The stage-dependent prognostic role of ARID1A in hepatocellular carcinoma
Highlight box
Key findings
• Genomic alterations in the ARID family, especially alterations in ARID1A, are biomarkers for the poor prognosis of hepatocellular carcinoma (HCC) and contribute to the failure of sorafenib treatment. ARID1A expression has a stage-dependent effect on the prognosis of HCC.
What is known and what is new?
• As a tumor suppressor in HCC, and loss of or decreased ARID1A expression has been related to poor prognosis.
• The results of this study showed that ARID1A expression has a stage-dependent role on the prognosis of HCC. It serves as a risk factor for stage I–II patients but a protective factor for stage III–IV patients.
What is the implication, and what should change now?
• ARID1A has dual roles in HCC in a tumor stage-dependent manner. The novel nomogram could be used for the prognostic evaluation of HCC patients.
Introduction
Background
Liver cancer, which is mainly believed to be induced by hepatitis B virus (HBV) and/or hepatitis C virus (HCV) infection and long-term alcohol exposure, is one of the most common causes of cancer-related death worldwide and leads to a serious problem for public health (1). Especially in China, due to the highly infectious nature of HBV, liver cancer ranks fifth in morbidity and fourth in mortality among all cancers. Hepatocellular carcinoma (HCC) is the most common pathological type of primary liver cancer (2), and it demonstrates insensitivity to a variety of therapeutics, leading to poor prognosis of patients (3). Alpha-fetoprotein (AFP) is the most commonly used biomarker for HCC surveillance, but is limited by its insensitivity. Serum VEGF, and ANG2 baseline levels were also shown to be prognostic factors (4). Recent literature reveals that circulating nucleic acid biomarkers, which include cell-free DNA could monitor therapy and predict drug response in HCC (5). With the evolution of precision medicine, molecular targeted therapy and immunotherapy have become an increasingly valuable research direction on HCC (6,7). Therefore, the optimal biomarker for predicting the efficacy is crucial (8). Zhu et al. found overexpression MYBL1 induced angiogenesis and caused sorafenib resistance to HCC cells, and may represent a novel prognostic biomarker (9). High baseline c-KIT and low baseline HGF were confirmed to presage treatment efficacy of sorafenib (4). In a published study (10), novel biomarkers related to the tumor immune microenvironment, such as the CD4+/CD25+/Foxp3+ regulatory T lymphocytes, play important roles in the prognosis and the development of emerging immunotherapy of HCC. Given the significant heterogeneity of HCC, biomarkers for the prognosis of HCC and other therapeutics for the patients, such as sorafenib, still need to be further explored and studied.
The ARID family is a group of essential molecules that form switch/sucrose nonfermenting (SWI/SNF) chromatin remodeling complexes, which participate in a variety of biological processes, including DNA replication, gene expression, and cell differentiation (11,12). In particular, ARID1A, a subunit of ARID1, plays a critical role in inhibiting the invasion, migration, and metastasis of lung cancer cells, as discovered in a previous study (13). In addition, ARID1A deficiency could also serve as a predictor of the outcome of the treatment of cancer with immune checkpoint inhibitors (ICIs) (14).
Recently, multiple studies have focused on the role of ARID1A in HCC. Most of them have confirmed the role of ARID1A as a tumor suppressor in HCC, and loss of or decreased ARID1A expression has been related to poor prognosis (15,16). Nevertheless, other studies reported that ARID1A plays different roles at different times during the development of HCC (17) and serves as a dual-purpose subunit of SWI/SNF complexes (18). This result suggested that ARID1A is required in the early stage of tumor initiation but is then lost in the advanced stage of HCC, which finally leads to metastasis. However, the existent studies for the dual role of ARID1A in tumor initiation or tumor suppression in HCC were based on animal models and lack of verification clinically. In this study, we conducted bioinformatic analysis and assessed a cohort of HCC patients from China to test the roles of the ARID family, especially the dual role of ARID1A, in different stages of the disease and the relationships between the expression or genomic alteration of the family and the prognosis of HCC patients. Furthermore, we established a novel prognostic model to predict the overall survival (OS) of HCC patients. We present this article in accordance with the TRIPOD reporting checklist (available at https://tcr.amegroups.com/article/view/10.21037/tcr-23-645/rc).
Methods
Patient samples, tissue microarrays (TMAs), and immunohistochemical (IHC) analysis
A cohort of 206 HCC patients seen at Qidong Liver Cancer Institute (Qidong, China) between March 2001 and May 2009 was involved in this study. The 206 HCC patients included 166 males and 40 females (mean age: 51.10 years, ranging from 21 to 83 years). No patient received preoperative chemotherapy or radiotherapy. All patients were in good performance status [Eastern Cooperative Oncology Group (ECOG): 0–1; Child-Pugh score: A/B]. In total, 83.01% of patients (n=171) had cirrhosis, while 79.13% of patients (n=163) and 5.34% of patients (n=11) had an infection of HBV and HCV, respectively. The patients involved received blood tests for tumor markers including AFP and 29.09% of patients (n=48) were detected with a serum AFP level over 400 µg/L. All tumors were staged according to the 8th American Joint Committee on Cancer (AJCC) tumor-node-metastasis (TNM) staging system for liver cancer. The study was conducted in accordance with the Declaration of Helsinki (as revised in 2013). The study was approved by the Ethics Committee of Qidong Liver Cancer Institute (No. QDRMYY-003) and Shanghai Jiao Tong University School of Medicine (No. RA-2019-250). Informed consents were taken from all patients.
TMAs for the tissue of the primary tumor and paired adjacent normal tissue of the 206 involved patients were then sent for IHC analysis. Antigen retrieval was performed by boiling the slides in 10 mM citrate buffer (pH 6.0) for 10 minutes, followed by cooling at room temperature for 20 minutes. TMAs were incubated at 4 ℃ with primary antibodies at appropriate concentrations (ARID1A: 1:500; Abcam: ab182560, Cambridge, UK) overnight. Two investigators independently evaluated the IHC slides. Three fields of each slide were selected for the evaluation of IHC scores. Given that ARID1A is localized in the nucleus, the proportion of cells with nuclear staining and the strength of staining was evaluated. We followed the method for scoring reported in a previous study (13). The intensity of staining was scored as 0 (no staining), 1 (weak), 2 (medium), and 3 (strong). Percentage scores were assigned as 0 (<5%), 1 (5–25%), 2 (26–50%), 3 (51–75%), and 4 (76–100%). Images were scanned using a NanoZoomer slide scanner (NanoZoomer-XR C12000, Hamamatsu Photonics, Hamamatsu, Japan) and viewed using NDP.view software (NDP.view2 U12388-01, Hamamatsu). The final score of each slide was calculated as the average score of the three fields selected randomly and ranged from 0 to 12 (intensity score × percentage score). Specifically, low expression of ARID1A was defined as a final score of less than 4 (IHC score <4) for primary tumor tissue and adjacent normal tissue (13). IHC score ≥4 was defined as high ARID1A expression. The expression differences between tumor and normal tissue were also calculated, and differences larger than 4 [(IHC-tumor − IHC-normal) ≥4] were defined as significant differences. As a positive control for ARID1A IHC analyses, we used human kidney tissue, while as a negative control, normal human lung tissue was used (13).
Statistical analysis
In this study, we used Kaplan-Meier methods to perform the survival analysis and the log-rank P value for the detection of significance. The Cox regression model was used for the multivariate analysis. All statistical analyses were performed using GraphPad Prism 8.0 software (GraphPad, La Jolla, CA, USA) or SPSS software version 23, and Student’s t-tests were used to determine statistical significance. P values were determined by two-tailed tests, and P<0.05 was used to define statistical significance.
Nomogram construction and verification
We used the variables selected by the Cox regression model in the process of nomogram construction. The “rms” package (https://github.com/harrelfe/rms) of R software version 3.1.2 (The R Foundation for Statistical Computing, Vienna, Austria) was used to construct the nomogram. The discriminating capacity of the nomogram was tested using Harrell’s C-indexes, which range from 0.5 (no discrimination) to 1 (perfect discrimination) (19), and visual calibration plots were utilized to assess the nomogram’s capacity for calibration (20). Bootstrapping with 1,000 resamples was used for these analyses. The area under the receiver operating characteristic (ROC) curve (AUC) was also used to assess the efficacy of the nomogram in predicting the prognosis of involved HCC patients. Internal verification was performed based on our cohort of patients. Each patient was scored through the nomogram and then divided into a high- or low-risk group based on the cutoff determined by the Youden index.
Bioinformatic analysis
To expand our results to a large sample size of patients, we used a series of databases to verify our results and to explore factors related to patient survival. First, data from the cBioPortal for Cancer Genomics (21,22) were employed for the detection of ARID family genomic alterations among HCC patients and their relationship with the prognosis of HCC patients received sorafenib treatment. Kaplan-Meier plotter (23) was used to perform survival analysis. A total of 338 patient samples from The Cancer Genome Atlas (TCGA) and its related database, the Human Protein Atlas, were used for the verification of the study. Metascape (24) was used for the enrichment analysis based on the co-mutated genes of ARID family genomic alterations.
Results
The prevalence of ARID family genomic alterations and their effects on the prognosis of HCC patients
In this study, we explored the incidences of genomic alterations of ARID family members through cBioPortal and found that ARID1A alterations were the most common genomic alterations in the ARID family, present in 8% of all 1,085 HCC patients, followed by alterations in ARID2 (5%), ARID1B (2.2%), ARID4A (1.1%), ARID5B (1%), ARID3A (0.6%), ARID4B (0.6%), ARID3C (0.3%), ARID3B (0.1%), and ARID5A (0.1%), as shown in Figure 1A. Overall, 178 patients (16%) among the 1,085 HCC patients harbored at least one genomic alteration of an ARID family member, and we performed survival analysis according to the genomic alterations of HCC patients, as displayed in Figure 1B,1C. Genomic alterations in the ARID family served as biomarkers for poor prognosis of HCC patients in terms of both disease-free survival (DFS; P=0.0325, 20.24 vs. 35.84 months) and OS (P=0.0076, 60.84 vs. 90.64 months). Subgroup analysis of the common alterations of the ARID family was then performed as shown in Figure 1D. Genomic alterations of ARID1A (DFS: P=0.0417; OS: P<0.0001), ARID1B (DFS: P=0.0018; OS: P=0.0254), and ARID2 (DFS: P=0.0416; OS: P=0.0471) served as biomarkers for poor prognosis of HCC patients.
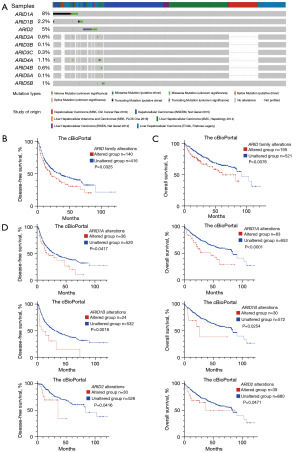
ARID family genomic alterations serve as biomarkers for sorafenib treatment
A series of genes were found to be co-mutated with ARID family members, including CRTAC1, TCEAL6, TSHR, and CSN3. Multiple signaling pathways were found to be related to these altered genes through enrichment analysis, as shown in Figure 2A, especially the PI3K/Akt signaling pathway, the alteration of which has been reported to be mechanisms underlying resistance to sorafenib treatment (25,26). Therefore, we further performed survival analysis for patients who received sorafenib treatment to explore the effect of ARID family members on sorafenib treatment response among HCC patients.
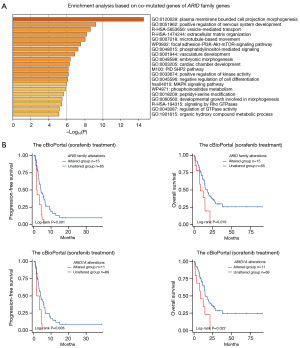
In total, 80 HCC patients who received sorafenib treatment (n=80) from the cBioPortal were involved in the survival analysis. Information on the patients’ genomic alterations and survival time is shown in Table S1. As shown in the survival analysis in Figure 2B, genomic alterations of the ARID family predicted poor prognosis of HCC patients who underwent sorafenib treatment in terms of both progression-free survival (PFS, P=0.001, 1.91 vs. 4.18 months) and OS (P=0.016, 8.4 vs. 17.6 months). Specifically, the subgroup analysis revealed that a single ARID1A alteration also displayed satisfactory efficacy in predicting the prognosis of patients who received sorafenib treatment (PFS: P=0.008, 2.54 vs. 4.18 months; OS: P=0.022, 8.4 vs. 15.2 months).
The stage-dependent role of ARID1A expression in HCC patients
Given that ARID1A alteration had the highest incidence among HCC patients and had satisfactory efficacy in predicting the prognosis of HCC, we further explored the effect of ARID1A expression on the prognosis of HCC patients. Generally, ARID1A usually serves as a tumor suppressor, and the alteration of ARID1A usually leads to a resultant expression loss (11). Therefore, the loss of ARID1A expression could be a biomarker for the poor prognosis of HCC patients, similar to the alteration of ARID1A. However, data from Kaplan-Meier plotter (PFS: P=0.054; OS: P=0.023) and the Human Protein Atlas (OS: P=0.004) revealed that high expression of ARID1A was related to poor prognosis, as shown in Figure 3A-3C. The survival analysis results for ARID1A alteration seemed to be contradictory to the results for ARID1A expression. Further exploration showed that early-stage (TNM stage I–II) HCC patients had significantly longer OS than late-stage (stage III–IV) patients (P<0.0001), as shown in Figure 3C. We then performed subgroup analysis in different stages of HCC patients to clarify the effect of ARID1A expression. As shown in Figure 3D, the ARID1A messenger RNA (mRNA) expression level data derived from Kaplan-Meier plotter haply demonstrated a stage-dependent role of ARID1A expression: high expression of ARID1A seemed to be a risk factor for stage I–II HCC patients but a protective factor for stage III–IV HCC patients. However, the sample size from the database was too small to draw any conclusions.
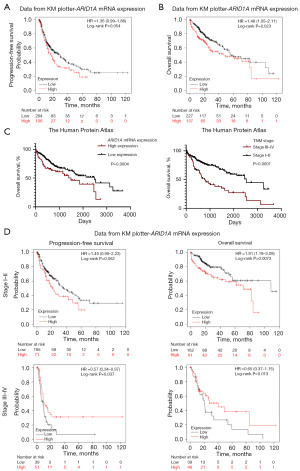
To clarify the stage-dependent role of ARID1A expression in HCC patients, 206 HCC patients (n=206), including 62 stage I patients (n=62), 103 stage II patients (n=103), 35 stage III patients (n=35) and 6 stage IV patients (n=6), were enrolled in another analysis. The basic information and clinical characteristics of these patients are summarized in Table 1.
Table 1
Variables | Stage I–II (n=165) | Stage III–IV (n=41) | |||||
---|---|---|---|---|---|---|---|
Patients’ information | Log-rank P value | Cox regression | Patients’ information | Log-rank P value | Cox regression† | ||
Age (years) | 51.8 (21.0–83.0) | 0.5385 | 0.181 | 48.2 (25.0–75.0) | 0.5547 | 0.079 | |
Sex | 0.0456* | 0.248 | 0.7932 | 0.019* | |||
Male | 130 (78.79) | 36 (87.80) | |||||
Female | 35 (21.21) | 5 (12.20) | |||||
Serum AFP level | 0.8375 | 0.03* | 0.1694 | 0.253 | |||
≥400 μg/L | 48 (29.09) | 21 (51.22) | |||||
<400 μg/L | 117 (70.91) | 20 (48.78) | |||||
HBV infection | 0.3152 | 0.427 | 0.937 | 0.276 | |||
HBsAg (+) | 131 (79.39) | 32 (78.05) | |||||
HBsAg (−) | 34 (20.61) | 9 (21.95) | |||||
HCV infection | 0.8884 | 0.916 | 0.0632 | 0.978 | |||
Anti-HCV (+) | 9 (5.45) | 2 (4.88) | |||||
Anti-HCV (−) | 156 (94.55) | 39 (95.12) | |||||
Tumor size | 0.4790 | 0.813 | 0.4426 | 0.346 | |||
≤5 cm | 93 (56.36) | 25 (60.98) | |||||
>5 cm | 72 (43.64) | 16 (39.02) | |||||
Intra-hepatic metastasis | 0.2677 | 0.146 | 0.4841 | 0.045* | |||
Positive | 51 (30.91) | 12 (29.27) | |||||
Negative | 114 (69.09) | 29 (70.73) | |||||
Histology | 0.1970 | 0.922 | 0.0261* | 0.004* | |||
Grade 1–2 | 84 (50.91) | 21 (51.22) | |||||
Grade 3–4 | 81 (49.09) | 20 (48.78) | |||||
Venous invasion | NA | NA | 0.2039 | 0.207 | |||
Positive | 0 (0.00) | 32 (78.05) | |||||
Negative | 165 (100.00) | 9 (21.95) | |||||
ARID1A expression of tumor tissue | <0.0001* | 0.031* | 0.018* | 0.691 | |||
Low expression (IHC <4) | 82 (49.70) | 27 (65.85) | |||||
High expression (IHC ≥4) | 83 (50.30) | 14 (34.15) | |||||
ARID1A expression of adjacent tissue | 0.8928 | 0.373 | 0.9391 | 0.959 | |||
Low expression (IHC <4) | 138 (83.64) | 29 (70.73) | |||||
High expression (IHC ≥4) | 23 (13.94) | 10 (24.39) | |||||
NA | 4 (2.42) | 2 (4.88) | |||||
Expression differences | <0.0001* | 0.253 | 0.0513 | 0.014* | |||
ΔIHC ≥4 | 46 (27.88) | 8 (19.51) | |||||
ΔIHC <4 | 115 (69.70) | 31 (75.61) | |||||
NA | 4 (2.42) | 2 (4.88) |
†, only patients with stage III (n=35) disease among stage III–IV patients were involved in the Cox regression model in this study; *, P<0.05. ΔIHC = tumor – normal. IHC, immunohistochemistry; AFP, alpha-fetoprotein; HBV, hepatitis B virus; HBsAg, hepatitis B surface antigen; HCV, hepatitis C virus; NA, not available.
Figure 4A displays representative images of ARID1A IHC staining in tumor tissue [scored as 0 (negative), 3 (weak staining), and 9 (strong staining)], adjacent normal tissue [scored as 0 (negative) and 6 (moderate staining)] and nonmalignant background of liver tissue as a control. Representative images of two HCC patients with different stages are shown in Figure 4B, which displays two paired samples of patients with poor prognosis. In the paired samples of the stage I–II patient, the tumor tissue had higher ARID1A expression than adjacent normal tissue, while the paired samples of the stage III–IV patient showed the opposite pattern. To ensure the accuracy of the results, we verified the results through HCC samples in the TCGA database, as shown in Figure 5. Subsequently, survival analysis was performed. We first performed survival analysis for the patients according to different stages. As shown in Figure 6A, ARID1A had the same effect on the prognosis of these groups of stage I and stage II patients, and highly expressed ARID1A was related to poor prognosis (stage I: P<0.0001; stage II: P=0.0048). As a result, stage I and stage II patients were grouped for analysis. Stage III and stage IV patients were also grouped due to the small sample size of stage IV patients. The analysis demonstrated a significant stage-dependent role of ARID1A expression in HCC patients, as shown in Figure 6B. In terms of the OS time of involved HCC patients, high ARID1A expression served as a risk factor in stage I–II patients [P<0.0001, 689 days vs. not reached (NR)] but a protective factor in stage III–IV patients (P=0.0180, 2,213.5 vs. 492 days), which verified the bioinformatic analysis results shown in Figure 3D.
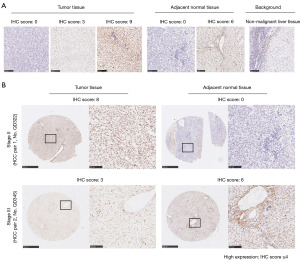
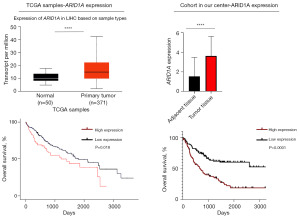
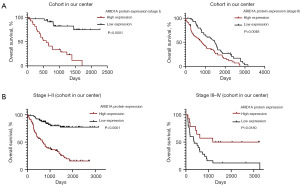
The regulatory status of ARID1A expression in tumor tissue compared with adjacent normal tissue is associated with the prognosis of HCC patients in a stage-dependent pattern
In this study, we discovered the stage-dependent role of ARID1A expression in tumor tissue. Furthermore, we compared the expression level of ARID1A in tumor tissue with that in adjacent normal tissue to clarify the regulatory status of ARID1A and further explore the stage-dependent role of ARID1A in HCC without interference from background expression. As shown in Figure 7A, ARID1A was upregulated in 78.8% of stage I–II patients (130 in 165 patients) and 56.1% of stage III–IV patients (23 in 41 patients). In addition, as shown in Figure 7B, ARID1A expression was lost with disease progression (mean score of expression differences between tumor and normal tissue: stage I–II: 2.34; stage III–IV: 1.02; P<0.0001). Survival analysis was then performed. We divided the patients into two groups, including a high-expression difference group and a low-expression group, as mentioned in the methods section. As a result, after the elimination of background interference, ARID1A still had a stage-dependent effect in HCC patients. Although expression differences in ARID1A served as a risk factor for the OS time of HCC patients as shown in Figure 7C, as did ARID1A expression, a stage-dependent role was again demonstrated through survival analysis as shown in Figure 7D,7E. High expression differences in ARID1A were a risk factor among stage I–II HCC patients (666 days vs. NR, P<0.0001) but a protective factor among stage III–IV patients (NR vs. 492 days, P=0.0426).
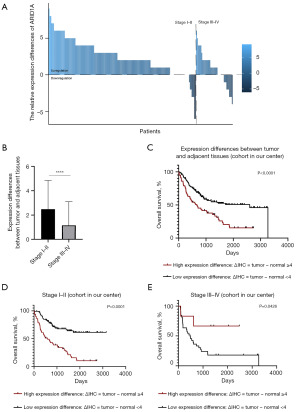
Construction and verification of a prognostic nomogram for stage I–II HCC patients
As shown in Figure 8A, we constructed a nomogram (P<0.0001) with variables, including the expression level of ARID1A and the serum AFP level, selected by the Cox regression model shown in Table 1 to efficaciously predict the OS of 165 stage I–II HCC patients. The C-index of this prognostic model was 0.722. We further scored every involved patient through this model and performed ROC curve analysis to verify the model. As shown in Figure 8B, the ROC curve (P<0.0001) had a satisfactory AUC of 0.80. By determining the best cutoff of the nomogram score with the Youden index, all HCC patients were divided into two groups: a high-risk group and a low-risk group. The nomogram score could efficaciously predict OS, and patients with a high-risk score calculated through the nomogram had a poor prognosis compared with those in the low-risk group (P<0.0001), as shown in Figure 8C. The calibration plots shown in Figure 8D demonstrated that the prognostic model was in agreement with the predicted accuracy on acceptable scales (the dashed lines in the calibration plots correspond to a 10% margin of error) for the 1-year OS of HCC patients but not for the 3-year OS and 5-year OS. Furthermore, we verified the nomogram according to different stages of early-stage HCC patients, as shown in Figure 8E, and the predictive efficiency of this nomogram was robust in both stage I patients (P<0.0001) and stage II patients (P<0.0001). In addition, the ROC curve for the nomogram and involved variables shown in Figure 8F revealed that the model had better efficacy in predicting OS than any single variable selected. Although a variety of variables related to prognosis in stage III patients were explored through the Cox regression model summarized in Table 1, a nomogram for stage III patients was not constructed due to the small sample size.
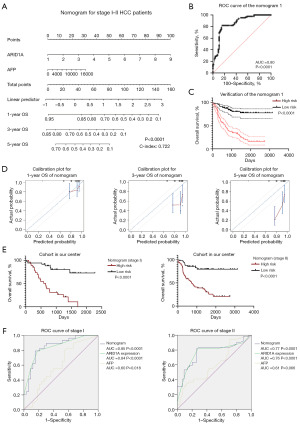
Discussion
HCC is one of the most common causes of cancer-related deaths worldwide and demonstrates insensitivity to multiple therapeutics. Although novel treatment strategies, including immunotherapy and targeted drugs, might currently achieve better responses than they did previously, the majority of HCC patients develop resistance and cannot benefit from these treatments (27). As a result, biomarkers for the prediction of the prognosis of HCC patients are needed. In this study, we found that genomic alterations in ARID family members are common among HCC patients through online databases, especially alterations in ARID1A, ARID2, and ARID1B. Survival analysis and the subsequent subgroup analysis suggested the important role of ARID family members in the prognosis of HCC patients. This result suggested that genomic alterations of ARID family members are all tightly associated with the poor prognosis of HCC patients. This finding is in agreement with previous studies in other types of malignancies such as lung cancer (13,28) and meningioma (29). From the above discussion, the conclusion can be reached that ARID family members are critical in determining the prognosis of malignancies.
According to the latest clinical trials, the atezolizumab plus bevacizumab regimen serves as a better choice for unresectable HCC (30) than sorafenib. Nevertheless, sorafenib still plays a role in the systematic treatment of HCC patients and can be used as the first-line choice for patients with contraindications to atezolizumab and/or bevacizumab (31). Regarding to the limited efficacy of sorafenib treatment, it is necessary to explore the underlying mechanisms of sorafenib resistance. According to a published study (32), activation of the PI3K/Akt signaling pathway serves as a potential mechanism related to acquired resistance to sorafenib. Other mechanism, such as epithelial-mesenchymal transition (EMT) (33) and modification of the tumor microenvironment (34), also play roles in the development of acquired resistance to sorafenib. Presently, few clinical predictors of sorafenib efficacy have been reported, as described in a comprehensive review (35). To explore potential biomarkers for sorafenib efficacy is of major clinical significance to help select the best first-line treatment. In this study, we explored the effect of ARID family genomic alterations on the outcome of sorafenib treatment in HCC. Genomic alterations of ARID family members were found to co-occur with alterations of a series of genes among HCC samples, and the genes with these co-mutations were enriched in the PI3K/Akt signaling pathway. Therefore, we deduced that genomic alterations of ARID family members are possibly associated with the prognosis of HCC patients treated with sorafenib. The subsequent survival analysis verified our hypothesis, and HCC patients harboring ARID family alterations or a single alteration of the ARID1A gene were likely to have a poor prognosis after the administration of sorafenib. However, when comparing our results to those of an older study (36), there was disagreement. A previous study reported that ARID1A deficiency sensitizes tumors to the ANG2 blockade induced by sorafenib treatment. Given that ARID1A alterations usually lead to protein deficiency, further larger studies are needed to address this contradiction. Importantly, due to the high similarity between sorafenib and regorafenib (37), ARID family alterations might also display satisfactory efficacy in predicting the prognosis of patients who receive regorafenib treatment, which warrant further clinical integration.
The specific significance of ARID family genomic alterations, especially alteration of the ARID1A gene, was observed among HCC patients, and we further aimed to clarify the role of ARID1A expression in predicting prognosis. However, our analysis suggested that high expression of ARID1A seemed to be a risk factor for HCC patients in two independent online databases (Kaplan-Meier plotter and the Human Protein Atlas), which was contradictory to the genomic alteration analysis findings (given that ARID1A alterations usually lead to the expression loss of the corresponding protein) (13). Further exploration and verification suggested that early-stage (stage I–II) and late-stage (stage III–IV) HCC patients represented two different groups of patients with significantly different prognoses and suggested that ARID1A possibly plays different roles in different stages of disease, as was also found in previous studies (17,18). To further confirm this phenomenon, a cohort of patients with different HCC stages was employed, and we eventually discovered a stage-dependent role of ARID1A expression. In the early stage of HCC, as described in a previous study (17), we found that ARID1A is a required molecule associated with the development of the disease and was related to the poor prognosis of HCC patients. However, with the progression of the disease, ARID1A loss is found to contribute to the invasion and metastasis of HCC, and highly expressed ARID1A serves as a protective factor in late-stage HCC patients. The same results were acquired after the elimination of the interference of background ARID1A expression. Sun et al. explored the underlying mechanism via a mouse model. High expression of ARID1A upregulates HNF4A’s transcriptional targets, and in addition, HNF4A has been shown to drive CYP450 genes transcription, thereby initiating an oxidative stress response mediating liver injury and hepatocarcinogenesis (17). In the advance stage, loss of ARID1A expression is associated with reduced chromatin accessibility and affects gene expression related to cell motility and metastasis, such as EMILIN1, MAT1A, IL1R1, and LCN2 (38-41). ARID1A shows physical interactions with the promoters of them. Decreased ARID1A expression directly regulates the expression of these genes, thereby promoting tumor metastasis and migration (17). However, ARID1A expression pattern in HCC needs to be further explored. In addition to performing a univariate analysis of ARID1A, we further constructed a nomogram based on ARID1A expression and the serum AFP level for the prediction of OS in involved HCC patients. The prognostic model was found to have satisfactory efficacy in predicting the 1-year OS of HCC patients and was tested by internal verification. The nomogram in this study is a novel and simple method for the clinical evaluation of HCC. In addition, several studies have demonstrated that ARID1A modification of immune activity might influence the response to ICIs and the prognosis of patients treated with ICIs (14,16,42), and further research is needed given the complicated role of ARID1A in HCC.
Conclusions
ARID family members are tightly associated with the prognosis of HCC. Genomic alterations in the ARID family, especially alterations in ARID1A, are biomarkers for the poor prognosis of HCC and contribute to the failure of sorafenib treatment. ARID1A expression has a stage-dependent effect on the prognosis of HCC. It serves as a risk factor for stage I–II patients but a protective factor for stage III–IV patients. The novel nomogram generated in this study could be used for the prognostic evaluation of HCC patients.
Acknowledgments
We kindly acknowledge the support of Prof. Jinjun Li of Shanghai Cancer Institute.
Funding: This work was funded by the National Natural Science Foundation of China (No. 81602113 to Helei Hou), the Natural Science Foundation of Shanghai (No. 20ZR1454000 to Hong Li), the Special Funding for Qilu Sanitation and Health Leading Talents Cultivation Project (to Helei Hou), and the Key Discipline and Specialty Foundation of Shanghai Municipal Commission of Health and Family Planning (No. 2018BR20 to Hong Li).
Footnote
Reporting Checklist: The authors have completed the TRIPOD reporting checklist. Available at https://tcr.amegroups.com/article/view/10.21037/tcr-23-645/rc
Data Sharing Statement: Available at https://tcr.amegroups.com/article/view/10.21037/tcr-23-645/dss
Peer Review File: Available at https://tcr.amegroups.com/article/view/10.21037/tcr-23-645/prf
Conflicts of Interest: All authors have completed the ICMJE uniform disclosure form (available at https://tcr.amegroups.com/article/view/10.21037/tcr-23-645/coif). The authors have no conflicts of interest to declare.
Ethical Statement: The authors are accountable for all aspects of the work in ensuring that questions related to the accuracy or integrity of any part of the work are appropriately investigated and resolved. The study was conducted in accordance with the Declaration of Helsinki (as revised in 2013). The study was approved by the Ethics Committee of Qidong Liver Cancer Institute (No. QDRMYY-003) and Shanghai Jiao Tong University School of Medicine (No. RA-2019-250). Informed consents were taken from all patients.
Open Access Statement: This is an Open Access article distributed in accordance with the Creative Commons Attribution-NonCommercial-NoDerivs 4.0 International License (CC BY-NC-ND 4.0), which permits the non-commercial replication and distribution of the article with the strict proviso that no changes or edits are made and the original work is properly cited (including links to both the formal publication through the relevant DOI and the license). See: https://creativecommons.org/licenses/by-nc-nd/4.0/.
References
- Hou J, Ge C, Cui M, et al. Pigment epithelium-derived factor promotes tumor metastasis through an interaction with laminin receptor in hepatocellular carcinomas. Cell Death Dis 2017;8:e2969. [Crossref] [PubMed]
- Chen W, Zheng R, Baade PD, et al. Cancer statistics in China, 2015. CA Cancer J Clin 2016;66:115-32. [Crossref] [PubMed]
- Llovet JM, Montal R, Sia D, et al. Molecular therapies and precision medicine for hepatocellular carcinoma. Nat Rev Clin Oncol 2018;15:599-616. [Crossref] [PubMed]
- Llovet JM, Peña CE, Lathia CD, et al. Plasma biomarkers as predictors of outcome in patients with advanced hepatocellular carcinoma. Clin Cancer Res 2012;18:2290-300. [Crossref] [PubMed]
- Johnson P, Zhou Q, Dao DY, et al. Circulating biomarkers in the diagnosis and management of hepatocellular carcinoma. Nat Rev Gastroenterol Hepatol 2022;19:670-81. [Crossref] [PubMed]
- Ho WJ, Zhu Q, Durham J, et al. Neoadjuvant Cabozantinib and Nivolumab Converts Locally Advanced HCC into Resectable Disease with Enhanced Antitumor Immunity. Nat Cancer 2021;2:891-903. [Crossref] [PubMed]
- Chen KY, Popovic A, Hsiehchen D, et al. Clinical Outcomes in Fibrolamellar Hepatocellular Carcinoma Treated with Immune Checkpoint Inhibitors. Cancers (Basel) 2022;14:5347. [Crossref] [PubMed]
- Mi H, Sivagnanam S, Betts CB, et al. Quantitative Spatial Profiling of Immune Populations in Pancreatic Ductal Adenocarcinoma Reveals Tumor Microenvironment Heterogeneity and Prognostic Biomarkers. Cancer Res 2022;82:4359-72. [Crossref] [PubMed]
- Zhu J, Wu Y, Yu Y, et al. MYBL1 induces transcriptional activation of ANGPT2 to promote tumor angiogenesis and confer sorafenib resistance in human hepatocellular carcinoma. Cell Death Dis 2022;13:727. [Crossref] [PubMed]
- Granito A, Muratori L, Lalanne C, et al. Hepatocellular carcinoma in viral and autoimmune liver diseases: Role of CD4+ CD25+ Foxp3+ regulatory T cells in the immune microenvironment. World J Gastroenterol 2021;27:2994-3009. [Crossref] [PubMed]
- Mullen J, Kato S, Sicklick JK, et al. Targeting ARID1A mutations in cancer. Cancer Treat Rev 2021;100:102287. [Crossref] [PubMed]
- Mittal P, Roberts CWM. The SWI/SNF complex in cancer - biology, biomarkers and therapy. Nat Rev Clin Oncol 2020;17:435-48. [Crossref] [PubMed]
- Sun D, Zhu Y, Zhao H, et al. Loss of ARID1A expression promotes lung adenocarcinoma metastasis and predicts a poor prognosis. Cell Oncol (Dordr) 2021;44:1019-34. [Crossref] [PubMed]
- Sun D, Tian L, Zhu Y, et al. Subunits of ARID1 serve as novel biomarkers for the sensitivity to immune checkpoint inhibitors and prognosis of advanced non-small cell lung cancer. Mol Med 2020;26:78. [Crossref] [PubMed]
- He F, Li J, Xu J, et al. Decreased expression of ARID1A associates with poor prognosis and promotes metastases of hepatocellular carcinoma. J Exp Clin Cancer Res 2015;34:47. [Crossref] [PubMed]
- Yim SY, Kang SH, Shin JH, et al. Low ARID1A Expression is Associated with Poor Prognosis in Hepatocellular Carcinoma. Cells 2020;9:2002. [Crossref] [PubMed]
- Sun X, Wang SC, Wei Y, et al. Arid1a Has Context-Dependent Oncogenic and Tumor Suppressor Functions in Liver Cancer. Cancer Cell 2017;32:574-589.e6. [Crossref] [PubMed]
- Otto JE, Kadoch C. A Two-Faced mSWI/SNF Subunit: Dual Roles for ARID1A in Tumor Suppression and Oncogenicity in the Liver. Cancer Cell 2017;32:542-3. [Crossref] [PubMed]
- Bandos AI, Rockette HE, Song T, et al. Area under the free-response ROC curve (FROC) and a related summary index. Biometrics 2009;65:247-56. [Crossref] [PubMed]
- Jin C, Cao J, Cai Y, et al. A nomogram for predicting the risk of invasive pulmonary adenocarcinoma for patients with solitary peripheral subsolid nodules. J Thorac Cardiovasc Surg 2017;153:462-469.e1. [Crossref] [PubMed]
- Gao J, Aksoy BA, Dogrusoz U, et al. Integrative analysis of complex cancer genomics and clinical profiles using the cBioPortal. Sci Signal 2013;6:pl1. [Crossref] [PubMed]
- Cerami E, Gao J, Dogrusoz U, et al. The cBio cancer genomics portal: an open platform for exploring multidimensional cancer genomics data. Cancer Discov 2012;2:401-4. [Crossref] [PubMed]
- Menyhárt O, Nagy Á, Győrffy B. Determining consistent prognostic biomarkers of overall survival and vascular invasion in hepatocellular carcinoma. R Soc Open Sci 2018;5:181006. [Crossref] [PubMed]
- Zhou Y, Zhou B, Pache L, et al. Metascape provides a biologist-oriented resource for the analysis of systems-level datasets. Nat Commun 2019;10:1523. [Crossref] [PubMed]
- Xie Y, Liu C, Zhang Y, et al. PKI-587 enhances radiosensitization of hepatocellular carcinoma by inhibiting the PI3K/AKT/mTOR pathways and DNA damage repair. PLoS One 2021;16:e0258817. [Crossref] [PubMed]
- Wu B, Li A, Zhang Y, et al. Resistance of hepatocellular carcinoma to sorafenib can be overcome with co-delivery of PI3K/mTOR inhibitor BEZ235 and sorafenib in nanoparticles. Expert Opin Drug Deliv 2020;17:573-87. [Crossref] [PubMed]
- Llovet JM, Castet F, Heikenwalder M, et al. Immunotherapies for hepatocellular carcinoma. Nat Rev Clin Oncol 2022;19:151-72. [Crossref] [PubMed]
- Zhang Y, Xu X, Zhang M, et al. ARID1A is downregulated in non-small cell lung cancer and regulates cell proliferation and apoptosis. Tumour Biol 2014;35:5701-7. [Crossref] [PubMed]
- Gill CM, Loewenstern J, Rutland JW, et al. SWI/SNF chromatin remodeling complex alterations in meningioma. J Cancer Res Clin Oncol 2021;147:3431-40. [Crossref] [PubMed]
- Galle PR, Finn RS, Qin S, et al. Patient-reported outcomes with atezolizumab plus bevacizumab versus sorafenib in patients with unresectable hepatocellular carcinoma (IMbrave150): an open-label, randomised, phase 3 trial. Lancet Oncol 2021;22:991-1001. [Crossref] [PubMed]
- Gordan JD, Kennedy EB, Abou-Alfa GK, et al. Systemic Therapy for Advanced Hepatocellular Carcinoma: ASCO Guideline. J Clin Oncol 2020;38:4317-45. [Crossref] [PubMed]
- Cao W, Liu X, Zhang Y, et al. BEZ235 Increases the Sensitivity of Hepatocellular Carcinoma to Sorafenib by Inhibiting PI3K/AKT/mTOR and Inducing Autophagy. Biomed Res Int 2021;2021:5556306. [Crossref] [PubMed]
- Xia S, Pan Y, Liang Y, et al. The microenvironmental and metabolic aspects of sorafenib resistance in hepatocellular carcinoma. EBioMedicine 2020;51:102610. [Crossref] [PubMed]
- Tian X, Yan T, Liu F, et al. Link of sorafenib resistance with the tumor microenvironment in hepatocellular carcinoma: Mechanistic insights. Front Pharmacol 2022;13:991052. [Crossref] [PubMed]
- Song S, Bai M, Li X, et al. Early predictive value of circulating biomarkers for sorafenib in advanced hepatocellular carcinoma. Expert Rev Mol Diagn 2022;22:361-78. [Crossref] [PubMed]
- Hu C, Li W, Tian F, et al. Arid1a regulates response to anti-angiogenic therapy in advanced hepatocellular carcinoma. J Hepatol 2018;68:465-75. [Crossref] [PubMed]
- Granito A, Forgione A, Marinelli S, et al. Experience with regorafenib in the treatment of hepatocellular carcinoma. Therap Adv Gastroenterol 2021;14:17562848211016959. [Crossref] [PubMed]
- Dagenais M, Dupaul-Chicoine J, Douglas T, et al. The Interleukin (IL)-1R1 pathway is a critical negative regulator of PyMT-mediated mammary tumorigenesis and pulmonary metastasis. Oncoimmunology 2017;6:e1287247. [Crossref] [PubMed]
- Danussi C, Petrucco A, Wassermann B, et al. An EMILIN1-negative microenvironment promotes tumor cell proliferation and lymph node invasion. Cancer Prev Res (Phila) 2012;5:1131-43. [Crossref] [PubMed]
- Wang YP, Yu GR, Lee MJ, et al. Lipocalin-2 negatively modulates the epithelial-to-mesenchymal transition in hepatocellular carcinoma through the epidermal growth factor (TGF-beta1)/Lcn2/Twist1 pathway. Hepatology 2013;58:1349-61. [Crossref] [PubMed]
- Zhang J, Gong C, Bing Y, et al. Hypermethylation-repressed methionine adenosyltransferase 1A as a potential biomarker for hepatocellular carcinoma. Hepatol Res 2013;43:374-83. [Crossref] [PubMed]
- Li L, Rao X, Wen Z, et al. Implications of driver genes associated with a high tumor mutation burden identified using next-generation sequencing on immunotherapy in hepatocellular carcinoma. Oncol Lett 2020;19:2739-48. [Crossref] [PubMed]