SMIM20: a new biological signal associated with the prognosis of glioblastoma
Highlight box
Key findings
• SMIM20 can be a new biomarker for the prognosis of glioblastoma multiforme (GBM).
What is known and what is new?
• SMIM20 leads to a poor prognosis and is clinically significant in GBM.
• SMIM20 showed good predictive abilities regarding prognosis of GBM.
What is the implication, and what should change now?
• SMIM20 could emerge as a new prognostic factor for GBM, although further research is required.
Introduction
Glioblastoma multiforme (GBM; World Health Organization Grade IV glioma) is the most lethal and primary brain cancer among adults, accounting for 14.2% of all primary brain and other central nervous system (CNS) tumors and 50.1% of malignant primary brain cancers. The estimated relative survival of patients with GBM is very low, and the 5-year survival rate of many patients is only 6.09% after surgery, active radiotherapy, and chemotherapy (1,2). Traditional treatment methods can no longer meet expectations regarding the prognosis of GBM. Personalized targeted therapies based on biomarkers have emerged as treatment strategies. However, it is difficult for the existing targeted drug therapies to achieve the expected results (3). Therefore, the requirement for new biomarkers for improving the diagnosis and prognosis of GBM is urgent.
SMIM20 is a small integral membrane protein, and its cleavage products, PNX-14 and PNX-20, play significant roles in the CNS and female reproductive system (4,5). Previous studies have showed that SMIM30 plays an important role in hepatocellular carcinoma (HCC) (6). In addition, patients with acute myeloid leukemia (AML) and high SMIM3 expression have a worse prognosis (7). Although SMIM20 is involved in the adverse outcomes of AML (8), the expression of SMIM20 in GBM and its prognostic value remain unclear. We found that SMIM20 can participate in the regulation and inflammatory response of microglia by cleavage to form PNX (4), and microglia play an important role in GBM, especially in promoting tumor development, immunosuppression, and drug resistance (9-11).
Based on the above conclusions, we hypothesized that SMIM20 is a potential prognostic marker for GBM. However, a literature search revealed almost no research on the role of SMIM20 in GBM. Therefore, in this study, we conducted a large-scale bioinformatics analysis using corresponding public datasets to verify our hypothesis, focusing on the potential role and mechanism of SMIM20 and its clinical significance in GBM. We present this article in accordance with the TRIPOD reporting checklist (available at https://tcr.amegroups.com/article/view/10.21037/tcr-23-796/rc).
Methods
Data acquisition and processing
We downloaded The Cancer Genome Atlas (TCGA) and Genotype-Tissue Expression (GTEx) pan-cancer RNA-seq data with toils processed uniformly from the public open online platform UCSC XENA (https://xenabrowser.net/datapages/) (8). The TCGA website (https://portal.gdc.cancer.gov/repository) provides the corresponding clinical information of the GBM samples, including age, sex, survival status, survival time, and gene expression data of High-Throughput Sequencing Fragments Per Kilobase of transcript per Million mapped reads (HTSeq-FPKM) and HTSeq-Count. The study was conducted in accordance with the Declaration of Helsinki (as revised in 2013). Our research is based on open-source data from public databases. The patients involved in the databases have obtained ethical approval, so there are no ethical issues.
Differential expression analysis of SMIM20
We performed a differential expression analysis of SMIM20 using the DESeq2 R package to identify differential expression genes (DEGs) (9). The first seven upregulated genes and three downregulated genes were selected to construct a heat map.
Functional enrichment analysis
Functional enrichment analysis was performed on DEGs with a log fold change (FC) criterion of >1.5 and adjusted P value (P.adj) <0.05. Gene Ontology (GO) enrichment and Kyoto Encyclopedia of Genes and Genomes (KEGG) pathway analyses were performed using ClusterProfiler (10).
Gene set enrichment analysis (GSEA)
Functional and pathway distinctions between the SMIM20 high- and low-expression groups were clarified using GSEA and the R package ClusteProfiler (3.14.3) (11). An P.adj <0.05 and false discovery rate (FDR) <0.25 were considered significantly enriched.
Immune cell infiltration analysis
SMIM20 immune infiltration analysis was performed using the R gene set variation analysis (GSVA) tool (4.2.1). Gene markers for 24 different types of infiltrating immune cells were obtained from literature (12). The correlation between SMIM20 and 24 immune cell infiltration was examined using Spearman correction (13).
Prognostic model generation and prediction
The RMS R software package (version 6.3-0) was used to visualize the nomogram of patients with GBM for 0.5-, 1-, and 3-year overall survival (OS) prediction model maps to personalize their OS. The model included two independent prognostic factors, isocitrate dehydrogenase (IDH) and SMIM20 expression. The model’s accuracy was explored using the consistency index (C-index) and receiver operating characteristic (ROC).
Statistical analysis
Statistical analysis was conducted using R software (version 4.2.1) (14). The Wilcoxon rank-sum test was used to evaluate the expression of SMIM20 in unpaired samples, and the Wilcoxon signed-rank test was used for paired samples. Cox regression analysis and the Kaplan-Meier test were used to evaluate survival data. Univariate and multivariate Cox regression analyses were used to evaluate independent prognostic factors. All tests were considered statistically significant at P<0.05. ROC analysis was performed using the pROC package to evaluate the efficacy of SMIM20 transcriptional expression for distinguishing SMIM20 from normal samples.
Results
SMIM20 expression in pan-cancers and GBM
By comparing normal and tumor samples in the TCGA and GTEx databases, SMIM20 was found to be highly expressed in various types of cancers (Figure 1A), including GBM (Figure 1B).
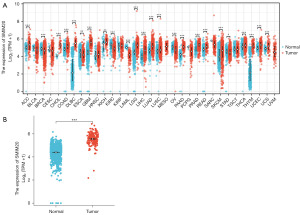
Identification of DEGs with low- and high-expressed SMIM20
According to the median value of SMIM20 expression, patients with GBM in the TCGA database were divided into low and high SMIM20 expression groups. In total, 156 DEGs (131 genes upregulated and 25 genes downregulated) were identified. |logFC| >1.5 and P<0.05 were considered significant (Figure 2A). The heat map shows the first seven upregulated DEGs and the first three downregulated DEGs between the SMIM20 high- and low-expression groups (Figure 2B).
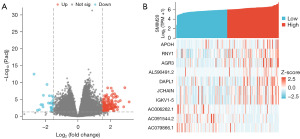
Functional enrichment analysis
To gain further insights into the biological functions associated with DEG, the clusterProfiler package was used for GO and KEGG functional enrichment analyses (Figure 3). From the analyses, we found that biological processes (BP) included immunoglobulin production, production of molecular mediators of immune response, phagocytosis and recognition, complement activation classical pathway, and cellular components (CC), including the immunoglobulin complex, circulating immunoglobulin complex, external side of the plasma membrane, and blood microparticles. Molecular functions (MF) included antigen and immunoglobulin receptor binding. In addition, we analyzed the GO joint logFC values, and all Z-scores were positive, indicating that SMIM20 may positively regulate them. However, the KEGG pathways were not effectively enriched.
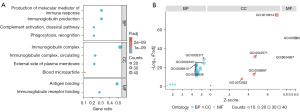
To better appreciate the SMIM20-related signaling pathways, we performed GSEA. GSEA was performed on all previously obtained DEGs between the high and low SMIM20 expression datasets, and we enriched some pathways with significant differences (P<0.05) (Table S1). With the increased expression of SMIM20 based on the normalize enrichment score (NES), immune and oxidative phosphorylation-related pathways were mainly enriched, such as the interleukin (IL)-5 pathway, IL-17 pathway, and oxidative phosphorylation (Figure 4A-4F).
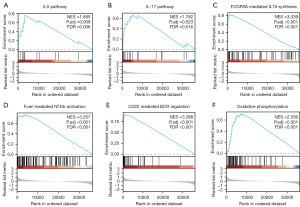
Immune infiltration analysis
Immune infiltration was analyzed using Spearman’s correlation analysis. SMIM20 expression correlated with diverse immune cell infiltration levels. Most significantly, SMIM20 expression was inversely associated with the number of effector memory T (Tem) cells (Figure 5).
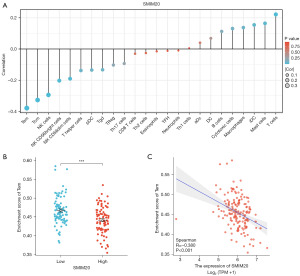
Prognostic model of SMIM20 in GBM
ROC curve analysis was used to examine the potential value of SMIM20 to distinguish patients with GBM from healthy individuals. As shown in Figure 6A, SMIM20 is a potential biomarker with an area under the curve (AUC) of 0.951 (Figure 6A). The association between SMIM20 expression and GBM prognosis was analyzed using the Kaplan-Meier method (Figure 6B). Compared to patients with low SMIM20 expression, patients with high SMIM20 expression tended to have a worse prognosis [hazard ratio (HR), 1.670; 95% confidence interval (CI): 1.174–2.375; P=0.004]. Subsequently, a univariate Cox proportional hazard regression was used to determine the factors that affect OS and SMIM20 (high vs. low, P=0.004) was found to be a predictor of decline in OS, as was IDH [mutant (Mut) vs. wild type (WT), P<0.001] (Table S2). IDH (WT) (P=0.025) and high SMIM20 expression (P=0.012) were independent prognostic factors associated with OS deterioration (P<0.05). Subsequently, a nomogram was constructed using the RMS package in R (Figure 7A). We created a nomogram for a GBM patient survival prediction map based on IDH and SMIM20 expression. As shown in Figure 7A, IDH, and SMIM20 expression affected the 0.5-, 1-, and 3-year OS of patients with GBM. The calibration curve showed that the nomogram consistently predicted the 0.5-, 1-, and 3-year OS of patients with GBM (Figure 7B). These results indicate that SMIM20 can predict the prognosis of GBM.
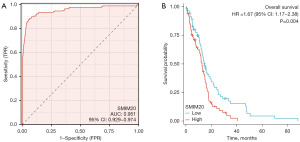
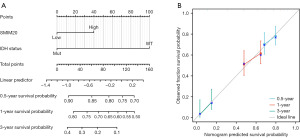
Discussion
The first step in this study was to discuss the expression of SMIM20 in various types of tumors. In GBM, the expression of SMIM20 was significantly higher than that in normal samples, prompting us to investigate SMIM20 expression further. GSEA revealed that the SMIM20 phenotype was primarily abundant in the IL-5 and IL-17 pathways, FCGR3A-mediated IL-10 synthesis, oxidative phosphorylation, FCERI-mediated NF-κB activation, and CD22-mediated B-cell receptor (BCR) regulation. ILs are immunosuppressive factors that promote tumor immune escape by promoting antitumor immune reactions in a tumor microenvironment. Previous studies have shown that IL-10 reduces or inhibits antigen presentation by downregulating major histocompatibility complex (MHC) class II expression in antigen-presenting cell (APC) (15) and MHC class I expression in tumor cells (16), thus contributing to an immunosuppressive environment and ultimately promoting tumor escape. In addition, IL-10 autocrine signaling in tumor cells may promote tumor development (17). Furthermore, IL-5 is closely associated with the survival of patients with GBM (18). Similarly, in multiple human malignancies, high expression of IL-17 signature genes can be found, including cervical, esophageal, gastric, hepatocellular, and colorectal cancers, which are involved in adverse outcomes of these cancers (19,20). IL-17 is also closely associated with breast cancer metastasis (21). Incorrect regulation of NF-κB has been associated with various tumors (22), and NF-κB contributes to tumorigenesis and escape from immune surveillance in GBM (23). In addition, studies have shown that tumor necrosis factor-like weak inducer of apoptosis (TWEAK) promotes the invasion of glioma cells by inducing NF-κB-induced kinase (NIK) and atypical NF-κB signal transduction (24). Mitochondrial oxidative phosphorylation plays a key role in tumor cell immortalization. Brain tumor cells generate energy through oxidative phosphorylation rather than glycolysis (25). Oxidative phosphorylation is an emerging target in cancer treatment (26). The results of the GSEA enrichment analysis were consistent with the core results of this study: high expression of SMIM20 was associated with poor prognosis of GBM.
In the infiltration analysis of immune cells, SMIM20’s high expression was associated with fewer central memory T (Tcm) and Tem cells. Both Tcm and Tem are memory T cells (Tm). Tcm expresses CCR7 and CD62L, produces IL-2, and proliferates in large quantities, whereas Tem proliferates less and produces effector cytokines such as interferon (IFN)-γ, CD4+ and CD8+ cells. Tcm dominates in secondary lymphoid organs, whereas T cells dominate in the peripheral compartment (27,28). Relevant studies have shown that both Tem and Tcm possess antitumor properties. Tem cells have been shown to express higher levels of receptors, are responsible for the migration to inflammatory tissues, and have stronger immediate effects than Tcm cells (29,30). However, compared to Tem cells, Tcm cells with lower terminal differentiation may have better persistence and antitumor efficacy (31,32), making them more suitable for adoptive immunotherapy of tumors.
Our most notable finding is that high expression of SMIM20 is associated with shorter life expectancy. According to multivariate Cox regression analysis, SMIM20 was another independent prognostic factor apart from IDH. Combining SMIM20 with IDH phenotype, a nomogram prediction model was constructed to obtain a more accurate prognostic prediction model. The Cox model based on the C-index SMIM20 predicts an OS of 0.577 (0.550–0.603). This calibration plot illustrates the best match between the SMIM20 nomogram predictions and the actual observations of 0.5-, 1-, and 3-year OS probabilities. Therefore, SMIM20 can be used as a novel adverse prognostic factor for GBM patients with GBM. In addition, our model can provide customized scores for each GBM.
However, this study has limitations owing to its small sample size. The sample size should be increased to improve the validity of future research and ensure greater reliability. In future studies, clinical samples should be used to validate the reliability of the GBM prognostic value of this signature.
Conclusions
In conclusion, our study confirmed the differential expression, immune infiltration, possible pathways, and prognostic significance of SMIM20 in GBM. To the best of our knowledge, it revealed for the first time that SMIM20 can be a new biomarker for the prognosis of GBM.
Acknowledgments
Funding: This study was supported by
Footnote
Reporting Checklist: The authors have completed the TRIPOD reporting checklist. Available at https://tcr.amegroups.com/article/view/10.21037/tcr-23-796/rc
Peer Review File: Available at https://tcr.amegroups.com/article/view/10.21037/tcr-23-796/prf
Conflicts of Interest: All authors have completed the ICMJE uniform disclosure form (available at https://tcr.amegroups.com/article/view/10.21037/tcr-23-796/coif). All authors report that this study was supported by the Luzhou Science and Technology Plan Project (No. 2020-SYF-29). The authors have no other conflicts of interest to declare.
Ethical Statement: The authors are accountable for all aspects of the work in ensuring that questions related to the accuracy or integrity of any part of the work are appropriately investigated and resolved. The study was conducted in accordance with the Declaration of Helsinki (as revised in 2013). Our research is based on open-source data from public databases. The patients involved in the databases have obtained ethical approval, so there are no ethical issues.
Open Access Statement: This is an Open Access article distributed in accordance with the Creative Commons Attribution-NonCommercial-NoDerivs 4.0 International License (CC BY-NC-ND 4.0), which permits the non-commercial replication and distribution of the article with the strict proviso that no changes or edits are made and the original work is properly cited (including links to both the formal publication through the relevant DOI and the license). See: https://creativecommons.org/licenses/by-nc-nd/4.0/.
References
- Alexander BM, Cloughesy TF. Adult Glioblastoma. J Clin Oncol 2017;35:2402-9. [Crossref] [PubMed]
- Ostrom QT, Price M, Neff C, et al. CBTRUS Statistical Report: Primary Brain and Other Central Nervous System Tumors Diagnosed in the United States in 2015-2019. Neuro Oncol 2022;24:v1-v95. [Crossref] [PubMed]
- Shergalis A, Bankhead A 3rd, Luesakul U, et al. Current Challenges and Opportunities in Treating Glioblastoma. Pharmacol Rev 2018;70:412-45. [Crossref] [PubMed]
- Billert M, Rak A, Nowak KW, et al. Phoenixin: More than Reproductive Peptide. Int J Mol Sci 2020;21:8378. [Crossref] [PubMed]
- Yosten GL, Lyu RM, Hsueh AJ, et al. A novel reproductive peptide, phoenixin. J Neuroendocrinol 2013;25:206-15. [Crossref] [PubMed]
- Unfried JP, Fortes P. SMIM30, a tiny protein with a big role in liver cancer. J Hepatol 2020;73:1010-2. [Crossref] [PubMed]
- Liu Y, Chen Y, Liu Y, et al. Downregulation of SMIM3 inhibits growth of leukemia via PI3K-AKT signaling pathway and correlates with prognosis of adult acute myeloid leukemia with normal karyotype. J Transl Med 2022;20:612. [Crossref] [PubMed]
- Vivian J, Rao AA, Nothaft FA, et al. Toil enables reproducible, open source, big biomedical data analyses. Nat Biotechnol 2017;35:314-6. [Crossref] [PubMed]
- Love MI, Huber W, Anders S. Moderated estimation of fold change and dispersion for RNA-seq data with DESeq2. Genome Biol 2014;15:550. [Crossref] [PubMed]
- Yu G, Wang LG, Han Y, et al. clusterProfiler: an R package for comparing biological themes among gene clusters. OMICS 2012;16:284-7. [Crossref] [PubMed]
- Subramanian A, Tamayo P, Mootha VK, et al. Gene set enrichment analysis: a knowledge-based approach for interpreting genome-wide expression profiles. Proc Natl Acad Sci U S A 2005;102:15545-50. [Crossref] [PubMed]
- Hänzelmann S, Castelo R, Guinney J. GSVA: gene set variation analysis for microarray and RNA-seq data. BMC Bioinformatics 2013;14:7. [Crossref] [PubMed]
- Bindea G, Mlecnik B, Tosolini M, et al. Spatiotemporal dynamics of intratumoral immune cells reveal the immune landscape in human cancer. Immunity 2013;39:782-95. [Crossref] [PubMed]
- Isidro-Sánchez J, Akdemir D, Montilla-Bascón G. Genome-Wide Association Analysis Using R. Methods Mol Biol 2017;1536:189-207. [Crossref] [PubMed]
- Steinbrink K, Jonuleit H, Müller G, et al. Interleukin-10-treated human dendritic cells induce a melanoma-antigen-specific anergy in CD8(+) T cells resulting in a failure to lyse tumor cells. Blood 1999;93:1634-42. [Crossref] [PubMed]
- Adris S, Klein S, Jasnis M, et al. IL-10 expression by CT26 colon carcinoma cells inhibits their malignant phenotype and induces a T cell-mediated tumor rejection in the context of a systemic Th2 response. Gene Ther 1999;6:1705-12. [Crossref] [PubMed]
- Mannino MH, Zhu Z, Xiao H, et al. The paradoxical role of IL-10 in immunity and cancer. Cancer Lett 2015;367:103-7. [Crossref] [PubMed]
- Zhenjiang L, Rao M, Luo X, et al. Cytokine Networks and Survivin Peptide-Specific Cellular Immune Responses Predict Improved Survival in Patients With Glioblastoma Multiforme. EBioMedicine 2018;33:49-56. [Crossref] [PubMed]
- McGeachy MJ, Cua DJ, Gaffen SL. The IL-17 Family of Cytokines in Health and Disease. Immunity 2019;50:892-906. [Crossref] [PubMed]
- Tosolini M, Kirilovsky A, Mlecnik B, et al. Clinical impact of different classes of infiltrating T cytotoxic and helper cells (Th1, th2, treg, th17) in patients with colorectal cancer. Cancer Res 2011;71:1263-71. [Crossref] [PubMed]
- Coffelt SB, Kersten K, Doornebal CW, et al. IL-17-producing γδ T cells and neutrophils conspire to promote breast cancer metastasis. Nature 2015;522:345-8. [Crossref] [PubMed]
- Verzella D, Pescatore A, Capece D, et al. Life, death, and autophagy in cancer: NF-κB turns up everywhere. Cell Death Dis 2020;11:210. [Crossref] [PubMed]
- Yi K, Cui X, Liu X, et al. PTRF/Cavin-1 as a Novel RNA-Binding Protein Expedites the NF-κB/PD-L1 Axis by Stabilizing lncRNA NEAT1, Contributing to Tumorigenesis and Immune Evasion in Glioblastoma. Front Immunol 2022;12:802795. [Crossref] [PubMed]
- Cherry EM, Lee DW, Jung JU, et al. Tumor necrosis factor-like weak inducer of apoptosis (TWEAK) promotes glioma cell invasion through induction of NF-κB-inducing kinase (NIK) and noncanonical NF-κB signaling. Mol Cancer 2015;14:9. [Crossref] [PubMed]
- Bonnay F, Veloso A, Steinmann V, et al. Oxidative Metabolism Drives Immortalization of Neural Stem Cells during Tumorigenesis. Cell 2020;182:1490-1507.e19. [Crossref] [PubMed]
- Ashton TM, McKenna WG, Kunz-Schughart LA, et al. Oxidative Phosphorylation as an Emerging Target in Cancer Therapy. Clin Cancer Res 2018;24:2482-90. [Crossref] [PubMed]
- Masopust D, Vezys V, Marzo AL, et al. Preferential localization of effector memory cells in nonlymphoid tissue. Science 2001;291:2413-7. [Crossref] [PubMed]
- Reinhardt RL, Khoruts A, Merica R, et al. Visualizing the generation of memory CD4 T cells in the whole body. Nature 2001;410:101-5. [Crossref] [PubMed]
- Sallusto F, Geginat J, Lanzavecchia A. Central memory and effector memory T cell subsets: function, generation, and maintenance. Annu Rev Immunol 2004;22:745-63. [Crossref] [PubMed]
- Geginat J, Sallusto F, Lanzavecchia A. Cytokine-driven proliferation and differentiation of human naive, central memory, and effector memory CD4(+) T cells. J Exp Med 2001;194:1711-9. [Crossref] [PubMed]
- Klebanoff CA, Gattinoni L, Torabi-Parizi P, et al. Central memory self/tumor-reactive CD8+ T cells confer superior antitumor immunity compared with effector memory T cells. Proc Natl Acad Sci U S A 2005;102:9571-6. [Crossref] [PubMed]
- Liu Q, Sun Z, Chen L. Memory T cells: strategies for optimizing tumor immunotherapy. Protein Cell 2020;11:549-64. [Crossref] [PubMed]