Molecular markers that predict response to combined radiotherapy and immunotherapy in patients with lung adenocarcinoma: a bioinformatics analysis
Highlight box
Key findings
• TLR8 and CCR5 expression are associated with responsiveness to combined radiotherapy (RT) and immunotherapy in patients with lung adenocarcinoma (LUAD).
What is known and what is new?
• A combination of immunotherapy and RT stimulates a good systemic immune response in some patients with LUAD; however, little is known about the molecular mechanisms whereby RT influences responsiveness to immunotherapy.
• TLR8 and CCR5 expression increased responsiveness to immunotherapy by promoting M0 macrophage and memory B cell infiltration of LUAD tissues.
What is the implication, and what should change now?
• Although these findings need to be confirmed by prospective studies, measuring TLR8 and CCR5 expression could enable precision therapy in patients with LUAD.
Introduction
Background
Lung cancer has one of the highest morbidity and mortality rates worldwide. The most common histological type of non-small cell lung cancer (NSCLC) is lung adenocarcinoma (LUAD). Depending on the molecular type, different therapies would be recommended on the treatment of LUAD. These therapeutic strategies include surgery, chemotherapy, radiotherapy (RT), and targeted therapy or a combination of these treatments (1,2). However, the prognosis of advanced LUAD is extremely poor, resulting in a five-year survival rate of 10–15%. Recent advances in immune checkpoint therapies have had unprecedented success and have, thus, become an important LUAD treatment modality (3-5).
Despite the success of anti-programmed death ligand-1 (PD-L1) agents in a subset of patients with LUAD, a sizable portion of patients do not respond to immune checkpoint inhibitors (ICIs) (6). Recent study has demonstrated that the tumor microenvironment (TME) and metabolism induce resistance to immunotherapies (7). However, RT can restore the TME and alter metabolism, thereby affecting the response to immune treatment (8). Combining immunotherapy and RT has been found to enhance antitumor effects in several clinical trials conducted recently (9,10). Patients with NSCLC can benefit from combination of RT and pembrolizumab. The same conclusion was reached in durvalumab and anti-cytotoxic T-lymphocyte-associated antigen 4 (CTLA-4) therapy (11-13).
Rationale and knowledge gap
The biological mechanisms of this combination are currently under investigation. Wang et al. (14) reported that RT can restore the TME and alter the immunophenotype of cancer. RT releases many cytokines and activates the body’s immune response. In addition, RT plays an immunostimulatory role by increasing T cell cytotoxicity, natural killer (NK) cell activation, and tumor-associated M1 macrophage levels; reducing the release of regulatory T cell lymphocytes; inhibiting the PD-1/PD-L1 and CTLA-4 pathways; activating the Fas/interferon gamma (IFN-γ) pathway (15-17); and inducing immune cell infiltration into the tumor (18,19). The effectiveness of certain immunotherapies is affected by tumor-infiltrating lymphocytes (TILs) in the TME (20). Conspicuous lymphocytic infiltration is frequently associated with better prognosis in patients with different tumors, such as melanoma, colorectal cancer, and NSCLC (21). Thus, TIL status has been proposed as a biomarker for checkpoint inhibitor immunotherapy.
Objective
In this study, we aimed to identify new biomarkers and related signaling pathways that influence cancer prognosis after immune therapies by modulating the TME involved in RT. Bioinformatics analysis has recently attracted worldwide attention as a method to explore the underlying molecular mechanisms of antitumor therapies. We present this article in accordance with the REMARK reporting checklist (available at https://tcr.amegroups.com/article/view/10.21037/tcr-23-968/rc).
Methods
Data collection
Three microarray datasets (GSE162945, GSE126044, and GSE135222) were downloaded from the Gene Expression Omnibus (GEO) database (http://www.ncbi.nlm.nih.gov/geo/). GSE162945 contains transcriptome RNA-sequencing data of patients with LUAD, before or after SBRT. GSE126044 and GSE135222 datasets contain clinical and transcriptome data of patients with LUAD after immunotherapy. The gene expression profile of LUAD was retrieved from TCGA (https://tcga-data.nci.nih.gov/tcga/). These RNA profiles were based on the GPL18573 (Illumina NextSeq 500) and GPL16791 (Illumina HiSeq 2500) platforms. The study was conducted in accordance with the Declaration of Helsinki (as revised in 2013)
Differentially expressed gene (DEG) analysis
DEG analysis was performed using R v4.0.3 (R Foundation for Statistical Computing, Vienna, Austria). Two-class paired significant microarray analysis was calculated using the limma package to determine DEGs before and after RT. Thresholds of P<0.05 and |logFC| >1 were defined as the screening criteria for DEGs. The DEGs overlapping among the three datasets were analyzed using the VENNY tool (v2.1, http://bioinfogp.cnb.csic.es/tools/venny/index.html).
Functional and pathway enrichment analysis
Functional gene ontology (GO) was performed to classify the DEGs. Cellular components, molecular functions (MFs), and biological processes were included in the GO enrichment analysis. Data from the Kyoto Encyclopedia of Genes and Genomes (KEGG) database (http://www.genome.jp/kegg/) were analyzed using the clusterProfiler R package to identify the pathways in which the candidate mRNAs were involved. The DAVID Bioinformatics Resource (https://david-d.ncifcrf.gov) was used for GO and KEGG pathway enrichment analysis.
Construction of protein-protein interaction (PPI) network
PPIs of DEGs were analyzed using the STRING database (https://string-db.org/) based on the Pearson correlation coefficient (|cor| >0.4, P<0.01). The software Cytoscape v3.8.2 was applied to build the PPI network.
Identification of hub genes
Hub genes were selected using Cytoscape v3.8.2 based on the STRING database, using the maximal clique centrality (MCC) algorithm cytoHubba to build a hub gene network. The five genes with the highest core-gene scores were identified as hub genes.
Gene mutation analysis
Microarray expression data of 586 LUAD samples from TCGA were obtained for DEG mutation analysis from cBioPortal (http://cbioportal.org). The cBioPortal is a publicly accessible tool for visualizing and analyzing multidimensional cancer genomics data.
Relationship between DEGs and survival
Kaplan-Meier survival outcome analysis was performed to determine the association between prognosis-related DEGs using the Kaplan-Meier plotter (http://kmplot.com). To determine the relationship between the DEGs and the effectiveness of immunotherapy, the results were analyzed and plotted using the Prism software (GraphPad, Inc., San Diego, CA, USA). Results with a P<0.05 were regarded as statistically significant.
The relationship between clinical parameters and DEGs
Clinical LUAD data were downloaded from the TCGA database. The association between clinicopathological parameters and gene expression in LUAD was determined using the PERL script (https://www.perl.org/) and R software (The R Foundation for Statistical Computing, Vienna, Austria).
The relationship between DEGs and the immune infiltrate
TIMER (https://cistrome.shinyapps.io/timer/) was applied to determine the potential correlation between the expression of DEGs and various TILs. Additionally, TISIDB (http://cis.hku.hk/TISIDB/), an online database for cancer and immune system interactions, integrating multiple heterogeneous data types and collecting many human cancer datasets from the TCGA database, was used to further explore the relationships between DEGs expression, immune subtypes, and TILs.
The relationship between DEGs and PD-L1 and CTLA-4 expression
Based on TIMER, we investigated the correlation between DEG and PD-L1 mRNA expression in LUAD using cBioPortal (https://www.cbioportal.org).
Statistical analysis
All statistical analyses were performed using the R v4.0.3 and GraphPad Prism 5 (GraphPad Software, San Diego, CA, USA). The distribution of variables that met the screening criteria were compared between groups using Wilcoxon signed-rank tests, Mann-Whitney U tests, t-tests, and logistic regression. Overall survival (OS) was determined using Kaplan-Meier survival analysis. P values <0.05 were regarded as statistically significant.
Results
Identification of DEGs and KEGG enrichment analysis in LUAD before/after SBRT
We analyzed gene expression profiles of LUAD from the GSE162945 dataset before and after SBRT. Of the 2,568 DEGs detected, 1,077 were downregulated, and 1,491 were upregulated. These DEGs were shown in a heatmap (Figure 1A) and volcano plot (Figure 1B). A bar graph of the top ten results from the KEGG enrichment analysis is shown in Figure 1C,1D. Pathway analysis of upregulated DEGs revealed the enrichment of the focal adhesion, protein digestion and absorption, and extracellular matrix-receptor (ECM)-receptor interaction. The downregulated DEGs resulted in significant enrichment of ribosomes, oxidative phosphorylation, thermogenesis, and other tumor-associated pathways.
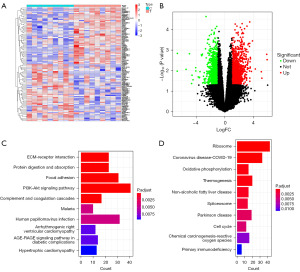
DEGs in tumors with high and low immune infiltration and KEGG enrichment analysis
We performed a DEG analysis using two sets of TIL data (GSE180347 and TCGA data). Of the 793 DEGs detected, 294 were downregulated, and 499 were upregulated. The top 50 DEGs were shown in a heatmap (Figure 2A), and all DEGs were shown in a volcano plot (Figure 2B). Pathway analysis of DEGs revealed the enrichment of viral protein interactions with cytokines, cytokine-cytokine receptor interactions and cytokine receptors, chemokine signaling pathways, and other tumor-associated pathways (Figure 2C).
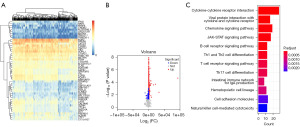
Construction of PPI networks and identification of hub genes
Overlapping genes are represented in a Venn diagram as the intersection of upregulated and downregulated genes after SBRT with DEGs of different immune-infiltrated tumors (Figure 3A). Of the 41 genes related to TILs, the expression of 26 genes was upregulated (Figure 3A), whereas the expression of 15 genes was downregulated (Figure 3B) after SBRT. The STRING database was applied to build PPI networks to investigate the interactions between overlapping DEGs (Figure 3C,3D). Using the cytoHubba algorithm, PTPRC, TLR8, LILRB2, CCR5, and PLEK were selected as hub genes of the DEGs upregulated after SBRT (Figure 3E), and LTA, CD19, and CXCL13 were selected as hub genes of the DEGs downregulated after SBRT (Figure 3F).
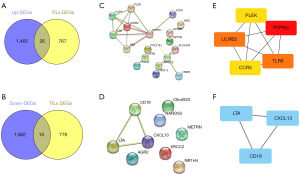
Functional analysis of hub genes and survival analysis
To explore the potential function of the eight identified hub genes in LUAD, GO functional enrichment analysis was performed. Gene set enrichment analysis was conducted to identify the KEGG pathways of the hub genes. As shown in Table 1, the eight hub genes were matched and assigned to their respective KEGG pathways as follows: viral protein interactions with cytokines and cytokine receptors, cytokine-cytokine receptor interaction and primary immunodeficiency. Survival curves constructed using the Kaplan-Meier plotter database showed that patients with LUAD with low PTPRC, CCR5, and TLR8 expression had a lower OS (Figure 4A-4C, respectively) whereas patients with low LILRB2 and LTA expression had higher OS (Figures 4D,4E, respectively). The expression of other hub genes including PLEK (Figure 4F), CD19 (Figure 4G) and CXCL13 (Figure 4H) was not associated with OS.
Table 1
Category | Term | P value |
---|---|---|
GOTERM_BP_DIRECT | Cell surface receptor signaling pathway | 2.56E−06 |
GOTERM_BP_DIRECT | Cell-cell signaling | 5.59E−05 |
GOTERM_BP_DIRECT | Immune response | 5.45E−04 |
GOTERM_BP_DIRECT | Cellular response to lipopolysaccharide | 0.001849 |
GOTERM_BP_DIRECT | Positive regulation of humoral immune response mediated by circulating immunoglobulin | 0.002168 |
GOTERM_CC_DIRECT | External side of plasma membrane | 4.11E−04 |
GOTERM_CC_DIRECT | Plasma membrane | 0.001644 |
GOTERM_CC_DIRECT | Integral component of plasma membrane | 0.009615 |
GOTERM_CC_DIRECT | Integral component of membrane | 0.015876 |
GOTERM_CC_DIRECT | Cell surface | 0.018104 |
GOTERM_CC_DIRECT | Membrane raft | 0.079134 |
GOTERM_MF_DIRECT | Heparin binding | 0.054256 |
KEGG_PATHWAY | Viral protein interaction with cytokine and cytokine receptor | 0.002137 |
KEGG_PATHWAY | Cytokine-cytokine receptor interaction | 0.017558 |
KEGG_PATHWAY | Primary immunodeficiency | 0.027476 |
KEGG_PATHWAY | B cell receptor signaling pathway | 0.058502 |
KEGG, Kyoto Encyclopedia of Genes and Genomes.
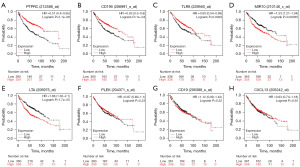
Expression of DEGs in normal and LUAD tissues
After analyzing the expression of hub genes in paired normal and tumor tissues, we found that all hub genes except for CCR5 were differentially expressed in tumor and normal lung tissues. Expression of PLEK, PTPRC, LILRB2, and TLR8 was downregulated in LUAD tissues (Figure 5A-5E), whereas expression of CD19, LTA, and CXCL13 was upregulated (Figure 5F-5H).
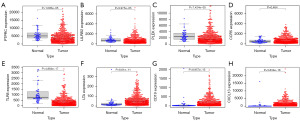
Hub gene mutations
Based on the cBioPortal database (TCGA, Firehose Legacy), among the eight hub genes, the PTPRC mutation rate (8%) was the highest, followed by LILRB2 (3%) and TLR8 (2.9%). Amplification was the most common mutation type (Figure 6).
Correlation between hub gene expression and response to immunotherapy in LUAD
To investigate the effect of hub genes on the response to immunotherapy in LUAD, we used the GSE126044 and GSE135222 mRNA microarray datasets. The DEGs associated with the response to immunotherapy were identified by comparing the mRNA microarray data in GSE126044 between responder and non-responder groups. We found that the expression of PTPRC, LILRB2, CCR5, and TLR8 was associated with responsiveness to immunotherapy (Figure 7A). In addition, we analyzed the effect of hub genes on the response to immunotherapy in patients with LUAD using the mRNA microarray data in GSE135222. Kaplan-Meier survival analysis confirmed that expression of CCR5 and TLR8 was associated with a better response to immunotherapy in patients with LUAD (Figure 7B).
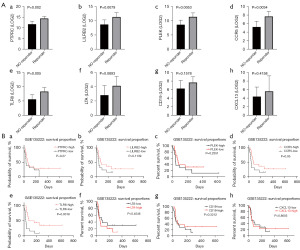
Association between hub gene expression and TILs
We subsequently investigated the association between the expression of CCR5 and TLR8 and immune subtypes. As shown in Figure 8A, a significant positive relationship was found between CCR5 and TLR8 expression and immune subtypes in LUAD (P<0.001). We then determined the correlations between these hub genes and 28 different TILs from the TISIDB database. The landscape of the correlations between the expression of two hub genes and TIL abundance in various types of cancer is shown in Figure 8A. To further investigate the association between CCR5 and TLR8 and these various ratios of TILs, we examined the correlation between CCR5 and TLR8 and markers of various immune cells. The results showed that the expression of CCR5 and TLR8 had a significant positive correlation with TILs in LUAD (Figure 8B). Previous studies have suggested that higher numbers of M0 macrophages and memory B cells are significantly associated with a better prognosis in patients with LUAD (22-24). In this study, we found that the expression of CCR5 and TLR8 was positively correlated with M0 macrophage and memory B cell infiltration in LUAD (Figure 9A,9B, respectively).
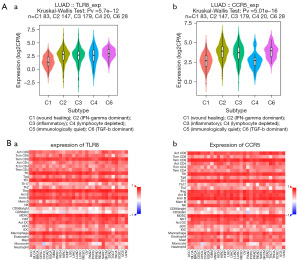
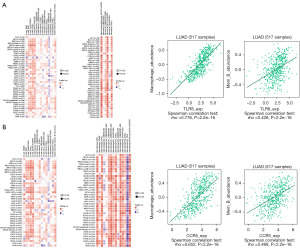
Relationship between hub genes and PD-L1 and CTLA-4 expression in LUAD at protein and mRNA levels
We analyzed the relationship between PD-L1 and hub gene expression using the cBioPortal and TIMER databases. We found that the expression of CCR5 and TLR8 was positively correlated with that of PD-L1 at the mRNA and protein level (Figure 10A,10B). CCR5 and TLR8 affected the transcription of CTLA-4 mRNA but had no effect on the CTLA-4 protein (Figure 10C,10D).
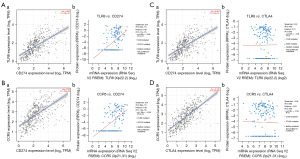
Discussion
Lung cancer is the most prevalent type of malignant tumor of the respiratory tract. Although considerable progress has been made in the treatment of lung cancer, the five-year survival rate and prognosis for patients with advanced lung cancer remain poor (25). Recently, there is growing evidence shown that dysregulated immune responses play essential roles in tumorigenesis and disease progression (26,27). Immunotherapy and SBRT are recognized as novel and effective lung cancer treatments. However, the effectiveness of RT or immunotherapy alone is limited. Thus, combining different therapies to control tumors is necessary.
Recently, scientists have observed that ionizing radiation delays and potentially inhibits tumor growth at distant sites. This phenomenon is known as the “abscopal effect”, which can be enhanced when combined with immunotherapy (28). “Abscopal effects” may lead to a survival benefit of the combination of RT and immunotherapy (29). There are evidence shown that the injury in the tumors may lead to the promotion of CD8+ T cells (30). Accumulating evidence suggests that some of the effects of ionizing radiation contribute to overall tumor immunity and the augmentation of tumor-specific immunity (31). Therefore, the combination of RT and immunotherapy is a potentially effective method of treating LUAD.
Evidence showing that RT releases tumor antigens, causing greater TIL activation. The density and diversity of TILs are closely related to prognosis and the effectiveness of immunotherapy (26,32). A high number of TILs have been related to favorable outcomes in a cohort of patients with NSCLC treated with immunotherapy (33,34).
Thorsson et al. (35) identified immune subtypes (C1–C6) based on the abundance of immune cells. The C3 (inflammatory) subtype had the best prognosis, whereas C4 (lymphocyte-depleted) and C6 [tumor growth factor beta (TGF-β)-dominant] had the worst prognosis. In this study, we found that the expression of CCR5 and TLR8 was significantly positively correlated with the immune subtype of LUAD. Immune-based subtypes may also affect immunotherapy responses. Therefore, the expression of CCR5 and TLR8 might be involved in the regulation of immune cell infiltration in LUAD. We observed a positive correlation between CCR5 and TLR8 expression and 28 TILs (including T cells, B cells, Treg cells, DC cells, and macrophages) in LUAD using TISIDB.
However, not all immune components affected the prognosis of patients with LUAD. A subset of TILs was associated with progression-free-survival (PFS) and OS in patients with lung cancer. Previous studies have found that M0 macrophages and memory B cells are associated with prediction of PFS and OS in LUAD patients treated with immunotherapy (22-24). We found that CCR5 and TLR8 expression were weakly positively correlated with the degree of macrophage M0 and memory B cell infiltration in LUAD. In addition, we found that CCR5 and TLR8 expression were associated with the expression of PD-L1 in LUAD tissues at both the mRNA and protein levels.
The results of this investigation are in line with those of previous studies. For example, TLR8 was previously found to play a vital role in tumor development by regulating the NF-κB pathway (36) and to regulate the function of CD4+ cells (37). TLR8, thus, plays a bridging role between innate immunity and adaptive immunity by activating the former. Several studies have found that TLR7/8 agonists have shown promise in preclinical cancer immunotherapy trials (38,39). Mullins et al. (40) reported that ICIs in combination with TLR7/8 agonists may have beneficial effects. CCR5 can be a homeostatic or inflammatory chemokine that is expressed in several types of cancer and immune cells. However, the critical mechanisms and roles of CCR5 in LUAD are yet to be understood. CCR5 was reported to dominate T cells homing and IFN-γ secretion (40). In addition to participating in the innate immune response, several studies have suggested that CCR5 can regulate immune check point responses. An ex vivo study showed that pembrolizumab could increase the apoptosis and decrease the proliferation of tumor cells in the CCR5+ CD66b+ high tumor-infiltrating neutrophils subgroup (41). In this study, expression of TLR8 and CCR5 was found to be upregulated after SBRT and was associated with high TIL levels in LUAD. Thus, SBRT might enhance the effects of the ICIs in LUAD through TLR8 and CCR5. Further research, however, is required to confirm this hypothesis.
In conclusion, this study demonstrated that SBRT can modify the TME, potentially affecting immune composition. Additionally, SBRT significantly upregulated PTPRC, LILRB2, TLR8, CCR5, and PLEK and significantly downregulated CXCL13, CD19, and LTA. Lastly, SBRT increased M0 macrophage and memory B cell infiltration, thereby increasing the effectiveness of immunotherapy. CCR5 and TLR8 expression are a potentially reliable predictor of an improved prognosis in patients with LUAD treated with a combination of immunotherapy and SBRT.
There are some limitations in this study. The sample size of the RNA-sequencing data of patients with LUAD before and after SBRT included in this study was small. Furthermore, the gene expression profile data for immunotherapy were not obtained from the same patient. Further studies should be conducted to confirm these results.
Conclusions
TLR8 and CCR5 expression were associated with an improved prognosis and increased responsiveness to immunotherapy by promoting M0 macrophage and memory B cell infiltration into LUAD tissues. TLR8 and CCR5 expression are thus a potential predictor of the effectiveness of combined immunotherapy and RT in patients with LUAD.
Acknowledgments
Funding: This work was supported by
Footnote
Reporting Checklist: The authors have completed the REMARK reporting checklist. Available at https://tcr.amegroups.com/article/view/10.21037/tcr-23-968/rc
Peer Review File: Available at https://tcr.amegroups.com/article/view/10.21037/tcr-23-968/prf
Conflicts of Interest: All authors have completed the ICMJE uniform disclosure form (available at https://tcr.amegroups.com/article/view/10.21037/tcr-23-968/coif). The authors have no conflicts of interest to declare.
Ethical Statement: The authors are accountable for all aspects of the work in ensuring that questions related to the accuracy or integrity of any part of the work are appropriately investigated and resolved. The study was conducted in accordance with the Declaration of Helsinki (as revised in 2013)
Open Access Statement: This is an Open Access article distributed in accordance with the Creative Commons Attribution-NonCommercial-NoDerivs 4.0 International License (CC BY-NC-ND 4.0), which permits the non-commercial replication and distribution of the article with the strict proviso that no changes or edits are made and the original work is properly cited (including links to both the formal publication through the relevant DOI and the license). See: https://creativecommons.org/licenses/by-nc-nd/4.0/.
References
- Relli V, Trerotola M, Guerra E, et al. Abandoning the Notion of Non-Small Cell Lung Cancer. Trends Mol Med 2019;25:585-94. [Crossref] [PubMed]
- Testa U, Pelosi E, Castelli G. Molecular charcterization of lung adenocarcinoma combining whole exome sequencing, copy number analysis and gene expression profiling. Expert Rev Mol Diagn 2022;22:77-100. [Crossref] [PubMed]
- Doroshow DB, Sanmamed MF, Hastings K, et al. Immunotherapy in Non-Small Cell Lung Cancer: Facts and Hopes. Clin Cancer Res 2019;25:4592-602. [Crossref] [PubMed]
- Suresh K, Naidoo J, Lin CT, et al. Immune Checkpoint Immunotherapy for Non-Small Cell Lung Cancer: Benefits and Pulmonary Toxicities. Chest 2018;154:1416-23. [Crossref] [PubMed]
- Reck M, Wehler T, Orlandi F, et al. Safety and Patient-Reported Outcomes of Atezolizumab Plus Chemotherapy With or Without Bevacizumab Versus Bevacizumab Plus Chemotherapy in Non-Small-Cell Lung Cancer. J Clin Oncol 2020;38:2530-42. [Crossref] [PubMed]
- Brahmer JR, Govindan R, Anders RA, et al. The Society for Immunotherapy of Cancer consensus statement on immunotherapy for the treatment of non-small cell lung cancer (NSCLC). J Immunother Cancer 2018;6:75. [Crossref] [PubMed]
- Bader JE, Voss K, Rathmell JC. Targeting Metabolism to Improve the Tumor Microenvironment for Cancer Immunotherapy. Mol Cell 2020;78:1019-33. [Crossref] [PubMed]
- Herrera FG, Ronet C, Ochoa de Olza M, et al. Low-Dose Radiotherapy Reverses Tumor Immune Desertification and Resistance to Immunotherapy. Cancer Discov 2022;12:108-33. [Crossref] [PubMed]
- Luke JJ, Lemons JM, Karrison TG, et al. Safety and Clinical Activity of Pembrolizumab and Multisite Stereotactic Body Radiotherapy in Patients With Advanced Solid Tumors. J Clin Oncol 2018;36:1611-8. [Crossref] [PubMed]
- Theelen WSME, Chen D, Verma V, et al. Pembrolizumab with or without radiotherapy for metastatic non-small-cell lung cancer: a pooled analysis of two randomised trials. Lancet Respir Med 2021;9:467-75. [Crossref] [PubMed]
- Theelen WSME, Peulen HMU, Lalezari F, et al. Effect of Pembrolizumab After Stereotactic Body Radiotherapy vs Pembrolizumab Alone on Tumor Response in Patients With Advanced Non-Small Cell Lung Cancer: Results of the PEMBRO-RT Phase 2 Randomized Clinical Trial. JAMA Oncol 2019;5:1276-82. [Crossref] [PubMed]
- Schoenfeld JD, Giobbie-Hurder A, Ranasinghe S, et al. Durvalumab plus tremelimumab alone or in combination with low-dose or hypofractionated radiotherapy in metastatic non-small-cell lung cancer refractory to previous PD(L)-1 therapy: an open-label, multicentre, randomised, phase 2 trial. Lancet Oncol 2022;23:279-91. [Crossref] [PubMed]
- Chen D, Menon H, Verma V, et al. Response and outcomes after anti-CTLA4 versus anti-PD1 combined with stereotactic body radiation therapy for metastatic non-small cell lung cancer: retrospective analysis of two single-institution prospective trials. J Immunother Cancer 2020;8:e000492. [Crossref] [PubMed]
- Wang J, Zhang J, Wen W, et al. Exploring low-dose radiotherapy to overcome radio-immunotherapy resistance. Biochim Biophys Acta Mol Basis Dis 2023;1869:166789. [Crossref] [PubMed]
- Yang Z, Luo Y, Yu H, et al. Reshaping the Tumor Immune Microenvironment Based on a Light-Activated Nanoplatform for Efficient Cancer Therapy. Adv Mater 2022;34:e2108908. [Crossref] [PubMed]
- Ozpiskin OM, Zhang L, Li JJ. Immune targets in the tumor microenvironment treated by radiotherapy. Theranostics 2019;9:1215-31. [Crossref] [PubMed]
- Merhi M, Raza A, Inchakalody VP, et al. Persistent anti-NY-ESO-1-specific T cells and expression of differential biomarkers in a patient with metastatic gastric cancer benefiting from combined radioimmunotherapy treatment: a case report. J Immunother Cancer 2020;8:e001278. [Crossref] [PubMed]
- Lin L, Kane N, Kobayashi N, et al. High-dose per Fraction Radiotherapy Induces Both Antitumor Immunity and Immunosuppressive Responses in Prostate Tumors. Clin Cancer Res 2021;27:1505-15. [Crossref] [PubMed]
- Tsoutsou PG, Zaman K, Martin Lluesma S, et al. Emerging Opportunities of Radiotherapy Combined With Immunotherapy in the Era of Breast Cancer Heterogeneity. Front Oncol 2018;8:609. [Crossref] [PubMed]
- Paijens ST, Vledder A, de Bruyn M, et al. Tumor-infiltrating lymphocytes in the immunotherapy era. Cell Mol Immunol 2021;18:842-59. [Crossref] [PubMed]
- Lin B, Du L, Li H, et al. Tumor-infiltrating lymphocytes: Warriors fight against tumors powerfully. Biomed Pharmacother 2020;132:110873. [Crossref] [PubMed]
- Liu X, Wu S, Yang Y, et al. The prognostic landscape of tumor-infiltrating immune cell and immunomodulators in lung cancer. Biomed Pharmacother 2017;95:55-61. [Crossref] [PubMed]
- Federico L, McGrail DJ, Bentebibel SE, et al. Distinct tumor-infiltrating lymphocyte landscapes are associated with clinical outcomes in localized non-small-cell lung cancer. Ann Oncol 2022;33:42-56. [Crossref] [PubMed]
- Chen P, Zhao L, Wang H, et al. Human leukocyte antigen class II-based immune risk model for recurrence evaluation in stage I-III small cell lung cancer. J Immunother Cancer 2021;9:e002554. [Crossref] [PubMed]
- Bade BC, Dela Cruz CS. Lung Cancer 2020: Epidemiology, Etiology, and Prevention. Clin Chest Med 2020;41:1-24. [Crossref] [PubMed]
- Jackaman C, Tomay F, Duong L, et al. Aging and cancer: The role of macrophages and neutrophils. Ageing Res Rev 2017;36:105-16. [Crossref] [PubMed]
- Giannone G, Ghisoni E, Genta S, et al. Immuno-Metabolism and Microenvironment in Cancer: Key Players for Immunotherapy. Int J Mol Sci 2020;21:4414. [Crossref] [PubMed]
- Ashrafizadeh M, Farhood B, Eleojo Musa A, et al. Abscopal effect in radioimmunotherapy. Int Immunopharmacol 2020;85:106663. [Crossref] [PubMed]
- Fiorica F, Belluomini L, Giuliani J, et al. Abscopal effect and resistance reversion in nivolumab-treated non-small-cell lung cancer undergoing palliative radiotherapy: a case report. Immunotherapy 2021;13:971-6. [Crossref] [PubMed]
- Ngwa W, Irabor OC, Schoenfeld JD, et al. Using immunotherapy to boost the abscopal effect. Nat Rev Cancer 2018;18:313-22. [Crossref] [PubMed]
- Arina A, Gutiontov SI, Weichselbaum RR. Radiotherapy and Immunotherapy for Cancer: From "Systemic" to "Multisite". Clin Cancer Res 2020;26:2777-82. [Crossref] [PubMed]
- Whiteside TL. Tumor-Infiltrating Lymphocytes and Their Role in Solid Tumor Progression. Exp Suppl 2022;113:89-106. [Crossref] [PubMed]
- Gataa I, Mezquita L, Rossoni C, et al. Tumour-infiltrating lymphocyte density is associated with favourable outcome in patients with advanced non-small cell lung cancer treated with immunotherapy. Eur J Cancer 2021;145:221-9. [Crossref] [PubMed]
- Zemek RM, De Jong E, Chin WL, et al. Sensitization to immune checkpoint blockade through activation of a STAT1/NK axis in the tumor microenvironment. Sci Transl Med 2019;11:eaav7816. [Crossref] [PubMed]
- Thorsson V, Gibbs DL, Brown SD, et al. The Immune Landscape of Cancer. Immunity 2018;48:812-830.e14. [Crossref] [PubMed]
- Urban-Wojciuk Z, Khan MM, Oyler BL, et al. The Role of TLRs in Anti-cancer Immunity and Tumor Rejection. Front Immunol 2019;10:2388. [Crossref] [PubMed]
- Shang W, Xu R, Xu T, et al. Ovarian Cancer Cells Promote Glycolysis Metabolism and TLR8-Mediated Metabolic Control of Human CD4(+) T Cells. Front Oncol 2020;10:570899. [Crossref] [PubMed]
- Chi H, Li C, Zhao FS, et al. Anti-tumor Activity of Toll-Like Receptor 7 Agonists. Front Pharmacol 2017;8:304. [Crossref] [PubMed]
- Serrano R, Wesch D, Kabelitz D. Activation of Human γδ T Cells: Modulation by Toll-Like Receptor 8 Ligands and Role of Monocytes. Cells 2020;9:713. Correction appears in Cells 2020;9.
- Mullins SR, Vasilakos JP, Deschler K, et al. Intratumoral immunotherapy with TLR7/8 agonist MEDI9197 modulates the tumor microenvironment leading to enhanced activity when combined with other immunotherapies. J Immunother Cancer 2019;7:244. [Crossref] [PubMed]
- Li J, McArdle S, Gholami A, et al. CCR5+T-bet+FoxP3+ Effector CD4 T Cells Drive Atherosclerosis. Circ Res 2016;118:1540-52. [Crossref] [PubMed]