Survival trends and conditional survival in primary non-metastatic esophageal cancer: a SEER population-based study and external validation
Highlight box
Key findings
• Conditional survival (CS) is more dynamic for long-term survivors of primary non-metastatic esophageal cancer (nMEC).
What is known and what is new?
• CS rates improved over time and became stable after a few years.
• The nomogram model is effective in predicting survival trends.
What is the implication, and what should change now?
• This study highlights the importance of CS nomogram in predicting survival trends for patients with nMEC in clinical practice.
Introduction
Esophageal cancer (EC) is a highly aggressive type of cancer that is a leading cause of cancer mortality globally. In 2018, there were an estimated 17,290 new cases and 15,850 deaths caused by primary EC in the United States (1). Despite improvements in treatment, the 5-year survival rate for primary EC is only 20% in many countries (2). Non-metastatic esophageal cancer (nMEC) accounts for approximately half of all EC cases, but 25% of nMEC patients eventually develop distant metastasis even after standard treatment (3). Clinicians often rely on the tumor node metastasis (TNM) staging system of the American Joint Committee on Cancer (AJCC) to predict survival in patients with primary nMEC (4). However, the TNM system has limited predictive performance.
Conditional survival (CS), which is the probability of surviving for a given period of time assuming that a patient has already survived for a specific period, has gained widespread use in recent years (5-7). CS can quantitatively reflect the risk of death in the remaining living population. Many studies have analyzed the trend of CS over time in various cancers, but the prediction of CS has largely been ignored (8-10). Nomograms, which are predictive models that can assess the risk of death or recurrence in many diseases, have become a popular tool for risk assessment (11,12). However, to the best of our knowledge, nomograms predicting CS in primary nMEC patients have not been well studied.
In this study, we used data from the Surveillance, Epidemiology, and End Results (SEER) database, which covers approximately 30% of the population in the United States (13), to conduct a CS analysis of primary nMEC patients. The aim of this analysis was to observe the dynamic survival trends in these patients. We also explored independent predictive factors of CS for cancer-specific survival (CSS) in nMEC patients and developed nomograms to predict CS, which were then validated internally and externally. We present this article in accordance with the TRIPOD reporting checklist (available at https://tcr.amegroups.com/article/view/10.21037/tcr-23-185/rc).
Methods
SEER data source
The SEER database contains data from 18 registries in the United States covering the period from 1973 to 2014. The data for this study were obtained using SEER*Stat software. All personal information in the SEER database was de-identified. We randomly divided the included patients into a training cohort and an internal validation cohort in a 9:1 ratio.
Inclusion and exclusion criteria
In this study, we included patients who met the following criteria: (I) diagnosed with primary EC (anatomic ICD-O-3 code: C15.0-15.9); (II) diagnosed between 2004 and 2014; (III) aged 18 years or older at diagnosis; (IV) limited to adenocarcinoma (histologic ICD-O-3 code: 8140-8389) or squamous cell carcinoma (histologic ICD-O-3 code: 8050-8089); (V) M0 status (no distant metastasis).
We excluded patients who met the following criteria: (I) unknown demographic information such as gender, age at diagnosis, race, and marital status; (II) unknown clinicopathologic information such as cancer site, histologic grade, AJCC stage, and TNM status; (III) unknown surgery or radiotherapy information; (IV) unknown survival month; (V) surviving for less than or equal to one month; (VI) with only death certificate or autopsy follow-up type.
Variable selection
We collected patient data from the SEER database, including demographic information such as gender, age, marital status, race, and cancer site; clinicopathologic information such as histology, histologic grade, and TNM stage; and treatment information such as surgical therapy, radiotherapy, and chemotherapy. We classified gender as male or female, age as 18–65 years (young group) or older than 65 years (old group), marital status as married or unmarried (including divorced, separated, single, or widowed patients), race as white or black, cancer site as lower third or other (including upper third, middle third, and overlapping lesion of the esophagus), histology as adenocarcinoma or squamous cell carcinoma, histologic grade as grade I–II or III–IV, TNM stage as stage I, II, or III, T status as T1–2 or T3–4, and N status as N0 or N1. We also classified whether patients received surgical treatment, radiotherapy, or chemotherapy. Deaths caused by EC were considered cancer-specific death events.
Validation cohort
We validated the nomogram using both an internal validation cohort (a subset of the included patients from the SEER database) and an external validation cohort. The external validation cohort consisted of 37 patients with primary nMEC who were diagnosed between July 2016 and July 2019 at the First Affiliated Hospital of Shantou University Medical College. We retrospectively collected necessary demographic, histopathological, treatment, and follow-up data for these patients. During external validation, we did not consider the race variable because all patients in this cohort were Asian. The study was conducted in accordance with the Declaration of Helsinki (as revised in 2013). The study was approved by the ethics committee of the First Affiliated Hospital of Shantou University Medical College (No. 2022-223) and individual consent for this retrospective analysis was waived.
Statistical analysis
We performed statistical analysis using R software v4.2.1. All P values were two-sided, and P<0.05 was considered statistically significant. We described continuous variables with skewed distributions as medians, first quartiles, and third quartiles, and described categorical variables as frequencies and percentages. For categorical variables, we used Pearson’s chi-squared test to detect statistical significance. The log-rank test and the multivariable Cox proportional hazard model were used to identify independent risk factors for CSS. The additive model test was used to detect statistical interactions between two variables in multivariable Cox analyses, including synergism and antagonism. We estimated the cumulative CSS probabilities using the Kaplan-Meier method. CS (y|x) was defined as the probability of surviving an additional number of years (y years) given that the patient had survived a specified number of years (x years). To calculate the y-year CS for patients surviving x years, we divided the (x+y)-year CSS by the x-year CSS. Standard errors were calculated using Davis’s method (14).
The nomogram models were constructed using the training cohort (Figure S1). For the CS nomograms, we used the forward variable selection method of Cox regression to select predictive factors based on independent prognostic factors. We calculated CS and then established the CS nomograms. The accuracies of the clinical CS nomograms were validated in the training, internal validation, and external validation cohorts using 500-resample bootstrapping. We used the calibration curve and Harrell’s concordance index (C-index) to evaluate the performance of the nomograms. The calibration curve shows the relationship between the predictive estimates of the models and the actual observations. The ideal predictions, known as the standard curve, should fall on the 45-degree straight line through the origin. The C-index represents the quantitative predictive performance of the model and ranges from 0.500 (representing random chance) to 1.000 (representing perfect discrimination). Additionally, we conducted 10-fold cross-validation in the included non-randomly grouped SEER patients to reduce the arbitrariness of data partitioning.
Results
We finally included 9,008 patients with primary nMEC in our study, which we randomly divided into 8,107 patients in the training cohort and 901 patients in the internal validation cohort (Figure 1). Over half of the patients received surgery (52.3%), chemotherapy (69.5%), and radiotherapy (69.7%). There were no statistically significant differences in any variables between the two groups (Table 1). The median follow-up period was 17.00 (8.00–38.00) months. Table S1 displays the baseline characteristics of the external validation cohort, which had a median follow-up period of 21.0 (13.0–35.0) months.
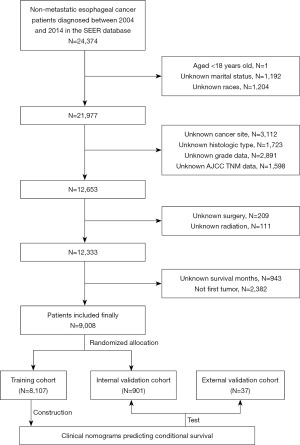
Table 1
Characteristic | Training cohort (N=8,107) | Validation cohort (N=901) | Total (N=9,008) | P value |
---|---|---|---|---|
Gender | 0.466 | |||
Male | 6,453 (79.6) | 727 (80.7) | 7,180 (79.7) | |
Female | 1,654 (20.4) | 174 (19.3) | 1,828 (20.3) | |
Age | 0.844 | |||
Young | 3,740 (46.1) | 412 (45.7) | 4,152 (46.1) | |
Old | 4,367 (53.9) | 489 (21.9) | 4,856 (53.9) | |
Marriage | 0.370 | |||
Married | 4,900 (60.4) | 559 (62.0) | 5,459 (60.6) | |
Unmarried | 3,207 (39.6) | 342 (38.0) | 3,549 (39.4) | |
Race | 0.993 | |||
White | 7,258 (89.5) | 806 (89.5) | 8,064 (89.5) | |
Black | 849 (10.5) | 95 (10.5) | 944 (10.5) | |
Site | 0.751 | |||
Lower one third | 5,848 (72.1) | 655 (72.7) | 6,503 (72.2) | |
Others | 2,259 (27.9) | 246 (27.3) | 2,505 (27.8) | |
Histology | 0.782 | |||
Adenocarcinoma | 5,611 (69.2) | 619 (68.7) | 6,230 (69.2) | |
Squamous cell carcinoma | 2,496 (30.8) | 282 (31.3) | 2,778 (30.8) | |
Grade | 0.746 | |||
Grade I/II | 4,385 (54.1) | 493 (54.7) | 4,878 (54.2) | |
Grade III/IV | 3,722 (45.9) | 408 (45.3) | 4,130 (45.8) | |
AJCC | 0.314 | |||
I | 2,083 (25.7) | 227 (25.2) | 2,310 (25.6) | |
II | 2,911 (35.9) | 346 (38.4) | 3,257 (36.2) | |
III | 3,113 (38.4) | 328 (36.4) | 3,441 (38.2) | |
AJCC-T | 0.833 | |||
T1–T2 | 3,877 (47.8) | 427 (47.4) | 4,304 (47.8) | |
T3–T4 | 4,230 (52.2) | 474 (52.6) | 4,704 (52.2) | |
AJCC-N | 0.423 | |||
N0 | 4,173 (51.5) | 477 (52.9) | 4,650 (51.6) | |
N1 | 3,934 (48.5) | 424 (47.1) | 4,358 (48.4) | |
Surgical treatment | 0.578 | |||
Yes | 4,250 (52.4) | 463 (51.4) | 4,713 (52.3) | |
No | 3,857 (47.6) | 438 (48.6) | 4,295 (47.7) | |
Chemotherapy | 0.334 | |||
Yes | 5,618 (69.3) | 639 (70.9) | 6,257 (69.5) | |
No/unknown | 2,489 (30.7) | 262 (29.1) | 2,751 (30.5) | |
Radiotherapy | 0.234 | |||
Yes | 5,634 (69.5) | 644 (71.5) | 6,278 (69.7) | |
No | 2,473 (30.5) | 257 (28.5) | 2,730 (30.3) |
All the variables are described as frequencies and percentages; Pearson’s chi-squared test was used to detect the statistical significance. AJCC, the American Joint Committee on Cancer; T/N, tumor/node.
In general, the 1-year CSS rate was 69.6%, and the 3-year CSS rate was 43.1% (Figure 2). The log-rank test and Cox analysis found that age, marital status, race, grade, T stage, N stage, surgery, and chemotherapy were associated with CSS outcomes in patients with primary nMEC (Figure 3, Table 2). Specifically, patients who did not receive surgery [hazard ratio (HR) =2.786, 95% confidence interval (CI): 2.609–2.975, P<0.001] and those with advanced T stage (HR =1.560, 95% CI: 1.459–1.667, P<0.001) had poorer CSS outcomes.
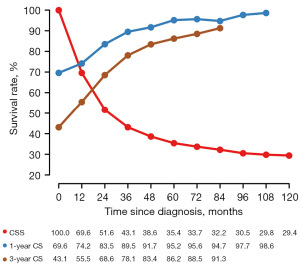
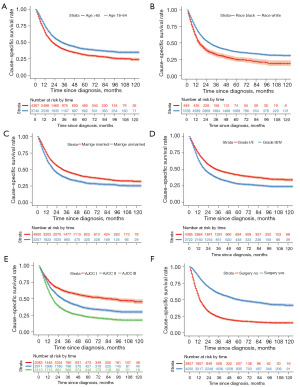
Table 2
Characteristics | Kaplan-Meier analysis | Multivariable Cox analysis | ||||
---|---|---|---|---|---|---|
1-year | 3-year | P value | HR (95% CI) | P value | ||
Gender | 0.062 | |||||
Male | 70.3% | 43.5% | ||||
Female | 66.8% | 41.6% | ||||
Age group | <0.001 | |||||
Young | 74.8% | 47.9% | Reference | |||
Old | 65.1% | 38.8% | 1.141 (1.072–1.214) | <0.001 | ||
Marriage | <0.001 | |||||
Married | 73.2% | 47.1% | Reference | |||
Unmarried | 64.1% | 36.7% | 1.157 (1.088–1.232) | <0.001 | ||
Race | <0.001 | |||||
White | 71.0% | 44.4% | Reference | |||
Black | 57.7% | 32.1% | 1.149 (1.045–1.262) | 0.004 | ||
Site | <0.001 | |||||
Lower 1/3 | 72.6% | 45.8% | ||||
Others | 61.7% | 36.1% | ||||
Histology | <0.001 | |||||
Adenocarcinoma | 73.5% | 45.6% | ||||
Squamous cell carcinoma | 60.7% | 37.4% | ||||
Grade | <0.001 | |||||
Grade I/II | 74.3% | 48.9% | Reference | |||
Grade III/IV | 64.1% | 36.4% | 1.357 (1.278–1.441) | <0.001 | ||
AJCC | <0.001 | |||||
I | 77.3% | 59.8% | ||||
II | 72.1% | 45.1% | ||||
III | 62.1% | 29.8% | ||||
AJCC-T | <0.001 | |||||
T1–T2 | 75.1% | 53.3% | Reference | |||
T3–T4 | 64.6% | 33.5% | 1.560 (1.459–1.667) | <0.001 | ||
AJCC-N | <0.001 | |||||
N0 | 73.1% | 52.0% | Reference | |||
N1 | 65.9% | 33.6% | 1.405 (1.315–1.501) | <0.001 | ||
Surgical treatment | <0.001 | |||||
Yes | 84.6% | 59.6% | Reference | |||
No | 52.6% | 23.8% | 2.786 (2.609–2.975) | <0.001 | ||
Chemotherapy | <0.001 | |||||
Yes | 70.4% | 40.6% | Reference | |||
No/unknown | 67.7% | 48.6% | 1.465 (1.362–1.575) | <0.001 | ||
Radiotherapy | <0.001 | |||||
Yes | 68.6% | 38.7% | ||||
No | 71.9% | 52.7% |
AJCC, the American Joint Committee on Cancer; T/N, tumor/node; HR, hazard ratio; CI, confidence interval.
Initially, the 1- and 3-year CS rates at diagnosis, also known as traditional 1- and 3-year CSS rates, were 69.6% and 43.1%. The 1-year CS rates gradually increased to 74.2% at the first conditional year since diagnosis, 83.5% at the second year, 89.5% at the third year, 91.7% at the fourth year, and 95.2% at the fifth year. After that, the 1-year CS rates became stable, which was similar to the rate at the fifth year (Figure 2). This pattern also applied to the 3-year CS rates, which continuously improved over time and eventually became stable at the fifth conditional year since diagnosis, with a rate of 86.2% (Figure 2). The CS rates of primary nMEC patients according to different variables increased over time, particularly in subgroups with lower initial CSS rates at diagnosis (Figure 4). For example, a significant improvement in CS rates was observed in patients with T3–4, with CS rates increasing from 33.5% at diagnosis to 79.8% at 5 years.
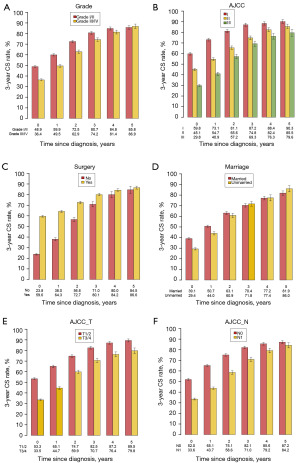
The nomogram model was developed using the proportional hazard model, and it is presented in Figure 5. As shown in Figure 5A and Table 3, each patient’s characteristics correspond to a certain number of points. The total score was obtained by adding up the points for all characteristics. To predict the 1- and 3-year CS probabilities given a certain time since diagnosis for a patient, we used the patient’s total score and survival time based on Figure 5B,5C.
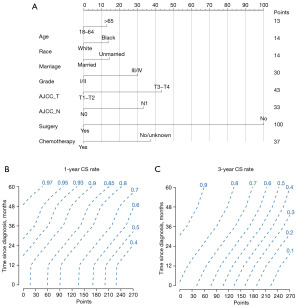
Table 3
Characteristics | Scores |
---|---|
Age group | |
Young | 0 |
Old | 13 |
Marriage | |
Married | 0 |
Unmarried | 14 |
Race | |
White | 0 |
Black | 14 |
Grade | |
I/II | 0 |
III/IV | 30 |
AJCC-T | |
T1–T2 | 0 |
T3–T4 | 43 |
AJCC-N | |
N0 | 0 |
N1 | 33 |
Surgical treatment | |
Yes | 0 |
No | 100 |
Chemotherapy | |
Yes | 0 |
No/unknown | 37 |
AJCC, the American Joint Committee on Cancer; T/N, tumor/node.
The calibration curves for 1- and 3-year CS prediction were close to the standard curve, indicating that the nomograms had good predictive ability in the training, internal, and external validation cohorts (Figure 6). The C-indexes were 0.700 (95% CI: 0.693–0.709) in the training cohort, 0.693 (95% CI: 0.669–0.717) in the internal validation cohort, and 0.683 (95% CI: 0.556–0.810) in the external validation cohort. The 1-year AUC was 0.755 (range, 0.735–0.761) for 10-fold cross-validation, and the 3-year AUC was 0.769 (range, 0.730–0.812), respectively.
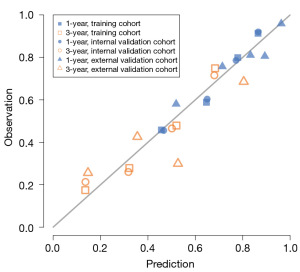
Discussion
In our retrospective cohort study, we analyzed data from the population-based SEER database to investigate the dynamic survival trends and CS in patients with primary nMEC. We found that the CS rates improved over time and reached a stable level by the fifth year, both for the overall study population and for specific subgroups. We also observed that the CS rates for subgroups with lower initial survival rates showed a more pronounced increase over time. We identified independent prognostic factors and used them to create nomograms for predicting CS in primary nMEC patients. The nomograms performed well in internal and external validation, with a strong predictive power.
Conditional analysis has become increasingly popular in oncology research (15,16). Unlike traditional survival statistics, which treat survival rate as a static index, CS more clearly shows the change in survival rate over time (17). CS quantitatively displays the dynamic trend of survival risks as time progresses from diagnosis, better reflecting the reality of cancer patients. Fukui et al. found that CS probabilities provide more meaningful information than traditional cumulative survival in patients with resected non-small cell lung cancer, and patients require a postoperative follow-up visit for three years (18). In patients with anal cancer, CS displays improvement over time after diagnosis, with those in advanced stages tending to experience the greatest improvement in CS (19).
Nomograms are visualization tools for predicting linear statistical models. They have been widely used in biomedical studies and have been shown to have more predictive power than a single clinicopathologic factor (20). However, there are few studies that have developed nomograms for CS probability. Thuret et al. developed and externally validated a conditional nomogram for predicting cancer-specific mortality in patients with squamous cell carcinoma of the penis (21). A robust nomogram for predicting survival in gastric cancer patients who are alive at certain time points after surgery has also been developed (22). There are no studies that have established clinical CS nomograms for primary nMEC.
Clinical nomograms that predict CS are important for both clinicians and patients (23,24). For example, consider a patient with primary nMEC who has been alive for several years and now comes to a clinician for help. At this point, the patient is more concerned about their survival risk from this point forward, rather than the traditional risk since diagnosis. One key aspect of our study is the development of CS nomograms that can be easily and quickly applied in clinical practice. These clinical CS nomograms incorporate independent prognostic factors and build predictive models, which have been shown to be more accurate than the single AJCC TNM staging system (25).
In our study, we found that being married was independently associated with long-term survival in patients with primary nMEC, consistent with previous studies (26,27). This may be due to the fact that being married is accompanied by positive emotions and strong social support, which can indirectly encourage patients to fight the disease to some extent (28,29). Cancer grade and stage remained significant predictive factors of CS in nMEC patients (27,30). Specifically, more advanced clinicopathologic factors were associated with poorer survival outcomes. Our nomograms showed that surgical treatment had the largest impact on CS prognosis. Radical surgery is still the most important approach for most solid tumors, including primary nMEC (31,32). However, there was a selection bias in surgical treatment. Patients who received surgical treatment were more likely to be younger, have better performance status, have fewer comorbidities, and respond better to neoadjuvant therapy. These factors could all improve survival in nMEC patients. Chemotherapy is also a critical treatment for nMEC patients (33), which is consistent with our study. Radiotherapy improved the prognosis of primary nMEC patients, but in our study, the protective effect of radiotherapy disappeared.
One of the strongest advantages of this study was the large sample size. As a large population-based analysis of the public SEER database, the 9,008 patients in our study represented the real-world survival of nMEC patients, resulting in reliable results. Another highlight was that we developed nomograms to assist clinicians and patients in easily predicting CS probability, which has been proven to be effective and practical in many other types of cancer (21,22,25). For patients with nMEC, CS rates increased over time, which not only reflects a decrease in mortality risk as time progresses since diagnosis, but also an increase in mental and psychological comfort for the patient. As patients with nMEC survived longer, their prognosis improved. It might be due to a variety of factors including the natural course of the disease, the cumulative effect of treatment, and improvements in the patient’s overall health.
There are some limitations to this study. Firstly, the current public SEER database lacks some important risk factors such as demographic, clinical, and pathologic variables. It also does not provide detailed information on radiotherapy and chemotherapy plans. Some comorbidities and complications that occur during the follow-up period are also not available. These factors should be taken into account to correct for the effect of covariates on outcomes. Secondly, in this study, most of the included variables were converted into bivariate, which resulted in a loss of clinical information contained in these variables. Thirdly, as this was a retrospective cohort study, selection bias is inevitable. Therefore, further studies are needed to confirm our results.
Conclusions
In this retrospective cohort study, we conducted a CS analysis to predict the survival trends in primary nMEC patients. We found that CS rates improved over time and became stable after a few years. CS probabilities are more dynamic and meaningful than traditional survival rates for patients surviving for a relatively longer period. We also developed and validated nomograms to predict CS probabilities.
Acknowledgments
The authors acknowledge the Surveillance, Epidemiology, and End Results database for the creation and maintenance of the database.
Funding: This work was supported by
Footnote
Reporting Checklist: The authors have completed the TRIPOD reporting checklist. Available at https://tcr.amegroups.com/article/view/10.21037/tcr-23-185/rc
Data Sharing Statement: Available at https://tcr.amegroups.com/article/view/10.21037/tcr-23-185/dss
Peer Review File: Available at https://tcr.amegroups.com/article/view/10.21037/tcr-23-185/prf
Conflicts of Interest: All authors have completed the ICMJE uniform disclosure form (available at https://tcr.amegroups.com/article/view/10.21037/tcr-23-185/coif). The authors have no conflicts of interest to declare.
Ethical Statement: The authors are accountable for all aspects of the work in ensuring that questions related to the accuracy or integrity of any part of the work are appropriately investigated and resolved. The study was conducted in accordance with the Declaration of Helsinki (as revised in 2013). The study was approved by the ethics committee of the First Affiliated Hospital of Shantou University Medical College (No. 2022-223) and individual consent for this retrospective analysis was waived.
Open Access Statement: This is an Open Access article distributed in accordance with the Creative Commons Attribution-NonCommercial-NoDerivs 4.0 International License (CC BY-NC-ND 4.0), which permits the non-commercial replication and distribution of the article with the strict proviso that no changes or edits are made and the original work is properly cited (including links to both the formal publication through the relevant DOI and the license). See: https://creativecommons.org/licenses/by-nc-nd/4.0/.
References
- Siegel RL, Miller KD, Jemal A. Cancer statistics, 2018. CA Cancer J Clin 2018;68:7-30. [Crossref] [PubMed]
- Lagergren J, Smyth E, Cunningham D, et al. Oesophageal cancer. Lancet 2017;390:2383-96. [Crossref] [PubMed]
- Enzinger PC, Ilson DH, Kelsen DP. Chemotherapy in esophageal cancer. Semin Oncol 1999;26:12-20. [PubMed]
- Cuccurullo V, Mansi L. AJCC Cancer Staging Handbook: from the AJCC Cancer Staging Manual (7th edition). Eur J Nucl Med Mol Imaging 2010;38:408.
- Merrill RM, Hunter BD. Conditional survival among cancer patients in the United States. Oncologist 2010;15:873-82. [Crossref] [PubMed]
- Tichanek F, Försti A, Hemminki A, et al. Survival in Kidney and Bladder Cancers in Four Nordic Countries through a Half Century. Cancers (Basel) 2023;15:2782. [Crossref] [PubMed]
- Modonutti D, Majdalany SE, Butaney M, et al. Conditional survival does not improve over time in metastatic castration-resistant prostate cancer patients undergoing docetaxel. Prostate 2023;83:1238-46. [Crossref] [PubMed]
- Abdallah NH, Smith AN, Geyer S, et al. Conditional survival in multiple myeloma and impact of prognostic factors over time. Blood Cancer J 2023;13:78. [Crossref] [PubMed]
- Tappero S, Cano Garcia C, Incesu RB, et al. Conditional survival for non-metastatic muscle-invasive adenocarcinoma of the urinary bladder after radical cystectomy. Surg Oncol 2023;48:101947. [Crossref] [PubMed]
- Eisfeld C, Kajüter H, Möller L, et al. Time trends in survival and causes of death in multiple myeloma: a population-based study from Germany. BMC Cancer 2023;23:317. [Crossref] [PubMed]
- Hirabayashi S, Kosugi S, Isobe Y, et al. Development and external validation of a nomogram for overall survival after curative resection in serosa-negative, locally advanced gastric cancer. Ann Oncol 2014;25:1179-84. [Crossref] [PubMed]
- Cheng H, Li J, Wei F, et al. A risk nomogram for predicting prolonged intensive care unit stays in patients with chronic obstructive pulmonary disease. Front Med (Lausanne) 2023;10:1177786. [Crossref] [PubMed]
- Hayat MJ, Howlader N, Reichman ME, et al. Cancer statistics, trends, and multiple primary cancer analyses from the Surveillance, Epidemiology, and End Results (SEER) Program. Oncologist 2007;12:20-37. [Crossref] [PubMed]
- Davis FG, McCarthy BJ, Freels S, et al. The conditional probability of survival of patients with primary malignant brain tumors: surveillance, epidemiology, and end results (SEER) data. Cancer 1999;85:485-91. [Crossref] [PubMed]
- Haydu LE, Scolyer RA, Lo S, et al. Conditional Survival: An Assessment of the Prognosis of Patients at Time Points After Initial Diagnosis and Treatment of Locoregional Melanoma Metastasis. J Clin Oncol 2017;35:1721-9. [Crossref] [PubMed]
- Bischof DA, Kim Y, Dodson R, et al. Conditional disease-free survival after surgical resection of gastrointestinal stromal tumors: a multi-institutional analysis of 502 patients. JAMA Surg 2015;150:299-306. [Crossref] [PubMed]
- Kato I, Severson RK, Schwartz AG. Conditional median survival of patients with advanced carcinoma: surveillance, epidemiology, and end results data. Cancer 2001;92:2211-9. [Crossref] [PubMed]
- Fukui T, Okasaka T, Kawaguchi K, et al. Conditional Survival After Surgical Intervention in Patients With Non-Small Cell Lung Cancer. Ann Thorac Surg 2016;101:1877-82. [Crossref] [PubMed]
- Kim E, Kim JS, Choi M, et al. Conditional Survival in Anal Carcinoma Using the National Population-Based Survey of Epidemiology and End Results Database (1988-2012). Dis Colon Rectum 2016;59:291-8. [Crossref] [PubMed]
- Sun W, Cheng M, Zhou H, et al. Nomogram Predicting Cause-Specific Mortality in Nonmetastatic Male Breast Cancer: A Competing Risk Analysis. J Cancer 2019;10:583-93. [Crossref] [PubMed]
- Thuret R, Sun M, Abdollah F, et al. Conditional survival predictions after surgery for patients with penile carcinoma. Cancer 2011;117:3723-30. [Crossref] [PubMed]
- Dikken JL, Baser RE, Gonen M, et al. Conditional probability of survival nomogram for 1-, 2-, and 3-year survivors after an R0 resection for gastric cancer. Ann Surg Oncol 2013;20:1623-30. [Crossref] [PubMed]
- Thomas CR Jr. Conditional Survival as a Pragmatic Resource for Cancer Survivors and Health Care Professionals. JAMA Oncol 2018;4:1130-1. [Crossref] [PubMed]
- Meng X, Cai Y, Chang X, et al. A novel conditional survival nomogram for monitoring real-time prognosis of non-metastatic triple-negative breast cancer. Front Endocrinol (Lausanne) 2023;14:1119105. [Crossref] [PubMed]
- Zhong Q, Chen QY, Li P, et al. Prediction of Conditional Probability of Survival After Surgery for Gastric Cancer: A Study Based on Eastern and Western Large Data Sets. Surgery 2018;163:1307-16. [Crossref] [PubMed]
- Wikman A, Ljung R, Johar A, et al. Psychiatric morbidity and survival after surgery for esophageal cancer: a population-based cohort study. J Clin Oncol 2015;33:448-54. [Crossref] [PubMed]
- Liu M, Wang C, Gao L, et al. A nomogram to predict long-time survival for patients with M1 diseases of esophageal cancer. J Cancer 2018;9:3986-90. [Crossref] [PubMed]
- Lejeune C, Sassi F, Ellis L, et al. Socio-economic disparities in access to treatment and their impact on colorectal cancer survival. Int J Epidemiol 2010;39:710-7. [Crossref] [PubMed]
- Sun W, Qiu Z, Tan W, et al. The influence of marital status on survival in patients with oral tongue squamous cell carcinoma. Oncotarget 2017;8:82092-102. [Crossref] [PubMed]
- Tang X, Zhou X, Li Y, et al. A Novel Nomogram and Risk Classification System Predicting the Cancer-Specific Survival of Patients with Initially Diagnosed Metastatic Esophageal Cancer: A SEER-Based Study. Ann Surg Oncol 2019;26:321-8. [Crossref] [PubMed]
- Zeng Y, Liang W, Liu J, et al. Esophageal cancer in elderly patients: a population-based study. J Thorac Dis 2018;10:448-57. [Crossref] [PubMed]
- Xie K, Liu S, Liu J. Nomogram predicts survival benefit for non- metastatic esophageal cancer patients who underwent preoperative radiotherapy. Cancer Manag Res 2018;10:3657-68. [Crossref] [PubMed]
- Chen SB, Liu DT, Chen YP. Impact of adjuvant chemotherapy for radically resected esophageal squamous cell carcinoma: a propensity score matching analysis. Front Surg 2023;10:1181505. [Crossref] [PubMed]