A polyamine metabolism risk signature for predicting the prognosis and immune therapeutic response of kidney cancer
Highlight box
Key findings
• We identified several markers based on polyamine metabolism in kidney renal clear cell (KIRC) and constructed a risk signature to predict the prognosis of patients with KIRC.
What is known and what is new?
• Several polyamine metabolism-related genes (PMRGs) showed expression alteration in KIRC and were closely related to the patients’ prognosis.
• The risk signature based on the expression of PMRGs could act as an independent factor to predict the prognosis and immune therapy response of patients with KIRC.
What is the implication, and what should change now?
• PMRGs may be prognostic biomarkers for KIRC. In addition, the PMRG risk signature could be employed by clinicians to estimate prognosis and immune therapeutic response.
Introduction
Renal cell carcinoma (RCC) is among the top 10 most prevalent malignancies worldwide and has exhibited increasing incidence over the past decade, with its most common histological type being kidney renal clear cell (KIRC) carcinoma (1). Although various treatment methods such as surgery, chemotherapy, targeted therapy, and immunotherapy have been used in clinical practice, their efficacy is still limited, especially for metastatic kidney cancer (2). Therefore, it is imperative that new therapeutic targets for kidney cancer be developed.
Polyamines are a class of compounds containing two or more amino groups, which exist in mammalian cells at millimolar levels and play a significant role in normal and neoplastic cell function and replication (3). Dysregulation of polyamine metabolism is a common phenomenon in cancer. The discovery of polyamine-related pathways in cancer has made it possible to develop cancer therapies targeting polyamine metabolism. The RAS–RAF–MEK–ERK signaling pathway has been shown to control polyamine metabolism in multiple aspects and may augment polyamine transport in colon cancer (4). PTEN–PI3K–mTOR complex 1 (mTORC1) has been found to play an essential role in prostate tumors, and inhibition of mTOR results in reduced levels of intracellular polyamines (5). In addition, polyamines and their metabolites have been developed as biomarkers in lung, liver, prostate, pancreatic, and colon cancers (6-9). A previous study showed that the regulation of polyamine metabolism can inhibit KIRC progression (10). However, the biomarkers and detailed molecular mechanism underlying the effect of polyamine metabolism on RCC remain unclear. The development of bioinformatics and biostatistical analyses have provided the means to exploring the correlation between tumors and gene alteration. Using large-scale multiomics databases, researchers have made significant progress in various fields of medical research by, for example, identifying target genes, clarifying mechanisms of disease, developing targeted drugs, and diagnosing disease (11-13). As polyamine influences KIRC, characterizing the relationship between polyamine metabolism-related genes (PMRGs) and KIRC based on large-scale public data with the bioinformatics method may be valuable in providing a foundation for subsequent experiments and the development of polyamine metabolism-based targeted drugs.
In this study, we aimed to investigate the function of PMRGs in KIRC and identify potential biomarkers. Through a series of analyses, we found 6 PMRGs related to the overall survival (OS) of patients with KIRC and constructed a 4-gene (SRM, AGMAT, HDAC10, and AOC1) risk signature. We further developed a nomogram that combined the PMRG signature and other clinical factors, which showed a better ability to predict patient outcomes. Additionally, our results suggested that the PMRG risk signature may also predict the response to immune therapy in KIRC. In conclusion, our study identified several PMRGs that may play an essential role in KIRC and constructed a clinical prediction model to predict prognosis and immune therapy response. These findings provide a theoretical basis for subsequent research. We present this article in accordance with the TRIPOD reporting checklist (available at https://tcr.amegroups.com/article/view/10.21037/tcr-23-344/rc).
Methods
Acquisition of public data
We acquired the PMRGs from the publicly available resources of the molecular signatures database (MSigDB) (https://www.gsea-msigdb.org/gsea/msigdb/index.jsp) (Table S1) and downloaded the gene expression data for KIRC from The Cancer Genome Atlas (TCGA) integrated website (https://xena.ucsc.edu/). We normalized and transformed the messenger RNA (mRNA) expression data to log2[transcripts per million (TPM) +1] units. To validate the differentially expressed genes (DEGs), we obtained two external RNA-sequencing (RNA-seq) expression datasets (GSE11151 and GSE36895) from the Gene Expression Omnibus (GEO) (https://www.ncbi.nlm.nih.gov/geo/). We also collected the expression profiles and clinical data of three additional external datasets (E-MTAB-1980, GSE78820, and IMvigor210). The E-MTAB-1980 dataset (https://www.ebi.ac.uk/arrayexpress) was used to validate the risk model, and the anti-programmed cell death protein 1 (PD1) datasets, GSE78820 (https://www.ncbi.nlm.nih.gov/geo/) and IMvigor210 (14) were used to evaluate the predictive accuracy of the risk model for immune therapy response. This study was conducted in accordance with the Declaration of Helsinki (as revised in 2013).
Verification of survival prediction
To investigate the impact of DEGs on the OS rate of patients with KIRC, we performed univariate Cox proportional hazards regression analysis using the “survival” package in R (The R Foundation for Statistical Computing). Kaplan-Meier analysis was used to assess the OS rate of patients with KIRC. The optimal cutoff value for DEGs was determined using the surv_cutpoint function from the “survminer” package in R. The log-rank test was used to evaluate the relationship between different variables and patient OS.
Differential expression and protein level analysis
DEGs between tumor and normal tissues were identified using the “limma” package in R and filtered with an adjusted P value of less than 0.05 and an absolute log2(fold change) greater than 0.5. Further exploration of protein levels was carried out using the Human Protein Atlas (HPA) database (https://www.proteinatlas.org/), which currently contains 44 protein datasets of human tissue, including 15,323 genes and corresponding antibodies.
Molecular mechanism and single-cell sequence analysis
The activity score of 50 cancer-related hallmark pathways and Kyoto Encyclopedia of Genes and Genomes (KEGG) pathways was evaluated using the single-sample gene set enrichment analysis (ssGSEA) method from the “GSVA” R package to identify the pathways affected by the PMRGs. Gene set variation analysis (GSVA) is a nonparametric and unsupervised method for estimating gene set enrichment variation through the expression data set of the samples (15). The Pearson correlation coefficient (PCC) between gene mRNA expression value and pathway activity score was calculated, and the P value was adjusted with the false discovery rate (FDR). To analyze the distribution of PMRGs in the tumor microenvironment (TME), we used a single-cell RNA-seq database of the TME from the Tumor Immune Single-cell Hub 2 (TISCH2) public website and obtained detailed cell-type annotation at the single-cell level (http://tisch.comp-genomics.org/home/) (16). For each individual single-cell sequence dataset, we calculated the top 100 coexpressed genes of the PMRGs and subjected them to gene enrichment analysis via the Database for Annotation, Visualization and Integrated Discovery (DAVID) website (https://david.ncifcrf.gov/).
Construction and validation of the PMRG risk signature
We randomly divided the patients into training and testing cohorts in a 1:1 ratio using the createDataPartition function in R. Subsequently, we employed the least absolute shrinkage and selection operator (LASSO)-penalized Cox (LASSO-Cox) regression model with the “glmnet” R package to identify candidate genes and develop a risk signature in the training cohort. LASSO-Cox is a Cox proportional hazards model that uses the LASSO for variable selection and shrinkage (17). The risk score formula was derived using the coefficients obtained from the LASSO regression algorithm and gene expression values and is expressed as follows:
After verifying the signature in the testing cohort and the E-MTAB-1980 dataset, we conducted 3-, 5-, and 7-year receiver operating characteristic (ROC) curve analyses using the “survivalROC” R package. We also performed univariate and multivariable Cox regression models for the prognosis analysis. In the multivariable Cox regression analysis, variables with a P value less than 0.05 were identified as independent risk factors.
Molecular mechanism analysis
We initially calculated the DEGs [absolute log2(fold change) greater than 0.5 and adjusted P value less than 0.05] between the high-risk group and the low-risk group. Subsequently, using the “clusterProfiler” package in R, we subjected these genes to gene set enrichment analysis (GSEA), a method that can identify common biological pathways.
Construction and verification of the nomogram
We employed stepwise regression methods to screen variables, selecting only those that yielded a minimum Akaike information criterion (AIC) value for our model. These variables were subsequently used to construct a nomogram for assessing the probability of 3- and 5-year OS via the “rms” R package. ROC curve, calibration plots, and decision curve analysis (DCA) were used to evaluate the model.
Immune cell infiltration
The R package “estimate” was used to determine the immune score, stromal score, and tumor purity for each patient. Additionally, we quantified the infiltration levels of immune cells using the ssGSEA method of GSVA via the “xCell” R package. “xCell” is a webtool that conducts cell type enrichment analysis from gene expression data for 64 immune and stroma cell types (https://github.com/dviraran/xCell).
Statistical analysis
The unpaired t-test was use to compare the mean difference of two groups for two consecutive variables. Univariate and multivariate Cox regression analysis was used to determine the relation between variables and clinical outcomes, and the log-rank test was used to evaluate the significance. Based on Pearson method, the PCC was computed to characterize the correlation between two consecutive variables.
Results
Identification of differential expression genes
We first identified nine DEGS of polyamine metabolism in TCGA-KIRC tissues. Among these, four genes (including HDAC10, SMOX, NNMT, and SRM) were upregulated, and five genes (including AOC1, SMS, ODC1, AGMAT, and SAT2) were downregulated in tumor tissues (Figure 1A,1B). In the GSE36895 and GSE11151 datasets, we also observed the same expression alteration (Figure S1A,S1B). We further used the HPA database to validate the results. We found that AOC1, AGMAT, ODC1, SAT2, and SMS Consistently showed a lower expression in kidney cancer tissues than in normal tissues, while SRM and NNMT showed a higher expression in kidney cancer tissues than in normal tissues (Figure S1C).
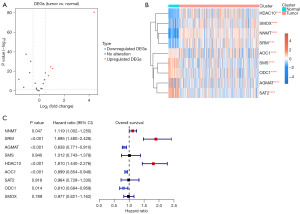
Identification of prognosis-related PMRGs and function analysis
To determine the prognosis-related PMRGs, we conducted the univariate Cox regression and found that six PMRGs correlated with the OS of patients with KIRC. NNMT, SRM, and HDAC10 were found to risk genes, while AGMAT, AOC1, and ODC1 were the protective genes for the OS of patients with KIRC (Figure 1C). The detailed information of these six genes are shown in Table S2. The patients with a high expression of NNMT, SRM, and HDAC10 and those with a low expression of AGMAT, AOC1, and ODC1 experienced worse clinical outcomes (Figure S2). In expression levels, these six genes showed a weaker correlation with each other (Figure S3A). In addition, we found that these PMRGs interacted with different proteins, indicating that these PMRGs might play various roles in regulating different substrates in cancer (Figure S3B, Table S3). Further analysis indicated that these six genes were correlated with different molecular mechanisms and located in different cells in the TME (Figure 2A-2D, details in available online: https://cdn.amegroups.cn/static/public/tcr-23-344-1.xls and Tables S4). For example, AGMAT was highly positively correlated with multiple metabolism-related pathways and located in malignant cells. SRM and HDAC10 showed a negative correlation with these metabolism-related pathways and were located in multiple immune-related cells. Overall, these PMRGs exerted a variety of functions in regulating KIRC.
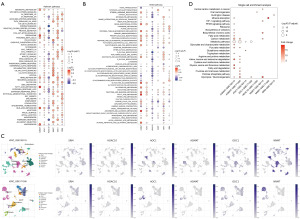
Construction of the risk signature
We further separated the TCGA-KIRC samples into training and test cohorts. In the training cohort, we constructed the risk signature based on the mRNA expression of these six PMRGs (Figure S4A,S4B). A 4-gene PMRG risk signature was finally constructed (Figure S4C), and the risk score was calculated as follows:
We found that patients with a high PMRG risk score showed worse outcomes in the training cohort. The area under the ROC curve (AUC) showed that the prediction accuracy of PMRGs at 3, 5, and 7 years was 0.6717, 0.7245, and 0.7593, respectively. As risk scores increased, the number of deaths in the patient groups increased. In addition, we found that SRM and HDAC10 had a higher expression while AGMAT and AOC1 had a lower expression in the high PMRG risk group than in the low PMRG risk group (Figure 3A). In the test and external cohorts, we also observed the same results (Figure 3B,3C). The AUC curve showed a feasible predicted accuracy on 3-, 5-, and 7-year survival (test cohort: AUC at 3 years =0.7081, AUC at 5 years =0.6848, AUC at 7 years =0.7377; E-MTAB-1980: AUC at 3 years =0.7991, AUC at 5 years =0.7776, AUC at 7 years =0.7124).
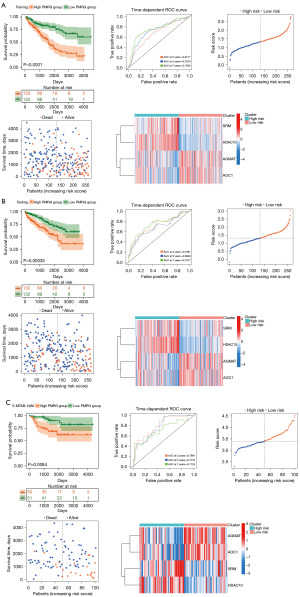
Correlation of PMRG risk score and clinical
In the whole TCGA-KIRC cohort, the risk score showed no significant difference in age or gender, but increasing risk score was associated with advanced histological grade and pathological stage (Figure 4A). We further divided the clinical information into different clinical subgroups and found that the high and low PMRG risk groups also experienced different clinical outcomes, indicating that the PMRG risk signature has strong robustness (Figure 4B). In addition, the prognosis-related clinical variables (including PMRG risk score, age, grade, and stage) analyzed via univariate Cox regression were used to perform multivariate Cox regression analysis. We found that the PMRG risk score was an independent prognostic factor (Figure 4C). Similar results were found in the E-MTAB-1980 dataset (Figure S5A-S5C). Overall, our PMRG risk signature could independently predict the prognosis of patients with KIRC and had robust accuracy.
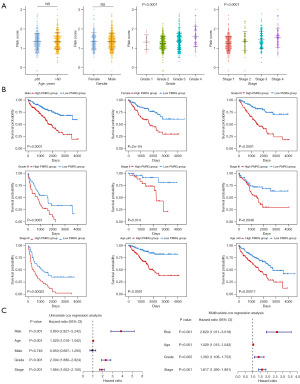
Nomogram construction of the PMRG risk signature
We used the “rms” in package in R to construct a nomogram based on the variables correlated with prognosis in multivariate Cox regression. The nomogram could predict the 3- and 5-year OS of the patients with KIRC (Figure 5A). The AUC and calibration curve indicated that the clinical model had good prediction accuracy (AUC at 3 years =0.8075, AUC at 5 years =0.7740), superior to that of using the PMRG risk signature alone. Furthermore, DCA revealed that combining all clinical variables could yield the best prediction of patients’ prognoses. The same results were observed in the E-MTAB-1980 dataset (AUC at 3 years =0.8868, AUC at 5 years =0.8438), indicating that PMRG risk signature could predict the prognosis of patients with KIRC (Figure 5B).
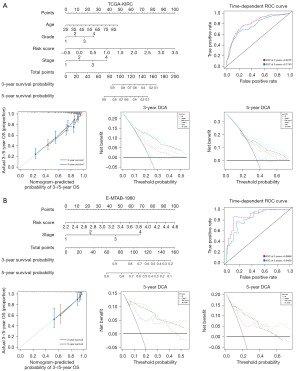
Mechanism analysis
To determine the potential mechanisms underlying the survival difference between the high and low PMRG risk groups, we screened the DEGs between these groups and input them into the “clusterProfiler” package in R (available online: https://cdn.amegroups.cn/static/public/tcr-23-344-2.xls). For cell components (CC), we found that blood microparticle, immunoglobulin complex, circulating immunoglobulin complex, nuclear body, nuclear lumen, nuclear protein-containing complex, nuclear speck, nucleolus, ribonucleoprotein complex, and spliceosomal complex were highly enriched in high PMRG risk group (Figure S6A). For biological process (BP), we found that several immune-related processes, such as humoral immune response, B cell-mediated immunity, complement activation, and regulation of B-cell activation, were highly enriched in the high PMRG risk group (Figure S6B). For molecular function (MF), we found that antigen binding, cytokine activity, immunoglobulin receptor binding, RNA binding, and small molecule binding were significantly enriched in the high PMRG risk group (Figure S6C). In addition, we found several immune-related pathways that were highly enriched in the high PMRG risk group, including the interleukin 17 (IL-17) signaling pathway and tumor necrosis factor (TNF) signaling pathway. In contrast, several metabolism-related pathways were highly enriched in the low PMRG risk group, including metabolic pathways and carbon metabolism (Figure S6D). These results suggested that immune- and metabolism-related pathways were highly enriched in either the low or high PMRG risk groups. We further calculated the activity score of 50 cancer-related hallmark pathways to characterize the activity difference between the high- and low-risk groups (Figure 6). We found that the activity score of some hallmark pathways, including DNA repair and MYC targets V2, was higher in the high PMRG risk group than in the low PMRG risk group. Moreover, the PMRG risk score showed a positive correlation with the activity of these two pathways. Interestingly, several metabolism-related pathways were activated in the low-risk group, such as adipogenesis, fatty acid metabolism, bile acid metabolism, and heme metabolism. The PMRG risk score showed a significantly negative correlation with these pathways.
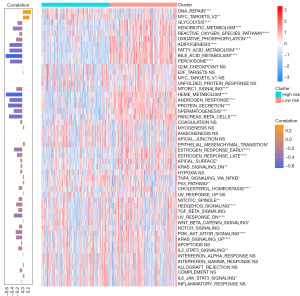
Immune infiltration and prediction of hot and cold tumors
Due to the PMRG risk score being correlated with immune-related pathways, we further observed the expression of immune checkpoints in the high and low PMRG risk groups. We found that the expression of CD274 [also knowns programmed death-ligand 1 (PDL1)] was higher in the low-risk group, whereas that of CTLA4 and PDCD1 (also known as PD1) was higher in the high-risk group (Figure 7A). In addition, the high-risk group showed a higher immune score and a higher activity score of CD8 T cells, cytotoxic cells, natural killer (NK) cells, and T regulatory cells (Tregs), among others; meanwhile, the low-risk group showed higher tumor purity and a higher activity score for mast cells and neutrophils (Figure S7). The same results were observed with the “xCell” R package (Figure 7B). CD8+ T cells, T helper type 1 (Th1) cells, B cells, and NK T cells showed a higher infiltration level in the high-risk group. The immune and microenvironment scores were also higher in the high-risk group. Tumors with a high degree of infiltration of CD4 and CD8 T cells are considered to be hot tumors, while tumors with a low degree of infiltration are considered to be cold tumors (18). Our study indicated that the PMRG risk signature could identify the hot and cold tumors of KIRC and thus may offer a feasible stratification method for selecting those patients who are sensitive to immune therapy. We used two external anti-PD1 datasets to explore the correlation between the PMRG risk signature and immune therapy response. The results pointed to a tendency of patients in the low-risk group to receive clinical benefit after immune therapy (Figure 7C). The AUC for the risk score in predicting immune therapy response was 0.59 and 0.60 in the IMvigor120 and GSE78820 datasets, respectively.
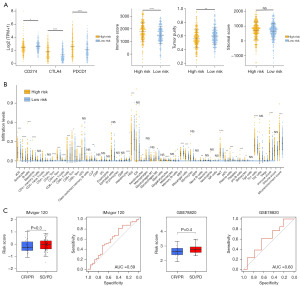
Discussion
Targeted and immune checkpoint therapy have shown promising results in clinical trials for RCC. Despite the relatively high response rate of RCC to immunotherapy and targeted therapy, the majority of patients with RCC fail to receive long-lasting clinical benefit due to the development of drug resistance (19). Therefore, there is an urgent need to develop new therapeutic targets for RCC.
In normal cells, polyamines are essential for cell growth, and the depletion of polyamines can result in cytostasis. In cancer, polyamine metabolism is frequently dysfunctional (6). Polyamines and their metabolites are involved in many significant tumor BPs, including metabolism, immunity, tumor cell genesis, and metastasis (3). Both experimental and clinical depletion of polyamines have shown that this metabolism is a promising target for therapy. For instance, ornithine decarboxylase (ODC) is a rate-limiting enzyme in polyamine biosynthesis, and inhibiting ODC was found to limit tumor formation and progression in preclinical models (20). The ODC inhibitor difluoro-methylornithine (DFMO) is a highly effective chemopreventive agent for cancer and is thought to act via polyamine depletion (21). KIRC is characterized by consistent metabolic abnormalities, such as highly elevated glycogen and lipid deposition. Alteration of polyamine levels may influence KIRC tumor growth. For example, ARG2 deletion can prevent the toxic polyamine accumulation that promotes KIRC tumor growth (10). However, there is little research concerning the relationship between polyamine metabolism and KIRC, and further investigation is needed to determine the exact mechanism of effect and function of polyamine metabolism in KIRC.
Polyamines and their metabolites are currently used as cancer biomarkers. Recently developed metabolomic techniques represent more effective methods for predicting the biomarkers in cancer and treatment response. For example, in lung and liver cancers, polyamines and their metabolites have been used in diagnosis and as markers of cancer progression (22). In prostate, pancreatic, and colon cancer, polyamines and metabolites have shown potential as biomarkers (7-9). In our study, we identified several PMRGs in KIRC. Among these, NNMT, SRM, and HDAC10 were upregulated in KIRC and were found to be correlated with worse prognosis in patients. In tumors, high NNMT expression is associated with worse prognosis (23). NNMT in combination with TTPAL promotes carcinogenesis through the P13K/AKT pathway (24). In colorectal cancer, ABHD5 suppresses the SRM-dependent spermidine production in tumor-associated macrophages and potentiates tumor growth (25). HDAC10, a histone deacetylase, has been reported to be correlated with tumor progression and poor prognosis in multiple cancers, including ovarian, lung, and colon cancer (26-28). AGMAT, AOC1, and ODC1 have been reported to be oncogenes in several types of tumor (29-32), promoting tumor progression and metastasis. Interestingly, we observed that their expression was downregulated in KIRC and that they acted as protective factors for patients with KIRC. In KIRC, multiple metabolic pathways are universally depleted, so KIRC is not generally heterogeneous compared with other cancers (10). Inhibition of toxic polyamine accumulation promotes KIRC growth, which might be why these genes are downregulated in the tumor; however, this is merely speculation and should be further validated.
We used the PMRGs to construct a risk model. A 4-gene risk signature was constructed to predict the prognosis of patients with KIRC. The patients with high PMRG risk showed worse outcomes and more advanced histological grade and pathological stage. Multivariate Cox regression revealed the PMRG risk signature to be an independent prediction factor, indicating that the PMRGs were not affected by other clinical variables. Interestingly, we found that the PMRG risk signature was negatively correlated with multiple metabolism-related pathways, which further indicated that depletion of metabolism pathways is correlated with poor outcomes for patients with KIRC. Furthermore, DNA repair showed high activity in the high-risk group. A considerable amount of research has been conducted concerning the relationship between polyamine and DNA repair. Mechanistically, polyamines promote homologous recombination-mediated double-strand break repair and maintain the integrity of the genome (33). Polyamines can facilitate the pairing between single strands of DNA and stabilize double-stranded DNA (34,35). DFMO has been found to induce DNA damage and prevent gastric carcinogenesis (36). Polyamine-depleted HeLa cells are at increased nickel-induced DNA damage (37). Therefore, polyamine has an important role in the maintenance of genome stability, which might be a potential mechanism of polyamine in regulating KIRC that is worthy of further exploration.
Tumors can be classified into three dominant immune phenotypes—immune excluded, immune desert, and immune inflamed—which show different responses to PD1 blockade (38). The immune-inflamed tumor, which is also known as a hot tumor, is characterized by increased infiltration of CD4 and CD8 T cells to the tumor cell nest and stroma. This tumor type is considered to be immunoreactive. Transforming the cold tumor to a hot tumor may elevate the tumor response for immune therapy (18).
Research indicates that polyamines have anti-inflammatory, immunosuppressive properties, which suggests that altering the levels of polyamines can improve the antitumor immune response (39). Recent data suggest that the level of polyamines may contribute to the presence of immune-desert tumors that do not respond to immune checkpoint blockade (3). Our study found multiple immune-related pathways highly enriched in the high PMRG risk group. The patients with high PMRG risk scores showed a high infiltration level of CD4 T cells and immune scores, indicating that the PMRG risk score can distinguish between the cold and hot tumors of KIRC. Furthermore, PD1 and CTLA4 showed a higher expression in the high PMRG risk group, indicating that the patients in this group might be sensitive to immune therapy. Two external anti-PD1 datasets provided indirect confirmatory evidence. Patients in the high PMRG risk group treated with anti-PD1 therapy tended to experience stable disease (SD) or progressive disease (PD), whereas patients in the low PMRG risk group tended experience to complete response (CR) or partial response (PR). Although the difference in risk scores between the CR/PR and SD/PD groups was not significant, this suggested the potential for the PMRG signature to predict response to anti-PD1 therapy. However, whether the alteration of polyamine levels influences antitumor immunotherapy still needs to be determined.
Conclusions
Our study identified several potential markers which may be critical factors in KIRC; however, these need to be verified with further experimental validation. In addition, although we constructed a risk signature to predict the prognosis and immune therapy response, more prospective clinical studies are needed to evaluate this signature. We hope these findings can help form the foundation of future research in polyamine metabolism and KIRC.
Acknowledgments
Funding: This work was supported by
Footnote
Reporting Checklist: The authors have completed the TRIPOD reporting checklist. Available at https://tcr.amegroups.com/article/view/10.21037/tcr-23-344/rc
Peer Review File: Available at https://tcr.amegroups.com/article/view/10.21037/tcr-23-344/prf
Conflicts of Interest: All authors have completed the ICMJE uniform disclosure form (available at https://tcr.amegroups.com/article/view/10.21037/tcr-23-344/coif). The authors have no conflicts of interest to declare.
Ethical Statement: The authors are accountable for all aspects of the work in ensuring that questions related to the accuracy or integrity of any part of the work are appropriately investigated and resolved. The study was conducted in accordance with the Declaration of Helsinki (as revised in 2013).
Open Access Statement: This is an Open Access article distributed in accordance with the Creative Commons Attribution-NonCommercial-NoDerivs 4.0 International License (CC BY-NC-ND 4.0), which permits the non-commercial replication and distribution of the article with the strict proviso that no changes or edits are made and the original work is properly cited (including links to both the formal publication through the relevant DOI and the license). See: https://creativecommons.org/licenses/by-nc-nd/4.0/.
References
- Hsieh JJ, Purdue MP, Signoretti S, et al. Renal cell carcinoma. Nat Rev Dis Primers 2017;3:17009. [Crossref] [PubMed]
- Makhov P, Joshi S, Ghatalia P, et al. Resistance to Systemic Therapies in Clear Cell Renal Cell Carcinoma: Mechanisms and Management Strategies. Mol Cancer Ther 2018;17:1355-64. [Crossref] [PubMed]
- Holbert CE, Cullen MT, Casero RA Jr, et al. Polyamines in cancer: integrating organismal metabolism and antitumour immunity. Nat Rev Cancer 2022;22:467-80. [Crossref] [PubMed]
- Roy UK, Rial NS, Kachel KL, et al. Activated K-RAS increases polyamine uptake in human colon cancer cells through modulation of caveolar endocytosis. Mol Carcinog 2008;47:538-53. [Crossref] [PubMed]
- Zabala-Letona A, Arruabarrena-Aristorena A, Martín-Martín N, et al. Corrigendum: mTORC1-dependent AMD1 regulation sustains polyamine metabolism in prostate cancer. Nature 2018;554:554. [Crossref] [PubMed]
- Murray-Stewart TR, Woster PM, Casero RA Jr. Targeting polyamine metabolism for cancer therapy and prevention. Biochem J 2016;473:2937-53. [Crossref] [PubMed]
- Giskeødegård GF, Bertilsson H, Selnæs KM, et al. Spermine and citrate as metabolic biomarkers for assessing prostate cancer aggressiveness. PLoS One 2013;8:e62375. [Crossref] [PubMed]
- Asai Y, Itoi T, Sugimoto M, et al. Elevated Polyamines in Saliva of Pancreatic Cancer. Cancers (Basel) 2018;10:43. [Crossref] [PubMed]
- Nakajima T, Katsumata K, Kuwabara H, et al. Urinary Polyamine Biomarker Panels with Machine-Learning Differentiated Colorectal Cancers, Benign Disease, and Healthy Controls. Int J Mol Sci 2018;19:756. [Crossref] [PubMed]
- Ochocki JD, Khare S, Hess M, et al. Arginase 2 Suppresses Renal Carcinoma Progression via Biosynthetic Cofactor Pyridoxal Phosphate Depletion and Increased Polyamine Toxicity. Cell Metab 2018;27:1263-1280.e6. [Crossref] [PubMed]
- Li K, Du Y, Li L, et al. Bioinformatics Approaches for Anti-cancer Drug Discovery. Curr Drug Targets 2020;21:3-17. [Crossref] [PubMed]
- Cortés-Ciriano I, Gulhan DC, Lee JJ, et al. Computational analysis of cancer genome sequencing data. Nat Rev Genet 2022;23:298-314. [Crossref] [PubMed]
- Jiang P, Sinha S, Aldape K, et al. Big data in basic and translational cancer research. Nat Rev Cancer 2022;22:625-39. [Crossref] [PubMed]
- Mariathasan S, Turley SJ, Nickles D, et al. TGFβ attenuates tumour response to PD-L1 blockade by contributing to exclusion of T cells. Nature 2018;554:544-8. [Crossref] [PubMed]
- Hänzelmann S, Castelo R, Guinney J. GSVA: gene set variation analysis for microarray and RNA-seq data. BMC Bioinformatics 2013;14:7. [Crossref] [PubMed]
- Han Y, Wang Y, Dong X, et al. TISCH2: expanded datasets and new tools for single-cell transcriptome analyses of the tumor microenvironment. Nucleic Acids Res 2023;51:D1425-31. [Crossref] [PubMed]
- Simon N, Friedman J, Hastie T, et al. Regularization Paths for Cox's Proportional Hazards Model via Coordinate Descent. J Stat Softw 2011;39:1-13. [Crossref] [PubMed]
- Ochoa de Olza M, Navarro Rodrigo B, Zimmermann S, et al. Turning up the heat on non-immunoreactive tumours: opportunities for clinical development. Lancet Oncol 2020;21:e419-30. [Crossref] [PubMed]
- Motzer RJ, Tannir NM, McDermott DF, et al. Nivolumab plus Ipilimumab versus Sunitinib in Advanced Renal-Cell Carcinoma. N Engl J Med 2018;378:1277-90. [Crossref] [PubMed]
- Coni S, Serrao SM, Yurtsever ZN, et al. Blockade of EIF5A hypusination limits colorectal cancer growth by inhibiting MYC elongation. Cell Death Dis 2020;11:1045. [Crossref] [PubMed]
- Witherspoon M, Chen Q, Kopelovich L, et al. Unbiased metabolite profiling indicates that a diminished thymidine pool is the underlying mechanism of colon cancer chemoprevention by alpha-difluoromethylornithine. Cancer Discov 2013;3:1072-81. [Crossref] [PubMed]
- Xu H, Liu R, He B, et al. Polyamine Metabolites Profiling for Characterization of Lung and Liver Cancer Using an LC-Tandem MS Method with Multiple Statistical Data Mining Strategies: Discovering Potential Cancer Biomarkers in Human Plasma and Urine. Molecules 2016;21:1040. [Crossref] [PubMed]
- Song M, Li Y, Miao M, et al. High stromal nicotinamide N-methyltransferase (NNMT) indicates poor prognosis in colorectal cancer. Cancer Med 2020;9:2030-8. [Crossref] [PubMed]
- Liu W, Gou H, Wang X, et al. TTPAL promotes gastric tumorigenesis by directly targeting NNMT to activate PI3K/AKT signaling. Oncogene 2021;40:6666-79. [Crossref] [PubMed]
- Miao H, Ou J, Peng Y, et al. Macrophage ABHD5 promotes colorectal cancer growth by suppressing spermidine production by SRM. Nat Commun 2016;7:11716. [Crossref] [PubMed]
- Tao X, Yan Y, Lu L, et al. HDAC10 expression is associated with DNA mismatch repair gene and is a predictor of good prognosis in colon carcinoma. Oncol Lett 2017;14:4923-9. [Crossref] [PubMed]
- Liu X, Wang Y, Zhang R, et al. HDAC10 Is Positively Associated With PD-L1 Expression and Poor Prognosis in Patients With NSCLC. Front Oncol 2020;10:485. [Crossref] [PubMed]
- Gu YY, Zhou GN, Li Y, et al. HDAC10 Inhibits Cervical Cancer Progression through Downregulating the HDAC10-microRNA-223-EPB41L3 Axis. J Oncol 2022;2022:8092751. [Crossref] [PubMed]
- Choi Y, Oh ST, Won MA, et al. Targeting ODC1 inhibits tumor growth through reduction of lipid metabolism in human hepatocellular carcinoma. Biochem Biophys Res Commun 2016;478:1674-81. [Crossref] [PubMed]
- Zhu HE, Yin JY, Chen DX, et al. Agmatinase promotes the lung adenocarcinoma tumorigenesis by activating the NO-MAPKs-PI3K/Akt pathway. Cell Death Dis 2019;10:854. [Crossref] [PubMed]
- Qiu L, Zhou R, Luo Z, et al. CDC27-ODC1 Axis Promotes Metastasis, Accelerates Ferroptosis and Predicts Poor Prognosis in Neuroblastoma. Front Oncol 2022;12:774458. [Crossref] [PubMed]
- Zhang Y, Cao L, Xie Y, et al. Agmatinase facilitates the tumorigenesis of pancreatic adenocarcinoma through the TGFβ/Smad pathway. Exp Ther Med 2022;24:490. [Crossref] [PubMed]
- Lee CY, Su GC, Huang WY, et al. Promotion of homology-directed DNA repair by polyamines. Nat Commun 2019;10:65. [Crossref] [PubMed]
- Ouameur AA, Tajmir-Riahi HA. Structural analysis of DNA interactions with biogenic polyamines and cobalt(III)hexamine studied by Fourier transform infrared and capillary electrophoresis. J Biol Chem 2004;279:42041-54. [Crossref] [PubMed]
- Saminathan M, Antony T, Shirahata A, et al. Ionic and structural specificity effects of natural and synthetic polyamines on the aggregation and resolubilization of single-, double-, and triple-stranded DNA. Biochemistry 1999;38:3821-30. [Crossref] [PubMed]
- Sierra JC, Suarez G, Piazuelo MB, et al. α-Difluoromethylornithine reduces gastric carcinogenesis by causing mutations in Helicobacter pylori cagY. Proc Natl Acad Sci U S A 2019;116:5077-85. [Crossref] [PubMed]
- Snyder RD. Effects of metal treatment on DNA repair in polyamine-depleted HeLa cells with special reference to nickel. Environ Health Perspect 1994;102:51-5. [PubMed]
- Chen DS, Mellman I. Elements of cancer immunity and the cancer-immune set point. Nature 2017;541:321-30. [Crossref] [PubMed]
- Casero RA Jr, Murray Stewart T, Pegg AE. Polyamine metabolism and cancer: treatments, challenges and opportunities. Nat Rev Cancer 2018;18:681-95. [Crossref] [PubMed]