RNA 5-Methylcytosine regulators are associated with cell adhesion and predict prognosis of endometrial cancer
Highlight box
Key findings
• The 5-Methylcytosine (m5C) modification pattern has the potential to be a prognostic marker for assessing endometrial cancer.
What is known and what is new?
• Development and progression of endometrial cancer are associated with m5C methylation.
• Diverse m5C modification patterns display distinct cell adhesion properties in uterine corpus endometrial carcinoma.
What is the implication, and what should change now?
• We found a correlation between cell adhesion-related pathways and m5C modification levels in endometrial cancer, thus providing a valuable reference for future studies.
Introduction
Uterine corpus endometrial carcinoma (UCEC) is a prevalent gynecological cancer, particularly in regions with moderate to high levels of development (1). It is projected that there will be approximately 65,950 new cases of UCEC and 12,550 deaths in the United States by 2022 (2). The mortality rate of endometrial cancer has experienced a yearly increase, reaching a rate of 1.4% in the year 2018 (3). Although our understanding of endometrial cancer has progressively improved, significant advancements in its treatment have yet to be achieved. The current treatment for endometrial cancer involves surgery, followed by postoperative adjuvant radiotherapy and chemotherapy, with chemotherapy being the primary approach (4). Surgical intervention is an effective treatment approach for low-grade endometrial cancer, achieving a 96.8% local control rate without the requirement of additional adjuvant therapies (5). High-grade endometrial cancer is commonly managed through a combined approach of post-surgical radiotherapy and chemotherapy, with carboplatin and paclitaxel being the first-line chemotherapy agents employed (6). Future advancements in endometrial cancer treatment are centered around targets like angiogenesis, DNA repair, and AKT serine/threonine kinase (7). Nevertheless, their practical application in clinical treatment remains limited.
Endometrial cancer staging currently relies on clinical features. However, there are instances where cancers diagnosed as advanced do not progress, while early-stage endometrial cancers progress to malignancy. As a result, formulating individualized treatments becomes crucial for patients. This relies on a more precise classification method. Thus, recent studies have started incorporating molecular analyses in conjunction with traditional prognostic factors. Based on an integration of numerous molecular phenotypes, The Cancer Genome Atlas (TCGA) has classified endometrial cancer into four categories (8). These categories include polymerase epsilon (POLE) ultramutated, microsatellite instability (MSI) hypermutated, copy-number (CN) low, and CN high. POLE ultramutated cases have the best prognosis, MSI hypermutated cases exhibit a good prognosis, CN low cases show a moderate prognosis, while CN high cases have the worst prognosis. However, due to its complexity and high cost, it is not currently feasible for clinical use. Currently, there is rapid development in epigenetic research, with epigenetic mechanisms playing significant roles in normal biological processes and tumor pathology. An increasing number of studies are now exploring the use of epigenetics in tumor classification and treatment. The extensively studied epigenetic mechanisms include DNA methylation, histone modification, chromatin reorganization, and non-coding RNAs, all of which significantly contribute to the risk stratification of UCEC (9-11). Despite RNA methylation being a recently studied epigenetic mechanism with prognostic relevance in many other tumors, 5-Methylcytosine (m5C) methylation has received limited attention in endometrial cancers.
Methylation modification is a major form of post-transcriptional RNA modification, which is still in its early stages. It encompasses various modifications such as, N1-methyladenosine (m1A), m5C, N6-Methyladenosine (m6A),7-Methylguanosine (m7G), and Um. Presently, research efforts on post-transcriptional RNA modifications have predominantly focused on m6A, with relatively limited investigations on m5C. Methylation of m5C involves the addition of a methyl group to the fifth carbon of the cytosine in the RNA sequence. This reversible process utilizes S-adenosylmethionine (SAM) as a carrier for methyltransferase, which facilitates the formation of m5C. The modified RNA then binds to a binding protein, enabling its functional role (12). This process includes writers (methyltransferases), erasers (demethyltransferases), and readers (associated binding proteins). Notably, this form of modification is widely observed in various types of RNA, particularly in tRNA and rRNA (13). RNA methylation has been shown to significantly impact RNA export capacity and stability. Dysregulation of RNA methylation modifications has been associated with a range of diseases, particularly tumors (14). For example, overexpression of NSUN2 in bladder cancer leads to aberrant methylation at the 3’UTR end, enhancing its interaction with Y-box binding protein 1 (YBX1). This interaction increases mRNA stability and promotes bladder cancer progression (15). Thus, m5C methylation plays a crucial role in tumorigenesis.
Cell adhesion primarily refers to intercellular adhesion and the adhesion of cells to the extracellular matrix (ECM), which is primarily mediated by cell adhesion molecules. Cell adhesion molecules can be classified into four major groups: calmodulin, integrins, selectins, and immunoglobulins (Igs). Cell adhesion molecules not only maintain cell adhesion-related functions but also mediate intracellular signaling pathways (16). Current cancer research is focused on calmodulin and integrins. Calmodulin is a class of calcium-dependent transmembrane proteins that hold cells together and maintain tissue stability (17). Integrin adhesion is a family of transmembrane multiprotein complexes that link the ECM (18). The aggregation of ligands and integrins and their related proteins activates downstream signaling pathways. After ligand binding, integrin complexes can be divided into canonical adhesions (CAs), hemidesmosomes, and reticular adhesions (RA). CAs can be further divided into focal adhesions (FAs), fibrillar adhesions and nascent focal complexes (19). Cell adhesion directly affects cell migration, proliferation, differentiation, growth, and the capacity for repair, and it has been shown to be associated with developmental and pathological processes (20). The association of synaptic extension and retraction of the cytosol, along with adhesion to specific enzymatic proteins through FA-related pathways, is recognized to be an essential part of cell migration. As a result, cell adhesions are crucial to tumor development (20). Hippo component YAP has been found to enhance breast cancer invasion by promoting FA pathways (21). The interaction between autophagy-associated protein 9B (ATG9B) and MYH9 promotes FAs and enhances metastasis in colorectal cancer (22). Recent tumor-related studies have found a close association between cell adhesion and m5C methylation. The pathways of FA, cell-substrate adhere junction, and cell adhesion molecule binding associated with m5C methylation suggest a prognostic diagnosis for Osteosarcoma (23). Furthermore, a significant association was observed between the Wnt signaling pathway, FA, and m5C methylation in high-grade plasmocytic ovarian cancer (24).
However, the impact of m5C methylation modification on UCEC remains unclear. In our study, we primarily analyzed the gene expression and genetic variation of m5C methylation regulators in UCEC, and we investigated the relationship between cell adhesion and m5C methylation in UCEC. This relationship may be associated with differences in patient survival. We present this article in accordance with the TRIPOD reporting checklist (available at https://tcr.amegroups.com/article/view/10.21037/tcr-23-742/rc).
Methods
Data resources
From TCGA database, fragments per kilobases per million mapped fragments (FPKM) files were downloaded, along with clinical information for 554 cases of UCEC and 35 normal cases. Matrix files in SOFT format for three Gene Expression Omnibus (GEO) datasets (GSE17025, GSE106191, and GSE115810) (25-27) were downloaded from GEO (https://www.ncbi.nlm.nih.gov/geo/). A copy number variation (CNV) database for UCEC was downloaded from Genomic Data Commons (http://portal.gdc.cancer.gov). This study was conducted in accordance with the Declaration of Helsinki (as revised in 2013).
RNA m5C regulators
In total, ten writers (DMNT3A, DMNT3B, NOP2, NSUN2, NSUN3, NSUN4, NSUN5, NSUN6, NSUN7, TRDMT1), four erasers (TET1, TET2, TET3, ALKBH1), and two readers (YBX1, ALYREF) have been identified for m5C regulators. It is important to note that DMNT3A and DMNT3B have only been reported in Arabidopsis thus far, therefore they will not be utilized in this paper (28).
Analysis of differentially expressed genes (DEGs)
The limma (29) package in R was utilized for the differential analysis of genes, while the ggplot2 package in R was mainly employed for principal component analysis (PCA). Additionally, the limma package was used to calculate the up-regulated DEGs within different clusters. DEGs were determined based on genes with a P value <0.05 and a fold change >2.
Interaction of m5C regulators
Based on STRING 11.0 b (https://string-db.org/) (30), the protein-protein interaction (PPI) network plots were generated. The relationship between mRNA expression and CNV levels of m5C regulators was assessed using the ggcorrplot (31) package in R.
Clustering analysis of 14 m5C regulators
Using the ConsensusClusterPlus (32) package in R, the clustering analysis of m5C regulators was performed. For accurate classification, the analysis was repeated 500 times based on the expression of 14 m5C regulators.
m5C regulators mutation and CNV analysis
The m5C regulator-associated somatic mutation and CNV analyses were conducted using the cBioPortal website (www.cbioportal.org) (33) for pan-cancer and UCEC. The somatic mutation and CNV analyses for pan-cancer were obtained from the TCGA database, while the mRNA and CNV correlation analyses for UCEC were obtained from the TCGA-UCEC database via the cBioPortal website. The correlation analysis was expressed as the Spearman correlation coefficient, with |Spearman| ≥0.8 indicating high correlation, 0.5≤ |Spearman| <0.8 indicating moderate correlation, 0.3≤ |Spearman| <0.5 indicating low correlation, and |Spearman| <0.3 indicating little to no correlation.
Cluster functional annotation and exploration of cluster cell adhesion pathway
After performing differential expression analysis using the limma package in R, we selected the top 1,000 differentially expressed genes per cluster for further Gene Ontology (GO) and Kyoto Encyclopedia of Genes and Genomes (KEGG) enrichment analysis using the Gene Set Variation Analysis (GSVA) package in R. We obtained marker gene sets for GO and KEGG from the MsigDB database (http://www.gsea-msigdb.org/gsea/msigdb). The functional annotation for the KEGG and GO analysis results was carried out using the ClusterProfiler (34) package in R, and gene set enrichment analysis (GSEA) was performed using the GSEABase package in R. Finally, we visualized the KEGG and GO analyses using the ggplot2 package in R.
Cell growth and cell culture
ISK and ECC1 cells were cultured using high sugar DMEM medium (MUTICELL, Nanjing, China) containing 10% fetal bovine serum (FBS; MUTICELL). The growth environment was 37 ℃ and 5% CO2 in a cell incubator.
Plasmid construction and transfection
The cDNA fragment of NSUN2 was inserted into the hU6-MCS-Ubiquitin-EGFP-IRES-puromycin vector and the plasmid was synthesized by JIKAI GENE (Shanghai, China) and the plasmid has been validated by DNA sequencing. Cell transfection was performed using Lipofectamine 2000 reagent (Invitrogen Life Technologies, Carlsbad, CA, USA). The sequence of short hairpin ribonucleic acid (shRNA) is as follows: shNSUN2: 5’- GAGCGATGCCTTAGGATATTA -3’.
Western blot and antibody
A 10% gel was prepared using the PAGE Gel Fast Preparation Kit (Epizyme, Shanghai, China). Total protein was extracted using RIPA lysis buffer (Beyotime, Shanghai, China) containing 1% phenylmethanesulfonyl fluoride (PMSF) and 0.1% protease inhibitor cocktail (Beyotime). Protein concentrations were determined using a BCA kit (Beyotime). A total of 25 µg of protein per well was sampled. After electrophoresis, proteins were transferred onto PVDF membranes activated with methanol (Millipore, Waltham, MA, USA). The transferred membrane was incubated in 5% skim milk at room temperature for 1 h, and subsequently rinsed with 0.1% Tris-HCl plus Tween-20 (TBST) three times for 5 min each. After blocking, the membranes were incubated with the primary antibody, washed three times with TBST, and further incubated with the specific secondary antibody. The membranes were tested using the ECL Luminol kit (BioVision, Palo Alto, CA, USA). Anti-NSUN2 (20854-1-AP) antibody and anti-GAPDH (60004-1-lg) antibody were supplied by Proteintech (Wuhan, China); Anti-rabbit (AS014) and anti-mouse (AS003) secondary antibodies were purchased from ABclonal (Wuhan, China).
Cell proliferation Cell Counting Kit-8 (CCK-8) assay
Cell proliferation was assessed using the CCK-8 (Beyotime). Transfected ISK and ECC1 cells were seeded into 96-well plates at a density of 2,000 cells/well with 4 replicates. Then, they were treated with CCK-8 reagent at various time points: 0, 24, 48, and 72 h. Microplate readers were used to measure optical density (OD) at 450 nm. DMEM containing 10% FBS was used as a blank control group.
Transwell cell migration assay
The upper cell chamber of the Transwell invasion assay was coated with a 60 µL solution of Matrigel (BD Pharmingen, San Jose, CA, USA) diluted in serum-free medium. The upper chamber was filled with 200 µL of serum-free medium containing 1×105 cells, while the lower chamber contained 700 µL of DMEM medium supplemented with 10% FBS. Following a 48-h incubation for the migration assay and a 72-h incubation for the invasion assay, the cells were fixed with 4% paraformaldehyde (Beyotime) for 30 minutes and then stained with 0.1% crystal violet (Beyotime) for 20 minutes. Finally, a random field of view was captured using a microscope (Leica, London, UK).
Statistical analysis
All experiments in this study were performed in triplicate, unless otherwise stated. Statistical analyses were performed using SPSS 19.0 software (SPSS, Chicago, IL, USA) and R 4.0.3. Graphs were generated using GraphPad Prism 8.0 software (San Diego, CA). Univariate and multivariate Cox regression analyses were conducted using the survival package (35) in R. Kaplan-Meier analysis of overall survival (OS) was performed using the survminer package (35) in R and Kaplan-Meier Plotter (36). Variables with P<0.1 in the results of the univariate Cox regression analysis were selected for least absolute shrinkage and selection operator (LASSO) regression using the glmnet package (37) in R. The ggplot2 package in R was used to visualize the risk factors. By using ggplot2 and the pROC (38) package in R, the receiver operating characteristic (ROC) curve’s area under the curve (AUC) was calculated. Forest plots are visualized using the ggplot2 package in R. A P value less than 0.05 was considered statistically significant.
Results
Gene expression and mutations of m5C regulators in endometrial cancer
Initially, we conducted a comprehensive analysis of the mutation, structural variant, and CNV data across multiple cancers. UCEC exhibited a high frequency of mutations and amplifications, positioning it as one of the most prevalent cancers among the pan-cancer cohort (Figure 1A). TET1, TET3, TET2, and NSUN2 were the prevailing eraser genes responsible for mutations and amplifications in UCEC, comprising 12%, 11%, 9%, and 9% of the cases, respectively (Figure 1B). Furthermore, we conducted an analysis of the gene expression patterns of m5C regulators in UCEC. The expression levels of writers were generally elevated, with the exception of NSUN3. TET3 displayed increased expression among the eraser genes. Additionally, the expressions of both YBX1 and ALYREF were significantly elevated in the reader genes (Figure 1C-1Q).
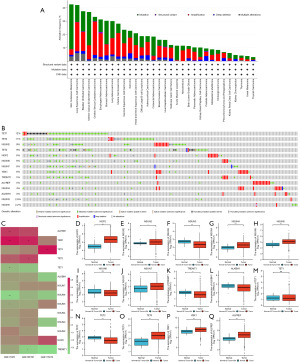
Analysis of m5C regulators interaction and correlation in endometrial cancer
In order to explore the interrelationships among m5C regulators in endometrial cancer, we conducted various analyses. Spearman correlation analysis was performed on the mRNA and CNV levels of m5C regulators. Based on the STRING 11.0 b website, we constructed a PPI network diagram to analyze the interrelationships among m5C regulators. The PPI network diagram reveals intricate connections between the reader and eraser genes, with a predominant association between readers and NOP2 (Figure 2A). Notably, there was a significant positive correlation in CNV between the writer and eraser genes, particularly TET1 and the writers (Figure 2B). Conversely, no significant correlation was observed between mRNA and CNV levels (Figure 2C). A significant correlation was found between the writer and reader genes, as well as the eraser and reader genes, at the mRNA level. Specifically, a significant positive correlation was observed between NSUN2 and readers, while a significant negative correlation existed between TET1 and readers. Additionally, there was a significant negative correlation between TET2 and NSUN5, while NSUN3 displayed a positive correlation (Figure 2D). These findings indicate the existence of a complex, yet closely interconnected relationship among m5C regulators.
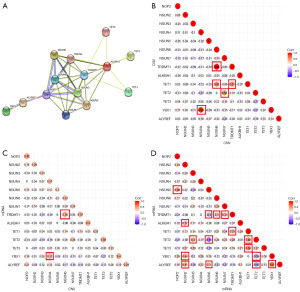
Consensus clustering analysis of m5C regulators in endometrial cancer defined two clusters with different OS
An unsupervised consensus clustering analysis was conducted on the expression matrix of m5C regulators in endometrial cancer sourced from the TCGA database (Figure 3A-3D). Among the range of k values tested (k=3–6), the clustering method with k=2 exhibited the highest stability (Figure S1A). Cluster 2 exhibited a generally higher expression of m5C regulators compared to cluster 1 (Figure 3E). Compared to cluster 1, cluster 2 had a significantly worse OS (P<0.0001) (Figure 3F).
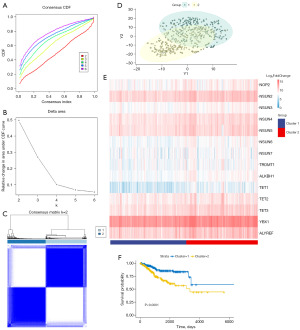
Functional annotation reveals two distinct clusters associated with cell adhesion
To study the impact of m5C methylation modification on the biological processes of UCEC, we conducted GO enrichment and KEGG analyses on the top 1,000 differentially expressed genes across the different clusters. The results indicated significant alterations in cell adhesion-related pathways within both cluster 1 and cluster 2 (Figure 4A-4D). Subsequently, we performed a differential analysis of the cell adhesion-related pathways in the two clusters with differential expression of m5C regulators (Table S1). Cytokine-cytokine receptor interaction (P=1.99E-19), the Wnt signaling pathway (P=1.65E-10), the MAPK signaling pathway (P=2.63E-05), actin binding (P=0.000765), FA (P=0.007493), and integrin binding (P=0.010301) exhibited significant differences. To investigate the relationship between cell adhesion-related pathways and m5C methylation, we calculated GSVA scores for marker gene sets associated with cell adhesion-related pathways. We conducted a correlation analysis to examine their relationship with mRNA expression levels of m5C regulators (Figure 4E). The findings revealed significant positive correlations between all m5C regulators and most cell adhesion-related pathways, except for NOP2 and NSUN7, which showed negative correlations with these pathways. To further explore the role of m5C methylation modification in endometrial cancer, we introduced shRNA into ISK and ECC1 cells to knock down NSUN2 expression. Western blotting confirmed a significant decrease in NSUN2 protein expression in ISK and ECC1 cells (Figure 4F). We subsequently conducted cell migration and invasion experiments. Compared to the control group, NSUN2 knockdown ISK and ECC1 cells exhibited a significant reduction in both migratory and invasive abilities (Figure 4G,4H). In conclusion, these results suggest that m5C methylation modification likely regulates cell adhesion ability.
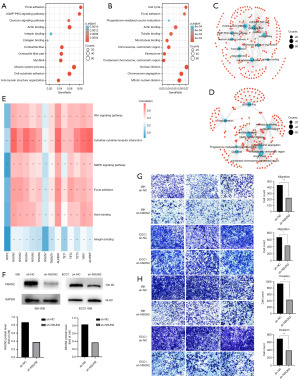
Cell adhesion-related genes with prognostic value under differential modification of m5C
Through overlap analysis, we identified cell adhesion-related genes that exhibited survival differences in the two clusters (Figure 5A). This analysis clarified the involvement of 25 cell adhesion-associated genes with prognostic value in differential m5C modifications. To visualize the impact of m5C modifications on cell adhesion genes and survival differences, we plotted a figure (Figure 5B). Additionally, we used a volcano map to visually represent nine representative genes (Figure 5C). Furthermore, we present the Kaplan-Meier analysis curves for these nine representative genes (Figure 5D-5L), while the remaining genes are shown in Figure S1B-S1Q.
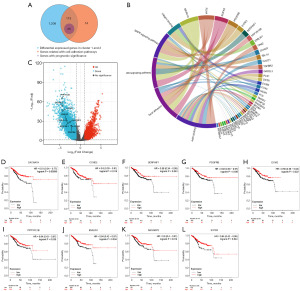
Establishing a risk score model associated with the m5C regulators
Given the alteration in cell adhesion-related pathways between clusters exhibiting different m5C modification patterns and their subsequent impact on prognosis, we conducted further investigation into the predictive value of m5C regulators for OS in endometrial cancer. Univariate Cox analysis of m5C regulators identified seven genes with P<0.1 (Table S2). These seven genes were then subjected to LASSO Cox regression analysis to construct a predictive model for OS-related risk scores, employing 10-fold cross-validation. Ultimately, NSUN2 and YBX1 were included in the final prediction model (Figure 6A-6C), which can be represented as (0.276) × NSUN2 + (0.1509) × YBX1. To evaluate the model’s efficacy, we determined cut-off values to categorize patients into high-risk and low-risk groups. Kaplan-Meier analysis demonstrated that patients in the high-risk group experienced significantly poorer OS (Figure 6D). Moreover, the predicted ROC curves for 3 and 5 years yielded an AUC of 0.626 and 0.638, respectively (Figure 6E). To further validate the significance of the key genes in the model, we conducted cell proliferation assays using ISK and ECC1 cells with NSUN2 knockdown. The results indicated a noticeable reduction in the proliferation ability of NSUN2 knockdown ISK and ECC1 cells (Figure 6F). In order to explore the additional value of m5C modification for risk prediction, we performed multivariate Cox analysis, establishing that m5C can serve as an independent risk factor (P=0.039, hazard ratio =1.673) (Figure 6G).
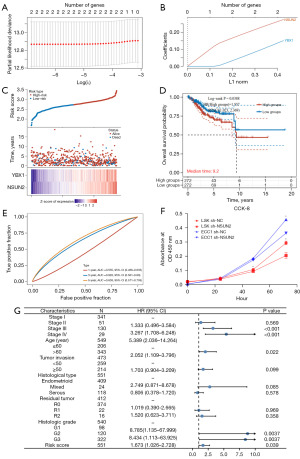
Discussion
The study of epigenetic mechanisms aims to explore individualized treatment options for different molecular subtypes of UCEC. Moreover, it has important clinical implications in various other areas. There is currently a trend of delayed childbearing in women, with 6.5% of UCEC patients being under the age of 45, and a significant proportion of these patients have not yet experienced childbirth (39). This group of patients strongly desires to preserve their fertility, resulting in an increasing demand for Fertility Sparing Treatments (FST). However, FST may lead to significant post-operative complications. Therefore, it is crucial to identify the appropriate candidates for FST. Molecular biology and epigenetics have now emerged as prognostic markers for UCEC, thereby providing physicians with additional standard options (40). Additionally, it is crucial to closely monitor UCEC patients during their follow-up. The concept of liquid biopsy has now been introduced in many tumor studies, involving the extraction of tumor derivatives from various liquid components. The current follow-up of UCEC patients relies on two serum tumor markers, CA125 and HE4. However, these markers face the challenge of achieving more accurate risk stratification for UCEC patients. Levels of cell free DNA (cfDNA) in uterine fluid have demonstrated superiority over CA125 and HE4 in predicting the prognostic repertoire of UCEC. Furthermore, they have significant potential in informing the indications for FST. However, its potential is currently limited due to the absence of prognostic markers that can be measured during disease progression (41). Therefore, there is a need to enhance our comprehension of epigenetic mechanisms and the epigenome for their clinical application. However, since epigenetic modifications do not modify the RNA sequence, the conventional detection of RNA mutations cannot elucidate epigenetic changes. There are ongoing clinical trials to develop inhibitors targeting the epigenome, signifying the need to intensify our exploration of epigenetic mechanisms (9,42,43).
Our study demonstrates a strong association between m5C regulator mRNA levels and cell adhesion-related pathways in endometrial cancer. We screened twenty-five cell adhesion genes associated with OS-related pathways in various clusters of m5C modification patterns. Furthermore, we investigated the prognostic value of m5C modification in UCEC and developed a risk prediction model comprising NSUN2 and YBX1, both of which demonstrate prognostic significance. Our findings indicate that mRNA levels of NSUN2 and YBX1 serve as valuable indicators for patients with poor prognosis. Previous studies have reported the diagnostic and prognostic significance of m5C modification in various cancers, including hepatocellular carcinoma (44), lung adenocarcinoma (45), colorectal cancer (46), cutaneous melanoma (47), clear cell renal cell carcinoma (48) and ovarian cancer (49). Our study aimed to investigate the diagnostic and prognostic significance of m5C modification in UCEC.
Loss of intercellular adhesion and anchoring-independent growth are characteristic features of tumors. Cell adhesion plays an increasingly important role in tumor pathogenesis. In our study, clusters with different m5C modification patterns were found to have alterations in cell adhesion-related pathways, and significant differences were observed between these clusters. One of these pathways is the MAPK signaling pathway, which includes various cascades involved in regulating intracellular signaling, such as cell adhesion, proliferation, migration, and differentiation (50). The Wnt signaling pathway, on the other hand, regulates the formation of calcium-dependent complexes between intercellular and intercellular E-calmodulin, thereby mediating homotypic intercellular adhesion (51). Among the proteins associated with cell adhesion-related pathways that we analyzed, most showed decreased expression in UCEC. For instance, CACNA1H regulates the activity of T-type calcium channels (52), and changes in Ca2+ levels can affect the activation of cell adhesion molecules (53). PDGFRB acts as a receptor for paracrine growth factors and interacts with integrins, which localizes PDGFRs to the beginning of FAs. This interaction can impact multiple signaling pathways and has important implications for cell migration and proliferation (54). ECM2, a member of the secreted protein acidic and rich in cysteine (SPARC)-related family, regulates the interaction between cells and the ECM and functions as a de-adhesive protein. Moreover, ECM2 can be used as a prognostic biomarker for low-grade gliomas, and high ECM2 expression promotes glioma proliferation, migration, and invasion (55,56). PPP1R12B is involved in the regulation of cell-matrix adhesion dynamics and traction force turnover (57,58). EMILIN1, an adhesive ECM glycoprotein, forms stable intra-chain salt bridges with α4β1 integrins, which enhance cell adhesion (59). RASGRP2 has been shown to participate in the cell adhesion process and can improve cell-matrix adhesion by increasing the levels of Ras and Rap1 in endothelial cells (60). Therefore, the different clusters identified in our study with distinct m5C modification patterns had varying prognoses due to differences in mRNA levels of cell adhesion-related factors. This suggests that alterations in cell adhesion have prognostic significance for UCEC.
Cell adhesion is observed in a diverse array of cancers. The prognostic value of the m5C-related cell adhesion pathways in tumors has also been documented. However, there is a notable absence of specific and relevant basic research on this topic. In our study, we discovered a correlation between the cell adhesion-related pathways in UCEC and m5C modification levels, thereby providing a valuable reference for future investigations.
Conclusions
In conclusion, our study examined the expression and interrelationship of m5C regulators in UCEC, and successfully constructed a prognostic prediction model for OS utilizing NSUN2 and YBX1. We discovered that m5C modification plays a significant role in cell adhesion-related pathways of UCEC. Our findings suggest that m5C methylation regulators hold potential as prognostic markers for assessing UCEC.
Acknowledgments
The authors acknowledge contributions from TCGA databases and GEO databases.
Funding: This work was sponsored by
Footnote
Reporting Checklist: The authors have completed the TRIPOD reporting checklist. Available at https://tcr.amegroups.com/article/view/10.21037/tcr-23-742/rc
Peer Review File: Available at https://tcr.amegroups.com/article/view/10.21037/tcr-23-742/prf
Conflicts of Interest: All authors have completed the ICMJE uniform disclosure form (available at https://tcr.amegroups.com/article/view/10.21037/tcr-23-742/coif). The authors have no conflicts of interest to declare.
Ethical Statement: The authors are accountable for all aspects of the work in ensuring that questions related to the accuracy or integrity of any part of the work are appropriately investigated and resolved. This study was conducted in accordance with the Declaration of Helsinki (as revised in 2013).
Open Access Statement: This is an Open Access article distributed in accordance with the Creative Commons Attribution-NonCommercial-NoDerivs 4.0 International License (CC BY-NC-ND 4.0), which permits the non-commercial replication and distribution of the article with the strict proviso that no changes or edits are made and the original work is properly cited (including links to both the formal publication through the relevant DOI and the license). See: https://creativecommons.org/licenses/by-nc-nd/4.0/.
References
- Koskas M, Amant F, Mirza MR, et al. Cancer of the corpus uteri: 2021 update. Int J Gynaecol Obstet 2021;155:45-60. [Crossref] [PubMed]
- Siegel RL, Miller KD, Fuchs HE, et al. Cancer statistics, 2022. CA Cancer J Clin 2022;72:7-33. [Crossref] [PubMed]
- Arend RC, Jones BA, Martinez A, et al. Endometrial cancer: Molecular markers and management of advanced stage disease. Gynecol Oncol 2018;150:569-80. [Crossref] [PubMed]
- Brooks RA, Fleming GF, Lastra RR, et al. Current recommendations and recent progress in endometrial cancer. CA Cancer J Clin 2019;69:258-79. [Crossref] [PubMed]
- Sznurkowski JJ, Rys J, Kowalik A, et al. The Polish Society of Gynecological Oncology Guidelines for the Diagnosis and Treatment of Endometrial Carcinoma (2023). J Clin Med 2023;12:1480. [Crossref] [PubMed]
- Fader AN, Roque DM, Siegel E, et al. Randomized Phase II Trial of Carboplatin-Paclitaxel Versus Carboplatin-Paclitaxel-Trastuzumab in Uterine Serous Carcinomas That Overexpress Human Epidermal Growth Factor Receptor 2/neu. J Clin Oncol 2018;36:2044-51. [Crossref] [PubMed]
- Lheureux S, McCourt C, Rimel BJ, et al. Moving forward with actionable therapeutic targets and opportunities in endometrial cancer: A NCI clinical trials planning meeting report. Gynecol Oncol 2018;S0090-8258(18)30124-0.
- Kandoth C, Schultz N, et al. Integrated genomic characterization of endometrial carcinoma. Nature 2013;497:67-73. [Crossref] [PubMed]
- Inoue F, Sone K, Toyohara Y, et al. Targeting Epigenetic Regulators for Endometrial Cancer Therapy: Its Molecular Biology and Potential Clinical Applications. Int J Mol Sci 2021;22:2305. [Crossref] [PubMed]
- Bartosch C, Lopes JM, Jerónimo C. Epigenetics in endometrial carcinogenesis - part 2: histone modifications, chromatin remodeling and noncoding RNAs. Epigenomics 2017;9:873-92. [Crossref] [PubMed]
- Piergentili R, Zaami S, Cavaliere AF, et al. Non-Coding RNAs as Prognostic Markers for Endometrial Cancer. Int J Mol Sci 2021;22:3151. [Crossref] [PubMed]
- Zhao BS, Roundtree IA, He C. Post-transcriptional gene regulation by mRNA modifications. Nat Rev Mol Cell Biol 2017;18:31-42. [Crossref] [PubMed]
- Zhang Q, Liu F, Chen W, et al. The role of RNA m(5)C modification in cancer metastasis. Int J Biol Sci 2021;17:3369-80. [Crossref] [PubMed]
- Nombela P, Miguel-López B, Blanco S. The role of m(6)A, m(5)C and Ψ RNA modifications in cancer: Novel therapeutic opportunities. Mol Cancer 2021;20:18. [Crossref] [PubMed]
- Chen X, Li A, Sun BF, et al. 5-methylcytosine promotes pathogenesis of bladder cancer through stabilizing mRNAs. Nat Cell Biol 2019;21:978-90. [Crossref] [PubMed]
- Janiszewska M, Primi MC, Izard T. Cell adhesion in cancer: Beyond the migration of single cells. J Biol Chem 2020;295:2495-505. [Crossref] [PubMed]
- Yu W, Yang L, Li T, et al. Cadherin Signaling in Cancer: Its Functions and Role as a Therapeutic Target. Front Oncol 2019;9:989. [Crossref] [PubMed]
- Revach OY, Grosheva I, Geiger B. Biomechanical regulation of focal adhesion and invadopodia formation. J Cell Sci 2020;133:jcs244848. [Crossref] [PubMed]
- Kamranvar SA, Rani B, Johansson S. Cell Cycle Regulation by Integrin-Mediated Adhesion. Cells 2022;11:2521. [Crossref] [PubMed]
- Mishra YG, Manavathi B. Focal adhesion dynamics in cellular function and disease. Cell Signal 2021;85:110046. [Crossref] [PubMed]
- Shen J, Cao B, Wang Y, et al. Hippo component YAP promotes focal adhesion and tumour aggressiveness via transcriptionally activating THBS1/FAK signalling in breast cancer. J Exp Clin Cancer Res 2018;37:175. [Crossref] [PubMed]
- Zhong Y, Long T, Gu CS, et al. MYH9-dependent polarization of ATG9B promotes colorectal cancer metastasis by accelerating focal adhesion assembly. Cell Death Differ 2021;28:3251-69. [Crossref] [PubMed]
- Zhang H, Xu P, Song Y. Machine-Learning-Based m5C Score for the Prognosis Diagnosis of Osteosarcoma. J Oncol 2021;2021:1629318. [Crossref] [PubMed]
- Meng L, Zhang Q, Huang X. Comprehensive Analysis of 5-Methylcytosine Profiles of Messenger RNA in Human High-Grade Serous Ovarian Cancer by MeRIP Sequencing. Cancer Manag Res 2021;13:6005-18. [Crossref] [PubMed]
- Day RS, McDade KK. A decision theory paradigm for evaluating identifier mapping and filtering methods using data integration. BMC Bioinformatics 2013;14:223. [Crossref] [PubMed]
- Sugiyama Y, Gotoh O, Fukui N, et al. Two Distinct Tumorigenic Processes in Endometrial Endometrioid Adenocarcinoma. Am J Pathol 2020;190:234-51. [Crossref] [PubMed]
- Hermyt E, Zmarzły N, Grabarek B, et al. Interplay between miRNAs and Genes Associated with Cell Proliferation in Endometrial Cancer. Int J Mol Sci 2019;20:6011. [Crossref] [PubMed]
- Chen YS, Yang WL, Zhao YL, et al. Dynamic transcriptomic m(5) C and its regulatory role in RNA processing. Wiley Interdiscip Rev RNA 2021;12:e1639. [Crossref] [PubMed]
- Ritchie ME, Phipson B, Wu D, et al. limma powers differential expression analyses for RNA-sequencing and microarray studies. Nucleic Acids Res 2015;43:e47. [Crossref] [PubMed]
- Szklarczyk D, Gable AL, Lyon D, et al. STRING v11: protein-protein association networks with increased coverage, supporting functional discovery in genome-wide experimental datasets. Nucleic Acids Res 2019;47:D607-13. [Crossref] [PubMed]
- GÃ3mez-Rubio V. ggplot2 - Elegant Graphics for Data Analysis (2nd Edition). Journal of statistical software. 2017;77: 1-3.
- Wilkerson MD, Hayes DN. ConsensusClusterPlus: a class discovery tool with confidence assessments and item tracking. Bioinformatics 2010;26:1572-3. [Crossref] [PubMed]
- Gao J, Aksoy BA, Dogrusoz U, et al. Integrative analysis of complex cancer genomics and clinical profiles using the cBioPortal. Sci Signal 2013;6:pl1. [Crossref] [PubMed]
- Yu G, Wang LG, Han Y, et al. clusterProfiler: an R package for comparing biological themes among gene clusters. OMICS 2012;16:284-7. [Crossref] [PubMed]
- Scrucca L, Santucci A, Aversa F. Competing risk analysis using R: an easy guide for clinicians. Bone Marrow Transplant 2007;40:381-7. [Crossref] [PubMed]
- Nagy Á, Munkácsy G, Győrffy B. Pancancer survival analysis of cancer hallmark genes. Sci Rep 2021;11:6047. [Crossref] [PubMed]
- Friedman J, Hastie T, Tibshirani R. Regularization Paths for Generalized Linear Models via Coordinate Descent. J Stat Softw 2010;33:1-22. [Crossref] [PubMed]
- Heagerty PJ, Zheng Y. Survival model predictive accuracy and ROC curves. Biometrics 2005;61:92-105. [Crossref] [PubMed]
- Floyd JL, Campbell S, Rauh-Hain JA, et al. Fertility preservation in women with early-stage gynecologic cancer: optimizing oncologic and reproductive outcomes. Int J Gynecol Cancer 2021;31:345-51. [Crossref] [PubMed]
- Cavaliere AF, Perelli F, Zaami S, et al. Fertility Sparing Treatments in Endometrial Cancer Patients: The Potential Role of the New Molecular Classification. Int J Mol Sci 2021;22:12248. [Crossref] [PubMed]
- Piñeiro-Pérez R, Abal M, Muinelo-Romay L. Liquid Biopsy for Monitoring EC Patients: Towards Personalized Treatment. Cancers (Basel) 2022;14:1405. [Crossref] [PubMed]
- Cuccu I, D'Oria O, Sgamba L, et al. Role of Genomic and Molecular Biology in the Modulation of the Treatment of Endometrial Cancer: Narrative Review and Perspectives. Healthcare (Basel) 2023;11:571. [Crossref] [PubMed]
- Golia D'Augè T, Cuccu I, Santangelo G, et al. Novel Insights into Molecular Mechanisms of Endometrial Diseases. Biomolecules 2023;13:499. [Crossref] [PubMed]
- He Y, Yu X, Li J, et al. Role of m(5)C-related regulatory genes in the diagnosis and prognosis of hepatocellular carcinoma. Am J Transl Res 2020;12:912-22. [PubMed]
- Sun L, Liu WK, Du XW, et al. Large-scale transcriptome analysis identified RNA methylation regulators as novel prognostic signatures for lung adenocarcinoma. Ann Transl Med 2020;8:751. [Crossref] [PubMed]
- Gong Y, Liu Y, Wang T, et al. Age-Associated Proteomic Signatures and Potential Clinically Actionable Targets of Colorectal Cancer. Mol Cell Proteomics 2021;20:100115. [Crossref] [PubMed]
- Huang M, Zhang Y, Ou X, et al. m5C-Related Signatures for Predicting Prognosis in Cutaneous Melanoma with Machine Learning. J Oncol 2021;2021:6173206. [Crossref] [PubMed]
- Wang G, Qu F, Liu S, et al. Nucleolar protein NOP2 could serve as a potential prognostic predictor for clear cell renal cell carcinoma. Bioengineered 2021;12:4841-55. [Crossref] [PubMed]
- Xu J, Liu X, Chen Y, et al. RNA 5-Methylcytosine Regulators Contribute to Metabolism Heterogeneity and Predict Prognosis in Ovarian Cancer. Front Cell Dev Biol 2022;10:807786. [Crossref] [PubMed]
- Asl ER, Amini M, Najafi S, et al. Interplay between MAPK/ERK signaling pathway and MicroRNAs: A crucial mechanism regulating cancer cell metabolism and tumor progression. Life Sci 2021;278:119499. [Crossref] [PubMed]
- Russell JO, Monga SP. Wnt/β-Catenin Signaling in Liver Development, Homeostasis, and Pathobiology. Annu Rev Pathol 2018;13:351-78. [Crossref] [PubMed]
- Harding EK, Zamponi GW. Central and peripheral contributions of T-type calcium channels in pain. Mol Brain 2022;15:39. [Crossref] [PubMed]
- Sheng L, Leshchyns'ka I, Sytnyk V. Cell adhesion and intracellular calcium signaling in neurons. Cell Commun Signal 2013;11:94. [Crossref] [PubMed]
- Pandey P, Khan F, Upadhyay TK, et al. New insights about the PDGF/PDGFR signaling pathway as a promising target to develop cancer therapeutic strategies. Biomed Pharmacother 2023;161:114491. [Crossref] [PubMed]
- Cheng X, Liu Z, Liang W, et al. ECM2, a prognostic biomarker for lower grade glioma, serves as a potential novel target for immunotherapy. Int J Biochem Cell Biol 2023;158:106409. [Crossref] [PubMed]
- Li J, Wang S, He Q, et al. High ECM2 Expression Predicts Poor Clinical Outcome and Promotes the Proliferation, Migration, and Invasiveness of Glioma. Brain Sci 2023;13:851. [Crossref] [PubMed]
- Fokkelman M, Balcıoğlu HE, Klip JE, et al. Cellular adhesome screen identifies critical modulators of focal adhesion dynamics, cellular traction forces and cell migration behaviour. Sci Rep 2016;6:31707. [Crossref] [PubMed]
- Yu X, He L, Chen Y, et al. Construction of a focal adhesion signaling pathway-related ceRNA network in pelvic organ prolapse by transcriptome analysis. Front Genet 2022;13:996310. [Crossref] [PubMed]
- Capuano A, Fogolari F, Bucciotti F, et al. The α4β1/EMILIN1 interaction discloses a novel and unique integrin-ligand type of engagement. Matrix Biol 2018;66:50-66. [Crossref] [PubMed]
- Zhao S, Jin X, Xu S. Expression of RASGRP2 in Lung Adenocarcinoma and Its Effect on Immune Microenvironment. Zhongguo Fei Ai Za Zhi 2021;24:404-11. [PubMed]