Development and validation of a nomogram for predicting the overall survival in non-small cell lung cancer patients with liver metastasis
Highlight box
Key findings
• A practical nomogram was developed and validated to predict the overall survival of non-small cell lung cancer (NSCLC) patients with liver metastasis (LM). Furthermore, the clinical value of the nomogram was confirmed by a risk stratification system.
What is known and what is new?
• In spite of a relatively low frequency, LM is considered the worst prognostic factor among all metastatic sites in NSCLC patients. A practical and convenient model to predict the survival of this unique group of patients is essential.
• This is the first study to build a prediction model focusing on the overall survival of NSCLC patients with LM.
What is the implication, and what should change now?
• The predictive model can be used to assist clinicians in applying personalized treatment.
• This user-friendly tool can allow physicians to identify the subgroup of patients requiring closer attention.
Introduction
As the first leading cause of tumor-related death worldwide, lung cancer is still a severe threat to global health (1). Of all lung cancer cases, more than 85% are non-small cell lung cancer (NSCLC) (2,3). Among them, up to 57% present with metastatic lesions at diagnosis (1). As distant metastasis contributes to a large portion of NSCLC-related death, the prognosis of metastatic NSCLC patients remains dismal (4). Although sites of distant metastases are equivalent in the American Joint Committee on Cancer (AJCC) stage system, previous studies revealed that the survival of patients varies with sites of metastases (5-7). Further, liver metastasis (LM) is the most lethal site compared with other metastatic lesions, with a median survival of only 3–5 months (5,6,8,9).
Despite the enormous progress of novel therapeutic modalities, including molecular targeted therapy and immunotherapy, the clinical outcome of NSCLC patients with LM has only marginally improved. The impact of LM on the therapeutic effect of first-line epidermal growth factor receptor (EGFR)-tyrosine kinase inhibitors (TKIs) in EGFR-mutant NSCLC was investigated in a multicenter study. It reported that patients with EGFR mutation and LM had significantly shorter progression-free survival (PFS) and overall survival (OS) than those without LM (10). Similarly, Meng and colleagues revealed that EGFR-TKIs failed to improve the clinical outcomes in patients with EGFR-mutant NSCLC and LM (11). In the setting of immunotherapy, preclinical studies revealed that LM diminished immunotherapy efficacy systemically by creating an ‘immune desert’ state (12,13). Similar results from multiple studies also indicated resistance to immunotherapy in NSCLC patients with LM (12,14-17). Therefore, it is necessary to predict the individualized survival of this unique group of patients.
The tumor node metastasis (TNM) staging system remains the most frequently used tool to predict patient prognosis. However, TNM classification only assesses the anatomic factors and fails to incorporate them as continuous variables (18). Previous studies showed that some demographic factors, including histology, age, and race, were significantly associated with prognosis in patients with metastatic NSCLC (19-21). Moreover, the factors like metastatic sites and treatment modalities have also been recognized as important prognostic factors of NSCLC patients with LM (9). Thus, developing a practical, convenient, and accurate model with the combination of these crucial predictors is essential. Nomograms, in recent decades, have served as a method to improve cancer prognostication (18). Based on statistical regression models, the nomograms provide more accessible results by integrating diverse determinant variables and graphically depicting them with intuitive graphs. Sporadic reports have created the nomograms for predicting the outcomes in metastatic NSCLC (22-25), whereas the nomogram specifically for the prediction of prognosis in patients with LM specifically has not been established.
As one of the largest and most comprehensive cancer registries in the United States (US), the Surveillance, Epidemiology, and End Results (SEER) database covers about 28% of the entire US population. It provides detailed information on a wide range of cancer cases, including patient demographics, tumor characteristics, treatment modalities, and vital status. It also provides long-term follow-up data, often spanning several decades, and continually updates its data. Further, SEER records follow standardized data collection and reporting protocols, ensuring uniformity and quality across all participating cancer registries. This consistency minimizes data discrepancies and errors, which are crucial for building reliable predictive models. Therefore, the SEER database is more accurate for predicting the OS compared to the results of prediction model studies with small sample sizes and single centers.
Herein we propose to construct an accurate and efficient nomogram for NSCLC with LM based on records extracted from the SEER database. With this tool, improved clinical decision-making and patient-clinician communication can be achieved. We present this article in accordance with the TRIPOD reporting checklist (available at https://tcr.amegroups.com/article/view/10.21037/tcr-23-899/rc).
Methods
Patient selection and data elements
Metastatic lung cancer cases diagnosed between 2010 and 2015 were selected from the SEER database using the SEER*Stat software (v8.3.5). Overall, 144,302 records were collected. The inclusion criteria were as follows: lung cancer was the first primary tumor; cases without other malignant cancers; NSCLC confirmed by histology according to the International Classification of Disease (ICD) code O-3; LM. Subtypes of histology included in the study were as follows: adenocarcinoma (ADC; ICD-O-3: 8140, 8141, 8144, 8250, 8251, 8255, 8260, 8310, 8323, 8480), squamous cell carcinoma (SCC; ICD-O-3: 8070–8075, 8083, 8084), and other NSCLC (ICD-O-3: 8012, 8013, 8030–8035). In contrast, cases ineligible for analysis were excluded: patients with an OS time of less than 1 month and patients with incomplete information [blanks, unknown, or not available (N/A)]. Ultimately, 2,367 patients were included for analysis. The detailed flow diagram of data collection is shown in Figure S1. The study was performed in accordance with the Declaration of Helsinki (as revised in 2013). As public and anonymous data were used, according to the ethics guidelines, neither informed consent nor approval of an ethics committee was required.
In total, fifteen variables, including clinical and pathological characteristics, were extracted and retrospectively reviewed in this study. Specifically, clinical factors consisted of sex, age, race, marital status, metastatic lesion, and treatment modalities (surgery, radiotherapy, chemotherapy). Pathological factors included primary site, histology, pathological grade, and TNM stage (based on the 7th edition of the American Joint Committee on Cancer staging). The optimal age-cut-off point was determined based on clinical consideration and prior reports. SEER patient’s follow-up is performed by hospital-based and many population-based registries, and generated each month. The time of OS was counted as the period between diagnosis and death from any cause or last follow-up.
Nomogram construction and evaluation
We randomly split all eligible patients into the training and validation cohort (7:3). Univariate and multivariate Cox regression analyses were applied in the training cohort to determine the parameters for generating the nomogram. The discriminative ability of the final nomogram was quantified by the concordance index (C-index). C-index varies from 0.5 to 1.0, where 0.5 represents random chance and 1.0 indicates a perfect fit. Typically, C-index value greater than 0.65 suggests a reasonable estimation (26-29). The consistency between predicted and actual 3-, 6-, and 12-month OS was displayed in the form of calibration curves. All validations for the model were performed using 1,000-resample bootstrapping. Besides, the decision curve analysis (DCA) was carried out to evaluate the clinical benefit of the nomogram by quantifying net benefits at different threshold probabilities (defined as the proportion of true positives minus the proportion of false positives, weighted by the relative harm of false-positive, and false-negative results) (30). The curves of treat-all-patients scheme (representing the highest clinical costs) and the treat-none scheme (representing no clinical benefit) were plotted as two references. Based on the sum scores of individuals on the nomogram, we further divided cases into high-score and low-score groups with the median score as the cut-off value. The Kaplan-Meier curves and log-rank tests were applied to compare the OS between different risk groups.
Statistical analysis
Variables with P<0.05 in the multivariate analysis were collected as the independent prognostic factors integrated into the nomogram, which was constructed by R software with survival and rms packages. Additionally, the Kaplan-Meier survival analysis and log-rank test were used to evaluate and compare the survival time. R software (version 4.1.0) and Statistical Package for the Social Sciences (SPSS) software (version 25.0.0, International Business Machines Corporation, IBM) were conducted for statistical analyses. All results with two-sided P<0.05 were deemed statistically significant.
Results
Patient demographics
In total, 2,367 records of NSCLC with LM diagnosed between 2010 and 2015 were enrolled in the final analysis. Specifically, 1,677 cases were included in the training set, while 690 cases were included in the validation set. The baseline demographic features of patients in the three cohorts are summarized in Table 1. The patients in this study were mainly male (59.3%), and most cases were married (83.1%) and White (77.3%). The median diagnostic age was 66 years (range, 18–94 years). Adenocarcinoma (ADC) accounted for the largest proportion (63.2%), followed by SCC (32.1%). Apart from LM, 54.2%, 26.7%, and 36.7% of patients also present with bone, brain, and lung metastases, respectively. Regarding treatments, the vast majority of patients did not receive primary tumor resection (96.6%). Meanwhile, 45.4% of patients underwent radiotherapy, and 64.1% had chemotherapy.
Table 1
Variables | Total cohort | Training cohort | Validation cohort | P value |
---|---|---|---|---|
Gender | 0.42 | |||
Male | 1,403 (59.3) | 997 (59.5) | 406 (58.8) | |
Female | 964 (40.7) | 680 (40.5) | 284 (41.2) | |
Age (years) | 0.28 | |||
<65 | 1,127 (47.6) | 785 (46.8) | 342 (49.6) | |
≥65 | 1,240 (52.4) | 892 (53.2) | 348 (50.4) | |
Race | 0.37 | |||
White | 1,829 (77.3) | 1,288 (76.8) | 541 (78.4) | |
Black | 326 (13.8) | 239 (14.3) | 87 (12.6) | |
Others | 212 (8.9) | 150 (8.9) | 62 (9.0) | |
Primary site | 0.14 | |||
Upper lobe | 1,228 (51.9) | 851 (50.7) | 377 (54.6) | |
Middle lobe | 117 (4.9) | 87 (5.2) | 30 (4.3) | |
Lower lobe | 660 (27.9) | 484 (28.9) | 176 (25.5) | |
Main bronchus | 115 (4.9) | 85 (5.1) | 30 (4.3) | |
Overlapping lesion | 247 (10.4) | 170 (10.1) | 77 (11.3) | |
Histology | 0.61 | |||
Adenocarcinoma | 1,497 (63.2) | 1,062 (63.3) | 435 (63.0) | |
SCC | 759 (32.1) | 531 (31.7) | 228 (33.0) | |
Others | 111 (4.7) | 84 (5.0) | 27 (4.0) | |
Grade | 0.27 | |||
Well differentiated (Grade I) | 102 (4.3) | 70 (4.2) | 32 (4.6) | |
Moderately differentiated (Grade II) | 638 (27.0) | 463 (27.6) | 175 (25.4) | |
Poorly differentiated (Grade III) | 1,570 (66.3) | 1,104 (65.8) | 466 (67.5) | |
Undifferentiated (Grade IV) | 57 (2.4) | 40 (2.4) | 17 (2.5) | |
T stage | 0.43 | |||
T1 | 173 (7.3) | 118 (7.0) | 55 (8.0) | |
T2 | 611 (25.8) | 450 (26.8) | 161 (23.3) | |
T3 | 682 (28.8) | 483 (28.8) | 199 (28.8) | |
T4 | 901 (38.1) | 626 (37.4) | 275 (39.9) | |
N stage | 0.73 | |||
N0 | 437 (18.5) | 319 (19.0) | 118 (17.1) | |
N1 | 197 (8.3) | 140 (8.4) | 57 (8.3) | |
N2 | 1,192 (50.3) | 837 (49.9) | 355 (51.4) | |
N3 | 541 (22.9) | 381 (22.7) | 160 (23.2) | |
Metastasis | 0.79 | |||
Bone | 1,284 (54.2) | 919 (54.8) | 365 (52.9) | |
Brain | 631 (26.7) | 440 (26.2) | 191 (27.7) | |
Lung | 868 (36.7) | 593 (35.4) | 275 (39.9) | |
Marital status | 0.66 | |||
Unmarried | 400 (16.9) | 296 (17.7) | 104 (15.1) | |
Married | 1,967 (83.1) | 1,381 (82.3) | 586 (84.9) | |
Surgery | 0.34 | |||
No | 2,287 (96.6) | 1,622 (96.7) | 665 (96.4) | |
Yes | 80 (3.4) | 55 (3.3) | 25 (3.6) | |
Radiotherapy | 0.39 | |||
No | 1,292 (54.6) | 917 (54.7) | 375 (54.3) | |
Yes | 1,075 (45.4) | 760 (45.3) | 315 (45.7) | |
Chemotherapy | 0.11 | |||
No | 850 (35.9) | 604 (36.0) | 246 (35.7) | |
Yes | 1,517 (64.1) | 1,073 (64.0) | 444 (64.3) |
Data are shown as n (%). SCC, squamous cell carcinoma.
Among the total cohort, the median follow-up was 4.0 months [interquartile range (IQR), 2–11 months] for all patients and 23 months (IQR, 16–35 months) for alive cases. The median OS was 5 months (IQR, 2–11 months). At the last follow-up, the death rate was 94.3%. Further, the 3-, 6-, and 12-month survival rates were 66.8%, 44.0%, and 22.6%, respectively.
Independent prognostic factors for OS
As shown in Table 2, eleven characteristics proved to be significantly associated with OS according to the univariate analysis (Figure S2). Subsequently, these factors were enrolled in further multivariate analysis. Nine variables (gender, age, race, grade, T stage, bone metastasis, brain metastasis, surgery, and chemotherapy) turned out to be the independent risk predictors for OS (all P<0.05).
Table 2
Variables | Median OS (months) (95% CI) | Univariate Cox regression | Multivariate Cox regression | |||
---|---|---|---|---|---|---|
HR (95% CI) | P value | HR (95% CI) | P value | |||
Gender | ||||||
Male | 4.0 (3.5–4.5) | Reference | Reference | |||
Female | 5.0 (4.3–5.7) | 0.849 (0.767–0.939) | 0.001* | 0.899 (0.809–0.998) | 0.046* | |
Age (years) | ||||||
<65 | 5.0 (4.4–5.6) | Reference | Reference | |||
≥65 | 4.0 (3.6–4.4) | 1.187 (1.075–1.311) | <0.001* | 1.134 (1.024–1.257) | 0.016* | |
Race | ||||||
White | 4.0 (3.6–4.4) | Reference | Reference | |||
Black | 4.0 (3.1–4.9) | 1.026 (0.891–1.181) | 0.725 | 0.982 (0.850–1.134) | 0.803 | |
Others | 7.0 (5.0–9.0) | 0.723 (0.604–0.866) | <0.001* | 0.803 (0.669–0.964) | 0.019* | |
Primary site | ||||||
Main bronchus | 4.0 (2.6–5.4) | Reference | ||||
Upper lobe | 5.0 (4.4–5.6) | 0.847 (0.675–1.063) | 0.151 | |||
Middle lobe | 6.0 (4.8–7.2) | 0.752 (0.553–1.023) | 0.069 | |||
Lower lobe | 5.0 (4.3–5.7) | 0.803 (0.635–1.016) | 0.068 | |||
Overlapping lesion | 3.0 (2.1–3.9) | 0.929 (0.712–1.212) | 0.586 | |||
Histology | ||||||
Adenocarcinoma | 5.0 (4.4–5.6) | Reference | Reference | |||
SCC | 4.0 (3.4–4.6) | 1.182 (1.061–1.316) | 0.002* | 1.112 (0.989–1.251) | 0.076 | |
Others | 3.0 (2.1–3.9) | 1.433 (1.144–1.797) | 0.002* | 1.251 (0.979–1.600) | 0.073 | |
Grade | ||||||
Well differentiated (Grade I) | 7.0 (4.3–9.7) | Reference | Reference | |||
Moderately differentiated (Grade II) | 6.0 (5.2–6.8) | 1.411 (1.072–1.858) | 0.014* | 1.213 (0.916–1.606) | 0.178 | |
Poorly differentiated (Grade III) | 4.0 (3.6–4.4) | 1.838 (1.408–2.399) | <0.001* | 1.565 (1.191–2.055) | 0.001* | |
Undifferentiated (Grade IV) | 2.0 (1.3–2.7) | 2.582 (1.711–3.859) | <0.001* | 1.889 (1.223–2.917) | 0.004* | |
T stage | ||||||
T1 | 7.0 (5.5–8.5) | Reference | Reference | |||
T2 | 5.0 (4.3–5.7) | 1.302 (1.055–1.606) | 0.014* | 1.172 (0.945–1.454) | 0.149 | |
T3 | 5.0 (4.3–5.7) | 1.270 (1.031–1.565) | 0.025* | 1.138 (0.919–1.408) | 0.235 | |
T4 | 4.0 (3.5–4.6) | 1.443 (1.176–1.769) | <0.001* | 1.356 (1.100–1.670) | 0.004* | |
N stage | ||||||
N0 | 5.0 (4.3–5.7) | Reference | ||||
N1 | 5.0 (3.4–6.6) | 0.978 (0.796–1.202) | 0.832 | |||
N2 | 5.0 (4.4–5.6) | 1.049 (0.918–1.199) | 0.481 | |||
N3 | 4.0 (3.2–4.9) | 1.126 (0.966–1.312) | 0.131 | |||
Bone metastasis | ||||||
No | 6.0 (5.3–6.7) | Reference | Reference | |||
Yes | 4.0 (3.6–4.4) | 1.326 (1.212–1.473) | <0.001 | 1.403 (1.263–1.558) | <0.001* | |
Brain metastasis | ||||||
No | 5.0 (4.6–5.4) | Reference | Reference | |||
Yes | 4.0 (3.3–4.7) | 1.147 (1.030–1.285) | 0.012* | 1.199 (1.055–1.364) | 0.005* | |
Lung metastasis | ||||||
No | 5.0 (4.5–5.5) | Reference | ||||
Yes | 4.0 (3.5–4.5) | 1.044 (0.941–1.157) | 0.418 | |||
Marital status | ||||||
Unmarried | 4.0 (3.1–4.9) | Reference | ||||
Married | 5.0 (4.6–5.4) | 0.983 (0.863–1.120) | 0.800 | |||
Surgery | ||||||
No | 4.0 (3.6–4.4) | Reference | Reference | |||
Yes | 11.0 (7.4–14.6) | 0.502 (0.370–0.670) | <0.001* | 0.602 (0.445–0.814) | 0.001* | |
Radiotherapy | ||||||
No | 5.0 (4.4–5.6) | Reference | Reference | |||
Yes | 4.0 (3.4–4.6) | 1.116 (1.017–1.235) | 0.022* | 1.049 (0.938–1.173) | 0.403 | |
Chemotherapy | ||||||
No | 2.0 (1.8–2.2) | Reference | Reference | |||
Yes | 7.0 (6.5–7.5) | 0.394 (0.352–0.433) | <0.001* | 0.414 (0.371–0.461) | <0.001* |
*, P<0.05. OS, overall survival; CI, confidence interval; SCC, squamous cell carcinoma.
Nomogram construction and validation
Based on the results above, a nomogram integrating all independent risk factors was constructed for the prediction of OS (Figure 1). By summing up the points of each variable on the point-scale axis, the total score of individual patients could be calculated, and patients’ 3-, 6-, and 12-month survival probabilities can be estimated. For example, a patient is 70 years old Asian female, grade II SCC, T4 stage with bone and brain metastasis, and she has received chemotherapy but not surgery. Her total points are 180, and the probabilities of 3-, 6-, and 12-month survival are 0.68, 0.5, and 0.28, respectively. As illustrated in the nomogram, chemotherapy contributed the most significant proportion to the OS, followed by grade and surgical resection. Bone metastasis and T stage presented a moderate impact on survival; on the contrary, gender and age showed a relatively small effect on survival.
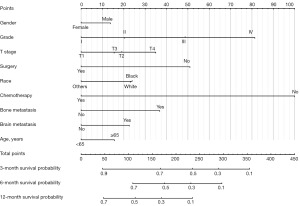
What is more, the nomogram exhibited good discriminative ability and accuracy at predicting OS. The C-index was 0.700 [95% confidence interval (CI): 0.684–0.716] in the training dataset and 0.677 (95% CI: 0.653–0.701) in the validation dataset. Meanwhile, the calibration curves confirmed an excellent consistency between the observed and predicted survival probabilities in both cohorts (Figure 2).
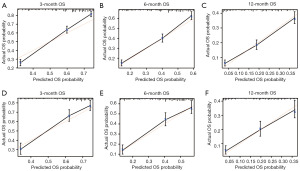
Clinical value of the nomogram
DCA evaluated the net benefit of patients in both cohorts, indicating a favorable clinical value of the proposed nomogram (Figure 3). Moreover, the total points of individuals were calculated based on the nomogram, and all patients were classified into two risk groups. The Kaplan-Meier survival analysis indicated that patients with lower scores achieved a significantly longer survival time than patients with higher scores (P<0.0001), providing solid evidence of the strong predictive ability of the model (Figure 4).
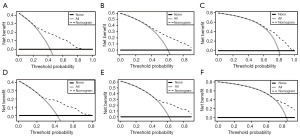
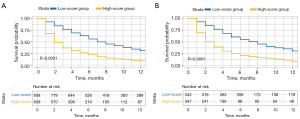
Discussion
In spite of a relatively low frequency (3–7%) (6,31,32), LM is considered the worst prognostic factor among all metastatic sites in NSCLC patients (8,33). The estimated OS of these patients is usually less than 6 months (5,6,15). Thus, predicting individual survival is critical to personalize treatment strategies and improve physician-patient communications. In this study, a nomogram was built to assess the OS probabilities of NSCLC patients with LM. Further analysis confirmed the model’s predictive ability and clinical value, which is highly significant for stratifying risk groups.
Our study revealed that sex, race, age, grade, and T stage were independent predictors of OS, consistent with prior studies (5,22,23). In addition to these classical risk factors, we found that in NSCLC patients with LM, the presence of bone or brain metastasis was significantly correlated with poor outcomes, whereas the impact of lung metastasis in the same group was negligible. Several reports have noted that the outcome among different metastatic sites varies (8,33). Yang et al. (34) pointed out that the prognosis in NSCLC patients with multiorgan metastases was substantially more unfavorable than those with single organ metastasis. All the evidence strongly supports metastatic heterogeneity in NSCLC. In terms of histologic types, our results found that the prognosis was better in ADC than in other subtypes of NSCLC, which was in line with earlier findings (22,23). Nevertheless, histology surprisingly did not remain significant statistically in the multivariate analysis. It has been reported that the survival benefit for ADC is only observed in recent decades, indicating the increasing impact of molecular targeted therapy in ADC patients (35). Unfortunately, recent studies demonstrated that LM was a negative prognostic factor in NSCLC patients receiving EGFR-TKIs therapy. Compared to NSCLC patients with an absence of LM, the treatment response was dramatically poorer in those with the presence of LM (10,11). Therefore, the discrepancy in our study could be attributed to the liver resistance to the molecular target therapy.
As shown in the nomogram, chemotherapy contributed the most to OS. In our study, the patients who received chemotherapy had a much better prognosis than those without chemotherapy (7 vs. 2 months, P<0.001). Similarly, prior studies suggested that chemotherapy made a large contribution to the survival outcomes for NSCLC patients with brain or bone metastasis (24,25,36-38). Moreover, given that LM was significantly related to dismal prognosis in patients who had targeted therapy and immunotherapy (10-12,14-16), chemotherapy still plays an essential role in the setting of LM. The significance of local treatment in patients with metastatic NSCLC remains controversial. The current study revealed a survival benefit in patients with surgically treated NSCLC with LM. Of patients who received resection of the primary tumor, 71% were treated with chemotherapy, and 33% were treated with radiotherapy, indicating that local resection was only a part of the multimodal therapy in most cases. David and colleagues also reported that the median OS time was significantly increased with surgical procedures in NSCLC patients with metastasis (39). Besides, they also stated that surgery might be considered part of multimodality therapy, given the crucial role of systemic therapies. Of note, not all patients can benefit from surgery. Various individual and tumor-related risk factors should be taken into consideration when surgical treatment is performed in a multidisciplinary setting (40-42). Interestingly, our study found that radiotherapy, as another option of local treatment strategies, was not able to independently predict the OS in NSCLC patients with LM (P=0.403). Our data indicated that radiotherapy alone might have a modest impact on prognosis. However, it should be noted that the prognosis of NSCLC patients with LM is unfortunately dismal, with a median OS of only 5 months. Due to a relatively long course of treatment, there might not be much of a chance to prove the value of radiotherapy in long-term prediction. Hence, Wallace and colleagues suggested that shorter courses of radiotherapy should be considered in patients with limited prognosis (43). Further, preclinical studies reported that radiotherapy-induced T-cell infiltration increased tumor antigen release, which led to an enhancement of antitumor immune response (44-46). As a result, Corrao and colleagues implied that the survival outcomes in NSCLC patients with LM might be improved when radiotherapy is combined with immunotherapy (47). Besides, for patients with severe pain or complications, radiotherapy may still be an option for palliative care.
Unlike bone or brain metastasis, limited data exist to look at LM of NSCLC. To date, this is the first study to establish a prediction model focusing on the OS of NSCLC patients with LM. As all factors in our nomogram can be readily obtained, it might be applicable in both developed and low or middle-income countries. This user-friendly tool will allow physicians to identify the subgroup of patients requiring closer attention. For example, EGFR-TKIs remain the standard first-line therapy for EGFR-mutated NSCLC patients with LM. Still, more extensive treatment like chemotherapy would be suggested for the high-risk subgroup with EFGR mutant recognized by our nomogram. Further, more palliative care and a shorter follow-up period might be considered for the high-risk subgroup. Similarly, hospice referral could be recommended for patients with limited life expectancy.
Nevertheless, limitations should be admitted in this study. First, there was an inevitable selection bias resulting from its retrospective nature. Second, the SEER database was unavailable for detailed treatment information, such as chemotherapy regimens, radiation doses, targeted therapy, and immunotherapy. Similarly, genomic data, other metastatic sites, including adrenal metastasis, and performance status cannot be obtained. Third, our nomogram was only validated internally with a relatively small cohort, and an independent external cohort with a larger sample size is still required for future application. Finally, given that all enrolled records were from the United States, the perspective of global data is warranted to apply our model to the Asian population and patients worldwide.
Conclusions
In summary, we developed and validated a practical nomogram to predict the OS of NSCLC patients with LM. The predictive model can be used to assist clinicians in deciding personalized treatment strategies.
Acknowledgments
Funding: This work was supported by the Scientific Research Launch Project for new employees of the Second Xiangya Hospital of Central South University, funded by
Footnote
Reporting Checklist: The authors have completed the TRIPOD reporting checklist. Available at https://tcr.amegroups.com/article/view/10.21037/tcr-23-899/rc
Peer Review File: Available at https://tcr.amegroups.com/article/view/10.21037/tcr-23-899/prf
Conflicts of Interest: All authors have completed the ICMJE uniform disclosure form (available at https://tcr.amegroups.com/article/view/10.21037/tcr-23-899/coif). The authors have no conflicts of interest to declare.
Ethical Statement: The authors are accountable for all aspects of the work in ensuring that questions related to the accuracy or integrity of any part of the work are appropriately investigated and resolved. The study was conducted in accordance with the Declaration of Helsinki (as revised in 2013).
Open Access Statement: This is an Open Access article distributed in accordance with the Creative Commons Attribution-NonCommercial-NoDerivs 4.0 International License (CC BY-NC-ND 4.0), which permits the non-commercial replication and distribution of the article with the strict proviso that no changes or edits are made and the original work is properly cited (including links to both the formal publication through the relevant DOI and the license). See: https://creativecommons.org/licenses/by-nc-nd/4.0/.
References
- Siegel RL, Miller KD, Wagle NS, et al. Cancer statistics, 2023. CA Cancer J Clin 2023;73:17-48. [Crossref] [PubMed]
- Thandra KC, Barsouk A, Saginala K, et al. Epidemiology of lung cancer. Contemp Oncol (Pozn) 2021;25:45-52. [Crossref] [PubMed]
- Padinharayil H, Varghese J, John MC, et al. Non-small cell lung carcinoma (NSCLC): Implications on molecular pathology and advances in early diagnostics and therapeutics. Genes Dis 2022;10:960-89. [Crossref] [PubMed]
- Leal T, Kotecha R, Ramlau R, et al. Tumor Treating Fields therapy with standard systemic therapy versus standard systemic therapy alone in metastatic non-small-cell lung cancer following progression on or after platinum-based therapy (LUNAR): a randomised, open-label, pivotal phase 3 study. Lancet Oncol 2023;24:1002-17. [Crossref] [PubMed]
- Ren Y, Dai C, Zheng H, et al. Prognostic effect of liver metastasis in lung cancer patients with distant metastasis. Oncotarget 2016;7:53245-53. [Crossref] [PubMed]
- Kagohashi K, Satoh H, Ishikawa H, et al. Liver metastasis at the time of initial diagnosis of lung cancer. Med Oncol 2003;20:25-8. [Crossref] [PubMed]
- Wei S, Wei W, Wu B, et al. The Incidence and Effect of Different Organ Metastasis on the Prognosis of NSCLC. Thorac Cardiovasc Surg 2023; Epub ahead of print. [Crossref] [PubMed]
- Riihimäki M, Hemminki A, Fallah M, et al. Metastatic sites and survival in lung cancer. Lung Cancer 2014;86:78-84. [Crossref] [PubMed]
- Choi MG, Choi CM, Lee DH, et al. Different prognostic implications of hepatic metastasis according to front-line treatment in non-small cell lung cancer: a real-world retrospective study. Transl Lung Cancer Res 2021;10:2551-61. [Crossref] [PubMed]
- Jiang T, Cheng R, Zhang G, et al. Characterization of Liver Metastasis and Its Effect on Targeted Therapy in EGFR-mutant NSCLC: A Multicenter Study. Clin Lung Cancer 2017;18:631-639.e2. [Crossref] [PubMed]
- Meng C, Ge X, Tian J, et al. Prognostic role of targeted therapy in patients with multiple-site metastases from non-small- cell lung cancer. Future Oncol 2020;16:1957-67. [Crossref] [PubMed]
- Yu J, Green MD, Li S, et al. Liver metastasis restrains immunotherapy efficacy via macrophage-mediated T cell elimination. Nat Med 2021;27:152-64. [Crossref] [PubMed]
- O'Leary K. Liver metastases cultivate an immune desert. Nat Rev Cancer 2021;21:143. [Crossref] [PubMed]
- Sridhar S, Paz-Ares L, Liu H, et al. Prognostic Significance of Liver Metastasis in Durvalumab-Treated Lung Cancer Patients. Clin Lung Cancer 2019;20:e601-8. [Crossref] [PubMed]
- Huang Y, Zhu L, Guo T, et al. Metastatic sites as predictors in advanced NSCLC treated with PD-1 inhibitors: a systematic review and meta-analysis. Hum Vaccin Immunother 2021;17:1278-87. [Crossref] [PubMed]
- Tumeh PC, Hellmann MD, Hamid O, et al. Liver Metastasis and Treatment Outcome with Anti-PD-1 Monoclonal Antibody in Patients with Melanoma and NSCLC. Cancer Immunol Res 2017;5:417-24. [Crossref] [PubMed]
- Xia H, Zhang W, Zhang Y, et al. Liver metastases and the efficacy of immune checkpoint inhibitors in advanced lung cancer: A systematic review and meta-analysis. Front Oncol 2022;12:978069. [Crossref] [PubMed]
- Balachandran VP, Gonen M, Smith JJ, et al. Nomograms in oncology: more than meets the eye. Lancet Oncol 2015;16:e173-80. [Crossref] [PubMed]
- Sperduto PW, Yang TJ, Beal K, et al. Estimating Survival in Patients With Lung Cancer and Brain Metastases: An Update of the Graded Prognostic Assessment for Lung Cancer Using Molecular Markers (Lung-molGPA). JAMA Oncol 2017;3:827-31. [Crossref] [PubMed]
- Reck M, Heigener DF, Mok T, et al. Management of non-small-cell lung cancer: recent developments. Lancet 2013;382:709-19. [Crossref] [PubMed]
- Wainer Z, Wright GM, Gough K, et al. Sex-Dependent Staging in Non-Small-Cell Lung Cancer; Analysis of the Effect of Sex Differences in the Eighth Edition of the Tumor, Node, Metastases Staging System. Clin Lung Cancer 2018;19:e933-44.
- Deng J, Ren Z, Wen J, et al. Construction of a nomogram predicting the overall survival of patients with distantly metastatic non-small-cell lung cancer. Cancer Manag Res 2018;10:6143-56. [Crossref] [PubMed]
- Wang Y, Pang Z, Chen X, et al. Survival nomogram for patients with initially diagnosed metastatic non-small-cell lung cancer: a SEER-based study. Future Oncol 2019;15:3395-409. [Crossref] [PubMed]
- Yang F, Gao L, Wang Q, et al. Development and validation of nomograms to predict early death in non-small cell lung cancer patients with brain metastasis: a retrospective study in the SEER database. Transl Cancer Res 2023;12:473-89. [Crossref] [PubMed]
- Shi S, Wang H, Liu X, et al. Prediction of overall survival of non-small cell lung cancer with bone metastasis: an analysis of the Surveillance, Epidemiology and End Results (SEER) database. Transl Cancer Res 2021;10:5191-203. [Crossref] [PubMed]
- Altman DG, Royston P. What do we mean by validating a prognostic model? Stat Med 2000;19:453-73. [Crossref] [PubMed]
- Collins GS, de Groot JA, Dutton S, et al. External validation of multivariable prediction models: a systematic review of methodological conduct and reporting. BMC Med Res Methodol 2014;14:40. [Crossref] [PubMed]
- Pencina MJ, D'Agostino RB Sr, D'Agostino RB Jr, et al. Evaluating the added predictive ability of a new marker: from area under the ROC curve to reclassification and beyond. Stat Med 2008;27:157-72; discussion 207-12. [Crossref] [PubMed]
- Reilly BM, Evans AT. Translating clinical research into clinical practice: impact of using prediction rules to make decisions. Ann Intern Med 2006;144:201-9. [Crossref] [PubMed]
- Vickers AJ, Elkin EB. Decision curve analysis: a novel method for evaluating prediction models. Med Decis Making 2006;26:565-74. [Crossref] [PubMed]
- Stenbygaard LE, Sørensen JB, Larsen H, et al. Metastatic pattern in non-resectable non-small cell lung cancer. Acta Oncol 1999;38:993-8. [Crossref] [PubMed]
- Oikawa A, Takahashi H, Ishikawa H, et al. Application of conditional probability analysis to distant metastases from lung cancer. Oncol Lett 2012;3:629-34. [Crossref] [PubMed]
- Li J, Zhu H, Sun L, et al. Prognostic value of site-specific metastases in lung cancer: A population based study. J Cancer 2019;10:3079-86. [Crossref] [PubMed]
- Yang J, Zhang Y, Sun X, et al. The prognostic value of multiorgan metastases in patients with non-small cell lung cancer and its variants: a SEER-based study. J Cancer Res Clin Oncol 2018;144:1835-42. [Crossref] [PubMed]
- Morgensztern D, Waqar S, Subramanian J, et al. Improving survival for stage IV non-small cell lung cancer: a surveillance, epidemiology, and end results survey from 1990 to 2005. J Thorac Oncol 2009;4:1524-9. [Crossref] [PubMed]
- Huang Z, Hu C, Tong Y, et al. Construction of a nomogram to predict the prognosis of non-small-cell lung cancer with brain metastases. Medicine (Baltimore) 2020;99:e21339. [Crossref] [PubMed]
- Dong Q, Deng J, Mok TN, et al. Construction and Validation of Two Novel Nomograms for Predicting the Overall Survival and Cancer-Specific Survival of NSCLC Patients with Bone Metastasis. Int J Gen Med 2021;14:9261-72. [Crossref] [PubMed]
- Zheng XQ, Huang JF, Lin JL, et al. Incidence, prognostic factors, and a nomogram of lung cancer with bone metastasis at initial diagnosis: a population-based study. Transl Lung Cancer Res 2019;8:367-79. [Crossref] [PubMed]
- David EA, Canter RJ, Chen Y, et al. Surgical Management of Advanced Non-Small Cell Lung Cancer Is Decreasing But Is Associated With Improved Survival. Ann Thorac Surg 2016;102:1101-9. [Crossref] [PubMed]
- Abdel-Rahman O. Outcomes of Surgery as Part of the Management of Metastatic Non-Small-Cell Lung Cancer: A Surveillance, Epidemiology and End Results Database Analysis. Cancer Invest 2018;36:238-45. [Crossref] [PubMed]
- Liang H, Liu Z, Huang J, et al. Identifying optimal candidates for primary tumor resection among metastatic non-small cell lung cancer patients: a population-based predictive model. Transl Lung Cancer Res 2021;10:279-91. [Crossref] [PubMed]
- Hanagiri T, Takenaka M, Oka S, et al. Results of a surgical resection for patients with stage IV non--small-cell lung cancer. Clin Lung Cancer 2012;13:220-4. [Crossref] [PubMed]
- Wallace AS, Fiveash JB, Williams CP, et al. Choosing Wisely at the End of Life: Use of Shorter Courses of Palliative Radiation Therapy for Bone Metastasis. Int J Radiat Oncol Biol Phys 2018;102:320-4. [Crossref] [PubMed]
- Obeid M, Panaretakis T, Joza N, et al. Calreticulin exposure is required for the immunogenicity of gamma-irradiation and UVC light-induced apoptosis. Cell Death Differ 2007;14:1848-50. [Crossref] [PubMed]
- Matsumura S, Wang B, Kawashima N, et al. Radiation-induced CXCL16 release by breast cancer cells attracts effector T cells. J Immunol 2008;181:3099-107. [Crossref] [PubMed]
- Burnette BC, Liang H, Lee Y, et al. The efficacy of radiotherapy relies upon induction of type i interferon-dependent innate and adaptive immunity. Cancer Res 2011;71:2488-96. [Crossref] [PubMed]
- Corrao G, Marvaso G, Ferrara R, et al. Stereotatic radiotherapy in metastatic non-small cell lung cancer: Combining immunotherapy and radiotherapy with a focus on liver metastases. Lung Cancer 2020;142:70-9. [Crossref] [PubMed]