Establishment of a prognostic signature for patients with advanced lung squamous cell carcinoma based on tumor-infiltrating immune cells
Highlight box
Key findings
• Based on the nomogram of natural killer cells resting, monocytes, and macrophages M2, the research can make specific prognostic predictions for advanced lung squamous cell carcinoma (LUSC) patients.
What is known and what is new?
• There is an important correlation between immune cell infiltration and the occurrence and development of LUSC.
• Establishment of a prognostic signature for patients with advanced LUSC based on tumor-infiltrating immune cells.
What is the implication, and what should change now?
• This prediction model can serve as a valuable tool for clinicians, and enable them to provide effective guidance based on tumor-infiltrating immune cells for advanced LUSC patients.
Introduction
According to Siegel’s estimation in Cancer Statistics [2022], lung and bronchus cancers stand as the primary cause of morbidity and mortality (1). Lung cancer can be broadly classified into two main types: small cell lung cancer, accounting for approximately 13% of all cases, and non-small cell lung cancer (NSCLC), accounting for around 87% of cases. NSCLC further encompasses subtypes such as lung adenocarcinoma (LUAD: 47.9%), lung squamous cell carcinoma (LUSC: 23.2%), and others (2-4). As genetic testing advances and immunotherapy exhibits remarkable efficacy, an increasing number of relevant factors are being utilized to predict patient survival. However, significant challenges persist in their clinical application.
Immune cell infiltration, dependent on the stage of the disease, is widely distributed in lung cancer, and the studies highlight the critical role of the tumor microenvironment (TME) in disease occurrence (5,6). The TME comprises tumor cells, surrounding immune and inflammatory cells, tumor-associated fibroblasts, neighboring interstitial tissues, microvessels, cytokines, and chemokines. Within this complex integrated system, the interaction between tumor cells and immune cells plays a pivotal role in tumor progression, with local cancer antigen-specific immune responses influencing disease advancement (7,8). Analyzing the genomic TME in a subtype-specific manner has the potential to impact immunotherapy approaches for LUSC patients (9). While an inflamed TME is positively correlated with patient survival in LUAD, the same does not hold true for LUSC (7). Nonetheless, LUSC cases with immune inflammation exhibit higher expression of immune checkpoints compared to LUAD (7). The immunophenotypic score based on the degree of immune cell infiltration in the TME can well predict the efficacy of immunotherapy in patients with LUSC, and has certain prognostic value (10). Recent investigations have emphasized the significance of tumor cells and the microenvironment in tumor development (11), such as tumor metastasis (12). Guo et al. reveals that, among the 27 tumor-infiltrating immune cells, 14 were associated with the prognosis of LUSC. Among them, the prediction model based on central memory CD8 T cells, memory B cells, plasmacytoid dendritic cells and regulatory T cells has good clinical application value (13).
Numerous immune cell subtypes, including natural killer (NK) cells, B cells, TH17 cells, regulatory T cells (Tregs), CD8+ T cells, TH1/2 cells, T follicular helper (TFH) cells, dendritic cells, and macrophages, are abundant in tumor tissues (14). Tumor-associated macrophages (TAMs) currently exert an inhibitory effect on tumors, and a higher number and density of macrophages correspond to worse patient prognosis (15). The postoperative survival time of lung cancer patients is commonly evaluated based on tumor stage and grade (14). Previous study has established a correlation between lymphoid structures and patient survival (8). Prediction of patient prognosis can be achieved by developing a model that reflects the impact of immunotherapy (12). Recent research has focused on predicting patient prognosis by analyzing patterns of immune cell infiltration, providing a foundation for future immunotherapy approaches (14). Standardized immunopathology assessment, along with predictive or prognostic scores, assists clinicians in determining appropriate treatment options (8). Sica et al. proposed that the recruitment and activation of TAMs may play a pivotal role in tumor progression, serving as a potential target for future prediction and treatment. TAMs-mediated M2 macrophages can induce persistent inflammatory response (15). Increased macrophage expression has been confirmed by Seo et al. to correlate with TAMs, inflammation, and mutation-driven tumors (9). In-depth exploration of the TME facilitates the implementation of tailored immunotherapy for patients (9). In many primary tumors, the infiltration of Th1 cells and cytotoxic T cells is associated with improved survival rates (12). Furthermore, macrophage M2 activation and elevated VEGF expression negatively correlate with patient prognosis (12).
Although various factors, including infections, can lead to immune cell infiltration, the administration of immunotherapy drugs in patients can help evaluate tumor-infiltrating immune cells using immunohistochemistry. Nevertheless, the use of the same marker expression across different cell types may introduce bias and result in incomplete identification of tumor-infiltrating immune cell types and their distribution. Fortunately, the advancement of bioinformatics tools enables the simulation of tumor immune cells using methods such as CIBERSORT (16). This approach facilitates accurate inference, calculation, and assessment of differences, polymorphisms, and landscapes of tumor-infiltrating immune cells. Previous investigations employed immunohistochemistry to preliminarily examine the tumor infiltration of NSCLC, indicating a positive correlation between B/T/dendritic cell (DC cell) infiltration and patient prognosis. Conversely, Treg and TAM cell infiltration demonstrated an inverse pattern with a negative correlation (16-19).
In our study, we employed the CIBERSORT method for bioinformatics analysis to accurately depict the landscape of immune cell infiltration and its impact on the survival prognosis of patients with advanced LUSC. Our findings provide valuable insights for clinicians. We present this article in accordance with the TRIPOD reporting checklist (available at https://tcr.amegroups.com/article/view/10.21037/tcr-23-545/rc).
Methods
Data sources
Gene expression and the relevant prognostic and clinicopathological data of LUSC were downloaded from The Cancer Genome Atlas (TCGA) (https://portal.gdc.cancer.gov/) databases, including 49 normal lung tissue samples and 447 LUSC samples. Patients in stages III and IV were enrolled in the study. Thus, a total of 79 cases advanced LUSC patients were included in the subsequent analyses.
Evaluation of immune infiltration
Counting tumor-infiltrating immune cells is crucial for studying the tumor immune microenvironment. Here, we provide a concise overview of a method utilizing CIBERSORT for immune cell counting in tumor tissues. CIBERSORT is a computational tool that predicts and identifies different immune cell types based on gene expression data and known immune cell gene signatures (20). The methodology involves the following steps: (I) collection of gene expression data from tumor tissues; (II) analysis of the data using the CIBERSORT algorithm; (III) calculation of relative percentages of different immune cell types using statistical methods. This methodology enables accurate quantification of tumor-infiltrating immune cells. This study was conducted in accordance with the Declaration of Helsinki (as revised in 2013).
Construction and validation of the tumor-infiltrating immune cells prognostic model
We performed a univariate Cox regression analysis on 22 immune cells. The immune cells related to the patient’s overall survival (OS) rate were selected (P<0.05). We identified four kinds of effective immune cells. Three immune cells were selected by Multivariate Cox regression analysis. Then the multivariate Cox regression analysis was performed to calculate the contribution rate of each immune cell for survival prediction. Based on these analyses, the risk score model was established, and the patients are divided into high-risk and low-risk groups as per the model (9,20-22). . In this formula, n represents the number of tumor-infiltrating immune cells that influence patient prognosis, Expi is the profile abundance of tumor-infiltrating immune cells, and Ci is the regression coefficient of each immune cell obtained on multivariate Cox regression analysis. The survival analysis curve was drawn using the Kaplan-Meier method. Log-rank test was used to determine if the difference was significant (21). The area under the curve (AUC) value was calculated by plotting the receiver operating characteristic (ROC) curves of the models based on the three immune cells. We further randomly divided the cohort into test set A and test set B to verify the stability of the model.
Establishment of the clinical diagnosis prediction model
The package “rms” was utilized to construct a nomogram, providing valuable clinical predictive information regarding the clinical characteristics and risk score of patients with advanced LUSC, particularly on 3- and 5-year OS. In the nomogram, each clinical variable was mapped with a score and the total score was calculated by adding the scores across all the variables.
Statistical analysis
Statistical analysis was conducted through R (version 4.0.2) (Boston, USA). Comparisons between two groups were presented via Wilcoxon rank-sum test. The cutoff point of each subgroup was identified by the survminer package in R. Kaplan-Meier curves for OS analysis were presented between different subgroups, followed by log rank test. ROCs for 3-year survival were delineated for evaluation of the predictive efficacy of the model. P<0.05 was considered to indicate statistical significance.
Results
Data acquisition and study procedures
Raw data of clinical information and RNA expression of patients were obtained from TCGA database, and patients with early LUSC were excluded. A total of 49 normal lung tissues and 79 patients with advanced LUSC were included. On this basis, we performed TME profile on the information, and subsequently performed univariate and multivariate Cox regression analysis on the relevant factors to establish the relevant prognostic model. Then the model was validated. Figure 1 showed the flow chart of the research.
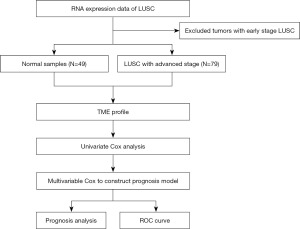
Comparing the infiltration rates of immune cell subsets in normal lung tissue and advanced LUSC
We compared the differences in the infiltration of immune cells between normal tissues and advanced LUSC. The light blue part represents normal lung tissue, and the light pink part shows advanced LUSC tissue, the results showed that there were significant differences between many tumors infiltrating immune cells (Figure 2A). Then, we compared the infiltration rates between normal tissues and advanced LUSC tissues by a violin map. The results showed that there were no differences between the two groups in macrophages M0 (P=0.11), T cell CD8 (P=0.576), B cell naive (P=0.056), NK cell activated (P=0.146), mast cell activated (P=0.319), B cell memory (P=0.64) or T cells CD4 naive (P=0.749) (Figure 2B). For other tumor-infiltrating immune cells, there were significant differences between advanced LUSC tissues and normal tissues (P<0.05).
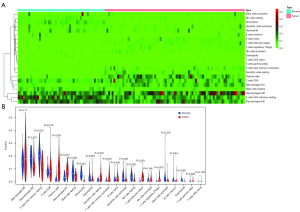
Construction of the prognostic model in advanced LUSC
Through the univariable Cox HR regression analysis, we selected 4 immune cells for further research. Multivariable Cox analysis was used for further modeling. Moreover, three types of immune cells remained after the forward and backward optimized screening, including NK cells resting, monocytes and macrophages M2. Table 1 describes the coefficient values, confidence intervals, and P values for the three types of immune cells with predictive effects.
Table 1
ID | Coef | HR | HR.95L | HR.95H | P value |
---|---|---|---|---|---|
NK cells resting | 0.156 | 1.169 | 0.996 | 1.370 | 0.056 |
Monocytes | −0.370 | 0.691 | 0.498 | 0.958 | 0.026 |
Macrophages M2 | 0.066 | 1.069 | 1.008 | 1.131 | 0.026 |
COX model: In (h(t, x)/h0(t)) = 0.156 × NK cells resting + (−0.370 × monocytes) + 0.066 × macrophages M2. NK, natural killer; HR, hazard ratio; L, low; H, high.
Kaplan-Meier survival analysis
ROC curve is a commonly used tool to evaluate the performance of binary classification models. It plots the true positive rate (also known as sensitivity) on the y-axis against the false positive rate on the x-axis. The ROC curve illustrates the trade-off between sensitivity and specificity at different classification thresholds. AUC represents the area under the ROC curve and ranges between 0 and 1. It represents the probability that the model will rank a randomly chosen positive example higher than a randomly chosen negative example. In other words, higher AUC values indicate better discriminative power of the model in distinguishing between positive and negative samples. Typically, the AUC values range from 0 to 1, where 0.5 indicates the model’s performance is equivalent to random guessing. AUC greater than 0.5 signifies that the model’s performance is better than random, and the closer the value is to 1, the better the model’s performance. As a general rule of thumb, an AUC greater than 0.8 is considered good performance, and an AUC greater than 0.9 is considered excellent.
Based on the above three immune cell subgroups, we calculated the risk score and divided the 79 patients with advanced LUSC into a high-risk group and a low-risk group based on median of the risk score. There were 39 patients in the high-risk group, and the median survival time was 1.745 years. There were 40 cases in low-risk group, and the median survival time was 5.279 years. The Kaplan-Meier curve suggests that patients with higher risk scores had a lower prognosis than those in the lower risk score group (Figure 3A,3B). Subsequently, we used ROC curve for model evaluation. The AUC was satisfactory at 0.76. As can be seen in Figure 3C, we can conclude that the model can accurately predict patient prognosis.
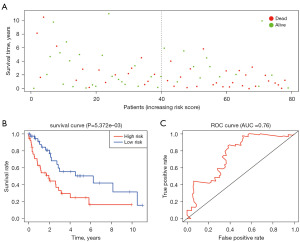
Validation of the prognosis model
To further verify the stability of this model, the patients included in this study were randomly divided into a test set A and a test set B, and served as test and validation sets of the model, respectively. The survival curve and ROC curve were drawn respectively. The results showed that patients in the high-risk group had worse prognosis (Figure 4A,4B). For the ROC curve, the AUC in the test set A and test set B are 0.741 and 0.632 respectively (Figure 4C,4D). It is further proved that the model has a high degree of fit and repeatability, and can accurately predict the prognosis of patients.
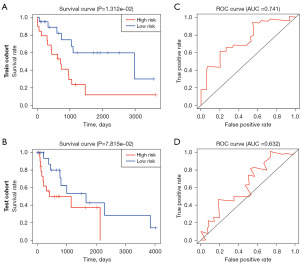
Construction of the nomogram
As was shown in Figure 5, we focused on the following three types of immune cells: NK cells resting, monocytes, and macrophages M2. We established a nomogram model to predicate 3- and 5-year survival rate. According to the nomogram, we can determine the scores corresponding to the relative expression content of the three types of immune cells. And then we calculate the total scores of NK cells resting, monocytes, and macrophages M2. Based on the sum of the three scores obtained, we can predict the 3- and 5-year survival rate of patients with advanced LUSC based on the percentage corresponding to the nomogram.
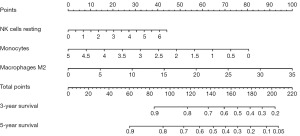
Discussion
LUSC accounts for about 25% of NSCLC (4), and the study of TME is of great value. In the analysis of the TCGA database, the subset of LUSC is characterized by a shorter survival period with more intensive inflammatory mononuclear (IM) cell infiltration (23). Through the high expression of factor XIIIA, IMs promote fibrin cross-linking to form LUSC stents and lead to invasion and metastasis of tumors, and result in poor prognosis of patients (23,24). An in-depth analysis of tumor samples from 101 LUSCs and 87 LUADs enabled Seo et al. to demonstrate that for LUSCs, TAMs play a crucial role in the TME, the immune subtypes of lung cancer and the effect on the TME can be used as an evaluation index for predicting the prognosis in lung cancer patients (25). Previous study has shown that the greater the number of helper T follicles in the LUSC cells, the better the prognosis; the higher the number of neutrophils, the worse the prognosis (26). Another analysis of immune infiltration in 1,430 NSCLC patients showed that CD4 T helper cells and resting mast cells were positively correlated with OS, while active dendritic cells and M2 macrophages showed the opposite result (27). Resting mast cells, resting CD4 T cells, and memory B cells were positively correlated with the survival rate (27). Smoking was common among patients with LUSC; the proportion of TFH cells, regulatory T cells, M2 macrophages, neutrophils, and fractions of plasma cells were higher among smokers, but the survival period was relatively shorter (27). Qu et al. argued that there was no significant difference in the OS of LUSC patients with stromal or immune scores (28). Most squamous cell carcinomas are associated with smoking. The proportion of regulatory T cells, TFH cells, neutrophils, and M2 macrophages is higher among smokers, and the survival rate is shorter (28). Qu et al. showed that the survival time of natural CD4 T cells and regulatory T cells was shorter in squamous cell carcinoma (28). Xu et al. analyzed the chemotherapy response and immune response in LUSC patients and concluded that patients with high TFH cell risk scores have a superior immune response, which can be used to predict the prognosis of LUSC patients and identify new therapeutic targets (3). TFH cells initiate the related reactions of foreign molecules and germinal centers via B cells to participate in the body’s immune response. For LUSC patients, the TFH cells may improve survival by lowering immunosuppression. Neutrophils are present in LUAD, which is positively correlated with their high invasiveness, while LUSC is lower but negatively correlated (26). Other predictors were reported in the literature, for example, Xu et al. screened out CSN1S1, MUC22 and KLK6 as specific prognostic indicators (21). In patients with high-risk factor scores, macrophages M1 and monocytes increased, and TFH cells and T cells CD8 decreased (21). This group of tumor mutational burden has a higher expression; this raises the sensitivity of immunotherapy and chemotherapy. TP53 is another effective gene and can be used as an unresolved therapeutic target and prognostic indicator (21). Zhang et al. used the Lasso-Cox method to screen 6 hot spot mutations, including EGFR, TP53, and PIK3CA to establish a survival time model for NSCLC patients (29). As per multivariate regression analysis, the independent predictors of patients with malignant solid solitary pulmonary nodules include previous history of the tumor, family history, age, boundary conditions, presence/absence of burrs, lobulation, calcification, and air bronchial signs (30). For melanoma patients, the use of anti-PD1 antibodies can change CD8+ from IM to CT. In tumor cells with low expression of major histocompatibility complex (MHC), recombinant IL15, autologous NK cell transfer or KIR blocking antibody can encourage the elimination of MHC-negative tumor cells and the activation of NK cells. With chemotherapy and radiotherapy, the immune microenvironment of the patient changes, creating a suitable environment related to the release of immunogenic molecules by tumor cells that occur to adapt to the bodily changes (24). For the prognosis of NSCLC patients, analyses of M2 macrophages, as well as programmed cell death ligand-1 (PD-L1), can improve the accuracy of the prediction model (31). In lung carcinoma, cancer lymphocyte infiltration is closely related to cancer survival. In adenocarcinoma, wild-type (WT) TP53 female patients have higher levels of tumor immune infiltration. The prognosis of cancer patients is further confirmed to be related to immunity (32).
With screening and systematic analyses and evaluation of the gene expression data in the TCGA database, we found that different immune infiltration patterns of different immune cell subpopulations in advanced LUSC are closely related to patient survival. For normal tissues and cancer tissues, infiltration types of immune cell subsets and corresponding proportions differ obviously. Thus, we applied a metagene deconvolution algorithm, CIBERSORT, to calculate the proportion of 22 immune cell subsets in the tumor transcriptome. We conducted a comprehensive analysis and summary of the effect of tumor-infiltrating immune cells on the prognosis of patients with advanced LUSC.
Based on the above results, it is easy to observe a significant difference in the infiltration pattern between normal lung tissue and advanced LUSC tissue. Moreover, we compared the infiltration rate of normal tissue and lung cancer tissue. Thus, most immune cells differ significantly in the two groups. Univariate Cox HR regression analysis of immune cells in 79 patients with advanced lung cancer showed that the M2 subpopulation of macrophages was positively correlated with patient prognosis. Although the P value was non-significant (P>0.05), the activation of monocytes, mast cells, and NK cell quiescence also exert a certain effect on the prognosis. Subsequently, we analyzed the common effects produced by macrophage M2, monocytes, mast cell activation, and NK cell resting cells using a multivariate Cox regression model. After repeated validation and optimization, the top three meaningful immune cells were selected to build the predicted model. Finally, we used the ROC curve to evaluate the model with an AUC of 0.76, suggesting a good evaluation value. Kaplan-Meier curve showed that patients with high-risk scores had significantly worse OS. In sum, this model can accurately predict patient prognosis. Combining with the results of the multi-factor analysis, a nomogram model of NK cells, monocytes, and macrophages M2 was established for more accurate determination of the 3- and 5-year OS of patients with advanced LUSC. Owing to the relatively smaller sample size used in the gene database screening, this experimental study has certain errors and biases, and there is no better distinction between different races. However, this model has good value for the preliminary survival rate estimation in patients and provides patients with a better prediction of disease progression.
Conclusions
In sum, we comprehensively analyzed and evaluated 22 types of tumor-infiltrating immune cells from advanced LUSC, revealing the correlation between immune cell infiltration and OS in clinical patients. These findings have important clinical significance and value for the prognosis assessment of patients based on the three types of immune cells. Based on the nomogram of NK cells resting, monocytes, and macrophages M2, we can make specific prognostic predictions for advanced LUSC patients.
Acknowledgments
Funding: This work was supported by
Footnote
Reporting Checklist: The authors have completed the TRIPOD reporting checklist. Available at https://tcr.amegroups.com/article/view/10.21037/tcr-23-545/rc
Peer Review File: Available at https://tcr.amegroups.com/article/view/10.21037/tcr-23-545/prf
Conflicts of Interest: All authors have completed the ICMJE uniform disclosure form (available at https://tcr.amegroups.com/article/view/10.21037/tcr-23-545/coif). The authors have no conflicts of interest to declare.
Ethical Statement: The authors are accountable for all aspects of the work in ensuring that questions related to the accuracy or integrity of any part of the work are appropriately investigated and resolved. The study was conducted in accordance with the Declaration of Helsinki (as revised in 2013).
Open Access Statement: This is an Open Access article distributed in accordance with the Creative Commons Attribution-NonCommercial-NoDerivs 4.0 International License (CC BY-NC-ND 4.0), which permits the non-commercial replication and distribution of the article with the strict proviso that no changes or edits are made and the original work is properly cited (including links to both the formal publication through the relevant DOI and the license). See: https://creativecommons.org/licenses/by-nc-nd/4.0/.
References
- Siegel RL, Miller KD, Fuchs HE, et al. Cancer statistics, 2022. CA Cancer J Clin 2022;72:7-33. [Crossref] [PubMed]
- Christian WJ, Vanderford NL, McDowell J, et al. Spatiotemporal Analysis of Lung Cancer Histological Types in Kentucky, 1995-2014. Cancer Control 2019;26:1073274819845873. [Crossref] [PubMed]
- Xu F, Zhang H, Chen J, et al. Immune signature of T follicular helper cells predicts clinical prognostic and therapeutic impact in lung squamous cell carcinoma. Int Immunopharmacol 2020;81:105932. [Crossref] [PubMed]
- Zhao X, Wang J. KEYNOTE-407: new hope for the treatment of lung squamous cell carcinoma. Transl Lung Cancer Res 2020;9:418-20. [Crossref] [PubMed]
- Bhargava A, Mishra DK, Tiwari R, et al. Immune cell engineering: opportunities in lung cancer therapeutics. Drug Deliv Transl Res 2020;10:1203-27. [Crossref] [PubMed]
- Banat GA, Tretyn A, Pullamsetti SS, et al. Immune and Inflammatory Cell Composition of Human Lung Cancer Stroma. PLoS One 2015;10:e0139073. [Crossref] [PubMed]
- Givechian KB, Garner C, Benz S, et al. An immunogenic NSCLC microenvironment is associated with favorable survival in lung adenocarcinoma. Oncotarget 2019;10:1840-9. [Crossref] [PubMed]
- Remark R, Becker C, Gomez JE, et al. The non-small cell lung cancer immune contexture. A major determinant of tumor characteristics and patient outcome. Am J Respir Crit Care Med 2015;191:377-90. [Crossref] [PubMed]
- Seo JS, Lee JW, Kim A, et al. Whole Exome and Transcriptome Analyses Integrated with Microenvironmental Immune Signatures of Lung Squamous Cell Carcinoma. Cancer Immunol Res 2018;6:848-59. [Crossref] [PubMed]
- Yang L, Wei S, Zhang J, et al. Construction of a predictive model for immunotherapy efficacy in lung squamous cell carcinoma based on the degree of tumor-infiltrating immune cells and molecular typing. J Transl Med 2022;20:364. [Crossref] [PubMed]
- Bremnes RM, Busund LT, Kilvær TL, et al. The Role of Tumor-Infiltrating Lymphocytes in Development, Progression, and Prognosis of Non-Small Cell Lung Cancer. J Thorac Oncol 2016;11:789-800. [Crossref] [PubMed]
- Giraldo NA, Becht E, Remark R, et al. The immune contexture of primary and metastatic human tumours. Curr Opin Immunol 2014;27:8-15. [Crossref] [PubMed]
- Guo L, Yi J, Liu M, et al. The prognostic landscape of tumor-infiltrating immune cells in lung squamous cell carcinoma. Ann Transl Med 2021;9:799. [Crossref] [PubMed]
- Fridman WH, Pagès F, Sautès-Fridman C, et al. The immune contexture in human tumours: impact on clinical outcome. Nat Rev Cancer 2012;12:298-306. [Crossref] [PubMed]
- Sica A, Bronte V. Altered macrophage differentiation and immune dysfunction in tumor development. J Clin Invest 2007;117:1155-66. [Crossref] [PubMed]
- Jang HJ, Lee HS, Ramos D, et al. Transcriptome-based molecular subtyping of non-small cell lung cancer may predict response to immune checkpoint inhibitors. J Thorac Cardiovasc Surg 2020;159:1598-1610.e3. [Crossref] [PubMed]
- Meraz IM, Majidi M, Cao X, et al. TUSC2 Immunogene Therapy Synergizes with Anti-PD-1 through Enhanced Proliferation and Infiltration of Natural Killer Cells in Syngeneic Kras-Mutant Mouse Lung Cancer Models. Cancer Immunol Res 2018;6:163-77. [Crossref] [PubMed]
- Jiang G, Zhang M, Tan Q, et al. Identification of the BRAF V600E mutation in a patient with sclerosing pneumocytoma: A case report. Lung Cancer 2019;137:52-5. [Crossref] [PubMed]
- Kinoshita T, Kudo-Saito C, Muramatsu R, et al. Determination of poor prognostic immune features of tumour microenvironment in non-smoking patients with lung adenocarcinoma. Eur J Cancer 2017;86:15-27. [Crossref] [PubMed]
- Wan H, Liu Z, Tan X, et al. Application of immune cell infiltration in the diagnosis and prognosis of non-small cell lung cancer. Sheng Wu Gong Cheng Xue Bao 2020;36:740-9. [PubMed]
- Xu F, Lin H, He P, et al. A TP53-associated gene signature for prediction of prognosis and therapeutic responses in lung squamous cell carcinoma. Oncoimmunology 2020;9:1731943. [Crossref] [PubMed]
- Zuo S, Wang L, Wen Y, et al. Identification of a universal 6-lncRNA prognostic signature for three pathologic subtypes of renal cell carcinoma. J Cell Biochem 2019;120:7375-85. [Crossref] [PubMed]
- Porrello A, Leslie PL, Harrison EB, et al. Factor XIIIA-expressing inflammatory monocytes promote lung squamous cancer through fibrin cross-linking. Nat Commun 2018;9:1988. [Crossref] [PubMed]
- Becht E, Giraldo NA, Dieu-Nosjean MC, et al. Cancer immune contexture and immunotherapy. Curr Opin Immunol 2016;39:7-13. [Crossref] [PubMed]
- Seo JS, Kim A, Shin JY, et al. Comprehensive analysis of the tumor immune micro-environment in non-small cell lung cancer for efficacy of checkpoint inhibitor. Sci Rep 2018;8:14576. [Crossref] [PubMed]
- Liu X, Wu S, Yang Y, et al. The prognostic landscape of tumor-infiltrating immune cell and immunomodulators in lung cancer. Biomed Pharmacother 2017;95:55-61. [Crossref] [PubMed]
- Tamminga M, Hiltermann TJN, Schuuring E, et al. Immune microenvironment composition in non-small cell lung cancer and its association with survival. Clin Transl Immunology 2020;9:e1142. [Crossref] [PubMed]
- Qu Y, Cheng B, Shao N, et al. Prognostic value of immune-related genes in the tumor microenvironment of lung adenocarcinoma and lung squamous cell carcinoma. Aging (Albany NY) 2020;12:4757-77. [Crossref] [PubMed]
- Zhang W, Lin X, Li X, et al. Survival prediction model for non-small cell lung cancer based on somatic mutations. J Gene Med 2020;22:e3206. [Crossref] [PubMed]
- Yu W, Ye B, Xu L, et al. Establishment of A Clinical Prediction Model of Solid Solitary Pulmonary Nodules. Zhongguo Fei Ai Za Zhi 2016;19:705-10. [PubMed]
- Cao L, Che X, Qiu X, et al. M2 macrophage infiltration into tumor islets leads to poor prognosis in non-small-cell lung cancer. Cancer Manag Res 2019;11:6125-38. [Crossref] [PubMed]
- Freudenstein D, Litchfield C, Caramia F, et al. TP53 Status, Patient Sex, and the Immune Response as Determinants of Lung Cancer Patient Survival. Cancers (Basel) 2020;12:1535. [Crossref] [PubMed]