Comprehensive analysis identifies DNA damage repair-related gene HCLS1 associated with good prognosis in lung adenocarcinoma
Highlight box
Key findings
• The risk score was an independent overall survival predictor. The established model was robust and could independently determine the clinical outcomes of patients.
• Immune cell infiltration, immune markers, and the tumor microenvironment were significantly related to DNA damage repair (DDR)-associated genes in lung adenocarcinoma (LUAD).
• HCLS1 expression was downregulated and associated with clinicopathological features in LUAD samples.
• HCLS1 high-expression group showed higher immune-related gene expression and ESTIMATE scores.
What is known and what is new?
• LUAD, the primary pathological lung cancer form, is the leading cause of cancer-related death across most countries.
• Our study provided novel insights into the prognostic performance of DDR and the potential role of HCLS1 within LUAD tumor immunity.
What is the implication, and what should change now?
• HCLS1 is a promising biomarker to predict LUAD patient prognosis.
Introduction
Lung cancer is a very common malignant disease worldwide (1). Lung adenocarcinoma (LUAD), the primary pathological type of lung cancer, remains the leading cause of cancer-related death in several countries (2). Despite advances in medical imaging and treatment options, the 5-year survival rate of LUAD remains low (3). Recent studies have shown that autophagy- (4), inflammation- (5), ferroptosis- (6), and hypoxia-related signatures (7) could work as prognostic markers to predict the prognosis of LUAD patients. However, tumor heterogeneity may benefit individual patients, and these markers are not used in routine clinical practice due to small sample sizes, inconsistent data, and insufficient evidence.
DNA damage is unavoidable in many biological activities, and protective cellular responses to DNA-damaging agents are required to maintain genome stability. DNA damage repair (DDR) is an essential process in organisms that maintains the integrity and stability of DNA structure, ensuring the continuation of life and species stability (8). The disruption of the DDR process is closely associated with the inability to accurately repair damaged DNA within cells, transforming normal cells into cancer cells and accumulating genetic changes (9). Therefore, incorporating DDR-associated genes into cancer progression and prognosis studies could provide new insights into the clinical management of cancer patients. Studies have demonstrated that DDR is related to chemotherapy resistance (10), metastasis (11), and prognosis (12) in LUAD. In the present study, DNA damage repair-related genes (DRGs) were extensively analyzed to investigate their effect on the tumor microenvironment (TME) and survival of LUAD patients. Furthermore, a DDR-based risk score model was established to determine the prognostic value of DRGs in LUAD. This study provides new clues for exploring the molecular mechanism of LUAD, yields novel ideas for targeted therapy strategies in LUAD, and promotes personalized patient care.
HCLS1 is a cortactin homolog expressed specifically in hematopoietic cells. Cavnar et al. found that in chronic lymphocytic leukemia, the level of HS1 phosphorylation in leukemic cells was associated with clinical prognosis, whereas HS1 hyperphosphorylation was associated with poor prognosis (13). Furthermore, HCLS1 knockdown reduced the rolling, adhesion, and migration of neutrophils on the endothelial cell layer in a mouse model (14). However, HCLS1’s correlation with prognosis and immune microenvironment has not been elucidated. This study used bioinformatics to determine HCLS1 expression, its prognostic value in LUAD tissues, and its correlation with immune cell infiltration in tumors. Studying the biological role of HCLS1 in LUAD could help the diagnosis, treatment, and prognosis prediction of LUAD. We present this article in accordance with the TRIPOD reporting checklist (available at https://tcr.amegroups.com/article/view/10.21037/tcr-23-921/rc).
Methods
Data retrieval
The normalized RNA-sequencing datasets (N=502) and clinically relevant information of LUAD samples (N=522) were retrieved from The Cancer Genome Atlas (TCGA) database (https://portal.gdc.cancer.gov/). Moreover, the mRNA expression data of GSE68465 (n=462) (15), GSE31210 (n=246) (16), and GSE72094 (n=442) (17) were obtained from the Gene Expression Omnibus (GEO) database as validation groups (https://www.ncbi.nlm.nih.gov/geo/). The detailed dataset information is represented in Table 1. The study was conducted in accordance with the Declaration of Helsinki (as revised in 2013).
Table 1
Consensus clustering
Consensus clustering (unsupervised clustering) enables common cancer-type samples to be differentiated into different subtypes according to different omics datasets to discover novel disease subtypes or compare different subtypes through the subtype classification method (18). This process was repeated 1,000 times to estimate the optimal number of clusters in the range k =2–10 and ensure result stability. The principal component analysis (PCA) package in R was utilized to evaluate gene expression arrays in the LUAD groups. Differences in clinical outcomes among the three clusters were assessed using Kaplan-Meier survival analysis.
Identification of differentially expressed genes (DEGs) and functional enrichment analyses
The Limma package helped compare differences between the healthy lung tissue and LUAD samples, and adjusted P<0.05 and |log FC| >1 were used as the cutoff criteria for statistically significant differences. To investigate the biological significance of DEGs, the pathway and functional enrichment were analyzed using the R packages “clusterProfiler” and “org.Hs.eg.db,” including analysis and visualization capabilities, in addition, to Gene Ontology (GO) and Kyoto Encyclopedia of Genes and Genomes (KEGG) analysis. Gene set variation analysis (GSVA) was performed using the “GSVA” R package to visualize changes in the signaling pathways between HCLS1 high- and low-expression groups.
Establishment and validation of the DRG prognostic model
Univariate Cox regression analysis helped analyze the association of age, sex, disease severity, risk score, and prognosis in patients with LUAD and HCLS1 high- and low-expression groups. Multivariate survival analysis using Cox regression was performed to assess which clinical factors alone predicted clinical outcomes in LUAD patients; hazard ratio (HR), 95% confidence interval (CI), and P values were also calculated. The least absolute shrinkage and selection operator (LASSO) regression model was used to avoid overfitting, and the glmnet R package was utilized to select the best candidate genes to enter the predictive model (19). Kaplan-Meier survival curves were generated using the “survminer” and “survival” R packages. Finally, time-dependent receiver operating characteristic (ROC) curves helped assess the predictive power of the predictive model using the “survival ROC” R package (20).
Exploration of the TME landscape
The immune score, stromal score, ESTIMATE score, and tumor purity of each sample belonging to different groups were evaluated using the “estimate” package in R. The composition of 22 tumor-infiltrating immune cells between the different groups was determined using the CIBERSORT algorithm. Furthermore, Spearman’s correlation analysis was performed among 30 types of immune checkpoint expression, 19 human leukocyte antigen (HLA)-related molecules, and the different groups using the R package “ggstatsplot”.
Construction and evaluation of nomogram
Seven independent prognostic factors, such as significant clinical characteristics and calibration plots, were added to a nomogram model to predict 1-, 3-, and 5-year survival. The calibration plots were developed using the rms R package (version 5.1–3; https://cran.r-project.org/web/packages/rms/index.html) to assess the relationship between actual survival and nomogram-predicted survival.
Statistical analyses
Statistical analyses were performed using R (version 4.1.3) and GraphPad Prism 8. Logistic regression analysis was performed in SPSS (version 26.0). Two groups were analyzed with Student’s t-test, and more than two groups were analyzed using the one-way analysis of variance. P<0.05 indicated statistical significance, and all analyses were performed with two-sided tests.
Results
Consensus clustering identified three DDR-associated subtypes
Around 150 DNA repair genes were retrieved from the Molecular Signatures Database (MSigDB) for use with the Gene Set Enrichment Analysis database. First, the expression patterns of DDR genes in LUAD and healthy samples were examined, revealing the overexpression of most DRGs, including NME1, ZWINT, POLR2H, TYMS, DGUOK, POLR1C, NME4, FEN1, CSTE3, RPA3, PCNA, and UPE3B, in LUAD (Figure 1A). Next, the patients were classified depending on the consistent clustering of 150 DRG expression profiles. The cumulative distribution function (CDF) curve and consensus heatmap revealed the optimal number of clusters as 3 (k value =3). The results included 128 samples in DDR cluster 1, 170 in cluster 2, and 155 in cluster 3 (Figure 1B-1D). The heatmap displayed the difference in the DRG expression in the three clusters (Figure 1E). The Kaplan-Meier survival analysis showed that the overall survival (OS) of cluster 2 was significantly better than clusters 1 and 3 (Figure 1F). To understand the molecular mechanisms underlying prognosis regulation in the three subtypes, 310 DEGs were evaluated, indicating 47 overlapping DEGs in the three clusters (Figure 1G). Then, GO and KEGG analyses were performed to obtain a novel understanding of the biological effects of the DEGs. As shown in Figure 1H,1I, the subclass-specific genes were primarily enriched in the DNA metabolic process, DNA replication, DNA-dependent DNA replication, DNA biosynthetic process, nuclear DNA replication, cell cycle DNA replication, nucleotide-excision repair, telomere maintenance through semiconservative replication, transcription-coupled nucleotide-excision repair, nucleotide-excision repair, and DNA gap-filling.
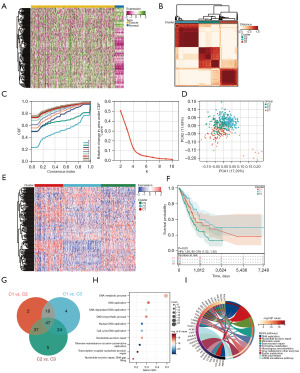
Patients in the three molecular subtypes exhibited different TME and immune status
TME is crucial in malignant tumor progression and can effectively block the immune system from attacking the tumors. Thus, the microenvironment composition of tumors among the three genomic subtypes was next evaluated. To calculate the immune scores of the molecular subtypes, ESTIMATE was used to assess the stromal score, immune score, and ESTIMATE score. The analysis showed that cluster 3 had the highest immune, stromal, and ESTIMATE scores, followed by clusters 2 and 1 (Figure 2A). With the significant difference in immune score identified among the subclasses, the CIBERSORT method was combined with the LM22 signature matrix to evaluate the difference in the immune infiltration of 22 immune cells. It was found that patients in cluster 2 exhibited considerably elevated percentages of naïve B cells, memory B cells, plasma cells, resting memory CD4 T cells, Tregs, activated NK cells, monocytes, resting dendritic cells, and resting mast cells compared to those in clusters 3 and 1. In contrast, the best prognosis for patients in cluster 3 was related to enriching many activated memory CD4 T cells, M0 macrophages, and activated dendritic cells (Figure 2B). Considering the predictive role of immune checkpoint inhibitors (ICIs) on the effect of immunotherapy, the difference in ICI expression among different subclasses was assessed, revealing a clear improvement in cluster 3 expression (Figure 2C). Furthermore, targeting programmed death ligand 1 (PD-L1) has demonstrated promise in advanced NSCLC patients. Therefore, we evaluated the expression of PD-L1 in LUAD, which was the lowest in cluster 2 (Figure 2D). Besides, significant differences were found in HLA genes among the three groups (Figure 2E).
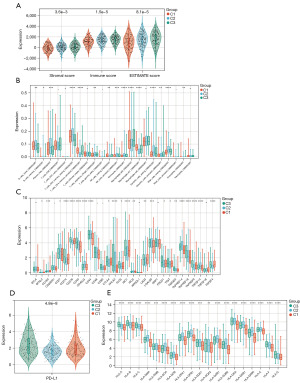
Construction of a DRG prognostic signature in the TCGA cohort
A predictive model was established using Cox univariate analysis according to the expression levels of 150 DDR-related genes; 25 DDR-related genes were considerably associated with the OS of patients (Figure 3A). Using the LASSO algorithm with an optimal lambda value of 0.030, 11 DDR-related genes were identified as potential prognosis-related genes (Figure 3B). From the LASSO-based Cox regression analysis, the risk score for each patient was calculated. The patients were classified into two groups based on the median value of the risk score (Figure 3C). Kaplan-Meier curves indicated that patients in the low-risk group had significantly higher OS than those in the high-risk group (Figure 3D). For the 1-, 3-, and 5-year OS rates, the predicted areas under the curve (AUC) were 0.69, 0.68, and 0.72, respectively (Figure 3E). In addition, the model established using the GEO cohorts GSE31210, GSE68465, and GSE72094 was validated (Figure 4), which revealed that the prognosis of high-risk patients was significantly worse than that of low-risk patients (Figure 4A,4C,4E). Simultaneously, ROC curves for 1-, 3-, and 5-year OS prediction depicted a predictive accuracy of AUC >0.700 (Figure 4B,4D,4F).
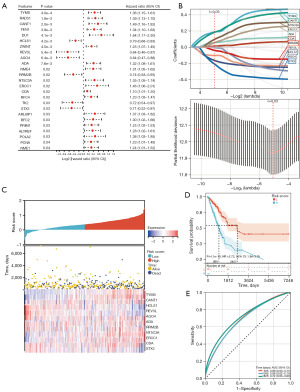
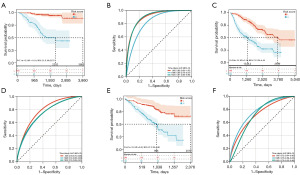
Relationship between risk score and immune cell infiltration
Due to the essential biological role of DRGs in antitumor immune responses, the relationship between DRG risk scores and TME was extensively evaluated. Among the three clustering subgroups, cluster 3 patients had the highest risk scores, and the risk score could differentiate patients into different subtypes (Figure 5A). The ESTIMATE algorithm results showed that the stromal, immune, and ESTIMATE scores of the high-risk group were lower than those of the low-risk group (Figure 5B).
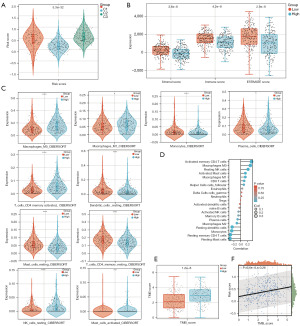
Among the immune cell infiltration signatures, the low-risk group was related to a higher infiltration of plasma cells, resting memory CD4 T cells, monocytes, resting dendritic cells, and resting mast cells and lower infiltration of activated memory CD4 T cells, resting NK cells, M0 macrophages, M1 macrophages, and activated mast cells (Figure 5C). In addition, according to the CIBERSORT analysis, the risk score was positively correlated with CD8 T cells, resting NK cells, follicular helper T cells, M1 macrophages, M0 macrophages, activated memory CD4 T cells, and activated mast cells. By contrast, the risk score was negatively correlated with resting dendritic cells, monocytes, resting mast cells, resting memory CD4 T cells, M2 macrophages, and plasma cells (Figure 5D). Clinical studies have revealed that somatic tumor mutational burden (TMB) is highly sensitive to immunotherapy and strongly correlates with treatment intensity and survival. TMB levels in low-risk patients were low (Figure 5E), and Spearman’s correlation analysis confirmed that the risk signature was positively associated with TMB (Figure 5F).
Relationships between different clinicopathological factors and risk scores
Univariate and multivariate Cox regression analyses helped assess whether the risk score was an independent clinical prognostic factor. The univariate analysis demonstrated that a high-risk score was significantly correlated with shorter OS (HR =4.56, 95% CI: 2.77–7.50, P=2.4e−9) (Figure 6A). Furthermore, multivariate analysis showed that the risk score was an independent prognostic factor in LUAD patients (HR =4.23, 95% CI: 2.55–7.02, P=2.2e−8) (Figure 6B).
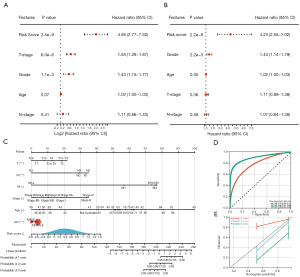
A nomogram with a model containing the factors of age, sex, tumor grade, TNM stage, and prognostic risk score was established for OS prediction in LUAD patients (Figure 6C). The AUCs of the 1-, 3-, and 5-year survival of the constructed nomogram were 0.78, 0.90, and 0.90, respectively (Figure 6D), indicating a good prediction performance of the model. The C-index of the nomogram was 0.6969 with 1,000 bootstrap replicates (95% CI: 0.7823–0.8607). The bias-corrected line on the calibration plot was close to the ideal curve (45° line), indicating good agreement between predictions and observations (Figure 6E). Therefore, the nomogram is stable and robust for predicting the probability of survival in LUAD patients.
Expression of HCLS1 in LUAD and its prognostic value
According to the LASSO-based Cox regression analysis, 11 DDR-related genes were selected as potential prognosis-related genes, validated to play an essential role in LUAD. However, the role of HCLS1 in tumor immunity remains unclear. The Gene Expression Profiling Interactive Analysis 2 (GEPIA2; http://gepia2.cancer-pku.cn/) web server helped compare the boxplots of mRNA expression differences between LUAD and normal tissues (Figure 7A). HCLS1 was expressed at low levels in LUAD patients. To evaluate the expression of HCLS1 at the protein level, IHC results provided by the human protein atlas (HPA) database (version 20.1; https://www.proteinatlas.org/) were assessed. As shown in Figure 7B, HCLS1 protein levels were not detected in LUAD tissues, whereas it was moderately expressed in healthy lung tissues. Further, immunofluorescence analysis indicated that HCLS1 was mainly distributed in the plasma membrane and cytosol in U2OS cells (Figure 7C). The association between HCLS1 expression levels and different tumor pathological stages was evaluated with the “pathological stage plot” module of the GEPIA2 web server (http://gepia2.cancer-pku.cn/#analysis). The low expression of HCLS1 was correlated with advanced clinical stages and poor OS (Figure 7D,7E). The diagnostic value of HCLS1 in LUAD evaluated using ROC curves revealed that HCLS1 had a moderate diagnostic accuracy (AUCs >0.7; Figure 7F).
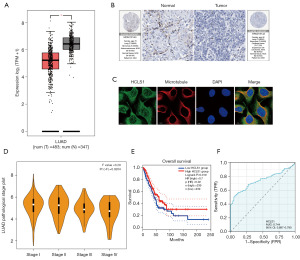
Correlation of HCLS1 expression with immune infiltration and TME in LUAD
The expression of HCLS1 in high- and low-risk patients was analyzed, indicating higher expression in the low-risk group (Figure 8A). To further clarify differences in the TME of patients in the HCLS1 low- and high-expression groups, a correlation analysis was performed between HCLS1 and different immune cells. The results indicated that HCLS1 expression levels were positively associated with the infiltration level of resting memory CD4 T cells, memory B cells, M1 macrophages, CD8 T cells, activated memory CD4 T cells, and Tregs. Moreover, the expression was negatively related to the infiltration levels of naïve B cells, follicular helper T cells, activated dendritic cells, activated mast cells, eosinophils, and activated NK cells (Figure 8B). Subsequently, the ESTIMATE results suggested that the stromal, immune, and ESTIMATE scores were higher in the high-expression group (Figure 8C). The analysis of different immune checkpoint-related genes and HLA genes in HCLS1 high- and low-expression groups revealed that the expression of these genes in the high-expression group was higher than that in the low-expression group (Figure 8D,8E). Cancer-associated fibroblasts (CAFs), major cellular components in TME, play a crucial role in tumor progression. The present study detected a positive correlation between HCLS1 expression and CAF infiltration score in LUAD with the TIMER 2.0 database (Figure S1). To further explore the relationship between the abundant signaling pathways and the prognosis of LUAD patients, the relative differences in the expression of signaling pathways between the two groups were evaluated using GSVA. GSVA identified many differentially expressed signaling pathways, visualized using a heatmap (Figure 8F). Compared with the low-expression group, immune pathways were significantly higher in the high-expression group. These results suggest that HCLS1 may regulate immune cell infiltration into LUAD, exerting specific effects on TME. Univariate analysis results revealed that HCLS1 expression [P=8.7e−3 and HR =0.78 (95% CI: 0.65–0.94)], grade [P=0.02 and HR =1.33 (95% CI: 1.05–1.69)], and N-stage [P=0.03 and HR =1.32 (95% CI: 1.03–1.71)] were significantly associated with OS in LUAD patients (Figure 8G). Multivariate analyses confirmed that HCLS1 expression [P=0.02 and HR =0.80 (95% CI: 0.67–0.96)] and grade [P=7.2e−3 and HR =1.37 (95% CI: 1.09–1.71)] were independent prognosis factors in LUAD patients (Figure 8H).
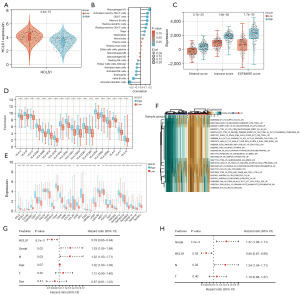
Discussion
LUAD is the most common subtype of lung cancer, accounting for 35–40% of all cases. The high mortality rate of patients with LUAD suggests a need to identify useful prognostic markers to help predict disease outcomes and develop targeted therapies (21). Recently, ICIs have achieved remarkable clinical efficacy in various malignancies and fundamentally changed the cancer treatment paradigm (22). Although ICIs may confer significant clinical benefit in a small subset of patients with LUAD, it is difficult to identify which group of patients are likely to respond to ICIs owing to the lack of clinically available predictive biomarkers. Therefore, it is essential to find biomarkers that can predict the prognosis of LUAD and improve the clinical outcomes of patients with LUAD. DRGs play a crucial role in the development and progression of spontaneous cancers and the control of cell growth and proliferation (23). A comprehensive understanding of DRG expression profiles in LUAD samples may provide new insights into improving clinical patient outcomes. The present study results indicated that the expression of DRGs is closely associated with the prognosis and TME of LUAD and elucidated the vital role of the key DDR-related gene HCLS1 in LUAD.
Three subgroups were identified using consensus clustering according to DRG expression. These three subtypes exhibited significantly different prognoses and distinct immunophenotypes. In addition, the TME characteristics of the three subtypes were significantly different. The infiltration abundance of activated memory CD4 T cells, M0 macrophages, and activated dendritic cells in cluster 3 were higher than the same cells in clusters 2 and 3. Most immune checkpoint and HLA genes exhibited higher expression in cluster 3. Moreover, cluster 3 had the highest immune, stromal, and ESTIMATE scores, followed by clusters 2 and 1. These results explain why cluster 3 had a significant survival advantage. However, cluster 2, which exhibited some immune cell infiltration, did not have the same survival advantage.
The prognostic risk signature of 11 selected genes was established and validated to classify LUAD patients into high- and low-risk cohorts. This signature classified patients into the different high and low-risk OS groups, and its predictive ability was validated with data from the GSE31210, GSE68465, and GSE72094 databases. In addition, the risk scores were identified as independent prognostic factors. Then, differences in immune infiltrate fractions between the two risk groups were assessed. The low-risk group showed greater immune cell infiltration, particularly plasma cells, resting memory CD4 T cells, monocytes, resting dendritic cells, and resting mast cells. In addition, the immune, stromal, and ESTIMATE scores were higher in the low-risk group than those in the high-risk group.
TMB is a hallmark of immunotherapy through biological mechanisms and somatic mutations in the immune response (24,25). In this study, the TMB value of the high-risk group was higher and had a significant positive correlation with the risk score.
HCLS1, a 75 kDa intracellular protein, is primarily expressed in hematopoietic cells. It is involved in many cellular processes, and its role in cell motility is well known, particularly in actin reorganization (13,14). Studies have revealed that HCLS1 actin filaments are involved in the motility of NK cells, DCs, and neutrophils (26). It also plays a vital role in regulating immune T-cell synapses. However, the prognostic correlation between HCLS1 expression and immune infiltration in LUAD has not been reported. TCGA datasets were analyzed in the current study, validating the distinct reduction of HCLS1 expressions in LUAD specimens. In addition, survival assays indicated that patients with low HCLS1 expression had a shorter OS than those with high HCLS1 expression. Thus, HCLS1 expression was positively correlated with tumor stage in advanced LUAD patients.
The correlation analysis between HCLS1 expression and immune system infiltration in LUAD revealed that stromal, immune, and ESTIMATE scores, along with the expression of immune checkpoint genes, were higher in the high-expression groups. In addition, there was a positive correlation between HCLS1 expression and infiltration abundance of M1 macrophages, activated memory CD4 T cells, CD8 T cells, memory B cells, resting dendritic cells, resting memory CD4 T cells, and Tregs. Therefore, the poor LUAD patient prognosis showed low HCLS1 expression. CAFs are the essential components of cancer cells and have been reported to be associated with poor prognosis, chemotherapy resistance, and recurrence in several cancers (27). The TIMER 2.0 database revealed a significantly positive correlation between the expression of HCLS1 and the infiltration value of CAFs in LUAD. GSVA demonstrated that immune pathways were significantly enriched in the HCLS1 high-expression phenotype. The above results suggest that HCLS1 plays a vital role in tumor immunity, opening a new research direction in LUAD.
Poly (ADP-ribose) polymerase (PARP) is a nuclear enzyme activated during DNA damage in eukaryotic cells (28). PARP inhibitors (PARPi) are combined with radiation therapy to inhibit DNA repair functions. This enhances enhancing radiation effects, thereby interacting with the antitumor immune response (29). Next, a PARPi-related prognostic model is urgently needed to improve treatment strategies.
Our study has some limitations. First, the small sample size and self-queuing retrospective design could lead to bias in patient selection. Therefore, these findings need to be validated with larger samples. Second, since the association between HCLS1 and LUAD-infiltrating immune cells has been established using a cancer database and bioinformatics, the immunomodulatory function of HCLS1 needs to be validated through in vivo and in vitro experiments. Finally, the downstream factors of HCLS1 regulation were not assessed. Thus, the action mechanism of HCLS1 in LUAD requires further exploration.
Conclusions
A signature of 150 DNA repair genes was constructed to predict LUAD. This feature was validated to accurately and independently predict patient outcomes. Nomograms combining the characteristics and stages of LUAD were also developed as individual clinical predictors. Furthermore, the study results indicated that HCLS1 may be involved in the immunoenhancement of TME and related to high response to immunotherapy. Thus, HCLS1 could be a novel diagnostic and prognostic biomarker and therapeutic target in LUAD.
Acknowledgments
Funding: This work was supported by grants from
Footnote
Reporting Checklist: The authors have completed the TRIPOD reporting checklist. Available at https://tcr.amegroups.com/article/view/10.21037/tcr-23-921/rc
Peer Review File: Available at https://tcr.amegroups.com/article/view/10.21037/tcr-23-921/prf
Conflicts of Interest: All authors have completed the ICMJE uniform disclosure form (available at https://tcr.amegroups.com/article/view/10.21037/tcr-23-921/coif). The authors have no conflicts of interest to declare.
Ethical Statement:
Open Access Statement: This is an Open Access article distributed in accordance with the Creative Commons Attribution-NonCommercial-NoDerivs 4.0 International License (CC BY-NC-ND 4.0), which permits the non-commercial replication and distribution of the article with the strict proviso that no changes or edits are made and the original work is properly cited (including links to both the formal publication through the relevant DOI and the license). See: https://creativecommons.org/licenses/by-nc-nd/4.0/.
References
- Hirsch FR, Scagliotti GV, Mulshine JL, et al. Lung cancer: current therapies and new targeted treatments. Lancet 2017;389:299-311. [Crossref] [PubMed]
- Wu P, Zheng Y, Wang Y, et al. Development and validation of a robust immune-related prognostic signature in early-stage lung adenocarcinoma. J Transl Med 2020;18:380. [Crossref] [PubMed]
- Denisenko TV, Budkevich IN, Zhivotovsky B. Cell death-based treatment of lung adenocarcinoma. Cell Death Dis 2018;9:117. [Crossref] [PubMed]
- Gong Z, Li Q, Li J, et al. A novel signature based on autophagy-related lncRNA for prognostic prediction and candidate drugs for lung adenocarcinoma. Transl Cancer Res 2022;11:14-28. [Crossref] [PubMed]
- Liu F, Wu H. CC Chemokine Receptors in Lung Adenocarcinoma: The Inflammation-Related Prognostic Biomarkers and Immunotherapeutic Targets. J Inflamm Res 2021;14:267-85. [Crossref] [PubMed]
- Ren Z, Hu M, Wang Z, et al. Ferroptosis-Related Genes in Lung Adenocarcinoma: Prognostic Signature and Immune, Drug Resistance, Mutation Analysis. Front Genet 2021;12:672904. [Crossref] [PubMed]
- Chen J, Fu Y, Hu J, et al. Hypoxia-related gene signature for predicting LUAD patients' prognosis and immune microenvironment. Cytokine 2022;152:155820. [Crossref] [PubMed]
- Carusillo A, Mussolino C. DNA Damage: From Threat to Treatment. Cells 2020;9:1665. [Crossref] [PubMed]
- Lans H, Hoeijmakers JHJ, Vermeulen W, et al. The DNA damage response to transcription stress. Nat Rev Mol Cell Biol 2019;20:766-84. [Crossref] [PubMed]
- Lu GS, Li M, Xu CX, et al. APE1 stimulates EGFR-TKI resistance by activating Akt signaling through a redox-dependent mechanism in lung adenocarcinoma. Cell Death Dis 2018;9:1111. [Crossref] [PubMed]
- Choi EB, Yang AY, Kim SC, et al. PARP1 enhances lung adenocarcinoma metastasis by novel mechanisms independent of DNA repair. Oncogene 2016;35:4569-79. [Crossref] [PubMed]
- Dong Y, Zhang D, Cai M, et al. SPOP regulates the DNA damage response and lung adenocarcinoma cell response to radiation. Am J Cancer Res 2019;9:1469-83. [PubMed]
- Cavnar PJ, Mogen K, Berthier E, et al. The actin regulatory protein HS1 interacts with Arp2/3 and mediates efficient neutrophil chemotaxis. J Biol Chem 2012;287:25466-77. [Crossref] [PubMed]
- Dehring DA, Clarke F, Ricart BG, et al. Hematopoietic lineage cell-specific protein 1 functions in concert with the Wiskott-Aldrich syndrome protein to promote podosome array organization and chemotaxis in dendritic cells. J Immunol 2011;186:4805-18. [Crossref] [PubMed]
- Director's Challenge Consortium for the Molecular Classification of Lung Adenocarcinoma. Gene expression-based survival prediction in lung adenocarcinoma: a multi-site, blinded validation study. Nat Med 2008;14:822-7. [Crossref] [PubMed]
- Okayama H, Kohno T, Ishii Y, et al. Identification of genes upregulated in ALK-positive and EGFR/KRAS/ALK-negative lung adenocarcinomas. Cancer Res 2012;72:100-11. [Crossref] [PubMed]
- Schabath MB, Welsh EA, Fulp WJ, et al. Differential association of STK11 and TP53 with KRAS mutation-associated gene expression, proliferation and immune surveillance in lung adenocarcinoma. Oncogene 2016;35:3209-16. [Crossref] [PubMed]
- Cui Y, Zhang S, Liang Y, et al. Consensus clustering of single-cell RNA-seq data by enhancing network affinity. Brief Bioinform 2021;22:bbab236. [Crossref] [PubMed]
- Wang Q, Qiao W, Zhang H, et al. Nomogram established on account of Lasso-Cox regression for predicting recurrence in patients with early-stage hepatocellular carcinoma. Front Immunol 2022;13:1019638. [Crossref] [PubMed]
- Yan C, Niu Y, Ma L, et al. System analysis based on the cuproptosis-related genes identifies LIPT1 as a novel therapy target for liver hepatocellular carcinoma. J Transl Med 2022;20:452. [Crossref] [PubMed]
- Bray F, Ferlay J, Soerjomataram I, et al. Global cancer statistics 2018: GLOBOCAN estimates of incidence and mortality worldwide for 36 cancers in 185 countries. CA Cancer J Clin 2018;68:394-424. [Crossref] [PubMed]
- Havel JJ, Chowell D, Chan TA. The evolving landscape of biomarkers for checkpoint inhibitor immunotherapy. Nat Rev Cancer 2019;19:133-50. [Crossref] [PubMed]
- Lin J, Shi J, Guo H, et al. Alterations in DNA Damage Repair Genes in Primary Liver Cancer. Clin Cancer Res 2019;25:4701-11. [Crossref] [PubMed]
- Gubin MM, Zhang X, Schuster H, et al. Checkpoint blockade cancer immunotherapy targets tumour-specific mutant antigens. Nature 2014;515:577-81. [Crossref] [PubMed]
- Chan TA, Yarchoan M, Jaffee E, et al. Development of tumor mutation burden as an immunotherapy biomarker: utility for the oncology clinic. Ann Oncol 2019;30:44-56. [Crossref] [PubMed]
- Latasiewicz J, Artz A, Jing D, et al. HS1 deficiency impairs neutrophil recruitment in vivo and activation of the small GTPases Rac1 and Rap1. J Leukoc Biol 2017;101:1133-42. [Crossref] [PubMed]
- Liu L, Liu L, Yao HH, et al. Stromal Myofibroblasts Are Associated with Poor Prognosis in Solid Cancers: A Meta-Analysis of Published Studies. PLoS One 2016;11:e0159947. [Crossref] [PubMed]
- Bai P. Biology of Poly(ADP-Ribose) Polymerases: The Factotums of Cell Maintenance. Mol Cell 2015;58:947-58. [Crossref] [PubMed]
- Césaire M, Thariat J, Candéias SM, et al. Combining PARP inhibition, radiation, and immunotherapy: A possible strategy to improve the treatment of cancer? Int J Mol Sci 2018;19:3793. [Crossref] [PubMed]