Proteomic analysis of small extracellular vesicles unique to cervical cancer
Highlight box
Key findings
• ERI3, COX5A, and SGSM3 were uniquely expressed in small extracellular vesicles (sEVs) derived from patients with cervical cancer (CC).
What is known and what is new?
• At present, there is lack of specific biomarker for non-invasive diagnosis in CC. Cancer-derived sEVs released into the blood may offer a snapshot of the whole of the cancer.
• Our analysis provided the evidence for diagnostic method based on EVs technology.
What is the implication, and what should change now?
• Our results suggest that cancer-derived EVs carry molecular cargoes such as ERI3, COX5A and SGSM3, which may be related to the development of the CC.
• Further research is required to validate these findings to explore their functional role and potential as diagnostic biomarkers for CC.
Introduction
Cervical cancer (CC) is the fourth most common cause of cancer-related deaths in females worldwide and is mostly diagnosed at an advanced stage. Human papillomavirus (HPV), which is sexually transmitted, is a common risk factor for CC, and the development of CC may take months to years (1). Moreover, CC incidence and mortality rates show an increasing trend with late-stage diagnosis in low- and middle-income countries, such as India, South Africa, and Thailand (2). In Thailand, CC is a major public health concern affecting the quality of life among females. Furthermore, in Thailand, there is no organized screening plan for HPV vaccination. Existing diagnostic procedures, such as the Papanicolaou (Pap) test and HPV testing, have limitations in terms of convenience, risk, and cost (3). The sensitivities of the Pap and HPV tests are 55.4% and 94.6%, respectively, whereas the specificities are 96.8% and 94.1%, respectively (4). Other markers, such as the squamous cell carcinoma antigen, also showed low specificity. Hence, a novel biomarker for the diagnosis of CC is required.
The discovery of small extracellular vesicles (sEVs) in many body fluids presents a new avenue for identifying molecular alterations. Blood-based biomarkers can be analyzed easily and quickly and therefore have the potential to greatly improve diagnostic efficiency. Immune-associated proteins found in serum sEVs of children with pneumonia have been proposed as a potential source of biomarkers for diagnosing pediatric pneumonia (5). sEVs are small membrane vesicles released by healthy and malignant cells that contain and transfer bio-active molecules affecting the tumor microenvironment and eliciting long-distance effects. Consequently, they may serve as potential biomarkers for cancer diagnosis. sEVs also contain oncogenic proteins, lipids, and mRNA, which reflect origin cell functions and can be horizontally transferred to recipient cells to regulate their characterization (6-12). sEVs have been studied in many cancer types, and the cargo of sEVs has been found to contain more oncogenic proteins than those of larger vesicles (8,13). Tetraspanin 1, a candidate protein marker, is detected at higher levels in plasma extracellular vesicles (EVs) from patients with colon cancer compared to those from healthy controls (HC), demonstrating a sensitivity of more than 75% (14). Moreover, several functional EV proteins have been identified in most cancers, including prostate cancer. Integrin alpha V, which induces protein kinase B activation, was found in EVs derived from prostate cancer cells (15).
Proteomic analysis has become a promising tool for obtaining insights into the mechanisms of biological processes by characterizing the composition of biological processes, molecular functions, cellular components, and conducting pathway analysis. It provides extensive knowledge of proteins expressed differentially in a particular condition, exposing unique signaling changes. The analysis of differential expression provides useful information regarding the molecular behavior in a particular condition of cancer and aids in biomarker development. Cancer-derived EVs carry cancer-associated molecular cargo and modulate the behavior of recipient cells towards a pro-oncogenic type. Cancer-derived EVs circulating in blood play a role in cancer progression and development, reflecting the pathological state of cancer cells. One of the key mechanisms by which sEVs affect different cells in the tumor microenvironment is through both surface proteins and protein cargoes (16). The involvement of EV proteins in multiple cancer types has been extensively investigated (8,13-15,17,18), there is limited data available on EVs in clinical samples of CC. In this study, we utilized proteomic analysis to differentiate between the EV protein profiles of healthy and CC serum samples with the aim to identify candidate sEV biomarkers for the diagnosis of CC. The data obtained from this study will lay the groundwork for future development and validation of diagnostic biomarkers for CC. We present this article in accordance with the MDAR reporting checklist (available at https://tcr.amegroups.com/article/view/10.21037/tcr-23-517/rc).
Methods
Study design and blood collection
Serum samples from patients with CC (n=90, aged 30–65 years) were collected at Songklanagarind Hospital, Faculty of Medicine of Prince of Songkla University, Hat Yai, Songkhla, Thailand, from 2016 to 2017. The inclusion criteria for the study were newly diagnosed and untreated CC cases. To serve as HC, blood samples were also collected from healthy females (n=30, aged 30–60 years) with no HPV infection and no history of cancer. The study was conducted in accordance with the Declaration of Helsinki (as revised in 2013). The study was approved by the Human Research Ethics Committee of the Faculty of Medicine, Prince of Songkla University, Thailand (No. REC 60-401-04-2). Written informed consent was obtained from all participants. Whole blood (5 mL) was collected from each participant and allowed to clot at room temperature for 1 h. Within 2 h of collection, blood samples were centrifuged at 2,500 ×g for 10 min at 4 °C. All serum samples were divided into multiple aliquots and immediately stored at −80 °C until further analysis.
Nanoparticle tracking analysis (NTA)
The particles in the serum were measured using a NanoSight NS300 Analyzer equipped with a 488 nm laser (Malvern Panalytical, Malvern, UK). The particle concentration and size distribution were analyzed using NanoSight NTA software version 3.2, as described previously (19). For analysis, thawed serum samples were diluted in distilled water to a final volume of 1 mL. Two dilutions within the range of 1:10,000 to 1:5,000 were prepared for each sample, achieving a particle concentration of 107–109 particles/mL (i.e., 20–100 particles/frame). Data were recorded using a camera level set at 15, with a detection threshold of 6. Video capture was performed for five cycles (30 s/cycle). The absolute concentrations of the particles in each dilution were calculated based on a dilution factor of 1,000 or 2,000. Statistical analysis was performed using GraphPad Prism version 6.0 (GraphPad Software). The particle concentration (109 particles/mL) and size distribution were reported as the median and interquartile range. The Shapiro-Wilk normality test was used for the sample distribution test, and significant differences between the two groups were calculated using the Mann-Whitney U test. Statistical significance was defined as P≤0.05.
Isolation and purification of EVs
EVs pellets were isolated using a series of differential ultracentrifugation (dUC) steps as previously described (20). Briefly, 1 mL serum samples from each individual were pooled into three pools for HCs and nine pools for patients with CC (10 mL per pool). Pooled samples were subjected to dUC at 800, 2,000, and 12,000 ×g and filtered through 0.22 µm Minisart pore filters (Cat No. 2SRF2-16534K, Millipore, Burlington, MA, USA). The filtered supernatant was then centrifuged at 110,000 ×g using an optimal MAX-XP ultracentrifuge (Beckman Coulter, Brea, CA, USA) with an MLA-55 fixed-angle rotor. All centrifugation steps were performed at 4 °C. The resulting pellets were resuspended in a qEV size exclusion chromatography (SEC) column (Izon Science, Medford, MA, USA). Fractions were collected according to the manufacturer’s protocol. Briefly, the void volume (volume of the mobile phase required to elute a molecule having zero retention in the stationary phase in SEC) was 5 mL. Using 1× phosphate-buffered saline (PBS) as the elution buffer, 550 µL of the pellet solution was loaded on the column. Directly after this, 500 µL per fraction was collected for a total of 20 fractions. Each collected fraction was then centrifuged at 110,000 ×g for 1.5 h to concentrate the small vesicles. The pooled fractions were collected for further analyses.
Transmission electron microscopy (TEM)
Purified EVs, fixed with 2.5% glutaraldehyde, were deposited on a Formvar-coated carbon grid. After staining with 2% uranyl acetate, the grids were dried at room temperature and visualized using a JEM-2010 TEM.
Immunoblotting
EV proteins (20 µg/lane) were resolved using sodium dodecyl sulphate-polyacrylamide gel electrophoresis, transferred onto polyvinylidene fluoride membranes, probed with each primary antibody, and incubated with a horseradish peroxidase-conjugated secondary antibody. The following primary antibodies were used: CD63 (D4I1X, 1:500) and CD9 (D801A, 1:500) from Cell Signaling Technology (Danvers, MA, USA), CD81 (sc166028, 1:500) from Santa Cruz Biotechnology Inc. (Santa Cruz, CA, USA), and cytochrome c (1:1,000) from BioLegend (San Diego, CA, USA).
Liquid chromatography-tandem mass spectrometry (LC-MS/MS)
The protein concentration of EVs purified from serum and resuspended in PBS was determined using a bicinchoninic acid assay (Bio-Rad, Hercules, CA, USA). MS analysis was conducted by the Proteomics Research Laboratory, National Center for Genetic Engineering and Biotechnology, and the National Science and Technology Development Agency, Pathum Thani, Thailand. EV protein (10 µg total) from HC or CC was exchanged with 50 mM ammonium bicarbonate buffer and dithiothreitol was then added to a final concentration of 10 mM. Samples were incubated at 60 °C for 30 min, then cooled to room temperature. Iodoacetamide was added to a final concentration of 10 mM and samples were incubated in the dark for 30 min at room temperature. Tryptic digestion was subsequently performed at 37 °C overnight. Each digested sample was then injected onto a column and into a nebulizer. Tryptic peptides were protonated with 0.1% formic acid before being injected into a NanoAcquity system equipped with asymmetric C18 5 µm, 180 µm × 20-mm trap column, and BEH130 C18 1.7 µm, 100 µm × 100-mm analytical reverse phase column. (Glu1)-fibrinopeptide B was used to calibrate the mass spectrometer and was delivered through the reference sprayer of the NanoLockSpray source. Tryptic peptides were analyzed using a SYNAPT HDMS mass spectrometer (Waters Corp., Manchester, UK). Argon gas was used in the collision cell to obtain the MS/MS data. The MS/MS spectra obtained were processed using MaxEnt 3, a deconvolution software for peptides (Ensemble 1, Iterations 50, auto peak width determination) within MassLynx 4.0.
Proteomics data processing and analysis
MS intensities of individual LC-MS/MS analyses were differentially quantified using DeCyder MS Differential Analysis Software (GE Healthcare, Chicago, IL, USA). The PepMatch module was used to evaluate the average abundance ratio of each sample peptide, allowing for the automated detection of peptides and assignment of charge states. The resulting peptide mass and associated fragmentation spectra were searched against the non-redundant database of the National Center for Biotechnology Information (NCBInr) and identified using Mascot software (Matrix Science, London, UK). Database interrogation parameters were as follows: taxonomy, Homo sapiens; database, NCBInr; enzyme, trypsin; fixed modification, carbamidomethyl; variable modification, oxidation of methionine residues; mass values, monoisotopic; peptide mass tolerance, 1.2 Da; peptide charge state, 1+, 2+, and 3+. Duplicate protein entries were removed from the lists of MS data. The accession numbers were entered into the UniProt database (https://www.uniprot.org/). EV protein data obtained from the UniProt database were filtered to remove Uniparc and unmapped identifier proteins. Venn diagrams were generated using the Jvenn interactive Venn diagram viewer and the JavaScript library plug-in (https://jvenn.toulouse.inra.fr/app/index.html) to identify proteins present in both the HC and CC groups. Further, unique and co-expressed proteins were categorized into biological processes, cellular components, molecular functions, and contributing pathways using PANTHER (version 16.0; https://pantherdb.org/). Differentially expressed proteins (DEPs) were analyzed using R software. The number of upregulated and downregulated proteins between the HC and CC groups was determined based on the log2fold change (log2FC) criteria. The peptide ion intensities for all the groups were compared and statistical significance was defined at P<0.05.
The expression levels of the upregulated and downregulated DEPs were validated using online clinical samples across cervical tumors and normal samples using the University of Alabama at Birmingham Cancer (UALCAN) database analysis portal.
Results
Detection of EVs in human sera
To identify potential biomarkers for the diagnosis of CC, sEVs derived from the sera of HC and patients with CC were compared in terms of the number of particles and EV protein information obtained this study. The research workflow is illustrated in Figure 1. We analyzed the particle concentration and size distribution in serum using NTA. The concentration of particles in the CC group was marginally higher than that in the HC group (Figure 2A). Size distribution showed that the major EV population sizes, ranging from 101–150 nm, were found in both HC and CC, which is consistent with typical sEVs (Figure 2B). However, the mode size of the particles in the CC group was significantly smaller than that in the HC group (P=0.0018) (Figure 2C).
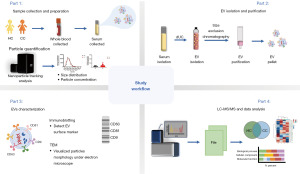
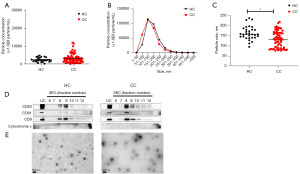
Isolation and purification of sEVs from human sera
We used dUC combined with SEC to isolate and purify EV pellets from the sera of HC and patients with CC. The typical EV marker proteins (CD9, CD63, and CD81) were detected using immunoblotting. Cytochrome c was used as a negative marker for EVs. The protein patterns showed that fractions 8–10 were highly enriched with EV markers in patients with CC compared with those in the HC group (Figure 2D, Figures S1,S2). Subsequently, we pooled EV pellets from fractions 8–10 for morphological characterization and further proteomic analysis. TEM revealed that the EV pellets contained a homogenous population of cup-shaped vesicles with a diameter of <2 µm (Figure 2E).
EV protein profiling
The EV protein expression profiles of both sample groups were identified using LC-MS/MS (table available at https://cdn.amegroups.cn/static/public/tcr-23-517-1.xlsx), and then compared and visualized using a two-way Venn diagram. We found probable relationships between protein expression and uniquely expressed proteins in sEVs derived from the sera of the HC and CC groups. The results identified five uniquely expressed EV proteins in the HC group and seventeen in the CC group (Figure 3A). A list of uniquely expressed proteins found in either the HC or CC group is presented in Table 1. The abundance of EV proteins in each group was used to generate a heat map using the MetaboAnalyst platform (https://www.metaboanalyst.ca/) (Figure 3B). The heatmap represents the protein expression profiles of the various pooled samples. Hierarchical clustering of the protein expression profiles showed significant differential expression.
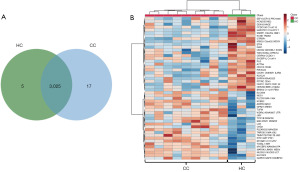
Table 1
Group | No. | Entry name | Protein name | Gene name |
---|---|---|---|---|
HC | 1 | KCNK4 | Potassium channel subfamily K member 4 | KCNK4, TRAAK |
2 | MYH16 | Putative uncharacterized protein MYH16 | MYH16, MYH5 | |
3 | HAPR1 | HUWE1-associated protein modifying stress responses | HAPSTR1, C16orf72, TAPR1 | |
4 | STAU1 | Double-stranded RNA-binding protein Staufen homolog 1 | STAU1, STAU | |
5 | PBIP1 | Pre-B-cell leukemia transcription factor-interacting protein 1 | PBXIP1, HPIP | |
CC | 1 | ZN185 | Zinc finger protein 185 | ZNF185 |
2 | CP51A | Lanosterol 14-alpha demethylase | CYP51A1, CYP51 | |
3 | IPO5 | Importin-5 | IPO5, KPNB3, RANBP5 | |
4 | UBP34 | Ubiquitin carboxyl-terminal hydrolase 34 | USP34, KIAA0570, KIAA0729 | |
5 | G3P | Glyceraldehyde-3-phosphate dehydrogenase | GAPDH, GAPD, CDABP0047 | |
6 | EFTU | Elongation factor Tu, mitochondrial (EF-Tu) (p43) | TUFM | |
7 | TSHB | Thyrotropin subunit beta | TSHB | |
8 | ADA32 | Disintegrin and metalloproteinase domain-containing protein 32 | ADAM32 | |
9 | ERI3 | ERI1 exoribonuclease 3 | ERI3 | |
10 | COX5A | Cytochrome c oxidase subunit 5A, mitochondrial | COX5A | |
11 | ATRX | Transcriptional regulator ATRX | ATRX, RAD54L, XH2 | |
12 | RUN3A | RUN domain-containing protein 3A | RUNDC3A, RAP2IP, RPIP8 | |
13 | VWA3B | von Willebrand factor A domain-containing protein 3B | VWA3B | |
14 | CHD2 | Chromodomain-helicase-DNA-binding protein 2 | CHD2 | |
15 | SGSM3 | Small G protein signaling modulator 3 | SGSM3, MAP, RABGAPLP, RUTBC3 | |
16 | PHF10 | PHD finger protein 10 | PHF10, BAF45A | |
17 | ETS1 | Protein C-ets-1 | ETS1, EWSR2 |
HC, healthy controls; CC, cervical cancer.
Proteomic and pathway analysis of unique EV proteins in the CC group
The EV protein expression profiles of both groups were determined using LC-MS/MS, and subsequently compared and visualized using a Venn diagram (Figure 4). The uniquely expressed proteins in the CC group were subsequently subjected to functional enrichment analysis using PANTHER, based on their biological processes, molecular functions, cellular components, and pathways they are related to. Because the program allows a limited number of protein inputs, only proteins found to be uniquely expressed in the CC sEVs, not in the HC sEVs, were analyzed. The proteins enriched in CC sEVs were involved in cellular processes, metabolic processes, and biological regulation (Figure 4A). In terms of molecular function, proteins involved in binding, catalytic activity, transcription, translation and ATP-dependent activity were identified (Figure 4B). Uniquely expressed proteins related to cellular components included cellular anatomical entities and protein-containing complexes (Figure 4C). Interestingly, the unique EV proteins found in the CC group were involved in pathways such as the platelet-derived growth factor (PDGF), thyrotropin-releasing hormone receptor, angiogenesis, Fas cell surface death receptor (FAS), vascular endothelial growth factor (VEGF), and Ras signaling pathways (Figure 4D).
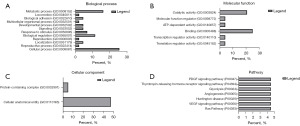
DEP and functional enrichment analysis
Using the R software, proteins expressed in both groups (Venn diagram overlap) were subjected to differential expression analysis comparing the CC group and the HC group (Figure 5, table available at https://cdn.amegroups.cn/static/public/tcr-23-517-2.xlsx). The histogram represents proteins that were differentially expressed in the CC group compared to the HC group. DEPs were identified based on the criteria of |log2FC| >2 and include both upregulated and downregulated proteins. These proteins were further subjected to functional enrichment analysis using PANTHER, based on their associated biological processes, molecular functions, cellular components, and pathways (Figure 6A-6E). We found that the majority of up- and downregulated proteins identified in CC sEVs showed similar functions with regards to their involvement in biological processes, cellular components, and molecular functions. They were associated with the following PANTHER-defined categories of biological processes: “cellular process”, “metabolic process”, and “biological regulation” (Figure 6A). Molecular function analysis showed that both groups of DEPs were mainly associated with “binding activity” and “catalytic activity” (Figure 6B). Furthermore, DEPs exhibited similar groupings in cellular components: “protein-containing complex” and “cellular anatomical entity” (Figure 6C). Pathway analysis revealed differences between up- and downregulated proteins (Figure 6D,6E). Upregulated proteins were associated with pathways such as the p53 pathway. Downregulated CC sEVs proteins were also associated with the p53 pathway, but also demonstrated association with pathways that were not associated with the upregulated proteins. These included the transforming growth factor (TGF), fibroblast growth factor (FGF), epidermal growth factor (EGF), VEGF, Wnt signaling, chemokine and cytokine signaling, angiogenesis, and apoptosis pathways.
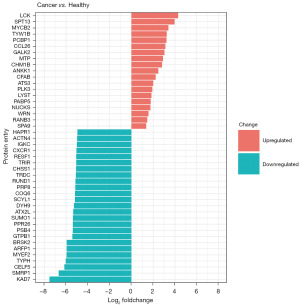
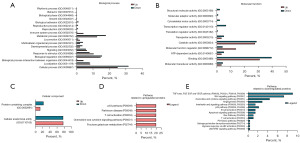
Validation of unique and upregulated proteins using The Cancer Genome Atlas (TCGA) datasets in UALCAN database
We used the UALCAN data analysis portal (https://ualcan.path.uab.edu/index.html) to explore the expression of CC-derived proteins (seventeen unique and nineteen upregulated proteins) in primary tumors compared to that in normal tissues. While 10 of the seventeen proteins unique to CC sEVs (IPO5, TSHB, ERI3, COX5A, ATRX, VWA3B, CHD2, SGSM3, PHF10, and ETS1) were found in the TCGA database, only three (ERI, COX5A, and SGSM3) were significantly increased in primary CC tissue compared to normal tissue (P<0.05) (Figure 7A). Among the DEPs identified in CC through LC-MS/MS analysis, a total of nineteen were found to be significantly upregulated. To further investigate the clinical relevance of these proteins, a search was conducted in the TCGA database. Nine proteins (LCK, TYW1B, PCBP1, CCL26, GALK2, ANKK1, PLK3, LYST, and WRN) were identified. Only four of these (LCK, PCBP1, GALK2, and ANKK1) displayed significant increases in primary CC tissue compared to normal tissue (Figure 7B).
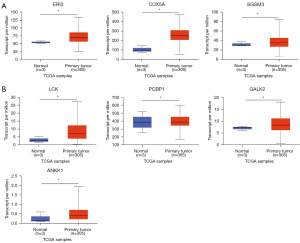
Discussion
In this study, we performed a quantitative comparison of the total particles circulating in the sera derived from HCs and patients with CC. We determined the particle concentration and size distribution of the total particles in serum using NTA. The levels of EV particles in human body fluids, particularly those in blood, may themselves be used as a diagnostic marker for cancer development and allow differentiation between patients with and without cancer. Our experiment determined the total number of serum particles in both the HC and CC groups. The results showed that sera from both HC and patients with CC had similar particle sizes in the range of 101–150 nm. However, the mode sizes of the particles in the HC group were significantly larger than those in the CC group. Furthermore, the particle concentrations of the CC group were marginally higher than those in the HC group. Consistent with recent clinical evidence, plasma particle levels were significantly higher in patients with cancer than in HCs (21-24). According to a proteomic study on EVs derived from patients with breast cancer, a single cancer cell can release more than 104 particles per day (25,26).
Further, we investigated the EV protein profiles derived from the sera of HCs and patients with CC. We isolated and purified EV particles using ultracentrifugation combined with SEC. We determined the surface markers of EV and visualized the morphology and size of EV particles using an electron microscope. As expected, the combination of ultracentrifugation and SEC successfully isolated sEVs. From the immunoblotting results, the abundance of EV markers, including CD63, CD9, and CD81, indicates the successful isolation of EVs from serum samples. Nevertheless, we used cytochrome c as a negative control for EV characterization following the MISEV2018 guidelines (27). Moreover, TEM images revealed a typical morphology and size range of sEVs. Interestingly, we successfully purified the EVs from abundant serum proteins, as shown in the TEM image, wherein slightly precipitated abundant proteins were detected.
Proteomics profiling using LC-MS/MS has become a widely utilized approach in various clinical settings to discover potential screening and diagnostic biomarkers. This method offers the advantage of mass screening for protein markers and the ability to identify signaling pathways potentially associated with diseases (17,18). In contrast, the analysis of protein isoforms resulting from somatic mutations through immunohistochemistry (IHC) is limited by the unavailability of antibodies to epitopes specific to particular isoforms. Therefore, MS-based technology provides a valuable alternative for the discovery of protein isoforms resulting from somatic mutations (28). In this study, proteomic profiling of sEVs derived from patients with CC and HC demonstrated that 3,025 proteins were expressed in both groups, while five and seventeen proteins were uniquely expressed in the HC and CC groups, respectively. The metabolic pathways with which the total proteins in each group were associated with, were similar. We further investigated the pathways of the 17 proteins found to be uniquely expressed in the CC group: ZN185, CP51A, IPO5, UBP34, G3P, EFTU, TSHB, ADA32, ERI3, COX5A, ATRX, RUN3A, VWA3B, CHD2, SGSM3, PHF10, and ETS1.
COX5A is a subunit of cytochrome c oxidase, an enzyme complex located in the mitochondria. Cytochrome c oxidase plays a major role in metabolic processes (29), including ATP production (30). Consequently, COX5A expression is associated with many cancers, including colorectal, breast, prostate, and lung cancer (31-34). Its expression has been associated with the proliferation of cancer cells and angiogenesis (35-37). Similarly, IPO5, also expressed in colorectal cancer cells, plays a role in promoting the proliferation and tumorigenesis of cancer cells (38). Furthermore, the IPO5 test with logistic regression analysis revealed that COX5A is the best individual potential biomarker for differentiating cancer cases from HCs (37). Interestingly, the correlated pathway analysis revealed that EV proteins that were uniquely expressed in the CC group were associated with angiogenesis, glycolysis, and the VEGF signaling pathway. Angiogenesis is the formation of new vessels that supply nutrients to cancer cells, and VEGF plays a pivotal role in the initial stages of angiogenesis (39). The proteins found to be uniquely expressed in the CC group were also associated with the Ras signaling pathway. Ras proteins are crucial mediators of several malignant characteristics in transformed cancer cells and function as activators in many cancers (40). Targeting the Ras pathway to inhibit tumor growth has shown promise as a therapeutic approach to inhibit tumor growth (40). However, further validation is necessary to confirm the clinical relevance of these unique EV proteins in CC. Nevertheless, these findings suggest that the unique expression of proteins in CC-derived EVs may serve as developmental signals in patients with CC.
In addition, 3,025 proteins were co-expressed in both the CC and HC groups, with both upregulated and downregulated protein expression seen in CC. DEPs were analyzed using a multilevel enriched Gene Ontology (GO) analysis. Notably, nineteen upregulated proteins were primarily associated with the following biological processes: “cellular process”, “metabolic process”, and “biological regulation”. Cellular processes are required to maintain homeostasis. EVs play an essential role in many pathways related to both physiological and pathological homeostasis by facilitating cellular communication, including the excretion of nucleic acids from cells (41). Both up- and downregulated proteins in CC were found to be involved in “binding” and “catalytic activity” within cellular processes, and cellular component analysis of these proteins also revealed similarities. Proteomic profiling of EVs derived from the blood samples of females with breast cancer revealed that 80% of EV proteins were involved in protein binding. This is consistent with our findings as well as those of studies on other cancers, including ovarian and colorectal cancer (42-45). Additionally, pathway analysis revealed distinct differences between the upregulated and downregulated DEPs in the CC and HC groups. The nineteen proteins upregulated in the CC group were associated with the following major pathways: p53, T cell activation, and chemokine and cytokine signaling. Chemokines are a group of soluble factors that play crucial roles in immune regulation during inflammatory response processes and in defense against foreign pathogens. Chemokines also regulate many biological processes such as angiogenesis and stem cell migration during embryo development. The chemokine signaling pathway is regulated in diverse cellular processes, such as angiogenesis, epithelial cell proliferation, and survival (46,47). Intriguingly, chemokines are critical for cancer development and exhibit high functionality in the tumor microenvironment (48). Together, these results suggest that chemokine signaling can be utilized to target and deliver chemokines in cancer treatment.
Pathway analysis of downregulated proteins in CC revealed their involvement in the Wnt signaling pathway, the chemokine and cytokine signaling pathway, angiogenesis, the p53 pathway, apoptosis, the Ras pathway, hypoxia response via the hypoxia-inducible factor (HIF) pathway, and the JAK/STAT pathway. The Wnt/β-catenin signaling pathway is highly conserved and plays a role in a variety of physiological processes, including proliferation, differentiation, apoptosis, invasion, migration, and homeostasis. Dysregulation of Wnt signaling leads to carcinogenesis (41,48,49). The detailed GO analysis revealed that EV proteins that were downregulated in patients with CC are associated with the Wnt signaling pathway. The current study highlights the crucial role of EVs in transporting and delivering signaling molecules involved in important processes, such as cancer development. EVs have been shown to transport and release Wnt signaling proteins in both homeostasis and cancer conditions (42). A recent study found that Wnt protein is located on the surface of EVs and activates Wnt signaling on the surface of target cells during disease progression (43). Moreover, p53, known as the “guardian protein of genome”, is a transcription factor involved in maintaining genome integrity. In response to various stressors, most notably genotoxic damage and hypoxia, p53 promotes permanent and/or transient growth arrest by repairing DNA or by activating cell death programs. Many malignancies, such as breast, colon, prostate, and bladder cancers, can result from p53 mutation and dysregulation (44,45,50). To validate the findings of EV protein analysis, the UALCAN database was utilized. Out of the 19 proteins upregulated in CC EVs, nine (LCK, ANKK1, CCL26, GALK2, LYST, PCBP1, PLK3, TYW1B, and WRN) were found in CC tissue based on TCGA data. Among these, LCK, PCBP1, GALK2, and ANKK1 showed significantly increased expression levels in CC tissue compared to normal tissue. This further supports their potential role as clinically relevant biomarkers in CC.
In addition, we found more than hundred finding proteins showed significant downregulated in CC. We found 17 out of 50 proteins, namely CELF5, MYEF2, SUMO1, SCY1, COQ6, CXCR1, ACTN4, STAU1, LTV1, HDAC4, WDR19, KCNK4, KANK2, BAZ2A, GAK, KCTD3, and FZD2 proteins. Further investigation using the TCGA data showed that three proteins, SCYL1, HDAC4, and WDR19, demonstrated significantly decreased expression levels in primary CC tissue compared to normal tissue. Previous studies on hepatocellular carcinoma and breast cancer have reported that SCYL1 is associated with the development of breast cancer and affects cell cycle arrest in hepatocellular carcinoma (51,52). The cell cycle is regulated by various biological mechanisms, and regulatory proteins that play a role in this process are crucial for controlled cell cycle expression. However, alterations in the balance of regulatory proteins can contribute to the development of several diseases, including cancer. SCYL1 has been shown to affect the cell cycle by enhancing the steady state of several proteins, including Cyclin F and RRM2, which constitute the regulatory circuit (52). In breast cancer, SCYL1 has demonstrated potential as a prognostic marker, as knockout of SCYL1 significantly reduced the progression and migration of breast cancer cells (51). Furthermore, HDAC4 is believed to target the p21 protein and participate in its repression. One study suggested that HDAC4 could serve as a promising target for novel anticancer therapies that inhibit specific HDACs, as silencing HDAC4 arrested cancer cell growth in the glioblastoma model (53,54). Moreover, the HeLa CC model showed that HDAC4 binds to the NAC1 protein, which is associated with CC progression (55).
Based on our investigations, the results obtained from EV proteins derived from serum samples, as well as the reported data from CC tissues, showed both consistent and discrepant results. It is important to note that some proteins from the cells of origin can potentially migrate to other distant cells through EV vehicles, and as a result, these trafficked proteins can be detected as cargo within EVs (56,57). Nevertheless, EVs hold significant potential as valuable markers for the screening and diagnosis of CC patients in the future. Our study specifically identified ERI3, COX5A, and SGSM3 as uniquely expressed proteins in sEVs derived from patients with CC. These proteins could be further developed as additional biomarkers and validated using cell-blocks of cytology specimens (58).
A challenge we faced in our study was the heterogeneity of clinical samples in terms of CC staging. We were unable to design a sample set in which age, CC stage, and number of CC samples were perfectly matched for each stage. Therefore, a limitation of our study was the relatively small sample used for the validation step. Despite these limitations, our findings provide valuable initial data that serve as a foundation for further studies aiming to develop combination panel markers instead of relying on a single biomarker for clinical application. It is essential to conduct future research with larger sample sizes to validate our findings and confirm the potential of these markers for improving CC diagnosis and management.
Conclusions
In this study, we conducted a comprehensive proteomic analysis of sEVs derived from the serum of patients with CC and healthy volunteers. The aim was to identify potential candidate proteins that could serve as biomarkers for CC and provide insights into the disease’s underlying mechanisms. Our findings revealed that the protein profiles of sEVs derived from cancer patients, compared to those from healthy serum, included both unique proteins specific to CC and proteins shared between the two groups. While these findings are promising, further validation studies are necessary to confirm the diagnostic potential of these identified proteins and translate them into clinical practice. The development of CC markers as a non-invasive technique can help to reduce the diagnostic window and enable early detection of CC, leading to improved clinical outcomes for patients.
Acknowledgments
Funding: This work was supported by the Faculty of Medicine, Prince of Songkla University (No. REC60-401-04-2).
Footnote
Reporting Checklist: The authors have completed the MDAR reporting checklist. Available at https://tcr.amegroups.com/article/view/10.21037/tcr-23-517/rc
Data Sharing Statement: Available at https://tcr.amegroups.com/article/view/10.21037/tcr-23-517/dss
Peer Review File: Available at https://tcr.amegroups.com/article/view/10.21037/tcr-23-517/prf
Conflicts of Interest: All authors have completed the ICMJE uniform disclosure form (available at https://tcr.amegroups.com/article/view/10.21037/tcr-23-517/coif). The authors have no conflicts of interest to declare.
Ethical Statement: The authors are accountable for all aspects of the work in ensuring that questions related to the accuracy or integrity of any part of the work are appropriately investigated and resolved. The study was conducted in accordance with the Declaration of Helsinki (as revised in 2013). The study was approved by the Human Research Ethics Committee of the Faculty of Medicine, Prince of Songkla University, Thailand (No. REC 60-401-04-2). Written informed consent was obtained from all participants.
Open Access Statement: This is an Open Access article distributed in accordance with the Creative Commons Attribution-NonCommercial-NoDerivs 4.0 International License (CC BY-NC-ND 4.0), which permits the non-commercial replication and distribution of the article with the strict proviso that no changes or edits are made and the original work is properly cited (including links to both the formal publication through the relevant DOI and the license). See: https://creativecommons.org/licenses/by-nc-nd/4.0/.
References
- World Health Organization. Cervical cancer. 2020. Available online: https://www.who.int/health-topics/cervical-cancer#tab=tab_1
- Ploysawang P, Rojanamatin J, Prapakorn S, et al. National Cervical Cancer Screening in Thailand. Asian Pac J Cancer Prev 2021;22:25-30. [Crossref] [PubMed]
- Balasubramanian A, Kulasingam SL, Baer A, et al. Accuracy and cost-effectiveness of cervical cancer screening by high-risk human papillomavirus DNA testing of self-collected vaginal samples. J Low Genit Tract Dis 2010;14:185-95. [Crossref] [PubMed]
- Seton-Rogers S. Taking an alternative route. Nat Rev Cancer 2007;7:893. [Crossref]
- Cheng J, Ji D, Yin Y, et al. Proteomic profiling of serum small extracellular vesicles reveals immune signatures of children with pneumonia. Transl Pediatr 2022;11:891-908. [Crossref] [PubMed]
- Sato Y, Matoba R, Kato K. Recent Advances in Liquid Biopsy in Precision Oncology Research. Biol Pharm Bull 2019;42:337-42. [Crossref] [PubMed]
- Zheng X, Li X, Wang X. Extracellular vesicle-based liquid biopsy holds great promise for the management of ovarian cancer. Biochim Biophys Acta Rev Cancer 2020;1874:188395. [Crossref] [PubMed]
- Azmi AS, Bao B, Sarkar FH. Exosomes in cancer development, metastasis, and drug resistance: a comprehensive review. Cancer Metastasis Rev 2013;32:623-42. [Crossref] [PubMed]
- Valadi H, Ekström K, Bossios A, et al. Exosome-mediated transfer of mRNAs and microRNAs is a novel mechanism of genetic exchange between cells. Nat Cell Biol 2007;9:654-9. [Crossref] [PubMed]
- Record M, Subra C, Silvente-Poirot S, et al. Exosomes as intercellular signalosomes and pharmacological effectors. Biochem Pharmacol 2011;81:1171-82. [Crossref] [PubMed]
- Putz U, Howitt J, Doan A, et al. The tumor suppressor PTEN is exported in exosomes and has phosphatase activity in recipient cells. Sci Signal 2012;5:ra70. [Crossref] [PubMed]
- Théry C, Zitvogel L, Amigorena S. Exosomes: composition, biogenesis and function. Nat Rev Immunol 2002;2:569-79. [Crossref] [PubMed]
- Keerthikumar S, Gangoda L, Liem M, et al. Proteogenomic analysis reveals exosomes are more oncogenic than ectosomes. Oncotarget 2015;6:15375-96. [Crossref] [PubMed]
- Lee CH, Im EJ, Moon PG, et al. Discovery of a diagnostic biomarker for colon cancer through proteomic profiling of small extracellular vesicles. BMC Cancer 2018;18:1058. [Crossref] [PubMed]
- Ciardiello C, Leone A, Lanuti P, et al. Large oncosomes overexpressing integrin alpha-V promote prostate cancer adhesion and invasion via AKT activation. J Exp Clin Cancer Res 2019;38:317. [Crossref] [PubMed]
- Monnamorn L, Seree-Aphinan C, Molika P, et al. The Concentration of Large Extracellular Vesicles Differentiates Early Septic Shock From Infection. Front Med (Lausanne) 2021;8:724371. [Crossref] [PubMed]
- Soares Martins T, Marçalo R, da Cruz E. Novel Exosome Biomarker Candidates for Alzheimer's Disease Unravelled Through Mass Spectrometry Analysis. Mol Neurobiol 2022;59:2838-54. [Crossref] [PubMed]
- Zhao L, Shi J, Chang L, et al. Serum-Derived Exosomal Proteins as Potential Candidate Biomarkers for Hepatocellular Carcinoma. ACS Omega 2021;6:827-35. [Crossref] [PubMed]
- Navakanitworakul R, Hung WT, Gunewardena S, et al. Characterization and Small RNA Content of Extracellular Vesicles in Follicular Fluid of Developing Bovine Antral Follicles. Sci Rep 2016;6:25486. [Crossref] [PubMed]
- Ding XQ, Wang ZY, Xia D, et al. Proteomic Profiling of Serum Exosomes From Patients With Metastatic Gastric Cancer. Front Oncol 2020;10:1113. [Crossref] [PubMed]
- Rosell R, Wei J, Taron M. Circulating MicroRNA Signatures of Tumor-Derived Exosomes for Early Diagnosis of Non-Small-Cell Lung Cancer. Clin Lung Cancer 2009;10:8-9. [Crossref] [PubMed]
- Taylor DD, Gercel-Taylor C. MicroRNA signatures of tumor-derived exosomes as diagnostic biomarkers of ovarian cancer. Gynecol Oncol 2008;110:13-21. [Crossref] [PubMed]
- Shen M, Di K, He H, et al. Progress in exosome associated tumor markers and their detection methods. Mol Biomed 2020;1:3. [Crossref] [PubMed]
- Riches A, Campbell E, Borger E, et al. Regulation of exosome release from mammary epithelial and breast cancer cells - a new regulatory pathway. Eur J Cancer 2014;50:1025-34. [Crossref] [PubMed]
- Lee H, Castro CM. Thermophoretically enriched detection. Nat Biomed Eng 2019;3:163-4. [Crossref] [PubMed]
- Tian F, Zhang S, Liu C, et al. Protein analysis of extracellular vesicles to monitor and predict therapeutic response in metastatic breast cancer. Nat Commun 2021;12:2536. [Crossref] [PubMed]
- Huang Y, Li SN, Zhou XY, et al. The Dual Role of AQP4 in Cytotoxic and Vasogenic Edema Following Spinal Cord Contusion and Its Possible Association With Energy Metabolism via COX5A. Front Neurosci 2019;13:584. [Crossref] [PubMed]
- Shidham VB. Cell-blocks and other ancillary studies (including molecular genetic tests and proteomics). Cytojournal 2021;18:4. [Crossref] [PubMed]
- Pecina P, Houstková H, Hansíková H, et al. Genetic defects of cytochrome c oxidase assembly. Physiol Res 2004;53:S213-23. [Crossref] [PubMed]
- Chen WL, Kuo KT, Chou TY, et al. The role of cytochrome c oxidase subunit Va in non-small cell lung carcinoma cells: association with migration, invasion and prediction of distant metastasis. BMC Cancer 2012;12:273. [Crossref] [PubMed]
- Ali HEA, Lung PY, Sholl AB, et al. Dysregulated gene expression predicts tumor aggressiveness in African-American prostate cancer patients. Sci Rep 2018;8:16335. [Crossref] [PubMed]
- Zeng J, Li G, Xia Y, et al. miR-204/COX5A axis contributes to invasion and chemotherapy resistance in estrogen receptor-positive breast cancers. Cancer Lett 2020;492:185-96. [Crossref] [PubMed]
- Zhang K, Chen Y, Huang X, et al. Expression and clinical significance of cytochrome c oxidase subunit IV in colorectal cancer patients. Arch Med Sci 2016;12:68-77. [Crossref] [PubMed]
- Mimeault M, Batra SK. Hypoxia-inducing factors as master regulators of stemness properties and altered metabolism of cancer- and metastasis-initiating cells. J Cell Mol Med 2013;17:30-54. [Crossref] [PubMed]
- Jariwala U, Prescott J, Jia L, et al. Identification of novel androgen receptor target genes in prostate cancer. Mol Cancer 2007;6:39. [Crossref] [PubMed]
- Takayama K, Tsutsumi S, Suzuki T, et al. Amyloid precursor protein is a primary androgen target gene that promotes prostate cancer growth. Cancer Res 2009;69:137-42. [Crossref] [PubMed]
- Zhang W, Lu Y, Li X, et al. IPO5 promotes the proliferation and tumourigenicity of colorectal cancer cells by mediating RASAL2 nuclear transportation. J Exp Clin Cancer Res 2019;38:296. [Crossref] [PubMed]
- Li XF, Aierken AL, Shen L. IPO5 promotes malignant progression of esophageal cancer through activating MMP7. Eur Rev Med Pharmacol Sci 2020;24:4246-54. [PubMed]
- Beck M, Schirmacher P, Singer S. Alterations of the nuclear transport system in hepatocellular carcinoma - New basis for therapeutic strategies. J Hepatol 2017;67:1051-61. [Crossref] [PubMed]
- van der Watt PJ, Okpara MO, Wishart A, et al. Nuclear transport proteins are secreted by cancer cells and identified as potential novel cancer biomarkers. Int J Cancer 2022;150:347-61. [Crossref] [PubMed]
- Li M, Ransohoff RM. The roles of chemokine CXCL12 in embryonic and brain tumor angiogenesis. Semin Cancer Biol 2009;19:111-5. [Crossref] [PubMed]
- Hembruff SL, Cheng N. Chemokine signaling in cancer: Implications on the tumor microenvironment and therapeutic targeting. Cancer Ther 2009;7:254-67. [PubMed]
- Grant K, Loizidou M, Taylor I. Endothelin-1: a multifunctional molecule in cancer. Br J Cancer 2003;88:163-6. [Crossref] [PubMed]
- Rosanò L, Spinella F, Bagnato A. Endothelin 1 in cancer: biological implications and therapeutic opportunities. Nat Rev Cancer 2013;13:637-51. [Crossref] [PubMed]
- Shankar A, Loizidou M, Aliev G, et al. Raised endothelin 1 levels in patients with colorectal liver metastases. Br J Surg 1998;85:502-6. [Crossref] [PubMed]
- Režen T, Ogris I, Sever M, et al. Evaluation of Selected CYP51A1 Polymorphisms in View of Interactions with Substrate and Redox Partner. Front Pharmacol 2017;8:417. [Crossref] [PubMed]
- Dhillon AS, Hagan S, Rath O, et al. MAP kinase signalling pathways in cancer. Oncogene 2007;26:3279-90. [Crossref] [PubMed]
- Loizzi V, Del Vecchio V, Gargano G, et al. Biological Pathways Involved in Tumor Angiogenesis and Bevacizumab Based Anti-Angiogenic Therapy with Special References to Ovarian Cancer. Int J Mol Sci 2017;18:1967. [Crossref] [PubMed]
- Salamonsen LA, Hannan NJ, Dimitriadis E. Cytokines and chemokines during human embryo implantation: roles in implantation and early placentation. Semin Reprod Med 2007;25:437-44. [Crossref] [PubMed]
- Moriyama A, Masumoto A, Nanri H, et al. High plasma concentrations of nitrite/nitrate in patients with hepatocellular carcinoma. Am J Gastroenterol 1997;92:1520-3. [PubMed]
- Martin-Orozco E, Sanchez-Fernandez A, Ortiz-Parra I, et al. WNT Signaling in Tumors: The Way to Evade Drugs and Immunity. Front Immunol 2019;10:2854. [Crossref] [PubMed]
- Zhang L, Wrana JL. The emerging role of exosomes in Wnt secretion and transport. Curr Opin Genet Dev 2014;27:14-9. [Crossref] [PubMed]
- Gross JC, Chaudhary V, Bartscherer K, et al. Active Wnt proteins are secreted on exosomes. Nat Cell Biol 2012;14:1036-45. [Crossref] [PubMed]
- Riihilä P, Nissinen L, Farshchian M, et al. Complement Component C3 and Complement Factor B Promote Growth of Cutaneous Squamous Cell Carcinoma. Am J Pathol 2017;187:1186-97. [Crossref] [PubMed]
- Corrales L, Ajona D, Rafail S, et al. Anaphylatoxin C5a creates a favorable microenvironment for lung cancer progression. J Immunol 2012;189:4674-83. [Crossref] [PubMed]
- Youn A, Simon R. Identifying cancer driver genes in tumor genome sequencing studies. Bioinformatics 2011;27:175-81. [Crossref] [PubMed]
- Roach TG, Lång HKM, Xiong W, et al. Protein Trafficking or Cell Signaling: A Dilemma for the Adaptor Protein TOM1. Front Cell Dev Biol 2021;9:643769. [Crossref] [PubMed]
- Cibas ES. CellBlockistry 101: The textbook of cell-blocking science. Cytojournal 2021;18:24. [Crossref]