through autophagy-dependent pathways, which in turn affects the prognosis of ccRCC patients (19,20). CCL5 can block epithelial-mesenchymal transformation in ccRCC cells via the PI3K/AKT pathway. In addition, extensive invasion of CCL5+ tumour-associated macrophages often indicates a poor prognosis in patients with ccRCC (21). RBM15 is likewise found to affect patient survival by promoting the polarisation of the macrophage M2 phenotype (22). Patients with different macrophage phenotypes also have different sensitivities for receiving sunitinib (23), those with high cathepsin Z expression are better served with anti-programmed cell death protein 1 (PD-1) immunotherapy (24).
Lymphocyte and neutrophil infiltration in the tumour microenvironment can also help predict the prognosis and treatment of patients with ccRCC. Intratumoural CXCL13+CD8+ T cell infiltration in patients with ccRCC often indicates a poor prognosis (25). In addition, lower T cells and higher neutrophil infiltration often lead to tumour recurrence and metastasis (26). Therefore, we can predict the prognosis of patients based on the ratio of neutrophils to lymphocytes in tumour tissue (27). Regulatory T cell (Treg) is thought to act as an inhibitor of the anti-tumour immune response (28). Infiltration of activated CD8+ cells suggests a better prognosis (12). TNFRSF9+CD8+ T cells can also predict the prognosis of kidney cancer (29). CD103+ lymphocytes are an indicator of poor prognosis in patients with ccRCC, and CD103+ lymphocytes usually accumulate in metastases in the lungs (30). In contrast, CD45RO+CD8+ T cells can delay the progression of ccRCC through various pathways (31). However, the antitumor activity of T cells may be limited by TGFβ1 (32). CD8+ T cell infiltration can also affect the prognosis of tumour cell PD-L1 (TC-PD-L1)-positive ccRCC; thus, patients with a large number of CD8+ T cell infiltrates are at higher risk of ccRCC recurrence and death (33). IL-8 is associated with the stem-like properties of ccRCC (34). While IL-23 is expected to provide a new therapeutic target for renal cell carcinoma (35), high IL-6 expression suggests a worse prognosis (13).
Therefore, there is a close relationship between the inflammatory response and tumour as a variety of inflammatory indicators are related to the prognosis of ccRCC. However, to the best of our knowledge, there has been no research on the relationship between genes related to inflammatory response and ccRCC. With the development of genetic testing technology, we have been able to obtain the expression of inflammation-related genes, available from public databases for analysis. In this study, we combined this data with bioinformatics to determine the relationship between genes related to inflammatory response and ccRCC and develop a prognostic model. On this basis, a more detailed analysis was carried out to facilitate personalized treatment development for ccRCC. We present this article in accordance with the TRIPOD reporting checklist (available at https://tcr.amegroups.com/article/view/10.21037/tcr-23-1183/rc).
Methods
Data screening
All relevant data for this study are freely available through The Cancer Genome Atlas (TCGA), International Cancer Genome Consortium (ICGC), Gene Expression Omnibus (GEO), Human Protein Atlas (HPA) databases and Internet public sources. The genes associated with inflammation were retrieved from The Human Gene Database. The study was conducted in accordance with the Declaration of Helsinki (as revised in 2013). The data used to build the model corresponding to the ccRCC cohort and GSE29609 dataset were from the TCGA and GEO databases, respectively. In addition, ICGC data were used for external verification. To prove the applicability of the model, we added the E-MTAB-1980 cohort to double validate externally of the model. The ccRCC transcriptome data in the TCGA database was obtained through the TCGAbiolinks software package. ICGC data and E-MTAB-1980 cohort data were obtained directly from the website. In addition, ‘ccRCC’ was used to search the GEO database and a dataset with complete survival information and a sample size of not less than 30 was selected for the model construction. TCGA data, ICGC data, GSE29609 dataset, and data with incomplete survival information in the E-MTAB-1980 cohort were excluded. The GSE40435 and GSE53757 datasets from the GEO database were used to verify the differential expression of model-related genes. We searched the HPA database for immunohistochemistry of model genes using the HPAanalyze package. Immunohistochemical images were obtained from the HPA database.
Preliminary gene screening and data standardization
We downloaded a total of 15,631 genes related to inflammation from The Human Gene Database. We obtained 3,300 genes through differential analysis that were differentially expressed in normal tissue and ccRCC. To make the data comparable, we first standardised the data using the surrogate variable analysis (SVA) R package. After normalisation, we removed the samples with incomplete survival information and obtained a total of 759 standardised samples with complete survival information, including 567 samples from the TCGA and GSE29609 datasets. This part of the sample was used to build the model and validate it internally. A total of 91 cases were sourced from the ICGC database. The data were used for the external validation of the model. In addition, the E-MTAB-1980 cohort had a total of 101 samples. The data from the E-MTAB-1980 cohort were used for additional external validation. Through further analysis of the standardised samples, we obtained 1,353 prognostically relevant differential genes. These preliminary screened data were used to build the inflammation-related gene model (IRGM).
IRGM building and external data validation
We increased the number of samples to make our findings more reliable by combining the ccRCC data in the TCGA database with that from the GSE29609 dataset into one cohort. The patients with ccRCC were randomly divided into M and T cohorts using a 1:1 ratio with the createDataPartition function in the CARET package. The patients from the M cohort were used to construct the IRGM. We obtained the genes that were best used to build the model using a least absolute shrinkage and selection operator (LASSO) regression and cross-validation. After obtaining the formula for the IRGM, each ccRCC sample received an IRGM score. All normalised samples were divided into high-risk (HR) and low-risk (LR) groups based on the median sample score in the M cohort. Patients with IRGM scores above the median were classified into the HR group, and patients with scores below median were classified into the LR group. Tumour samples from the T cohort were used internally to verify the accuracy of the IRGM predictions. Next, the ICGC and E-MTAB-1980 cohort samples with ccRCC provided a dual external validation. Specifically, the survival and ROC curves were drawn to display the verification results.
Differential expression of IRGM signatures (IRGMS) verification and query immunohistochemistry
Through the construction of IRGM and comprehensive verification, we confirmed that the genes related to the inflammatory response have a strong predictive ability for the prognosis of ccRCC. If the expression of model-related genes in ccRCC and normal kidney tissues was different, their important role was further confirmed. Therefore, we retrieved datasets from tumours as well as normal samples from the GEO database for validation and obtained the GSE40435 (N=101, T=101) and GSE53757 (N=72, T=72) datasets. The two datasets were used separately to validate the conclusions. In addition, immunohistochemical validation was used to characterise the genes using data from the HPA database.
Integration of common clinical indicators, nomogram construction and verification of its predictive efficacy
Although the IRGM’s ability to predict the prognosis of ccRCC is good, it still has certain limitations for daily use as it does not accurately predict patient survival. Nomograms have an excellent ability to accurately predict patient survival and guide clinical work. Combining the predictive power of IRGM and the function of nomograms, we constructed a nomogram for clinical guidance that uses common clinical information as indicators. Calibration and decision curve analysis (DCA) were used to verify the predictive power of nomograms. Further, the area under the receiver operating characteristic (ROC) curve (AUC) recognised the ability of the nomogram to predict ccRCC prognosis.
Pathway enrichment analysis
Next, we analysed the pathway enrichment of patients with different IRGM scores through multiple pathway enrichment assays.
Immune-related analysis
Immunotherapy has played a great role in the treatment of various tumours. Because research on immunotherapy for ccRCC started late, treatment options are limited for patients with advanced ccRCC. Therefore, immunotherapy for ccRCC has great research value. For different IRGM scores in patients with ccRCC, we performed immune-related analyses to develop personalised treatment plans for patients with different IRGM scores.
Drug susceptibility analysis
Although chemotherapy is the classic treatment for ccRCC, the sensitivity of drugs varies greatly amongst patients. Moreover, the side effects of chemotherapy drugs are considerable. Therefore, it is very important to choose fewer and more effective drugs for patients with ccRCC. In this study, we used the oncoPredict package for drug susceptibility analysis to inform the selection of clinical chemotherapy drugs.
Subtype identification of ccRCC
In previous studies, we demonstrated that IRGMS can predict the prognosis of ccRCC. On this basis, we classified the samples into types by the expression of IRGMS. We analysed the relationship between different types and IRGM groupings using the Sankey plots. In addition, we clarified the value of type classification by conducting survival analysis of different types. Finally, the expression of immune checkpoint-related genes in different types was used to understand the therapeutic effect of immune checkpoint inhibitors.
Statistical analyses
All statistical analyses in this study were performed using R software (version 4.2.2; The R Project for Statistical Computing, Vienna, Austria). Unless otherwise stated, a P<0.05 was set as the significance value in this study. Also, in all pictures: *, P<0.05; **, P<0.01; ***, P<0.001.
Results
Research process and preliminary data processing results
The flowchart of this study is shown in Figure 1. First, we obtained 15,631 genes related to inflammation from the databases and obtained differential genes related to inflammatory response. We selected some genes to display through heat maps as well as volcano maps (Figure 2A,2B).
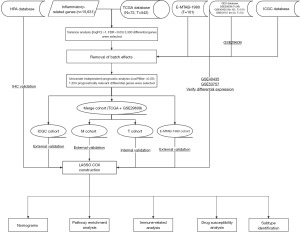
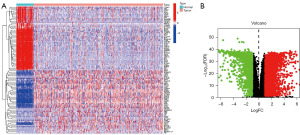
IRGM can stably predict patient prognosis in different datasets
After LASSO regression and cross-validation, we identified ten IRGMS (IGFBP3, SCNN1B, IFI16, LRRC19, GSTM3, IFI44, APOLD1, HPGD, CPA3, and PROM1), and obtained a model formula: IRGM score = EXP[(IGFBP3* − 0.512599540192771) + (SCNN1B* − 0.382216902501755) + (IFI16* − 0.41271812853661) + (LRRC19* − 0.148895552969845) + (GSTM3* − 0.319391421782252) + (IFI44* − 0.34161975189148) + (APOLD1* − 0.234401991849677) + (HPGD* − 0.28831346490327) + (CPA3* − 0.226306954924149) + (PROM1* −0.105416861915745)] (Figure 3A,3B). From the principal components analysis (PCA), the inflammation-related genes alone could not distinguish high IRGM scores from patients with low IRGM scores (Figure 3C). However, the 10 identified IRGMS made a good distinction between patients with different IRGM scores (Figure 3D). The ROC curves of the M and T, ICGC, and E-MTAB-1980 cohorts were all greater than 0.65 (Figure 3E-3H), which corresponded to the queue for modelling, internal validation, and double external validations of the model for ICGC cohort and E-MTAB-1980, respectively. Under such rigorous validation, we demonstrated that the model had the ability to predict the prognosis of patients from different sources. In addition, patients in the HR group always showed lower survival times than patients in the LR group (Figure 3I-3L). This suggested that patients with high IRGM scores have a worse prognosis. That is, IRGMS (IGFBP3, SCNN1B, IFI16, LRRC19, GSTM3, IFI44, APOLD1, HPGD, CPA3, and PROM1) is valuable in predicting the prognosis of renal clear cell carcinoma. From the above results, we can conclude that IRGM can stably predict the prognosis of patients with ccRCC in different datasets.
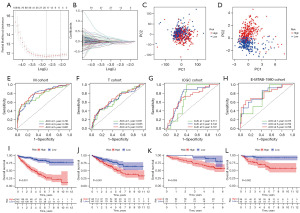
Differential expression and immunohistochemical validation of IRGMS
In a previous analysis, we screened ten IRGMS and further verified their significance. From the analysis of ten IRGMS (IGFBP3, SCNN1B, IFI16, LRRC19, GSTM3, IFI44, APOLD1, HPGD, CPA3, and PROM1) copy data, we found that the copy number of CPA3, IFI16, IGFBP3, LRRC19, PROM1, and SCNN1B increased, and the copy number of IFI44, HPGD, APOLD1, and GSTM3 decreased (Figure 4A). In addition, circle map showed that IFI44, GSTM3, and IFI16 are located on chromosome 1, CPA3 is located on chromosome 3, PROM1 and HPGD are located on chromosome 4, IGFBP3 is located on chromosome 7, LRRC19 is located on chromosome 9, APOLD1 is located on chromosome 12, and SCNN1B is located on chromosome 16 (Figure 4B). In the mutation data analysis results, we only found that SCNN1B had mutations (Figure 4C). For the ten IRGMS screened, the TCGA database analysis showed that CPA3, APOLD1, IGFBP3, IFI44, and IFI16 were highly expressed in tumour tissues, while HPGD, SCNN1B, GSTM3, LRRC19, and PROM1 were lowly expressed in renal clear cell carcinoma (Figure 4D). This was verified in the GSE40435 and GSE53757 datasets (Figure 4E,4F). However, through the HPA database, we only queried the immunohistochemistry of eight IRGMS (SCNN1B, IFI16, GSTM3, IFI44, APOLD1, HPGD, CPA3, and PROM1). Therefore, the results of the HPA database query were in line with our conclusions (Figure 4G).
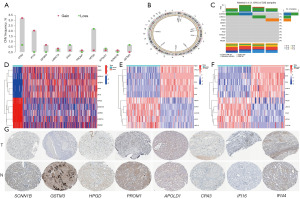
Excellent ability of the nomogram to predict patient survival
The nomogram constructively predicted that the 5-year survival rate of the sixth patient with ccRCC in the M cohort was 0.847 (Figure 5A). The calibration curve showed that the predicted results of the nomogram were almost identical to the actual results (Figure 5B). The AUC of the nomogram was 0.845, which fully demonstrated the prediction efficiency of the nomogram (Figure 5C). The DCA decision curve also highlighted the predictive power of the nomogram (Figure 5D). Both univariate and multivariate independent prognostic analyses showed that the nomogram had the ability to predict survival in patients with ccRCC independently of other factors (Figure 5E,5F).
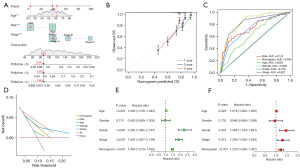
Pathway enrichment analysis of patients with different IRGM scores
First, the Kyoto Encyclopaedia of Genes and Genomes (KEGG) analysis showed that the pathways enriched by patients in the LR group were mainly related to compound metabolism and neurological activity. The pathways enriched in the HR group were related to diabetes, graft-versus-host response, and coagulation function (Figure 6A). The Gene Ontology (GO) enrichment analysis demonstrated that the ontology enriched in patients in the LR group had a molecular function and cellular component. On the contrary, patients in the HR group were closely related to the biological process. Patients in the HR group presented acute inflammatory response, antimicrobial tumour response, humoral immune response, and other biological process pathways closely related to tumours (Figure 6B).
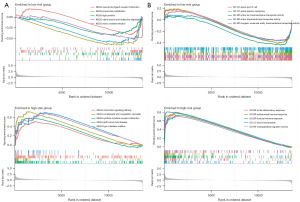
Patients with high IRGM scores benefit more from immunotherapy
Immune cells and immune-related functions of patients with ccRCC with different IRGM scores were enriched via single-sample gene set enrichment analysis (ssGSEA) enrichment analysis. Approximately 75% of immune cells showed differences in patients with different IRGM scores. Among them, 62.5% of the cells were more infiltrated in the HR group, and only 12.5% of the immune cells were more infiltrated in the LR group (Figure 7A). In the analysis of immune-related functions, 92% of immune-related functions had significant differences between patients with different IRGM scores. Further, all immune-related functions were more relevant in patients in the HR group (Figure 7B). Immune checkpoint inhibitors may thus offer new hope for patients with advanced ccRCC. We also observed that 36 immune checkpoint-related genes were significantly different in both the HR and LR groups, while 30 more genes were expressed in the HR group than in the LR group (Figure 7C). This provides valuable directions to inform immunotherapy regimens.
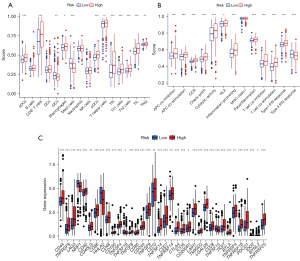
Chemotherapy drug susceptibility
The HR group was more sensitive to most chemotherapy drugs, including 5-fluorouracil, AZ960, AZD7762, bortezomib, buparlisib, dasatinib, pictilisib, PRT062607, taselisib, XAV939. AZD3759, BI-2536, erlotinib, ibrutinib, and osimertinib were more effective in patients in the LR group (Figure 8).
Subtype identification
Using matrix plots, delta area plots, consistency cumulative distribution function plots, and tracking plot as criteria, we divided the samples of patients with ccRCC into three subtypes (Figure 9A-9D). In the Sankey chart, we observed that patients with the C1 subtype were almost evenly distributed in the HR and LR groups, patients with the C2 subtype were almost all distributed in the HR group, while patients with the C3 subtype were distributed in both HR and LR groups (Figure 9E). In the t-distributed stochastic neighbour embedding (tSNE) plot, we observed well-distinguished subtypes (Figure 9F,9G). The survival analysis results of the three subtypes were significantly different; the C1 subtype had the best prognosis whereas the C2 subtype had the worst prognosis (Figure 9H). Further research is warranted to better clarify these findings.
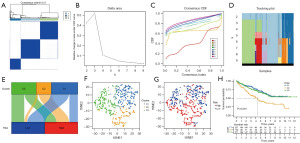
Discussion
We constructed a prognostic model containing ten IRGMS and verified it with an internal and two sets of external data. The validation showed that the model can stably predict the prognosis of patients in various datasets. Subsequently, the differential expression of IRGMS and immunohistochemistry were also verified. However, we could not validate the model with local samples, which could be improved using adequate external data validation. After completing the model construction, we developed nomograms and performed pathway enrichment analysis, immunocorrelation analysis, drug susceptibility analysis, and subtype identification. Nomograms have been used to determine the survival rate of patients. The pathway enrichment analysis showed that ccRCC was closely related to inflammatory responses. Immune-related analyses can identify patients who have high IRGM scores and are better candidates for immunotherapy. The results of the drug susceptibility analysis showed that patients in the HR group had more options when choosing chemotherapy drugs. The subtype identification allows us to develop more personalised treatment plans for patients with ccRCC. Therefore, IRGMS (IGFBP3, SCNN1B, IFI16, LRRC19, GSTM3, IFI44, APOLD1, HPGD, CPA3, and PROM1) are valuable in predicting the prognosis of patients with ccRCC and guiding treatment.
In humans, the main function of APOLD1 is to encode apolipoprotein, which has an important role in regulating vascular function (36). APOLD1 plays an important role in inflammatory response-related diseases, such as diabetic nephropathy and osteoarthritis (36,37). Similarly, this gene can predict the prognosis of patients with ccRCC (38). CPA3 is associated with histone deacetylase activation (39). This gene has played a remarkable role in predicting the prognosis of breast, skin, lung, adenocarcinoma, colorectal, and ovarian cancers (40-44). As a member of the glutathione-S-transferase (GST) family, GSTM3 can regulate tumour susceptibility (45). GSTM3 is associated with the risk of kidney cancer (46). A study of 329 cases of ccRCC and 420 healthy controls found that GSTM3 has an inhibitory effect on ccRCC (46). Similarly, GSTM3 plays an important role in glioma, pancreatic cancer, and oesophageal cancer (47-50). Overall, these studies also confirm the value of our study. HPGD undertakes a wide range of functions in the body and the proteins it encodes are distributed throughout the body (51). In addition, HPGD is associated with a variety of tumours (52-54). The effects of IFI16 on various tumours are different. IFI16 can accelerate the progression of cervical cancer through the STING-TBK1-NF-κB pathway (55). However, in triple-negative breast cancer, IFI16 has an anti-tumour effect (56). In addition, ARPC1B can promote radiotherapy resistance by blocking the degradation of IFI16 in glioma stem cells (57). IFI44 is an important protein-coding gene in the human body that can influence the formation of microtubule structures (58), while it can be a prognostic marker for osteosarcoma as well as head and neck cancer (59,60). In addition, IFI44 in small-cell lung cancer can lead to gefitinib resistance (61). As a member of the family of insulin-like growth factor (IGF)-binding proteins, IGFBP3 does not have the same effects on various tumours (62), including ccRCC, when it is highly expressed in breast cancer, pancreatic cancer, and squamous cell carcinoma of the head and neck (63-65). Overexpression of IGFBP3 is also associated with a poor prognosis for ccRCC, which is consistent with the conclusions of this study. Nonetheless, cyclovirobuxine can inhibit IGFBP3 and thus the deterioration of ccRCC (66). In addition, IGFBP3 expression in prostate cancer tends to promote the progression of the disease (67). However, in lung cancer, IGFBP3 can improve the survival rate of patients and delay the invasion of tumour cells (68). LRRC19 is a transmembrane receptor in the LRR family (69). The protein product of LRRC19 is specifically expressed in the kidney, spleen, and intestine and is involved in local inflammatory responses (70). In addition, LRRC19 is associated with the prognosis and adjuvant treatment of ccRCC (71,72). As a member of the prominin family, PROM1 is often considered a biomarker for cancer stem cells (73). CD133 is downregulated in ccRCC as an encoding product of PROM1 (74). Moreover, PROM1 can be used as a prognostic marker in liver cancer and ovarian cancer (74,75), while the function of SCNN1B is to encode the formation of partial epithelial sodium channels (76). Lastly, SCNN1B can inhibit colorectal cancer in various ways (77).
Conclusions
IRGMS (IGFBP3, SCNN1B, IFI16, LRRC19, GSTM3, IFI44, APOLD1, HPGD, CPA3, and PROM1) are valuable to the prognosis of ccRCC. Patients with higher IRGM scores may be better candidates for treatment with immune checkpoint inhibitors and can have more chemotherapy options.
Acknowledgments
We want to thank the data contributors and curators of the TCGA, ICGC, GEO, HPA databases.
Funding: None.
Footnote
Reporting Checklist: The authors have completed the TRIPOD reporting checklist. Available at https://tcr.amegroups.com/article/view/10.21037/tcr-23-1183/rc
Peer Review File: Available at https://tcr.amegroups.com/article/view/10.21037/tcr-23-1183/prf
Conflicts of Interest: All authors have completed the ICMJE uniform disclosure form (available at https://tcr.amegroups.com/article/view/10.21037/tcr-23-1183/coif). The authors have no conflicts of interest to declare.
Ethical Statement: The authors are accountable for all aspects of the work in ensuring that questions related to the accuracy or integrity of any part of the work are appropriately investigated and resolved. The study was conducted in accordance with the Declaration of Helsinki (as revised in 2013).
Open Access Statement: This is an Open Access article distributed in accordance with the Creative Commons Attribution-NonCommercial-NoDerivs 4.0 International License (CC BY-NC-ND 4.0), which permits the non-commercial replication and distribution of the article with the strict proviso that no changes or edits are made and the original work is properly cited (including links to both the formal publication through the relevant DOI and the license). See: https://creativecommons.org/licenses/by-nc-nd/4.0/.
References
- Grivennikov SI, Greten FR, Karin M. Immunity, inflammation, and cancer. Cell 2010;140:883-99. [Crossref] [PubMed]
- Coussens LM, Werb Z. Inflammation and cancer. Nature 2002;420:860-7. [Crossref] [PubMed]
- Colotta F, Allavena P, Sica A, et al. Cancer-related inflammation, the seventh hallmark of cancer: links to genetic instability. Carcinogenesis 2009;30:1073-81. [Crossref] [PubMed]
- Kuper H, Adami HO, Trichopoulos D. Infections as a major preventable cause of human cancer. J Intern Med 2000;248:171-83. [Crossref] [PubMed]
- Hanahan D, Weinberg RA. Hallmarks of cancer: the next generation. Cell 2011;144:646-74. [Crossref] [PubMed]
- Diakos CI, Charles KA, McMillan DC, et al. Cancer-related inflammation and treatment effectiveness. Lancet Oncol 2014;15:e493-503. [Crossref] [PubMed]
- Geng Y, Shao Y, Zhu D, et al. Systemic Immune-Inflammation Index Predicts Prognosis of Patients with Esophageal Squamous Cell Carcinoma: A Propensity Score-matched Analysis. Sci Rep 2016;6:39482. [Crossref] [PubMed]
- Passardi A, Scarpi E, Cavanna L, et al. Inflammatory indexes as predictors of prognosis and bevacizumab efficacy in patients with metastatic colorectal cancer. Oncotarget 2016;7:33210-9. [Crossref] [PubMed]
- Huang H, Liu Q, Zhu L, et al. Prognostic Value of Preoperative Systemic Immune-Inflammation Index in Patients with Cervical Cancer. Sci Rep 2019;9:3284. [Crossref] [PubMed]
- Nøst TH, Alcala K, Urbarova I, et al. Systemic inflammation markers and cancer incidence in the UK Biobank. Eur J Epidemiol 2021;36:841-8. [Crossref] [PubMed]
- Chakiryan NH, Hajiran A, Kim Y, et al. Correlating Immune Cell Infiltration Patterns with Recurrent Somatic Mutations in Advanced Clear Cell Renal Cell Carcinoma. Eur Urol Focus 2022;8:784-93. [Crossref] [PubMed]
- Hu J, Chen Z, Bao L, et al. Single-Cell Transcriptome Analysis Reveals Intratumoral Heterogeneity in ccRCC, which Results in Different Clinical Outcomes. Mol Ther 2020;28:1658-72. [Crossref] [PubMed]
- Kays JK, Koniaris LG, Cooper CA, et al. The Combination of Low Skeletal Muscle Mass and High Tumor Interleukin-6 Associates with Decreased Survival in Clear Cell Renal Cell Carcinoma. Cancers (Basel) 2020;12:1605. [Crossref] [PubMed]
- Shen T, Miao S, Zhou Y, et al. Exosomal AP000439.2 from clear cell renal cell carcinoma induces M2 macrophage polarization to promote tumor progression through activation of STAT3. Cell Commun Signal 2022;20:152. [Crossref] [PubMed]
- Di Conza G, Tsai CH, Gallart-Ayala H, et al. Tumor-induced reshuffling of lipid composition on the endoplasmic reticulum membrane sustains macrophage survival and pro-tumorigenic activity. Nat Immunol 2021;22:1403-15. [Crossref] [PubMed]
- Goswami KK, Ghosh T, Ghosh S, et al. Tumor promoting role of anti-tumor macrophages in tumor microenvironment. Cell Immunol 2017;316:1-10. [Crossref] [PubMed]
- Brech D, Herbstritt AS, Diederich S, et al. Dendritic Cells or Macrophages? The Microenvironment of Human Clear Cell Renal Cell Carcinoma Imprints a Mosaic Myeloid Subtype Associated with Patient Survival. Cells 2022;11:3289. [Crossref] [PubMed]
- Dong K, Chen W, Pan X, et al. FCER1G positively relates to macrophage infiltration in clear cell renal cell carcinoma and contributes to unfavorable prognosis by regulating tumor immunity. BMC Cancer 2022;22:140. [Crossref] [PubMed]
- Xu Y, Li L, Yang W, et al. TRAF2 promotes M2-polarized tumor-associated macrophage infiltration, angiogenesis and cancer progression by inhibiting autophagy in clear cell renal cell carcinoma. J Exp Clin Cancer Res 2023;42:159. [Crossref] [PubMed]
- Wang H, Wang Q, Wu Y, et al. Autophagy-related gene LAPTM4B promotes the progression of renal clear cell carcinoma and is associated with immunity. Front Pharmacol 2023;14:1118217. [Crossref] [PubMed]
- Liu Q, Zhao E, Geng B, et al. Tumor-associated macrophage-derived exosomes transmitting miR-193a-5p promote the progression of renal cell carcinoma via TIMP2-dependent vasculogenic mimicry. Cell Death Dis 2022;13:382. [Crossref] [PubMed]
- Zeng X, Chen K, Li L, et al. Epigenetic activation of RBM15 promotes clear cell renal cell carcinoma growth, metastasis and macrophage infiltration by regulating the m6A modification of CXCL11. Free Radic Biol Med 2022;184:135-47. [Crossref] [PubMed]
- Zhang D, Ni Y, Wang Y, et al. Spatial heterogeneity of tumor microenvironment influences the prognosis of clear cell renal cell carcinoma. J Transl Med 2023;21:489. [Crossref] [PubMed]
- Zhang F, Liang J, Lu Y, et al. Macrophage-Specific Cathepsin as a Marker Correlated with Prognosis and Tumor Microenvironmental Characteristics of Clear Cell Renal Cell Carcinoma. J Inflamm Res 2022;15:6275-92. [Crossref] [PubMed]
- Dai S, Zeng H, Liu Z, et al. Intratumoral CXCL13(+)CD8(+)T cell infiltration determines poor clinical outcomes and immunoevasive contexture in patients with clear cell renal cell carcinoma. J Immunother Cancer 2021;9:e001823. [Crossref] [PubMed]
- Ghatalia P, Gordetsky J, Kuo F, et al. Prognostic impact of immune gene expression signature and tumor infiltrating immune cells in localized clear cell renal cell carcinoma. J Immunother Cancer 2019;7:139. [Crossref] [PubMed]
- Cordeiro MD, Ilario EN, Abe DK, et al. Neutrophil-to-Lymphocyte Ratio Predicts Cancer Outcome in Locally Advanced Clear Renal Cell Carcinoma. Clin Genitourin Cancer 2022;20:102-6. [Crossref] [PubMed]
- Chen YH, Chen SH, Hou J, et al. Identifying hub genes of clear cell renal cell carcinoma associated with the proportion of regulatory T cells by weighted gene co-expression network analysis. Aging (Albany NY) 2019;11:9478-91. [Crossref] [PubMed]
- Lee MH, Järvinen P, Nísen H, et al. T and NK cell abundance defines two distinct subgroups of renal cell carcinoma. Oncoimmunology 2022;11:1993042. [Crossref] [PubMed]
- O'Connell P, Hyslop S, Blake MK, et al. SLAMF7 Signaling Reprograms T Cells toward Exhaustion in the Tumor Microenvironment. J Immunol 2021;206:193-205. [Crossref] [PubMed]
- Wu K, Zheng X, Yao Z, et al. Accumulation of CD45RO+CD8+ T cells is a diagnostic and prognostic biomarker for clear cell renal cell carcinoma. Aging (Albany NY) 2021;13:14304-21. [Crossref] [PubMed]
- Wu P, Geng B, Chen Q, et al. Tumor Cell-Derived TGFβ1 Attenuates Antitumor Immune Activity of T Cells via Regulation of PD-1 mRNA. Cancer Immunol Res 2020;8:1470-84. [Crossref] [PubMed]
- Zhu Q, Cai MY, Weng DS, et al. PD-L1 expression patterns in tumour cells and their association with CD8(+) tumour infiltrating lymphocytes in clear cell renal cell carcinoma. J Cancer 2019;10:1154-61. [Crossref] [PubMed]
- Corrò C, Healy ME, Engler S, et al. IL-8 and CXCR1 expression is associated with cancer stem cell-like properties of clear cell renal cancer. J Pathol 2019;248:377-89. [Crossref] [PubMed]
- Fu Q, Xu L, Wang Y, et al. Tumor-associated Macrophage-derived Interleukin-23 Interlinks Kidney Cancer Glutamine Addiction with Immune Evasion. Eur Urol 2019;75:752-63. [Crossref] [PubMed]
- Liang Y, Lin F, Huang Y. Identification of Biomarkers Associated with Diagnosis of Osteoarthritis Patients Based on Bioinformatics and Machine Learning. J Immunol Res 2022;2022:5600190. [Crossref] [PubMed]
- Li C, Su F, Zhang L, et al. Identifying Potential Diagnostic Genes for Diabetic Nephropathy Based on Hypoxia and Immune Status. J Inflamm Res 2021;14:6871-91. [Crossref] [PubMed]
- Chen L, Xiang Z, Chen X, et al. A seven-gene signature model predicts overall survival in kidney renal clear cell carcinoma. Hereditas 2020;157:38. [Crossref] [PubMed]
- Huang H, Reed CP, Zhang JS, et al. Carboxypeptidase A3 (CPA3): a novel gene highly induced by histone deacetylase inhibitors during differentiation of prostate epithelial cancer cells. Cancer Res 1999;59:2981-8. [PubMed]
- Gopinath P, Veluswami S, Gopisetty G, et al. Identification of tumor biomarkers for pathological complete response to neoadjuvant treatment in locally advanced breast cancer. Breast Cancer Res Treat 2022;194:207-20. [Crossref] [PubMed]
- Thaiwong T, Cirillo JV, Heller J, et al. Expression of Carboxypeptidase A3 and Tryptase as Markers for Lymph Node Metastasis of Canine Cutaneous Mast Cell Tumors. Front Vet Sci 2022;9:815658. [Crossref] [PubMed]
- Wen S, Peng W, Chen Y, et al. Four differentially expressed genes can predict prognosis and microenvironment immune infiltration in lung cancer: a study based on data from the GEO. BMC Cancer 2022;22:193. [Crossref] [PubMed]
- Wu B, Tao L, Yang D, et al. Development of an Immune Infiltration-Related Eight-Gene Prognostic Signature in Colorectal Cancer Microenvironment. Biomed Res Int 2020;2020:2719739. [Crossref] [PubMed]
- Yang J, Hong S, Zhang X, et al. Tumor Immune Microenvironment Related Gene-Based Model to Predict Prognosis and Response to Compounds in Ovarian Cancer. Front Oncol 2021;11:807410. [Crossref] [PubMed]
- Loktionov A, Watson MA, Gunter M, et al. Glutathione-S-transferase gene polymorphisms in colorectal cancer patients: interaction between GSTM1 and GSTM3 allele variants as a risk-modulating factor. Carcinogenesis 2001;22:1053-60. [Crossref] [PubMed]
- Wang Y, Yang ZY, Chen YH, et al. A novel functional polymorphism of GSTM3 reduces clear cell renal cell carcinoma risk through enhancing its expression by interfering miR-556 binding. J Cell Mol Med 2018;22:3005-15. [Crossref] [PubMed]
- Li G, Cai Y, Wang C, et al. LncRNA GAS5 regulates the proliferation, migration, invasion and apoptosis of brain glioma cells through targeting GSTM3 expression. The effect of LncRNA GAS5 on glioma cells. J Neurooncol 2019;143:525-36. [Crossref] [PubMed]
- Wang S, Yang J, Ding C, et al. Glutathione S-Transferase Mu-3 Predicts a Better Prognosis and Inhibits Malignant Behavior and Glycolysis in Pancreatic Cancer. Front Oncol 2020;10:1539. [Crossref] [PubMed]
- Yang F, Wen J, Luo K, et al. Low GSTM3 expression is associated with poor disease-free survival in resected esophageal squamous cell carcinoma. Diagn Pathol 2021;16:10. [Crossref] [PubMed]
- Zhang J, Li Y, Zou J, et al. Comprehensive analysis of the glutathione S-transferase Mu (GSTM) gene family in ovarian cancer identifies prognostic and expression significance. Front Oncol 2022;12:968547. [Crossref] [PubMed]
- Anggård E, Larsson C, Samuelsson B. The distribution of 15-hydroxy prostaglandin dehydrogenase and prostaglandin-delta 13-reductase in tissues of the swine. Acta Physiol Scand 1971;81:396-404. [Crossref] [PubMed]
- Fan Y, Yang L, Ren Y, et al. Sp1-Induced SETDB1 Overexpression Transcriptionally Inhibits HPGD in a β-Catenin-Dependent Manner and Promotes the Proliferation and Metastasis of Gastric Cancer. J Gastric Cancer 2022;22:319-38. [Crossref] [PubMed]
- Ghatak S, Mehrabi SF, Mehdawi LM, et al. Identification of a Novel Five-Gene Signature as a Prognostic and Diagnostic Biomarker in Colorectal Cancers. Int J Mol Sci 2022;23:793. [Crossref] [PubMed]
- Kim MJ, Min Y, Jeong SK, et al. USP15 negatively regulates lung cancer progression through the TRAF6-BECN1 signaling axis for autophagy induction. Cell Death Dis 2022;13:348. [Crossref] [PubMed]
- Cai H, Yan L, Liu N, et al. IFI16 promotes cervical cancer progression by upregulating PD-L1 in immunomicroenvironment through STING-TBK1-NF-kB pathway. Biomed Pharmacother 2020;123:109790. [Crossref] [PubMed]
- Ka NL, Lim GY, Hwang S, et al. IFI16 inhibits DNA repair that potentiates type-I interferon-induced antitumor effects in triple negative breast cancer. Cell Rep 2021;37:110138. [Crossref] [PubMed]
- Gao Z, Xu J, Fan Y, et al. ARPC1B promotes mesenchymal phenotype maintenance and radiotherapy resistance by blocking TRIM21-mediated degradation of IFI16 and HuR in glioma stem cells. J Exp Clin Cancer Res 2022;41:323. [Crossref] [PubMed]
- Shimizu YK, Oomura M, Abe K, et al. Production of antibody associated with non-A, non-B hepatitis in a chimpanzee lymphoblastoid cell line established by in vitro transformation with Epstein-Barr virus. Proc Natl Acad Sci U S A 1985;82:2138-42. [Crossref] [PubMed]
- Pan H, Wang X, Huang W, et al. Interferon-Induced Protein 44 Correlated With Immune Infiltration Serves as a Potential Prognostic Indicator in Head and Neck Squamous Cell Carcinoma. Front Oncol 2020;10:557157. [Crossref] [PubMed]
- Jiang S, Zhou F, Zhang Y, et al. Identification of tumorigenicity-associated genes in osteosarcoma cell lines based on bioinformatic analysis and experimental validation. J Cancer 2020;11:3623-33. [Crossref] [PubMed]
- Wang H, Lu B, Ren S, et al. Long Noncoding RNA LINC01116 Contributes to Gefitinib Resistance in Non-small Cell Lung Cancer through Regulating IFI44. Mol Ther Nucleic Acids 2020;19:218-27. [Crossref] [PubMed]
- Hwa V, Oh Y, Rosenfeld RG. The insulin-like growth factor-binding protein (IGFBP) superfamily. Endocr Rev 1999;20:761-87. [PubMed]
- Ingermann AR, Yang YF, Han J, et al. Identification of a novel cell death receptor mediating IGFBP-3-induced anti-tumor effects in breast and prostate cancer. J Biol Chem 2010;285:30233-46. [Crossref] [PubMed]
- Ho PJ, Baxter RC. Characterization of truncated insulin-like growth factor-binding protein-2 in human milk. Endocrinology 1997;138:3811-8. [Crossref] [PubMed]
- Cai Q, Dozmorov M, Oh Y. IGFBP-3/IGFBP-3 Receptor System as an Anti-Tumor and Anti-Metastatic Signaling in Cancer. Cells 2020;9:1261. [Crossref] [PubMed]
- Liu Y, Lv H, Li X, et al. Cyclovirobuxine inhibits the progression of clear cell renal cell carcinoma by suppressing the IGFBP3-AKT/STAT3/MAPK-Snail signalling pathway. Int J Biol Sci 2021;17:3522-37. [Crossref] [PubMed]
- Chen X, Shao Y, Wei W, et al. Downregulation of LOX promotes castration-resistant prostate cancer progression via IGFBP3. J Cancer 2021;12:7349-57. [Crossref] [PubMed]
- Kuhn H, Frille A, Petersen MA, et al. IGFBP3 inhibits tumor growth and invasion of lung cancer cells and is associated with improved survival in lung cancer patients. Transl Oncol 2023;27:101566. [Crossref] [PubMed]
- Dolan J, Walshe K, Alsbury S, et al. The extracellular leucine-rich repeat superfamily; a comparative survey and analysis of evolutionary relationships and expression patterns. BMC Genomics 2007;8:320. [Crossref] [PubMed]
- Chai L, Dai L, Che Y, et al. LRRC19, a novel member of the leucine-rich repeat protein family, activates NF-kappaB and induces expression of proinflammatory cytokines. Biochem Biophys Res Commun 2009;388:543-8. [Crossref] [PubMed]
- Zhang Y, Wang J, Liu X. LRRC19-A Bridge between Selenium Adjuvant Therapy and Renal Clear Cell Carcinoma: A Study Based on Datamining. Genes (Basel) 2020;11:440. [Crossref] [PubMed]
- Zhang Y, Tang M, Guo Q, et al. The value of erlotinib related target molecules in kidney renal cell carcinoma via bioinformatics analysis. Gene 2022;816:146173. [Crossref] [PubMed]
- Saha SK, Islam SMR, Kwak KS, et al. PROM1 and PROM2 expression differentially modulates clinical prognosis of cancer: a multiomics analysis. Cancer Gene Ther 2020;27:147-67. [Crossref] [PubMed]
- Dansonka-Mieszkowska A, Szafron LA, Kulesza M, et al. PROM1, CXCL8, RUNX1, NAV1 and TP73 genes as independent markers predictive of prognosis or response to treatment in two cohorts of high-grade serous ovarian cancer patients. PLoS One 2022;17:e0271539. [Crossref] [PubMed]
- Zhou L, Yu KH, Wong TL, et al. Lineage tracing and single-cell analysis reveal proliferative Prom1+ tumour-propagating cells and their dynamic cellular transition during liver cancer progression. Gut 2022;71:1656-68. [PubMed]
- Lu A, Shi Y, Liu Y, et al. Integrative analyses identified ion channel genes GJB2 and SCNN1B as prognostic biomarkers and therapeutic targets for lung adenocarcinoma. Lung Cancer 2021;158:29-39. [Crossref] [PubMed]
- Qian Y, Zhou L, Luk STY, et al. The sodium channel subunit SCNN1B suppresses colorectal cancer via suppression of active c-Raf and MAPK signaling cascade. Oncogene 2023;42:601-12. [Crossref] [PubMed]